Hello everyone, I’m Mu Yi, an internet technology product manager who continuously focuses on the AI field, with a top 2 undergraduate degree in China, a top 10 CS graduate degree in the US, and an MBA. I firmly believe that AI is the “power-up” for ordinary people, which is why I created the WeChat public account “AI Information Gap”, focusing on sharing comprehensive knowledge about AI, including but not limited to AI popular science, AI tool evaluation, AI efficiency improvement, and AI industry insights. Follow me, and you won’t get lost on the road to AI; let’s become stronger together in 2024.
AI Agent has recently become a hot topic. I have previously written several articles introducing the applications of AI Agents, but I haven’t delved into the technical details behind them. Today, this article will discuss the story behind AI Agents. This is an article based on a paper titled “The Landscape of Emerging AI Agent Architectures for Reasoning, Planning, and Tool Calling: A Survey” published by researchers from IBM and Microsoft in April 2024, which investigates and analyzes the current development landscape of AI Agent architectures, focusing on the reasoning, planning, and tool-calling capabilities required for AI Agents to achieve complex goals.
Streamlined Overview
AI Agent technology is gradually becoming an indispensable force in solving complex tasks, particularly playing a key role in reasoning, planning, and tool calling. In different application scenarios, single-agent and multi-agent systems each exhibit unique advantages. Single-agent systems perform excellently in cases where task definitions are clear and dependence on external feedback is low, while multi-agent systems show their unique strengths in environments requiring multi-party collaboration and multi-path parallel processing of tasks, although they are more challenging to implement. To enhance the effectiveness of AI Agent systems, meticulous personalization of the agents must be conducted, equipping them with suitable tools and empowering them with strong reasoning capabilities. Additionally, clear role definitions, dynamic team building, and efficient information sharing mechanisms are other critical factors for improving Agent system performance.
Currently, research on AI Agent systems still faces several challenges and limitations. Inconsistencies in evaluation standards, adaptability to real-world application scenarios, and inherent biases in language models are issues that need to be prioritized in current research. Future studies may focus on establishing a more comprehensive and objective evaluation system to improve the reliability and robustness of AI Agent systems in real-world scenarios, and explore effective methods to reduce system biases. Although current AI Agent systems are not yet fully matured, their capabilities in handling reasoning, planning, and tool usage have already surpassed traditional static language models. With ongoing technological advancements, AI Agents are expected to play a key role in more fields, becoming an important link in driving the development of AI applications.
Below is an industry panorama titled “AI Agents Landscape” from E2B.
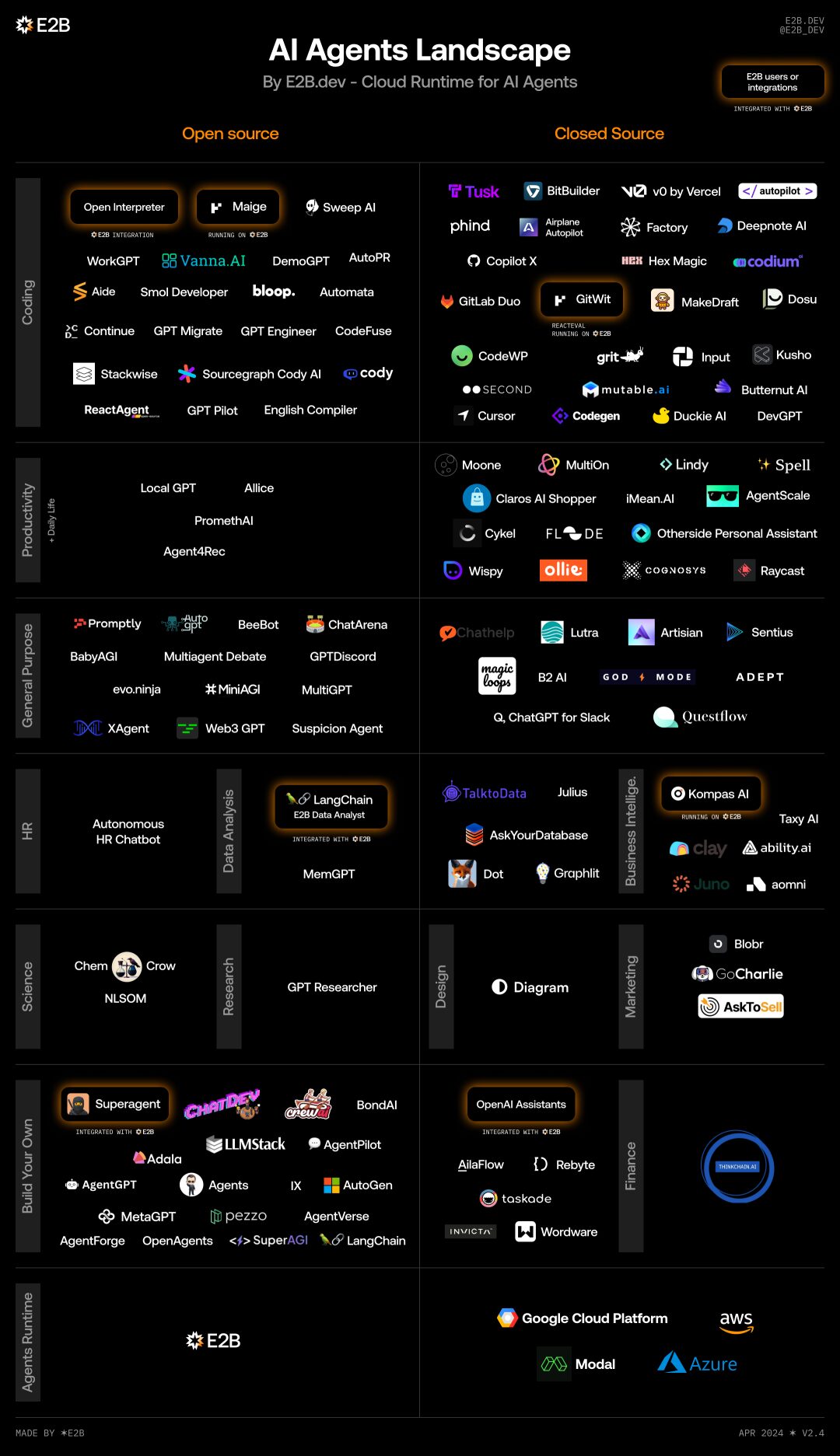
Background Introduction
Since the release of ChatGPT, the first wave of generative AI applications has mostly been based on the “Retrieval Augmented Generation” (RAG) model, interacting with document corpuses through chat interfaces. Although many studies are currently focused on improving the robustness of RAG systems, researchers are also actively exploring the development of the next generation of AI applications, with AI Agent technology being a common focal point.
Compared to the zero-shot prompting of traditional large language models (LLMs), AI Agents allow users to engage in more complex interactions and task coordination. AI Agent systems are equipped with control mechanisms such as planning, iteration, and reflection, which fully leverage the model’s inherent reasoning capabilities to achieve end-to-end processing from task initiation to completion. Moreover, AI Agents can call tools, plugins, and execute functions, enabling them to handle a wider range of general tasks.
Currently, there is some debate in academia regarding whether single-agent systems or multi-agent systems are more effective in solving complex tasks. Single-agent architectures demonstrate their advantages when dealing with tasks that are clearly defined and do not require additional agent roles or user feedback. Conversely, multi-agent architectures exhibit their unique strengths in situations requiring multi-party collaboration and the ability to take multiple execution paths.
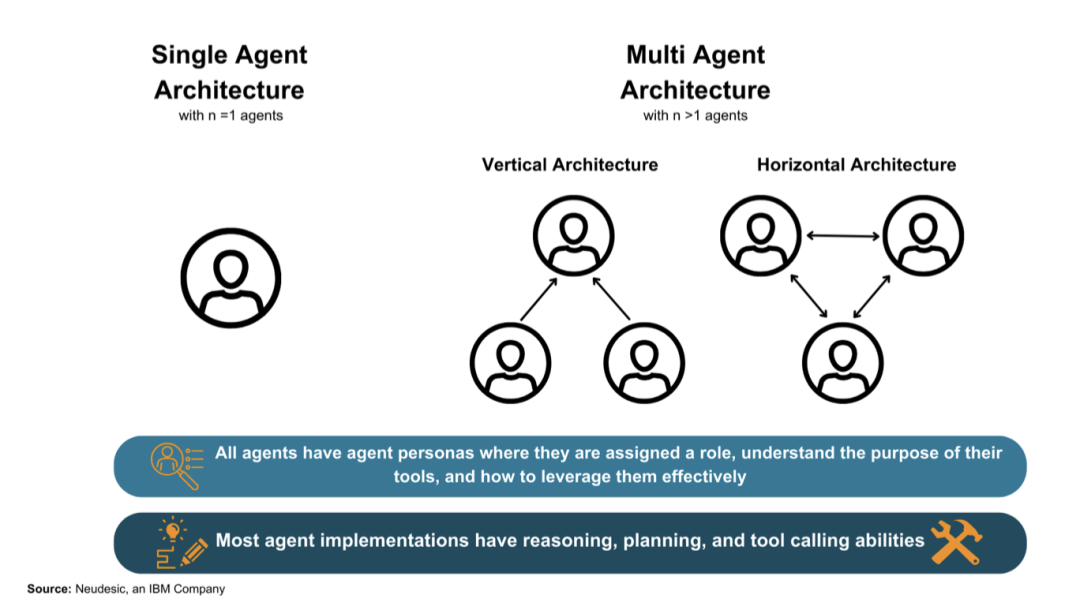
Classification of AI Agents
Agents: AI Agents are entities driven by language models that can plan and take actions through multiple iterations to achieve goals. Agent architectures can consist of a single agent or multiple agents working together to solve problems.
Typically, each agent is assigned a role persona and granted access to various tools that will assist them in completing tasks independently or as part of a team. Some agents also include a memory component that can save and load information beyond messages and prompts. In this article, we follow the definition of agents that includes three parts: “brain, perception, and action.” These components meet the minimum requirements for agents to understand, reason, and take action in their environment.
[Extended Insights]
Previously, I introduced several agent/platforms such as ChatGPT’s GPT Store (GPTs), ByteDance’s Coze and Button, Kimi+ from Dark Moon, and Baidu’s Wenxin Agent platform. These platforms initially followed a single-agent route, where a single independent agent completed a specific task. Later, GPTs and Kimi+ introduced the functionality of using other agents in the conversation by using @
, which represents the current prototype of multi-agent systems.
Agent Persona: The agent persona describes the role or personality the agent should play, including any specific instructions related to that agent. The persona also includes descriptions of any tools the agent can access. They make the agent aware of its role, the purpose of the tools, and how to use them effectively. Researchers have found that “shaped personalities do indeed affect the behavior of large language models (LLMs) in common downstream tasks (e.g., writing social media posts).” Compared to chain-of-thought (CoT) that allows the model to decompose its plans step by step, using multiple agent roles to solve problems has also shown significant improvements.
Tools: In the context of AI agents, tools refer to any functions that the model can call upon. They allow agents to interact with external data sources by pulling or pushing information. For example, in the case of a professional contract drafting agent, the agent is assigned a clear persona that specifies the role of the drafter and the various tasks it needs to complete. Additionally, to assist the contract drafting agent in working more efficiently, a range of tools has been equipped. These tools include, but are not limited to: the ability to annotate documents, read and understand existing document content, and send emails containing the final draft. With these tools, the agent can be more flexible and professional when executing contract drafting tasks.
[Extended Insights]
When creating personalized agents in GPTs or Coze/Button, you can customize the plugins that the agent needs to enhance its capabilities. These plugins are the tools mentioned above.
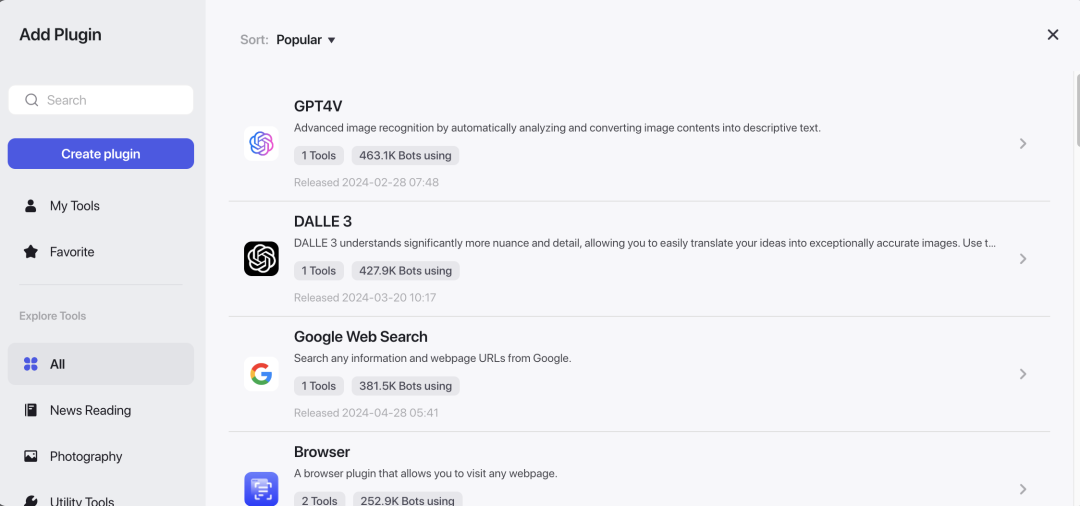
Single-Agent Architectures: These architectures are driven by a language model, which independently completes all reasoning, planning, and tool execution. The agent is assigned a system prompt and any tools necessary to complete its tasks. In single-agent mode, there is no feedback mechanism from other AI agents; however, human feedback may be provided to guide the agent’s options.
Multi-Agent Architectures: These architectures involve two or more agents, each of which may use the same language model or a set of different language models. Agents may have access to the same tools or different tools. Each agent typically has its own role persona.
Multi-agent architectures can have a wide variety of organizational forms at any level of complexity. In this paper, the researchers categorize them into two main categories: vertical and horizontal. It is worth noting that these categories represent the ends of a spectrum, with most existing architectures lying between these two extremes.
Vertical Architectures: In this structure, one agent acts as a leader, and other agents report directly to it. Depending on the architecture, the reporting agents may only communicate with the leading agent, or the leader can be defined as the shared dialogue among all agents. The defining characteristics of vertical architectures include having a leading agent and clear division of labor.
Horizontal Architectures: In this structure, all agents are treated as equals and are part of a group discussion about the task. Communication among agents occurs in a shared thread, where each agent can see all messages from other agents. Agents can also voluntarily complete specific tasks or call tools, meaning they do not need to be assigned by a leading agent. Horizontal architectures are often used for tasks where collaboration, feedback, and group discussions are crucial for overall task success.
Key Considerations: Building Effective AI Agents
AI Agents aim to extend the capabilities of language models to solve real-world tasks. Successful implementation requires strong problem-solving abilities, enabling agents to excel in new tasks. To this end, agents need to possess reasoning and planning capabilities, as well as the ability to call tools that interact with the external environment.
The Importance of Reasoning and Planning
Reasoning is the foundation of human cognition, giving us the ability to make decisions, solve problems, and understand the world around us. For AI Agents to interact effectively in complex environments, make autonomous decisions, and assist humans in a variety of tasks, strong reasoning capabilities are essential. This close integration of “action” and “reasoning” not only allows agents to quickly grasp new tasks but also enables them to make robust decisions and reasoning even when faced with unknown situations or unclear information. Moreover, agents rely on their reasoning abilities to adjust their plans based on new feedback or learned information. Agents lacking reasoning capabilities may misunderstand instructions, respond only based on literal meanings, or fail to foresee the consequences of multi-step actions when executing direct tasks.
Planning is another important application of reasoning capabilities, typically involving five main approaches: task decomposition, multi-plan selection, external module-assisted planning, reflection and improvement, and memory-enhanced planning. These methods enable agents to break complex tasks into subtasks, choose from multiple candidate plans, utilize existing external plans, update old plans based on new information, or optimize plans with external information. Most agent models have a dedicated planning step before taking action, employing one or more of these techniques to formulate action plans. For instance, the “Plan Like a Graph” (PLaG) method treats planning as a directed graph, allowing multiple steps to be executed in parallel, significantly enhancing performance when dealing with complex problems involving many independent subtasks compared to other methods.
The Importance of Effectively Calling Tools
Compared to direct prompts of base language models, the abstract level of AI Agents is more crucially reflected in their ability to call various tools to solve complex problems. These tools empower agents to interact with external data sources, such as sending or retrieving information via APIs. Typically, problems that require extensive tool calls are often accompanied by a demand for complex reasoning.
Whether in single-agent or multi-agent architectures, challenging tasks can be addressed by combining reasoning and tool-calling steps. When solving these problems, agents often need to undergo multiple iterations of reasoning, memory, and reflection to ensure the effectiveness and accuracy of problem-solving. They break large problems into a series of smaller sub-problems, then sequentially use appropriate tools to solve each sub-problem. Moreover, multi-agent modes are particularly adept at handling complex tasks that require parallel processing and high robustness, as each agent can independently tackle its sub-problem. In multi-agent modes, a complex large problem is typically first decomposed into several smaller tasks, with each agent utilizing its independent toolset to resolve these tasks. This approach not only improves the efficiency of problem-solving but also enhances the flexibility and robustness of the system.
Single-Agent Architectures
For single agents, each method has a dedicated reasoning phase for the problem before taking action. Researchers have chosen several single-agent architectures for discussion based on the reasoning and tool-calling capabilities of agents: ReAct, RAISE, Reflexion, AutoGPT + P, and LATS.
Research has found that the successful execution of goal tasks by agents depends on appropriate planning and self-correction. Without the ability to self-assess and formulate effective plans, single agents may become trapped in endless execution loops, failing to complete assigned tasks or returning results that do not meet user expectations. Single-agent architectures are particularly useful when tasks require direct method calls and do not need feedback from another agent.
ReAct
The ReAct (Reasoning + Action) method is a process that allows agents to first form thoughts regarding a specific task, then execute actions based on those thoughts and observe the results. This method has demonstrated higher effectiveness compared to traditional zero-shot prompting in diverse language and decision-making tasks, as the entire thought process of the model is recorded, thereby enhancing the model’s credibility and human interoperability. In evaluations on the HotpotQA dataset, the hallucination rate of the ReAct method was only 6%, while the hallucination rate of the chain-of-thought (CoT) method was 14%.
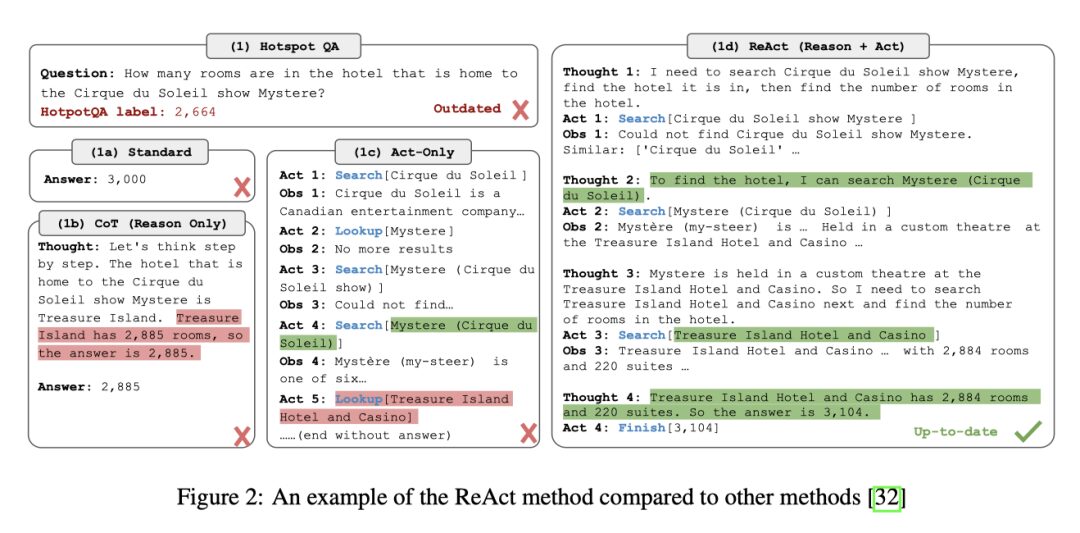
However, the ReAct method also has its limitations, especially in scenarios where the model may repeatedly generate the same ideas and actions without producing new ideas to complete the tasks. Introducing human feedback during task execution may enhance its effectiveness and improve its applicability in real-world scenarios.
RAISE
The RAISE method builds on the ReAct method by adding a memory mechanism that simulates human short-term and long-term memory, utilizing temporary storage and past similar case datasets for long-term storage. The addition of these components enhances the agent’s ability to maintain context in longer dialogues, and through fine-tuning the model, even smaller models can achieve optimal performance, demonstrating advantages in efficiency and output quality over ReAct. However, RAISE faces challenges in understanding complex logic, limiting its practicality in various scenarios. Additionally, RAISE agents often exhibit hallucinations regarding their roles or knowledge; for instance, a sales agent without a clear role definition might start writing Python code instead of focusing on its sales tasks, and may sometimes provide misleading or incorrect information to users. Although fine-tuning the model has addressed some issues, hallucinations remain a limitation of RAISE implementations.
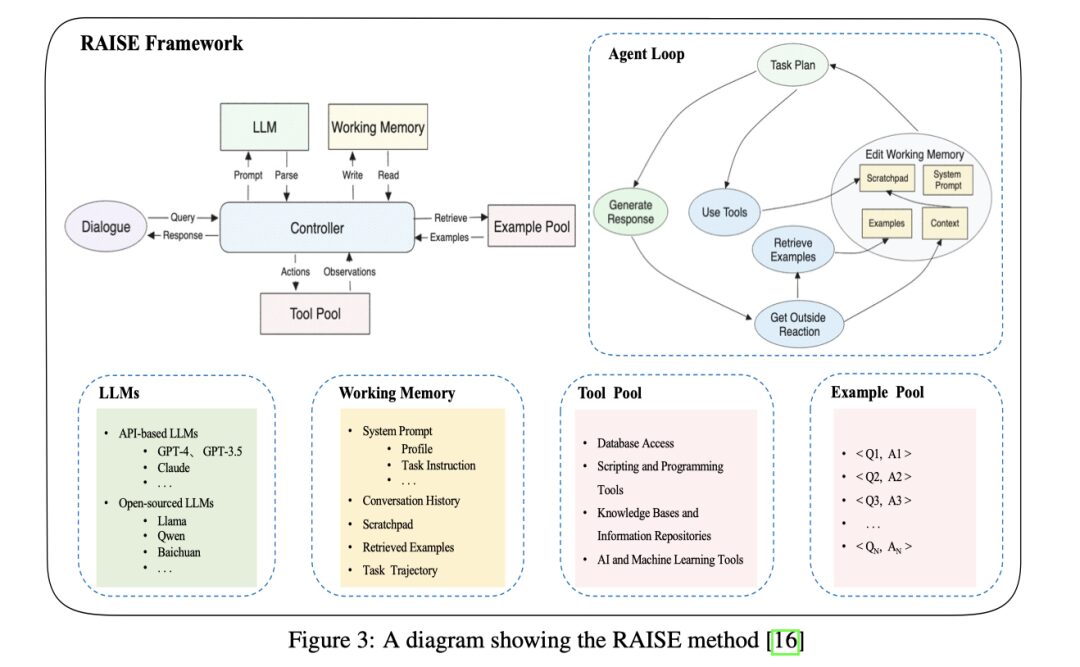
Reflexion
Reflexion is a single-agent mode that achieves self-reflection through language feedback, utilizing indicators such as successful states, current trajectories, and persistent memory, combined with a large language model evaluator to provide specific feedback to the agent, thereby improving success rates and reducing hallucinations compared to chain-of-thought (CoT) and ReAct methods. Despite some progress made by Reflexion, it also has limitations, including a tendency to get stuck in non-optimal local minima and the use of sliding windows rather than databases to handle long-term memory, which limits the capacity of long-term memory. Furthermore, while Reflexion outperforms other single-agent modes in terms of performance, there is still room for improvement in tasks requiring high diversity, exploration, and reasoning.
AutoGPT + P
AutoGPT + P (Planning) is a method aimed at addressing the reasoning limitations of agents commanded in natural language to control robots. It combines object detection, Object Availability Mapping (OAM), and a planning system driven by large language models, enabling agents to explore missing objects in the environment, propose alternatives, or request user assistance to achieve goals. AutoGPT + P first utilizes scene images to detect objects, then the language model selects from four tools based on these objects: planning tools, partial planning tools, suggestion alternative tools, and exploration tools. These tools not only allow the robot to generate a complete plan to achieve goals but also enable exploration of the environment, hypothesis generation, and creation of partial plans.
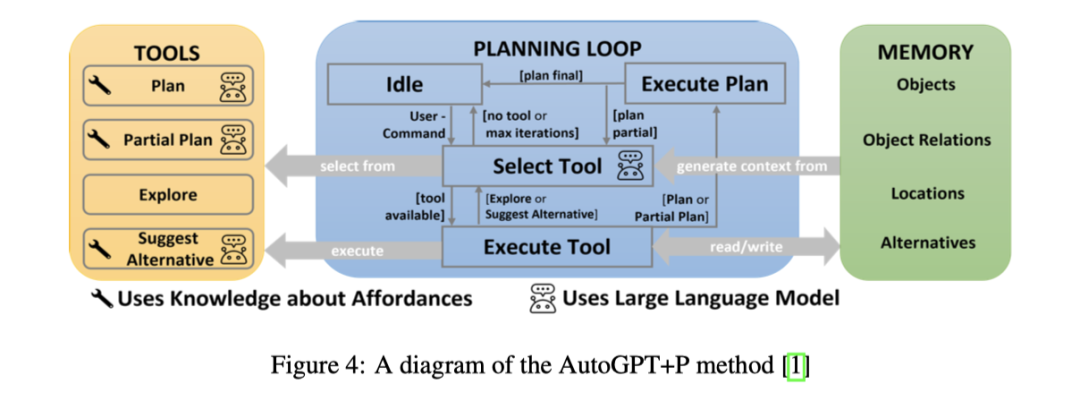
However, the language model does not independently generate plans. It collaborates with a classical planner to generate goals and steps, which executes plans using Planning Domain Definition Language (PDDL). Research has found that large language models currently cannot directly convert natural language instructions into plans for executing robotic tasks, primarily due to their limited reasoning capabilities. By combining the planning capabilities of LLMs with classical planners, this method significantly improves upon other purely language model-based robotic planning methods. Nonetheless, AutoGPT + P, as an emerging method, has some drawbacks, including varying accuracy in tool selection, which may sometimes lead to inappropriate tool calls or looping. In exploratory scenarios, tool selection may sometimes result in illogical exploration decisions, such as searching for objects in the wrong places. Additionally, the framework has limitations in human interaction, as agents cannot seek clarification, and users cannot modify or terminate plans during execution.
LATS
Language Agent Tree Search (LATS) is a single-agent architecture that coordinates planning, action, and reasoning through the use of tree structures. This technique is inspired by Monte Carlo Tree Search, representing states as nodes while actions are seen as traversals between nodes. LATS employs a language model-based heuristic approach to search for possible options, then utilizes a state evaluator to select actions. Compared to other tree-based methods, LATS incorporates self-reflective reasoning steps, significantly enhancing performance. After taking action, LATS not only utilizes environmental feedback but also combines feedback from the language model to determine if errors exist in reasoning and propose alternatives. This ability for self-reflection, combined with its powerful search algorithm, allows LATS to perform excellently across various tasks.
However, due to the complexity of the algorithm itself and the reflective steps involved, LATS typically consumes more computational resources than other single-agent methods and takes longer to complete tasks. Additionally, while LATS performs well in relatively simple question-answer benchmark tests, it has yet to be tested and validated in more complex scenarios involving tool calls or intricate reasoning. This indicates that while LATS has strong theoretical potential, it may require further adjustments and optimizations for practical applications.
Multi-Agent Architectures
Multi-agent architectures achieve goals through communication and collaborative planning execution among agents, providing opportunities for skill-based agent division and beneficial feedback from diverse agent roles. These architectures typically work in phases, with agent teams dynamically creating and reorganizing during each planning, execution, and evaluation phase. This reorganization achieves better outcomes by assigning specialized agents for specific tasks and removing them when no longer needed. By matching agents’ roles and skills with the tasks at hand, agent teams can improve accuracy and reduce the time taken to achieve goals. Key characteristics of effective multi-agent architectures include clear leadership within the agent team, dynamic team building, and effective information sharing among team members to ensure that important information is not lost in irrelevant chatter.
In the context of multi-agent systems, research and frameworks (such as “Embodied LLM Agents Learn to Cooperate in Organized Teams”, DyLAN, AgentVerse, and MetaGPT) demonstrate how collaboration between agents can facilitate goal execution. These methods aim to cover key themes and examples related to multi-agent modes extensively. Through collaboration and role allocation among agents, these architectures can handle complex tasks more efficiently while reducing the time required to complete tasks and improving overall accuracy.
Embodied LLM Agents Learn to Cooperate in Organized Teams
Another study by Xudong Guo et al. found that having a leading agent is crucial for enhancing the performance of multi-agent architectures. This architecture achieves vertical management by setting a leading agent while allowing horizontal communication among agents, meaning that in addition to communicating with the leading agent, agents can also exchange information directly with each other. The study found that organized teams of agents with a leader completed tasks nearly 10% faster than teams without a leader. Moreover, they discovered that in teams without designated leaders, agents spent most of their time giving each other instructions (about 50% of communications), with the remaining time spent sharing information or requesting guidance. In contrast, in teams with designated leaders, 60% of the leader’s communication involved giving directions, encouraging other members to focus more on exchanging and requesting information. The results indicate that when the leader is human, the agent team is most effective.
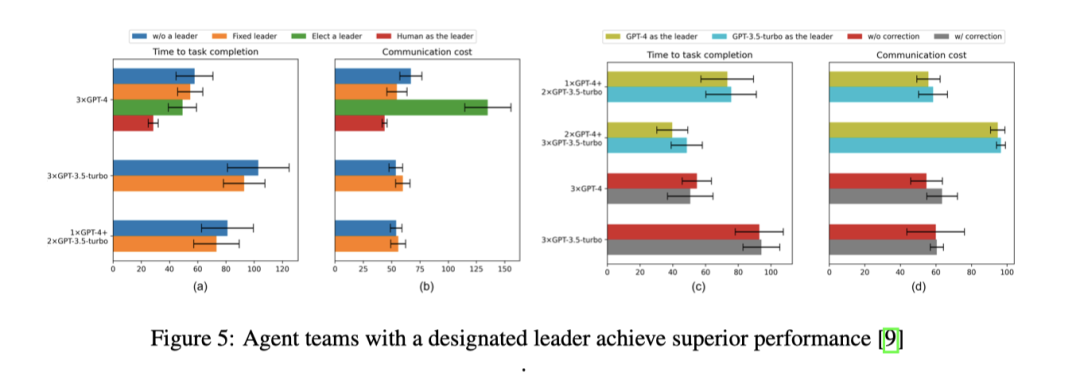
In addition to team structure, the research emphasizes the importance of adopting a “critique-reflection” step for generating plans, evaluating performance, providing feedback, and reorganizing teams. Their results indicate that agent teams with dynamic team structures and rotating leadership provide the best results in terms of task completion time and average communication costs. Ultimately, leadership and dynamic team structures enhance the overall reasoning, planning, and execution capabilities of the team.
DyLAN
DyLAN (Dynamic LLM-Agent Network) framework constructs a dynamic agent structure focused on complex tasks such as reasoning and code generation. This framework achieves a horizontal working mode by evaluating each agent’s contribution in the previous round of work and only bringing the most contributing agents into the next round of execution, allowing agents to share information with each other without a clear leader. DyLAN has demonstrated improved performance on various benchmarks measuring arithmetic and general reasoning abilities, highlighting the importance of dynamic teams and proving that continuously reassessing and ranking agents’ contributions can build multi-agent teams better suited to complete specific tasks.
AgentVerse
AgentVerse is a multi-agent architecture that enhances the reasoning and problem-solving capabilities of AI Agents through explicit team planning. It divides task execution into four main phases: recruitment, collaborative decision-making, independent action execution, and evaluation, which can be repeated based on the progress of the goals until the final objectives are achieved. By strictly defining each phase, AgentVerse guides agent sets to conduct reasoning, discussion, and execution more effectively.
For example, during the recruitment phase, agents may be added or removed based on the progress of the goals, ensuring that the right agents are involved at any stage of problem-solving. Researchers have found that horizontal teams are typically better suited for collaborative tasks, such as consulting, while vertical teams are more suitable for tasks requiring clear division of responsibilities and tool calls. This structured approach allows AgentVerse to effectively guide agent teams to adapt to the needs of different tasks, thereby improving overall execution efficiency and problem-solving capabilities.
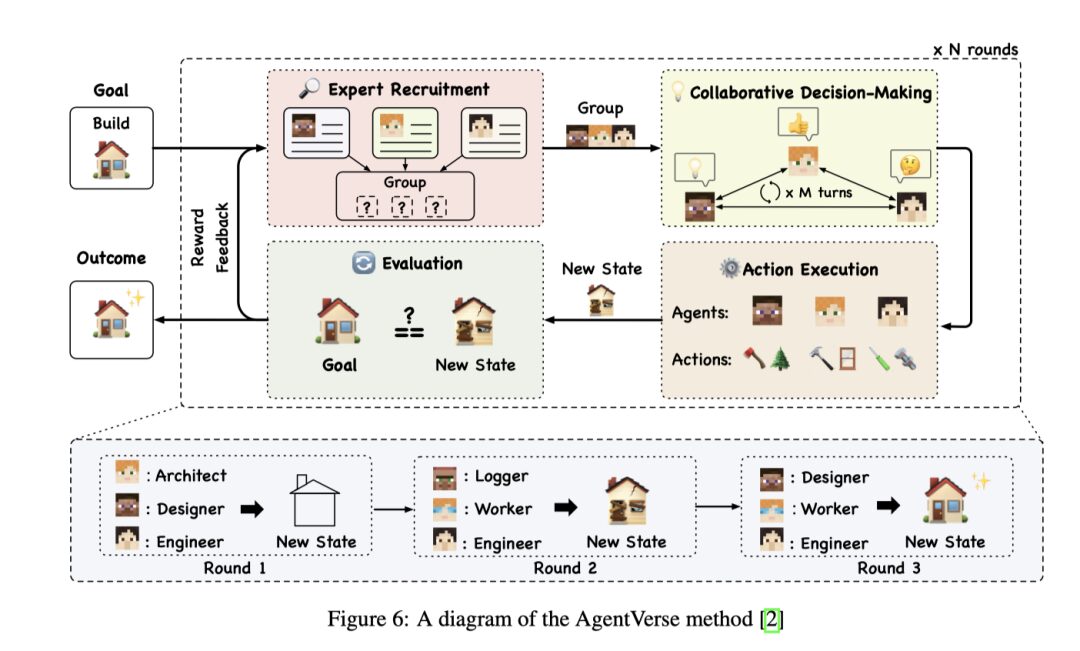
MetaGPT
MetaGPT is a multi-agent architecture designed to address the ineffective communication issues that agents may encounter when solving problems together. In many multi-agent systems, dialogues among agents may lead to irrelevant chit-chat, which does not help advance task objectives. MetaGPT addresses this by requiring agents to generate structured outputs, such as documents and charts, rather than exchanging unstructured chat logs.
Additionally, MetaGPT implements a “publish-subscribe” information-sharing mechanism. This mechanism allows all agents to share information in one place but only read information relevant to their respective goals and tasks. This not only simplifies the overall process of executing goals but also reduces communication noise among agents. In benchmark tests like HumanEval and MBPP, MetaGPT’s multi-agent architecture has shown significantly better performance compared to single-agent architectures.
Core Findings
Whether in single-agent or multi-agent architectures, their goal is to enhance the functionality of language models, enabling them to execute tasks independently or in collaboration with human users. Current implementations of most agents follow a process of planning, action, and evaluation to iteratively solve problems. Research has found that both single-agent and multi-agent architectures demonstrate remarkable performance in executing complex goals. Among various architectures, clear feedback, task decomposition, iterative refinement, and role definitions can enhance agent performance.
Single-Agent VS Multi-Agent
In terms of typical conditions for choosing between single-agent and multi-agent architectures, research has found that single-agent modes are generally best suited for tasks with limited tool lists and clearly defined processes. Single-agent architectures are not only relatively simple to implement, as they only require defining one agent and a set of tools, but they also do not face negative feedback or distracting irrelevant dialogues from other agents. However, if their reasoning and refinement capabilities are weak, they may get stuck in execution loops without making progress toward their goals.
Multi-agent architectures are particularly suited for tasks that can benefit from feedback from multiple agent roles, such as document generation. In such architectures, one agent can provide explicit feedback on a document written by another agent. Additionally, multi-agent systems also demonstrate their utility when tasks need to handle multiple different tasks or workflows simultaneously. Research has also shown that in the absence of examples, multi-agent modes can perform better than single-agent modes. Due to the complexity of multi-agent systems, they often require effective dialogue management and clear leadership to guide them.
Despite the differences in performance capabilities between single-agent and multi-agent modes, research indicates that if the prompts provided to agents are already sufficiently excellent, discussions among multi-agents may not further enhance their reasoning capabilities. This means that when deciding whether to adopt a single-agent or multi-agent architecture, consideration should be given to their specific use cases and contextual needs rather than solely based on reasoning capabilities.
Agents and Asynchronous Task Execution
Agents play a crucial role in handling asynchronous task execution. While a single agent is capable of initiating multiple asynchronous tasks simultaneously, its working mode does not inherently support responsibility allocation across different execution threads. This indicates that while tasks are processed concurrently, they do not equate to genuine parallel processing managed by independent decision-making entities. Therefore, a single agent must proceed sequentially when executing tasks, completing a batch of asynchronous operations before moving on to evaluations and the next steps. In contrast, multi-agent architectures allow each agent to operate independently, enabling more flexible and dynamic responsibility allocation. This architecture not only allows tasks under different domains or objectives to be conducted simultaneously but also enables each agent to independently advance its tasks without being constrained by the status of other agents’ tasks, reflecting a more flexible and efficient task management strategy.
Feedback and Human Supervision
Feedback and human supervision are crucial for agent systems. When solving complex problems, we rarely propose correct and robust solutions on the first attempt. Typically, a potential solution is first proposed, then critiqued and improved, or others are consulted for different perspectives. For agents, this iterative feedback and improvement concept is equally important, assisting them in solving complex problems. This is because language models often rush to provide answers early in their responses, which may lead them to gradually deviate from the target state, creating what is known as the “snowball effect.” By implementing feedback mechanisms, agents are more likely to correct their direction and reach their goals.
Moreover, incorporating human supervision can improve immediate results, making agents’ responses more aligned with human expectations and reducing the likelihood of agents adopting inefficient or incorrect methods to solve problems. To date, incorporating human validation and feedback into agent architectures has proven to produce more reliable and trustworthy results. However, language models have also shown a tendency to cater to user positions, even if it means sacrificing fairness or balance. Notably, the AgentVerse paper describes how agents can easily be influenced by feedback from other agents, even when that feedback is unreasonable. This may lead multi-agent architectures to formulate erroneous plans that deviate from their goals. While strong prompts can help mitigate this issue, it is important to recognize the risks involved when implementing user or agent feedback systems during the development of agent applications.
Group Dialogue and Information Sharing
In multi-agent architectures, challenges exist regarding information sharing and group dialogue among agents. Multi-agent modes tend to engage in non-task-related communication, such as greetings, while single-agent modes tend to focus more on the task at hand due to the absence of a team requiring dynamic management. In multi-agent systems, this excess dialogue may weaken agents’ abilities to reason effectively and execute tools correctly, ultimately distracting agents and reducing team efficiency. Particularly in horizontal architectures, agents often share a group chat, being able to see every message from every other agent in the dialogue. By employing message subscription or filtering mechanisms, agents can ensure that they only receive information relevant to their tasks, thereby enhancing the performance of multi-agent systems.
In vertical architectures, tasks are typically explicitly divided according to agents’ skills, which helps reduce distractions within the team. However, challenges arise when the leading agent fails to send key information to supporting agents and is unaware that other agents have not received necessary information. Such oversights may lead to confusion within the team or distorted outcomes. One way to resolve this issue is to explicitly include information about access rights in the system prompts, enabling agents to interact appropriately in context.
Role Definition and Dynamic Teams
Role definition is crucial for both single-agent and multi-agent architectures. In single-agent architectures, clear role definitions ensure that agents focus on designated tasks, execute tools correctly, and reduce hallucinations regarding other capabilities. In multi-agent architectures, role definitions also ensure that each agent understands its responsibilities within the team and does not undertake tasks beyond its described capabilities and scope. Moreover, establishing a clear team leader can simplify task allocation, thereby enhancing the overall performance of multi-agent teams. Defining clear system prompts for each agent can reduce ineffective communication and prevent agents from engaging in irrelevant discussions.
The concept of dynamic teams, which involves introducing or removing agents from the system as needed, has also proven effective. This ensures that all agents involved in planning or executing tasks are suitable for the current work’s needs, thereby improving the relevance of task execution and team efficiency.
Conclusion
Whether in single-agent or multi-agent systems, they excel at handling complex tasks requiring reasoning and tool calling. Single agents perform best when there is a clear role definition, tool support, the ability to receive human feedback, and a gradual approach toward goals. In building collaborative agent teams to jointly accomplish complex objectives, the presence of one or more of the following features among agents in the team is beneficial: clear leadership, well-defined planning phases that can continuously optimize plans based on new information, effective information filtering to optimize communication, and the ability to adjust team members based on task requirements to ensure they possess relevant skills. If agent architectures can incorporate one of these strategies, their performance is likely to surpass that of single-agent systems or multi-agent systems lacking these strategies.
Limitations and Future Research Directions for AI Agents
Despite the significant enhancement of language model capabilities by AI agent architectures in many aspects, challenges remain in evaluation, overall reliability, and addressing issues arising from the underlying language models.
Challenges in Agent Evaluation
While large language models can typically be evaluated for their understanding and reasoning capabilities through a standardized set of tests, there is no unified standard for evaluating agents. Many research teams design unique evaluation criteria for the agent systems they develop, making comparisons between different agent systems difficult. Additionally, some newly established evaluation standards for agents contain handcrafted complex test sets, which require manual scoring. While this provides an opportunity for in-depth evaluation of agent capabilities, they often lack the robustness of large-scale datasets and may introduce evaluation biases since the scorers may also be the developers of the agent systems. Furthermore, the ability of agents to generate consistent answers in continuous iterations may also be affected by changes in the model, environment, or problem state, with this instability being particularly pronounced in small and complex evaluation sets.
The Impact of Data Contamination and Static Benchmarks
Some researchers use standard large language model benchmarks to evaluate the performance of agent systems. However, recent studies have revealed potential data contamination issues within these models’ training data. When benchmark test questions are slightly modified, the model’s performance can drop sharply, confirming the existence of data contamination. This finding raises questions about the high scores achieved by language models and their driven agent systems in benchmark tests, as these scores may not accurately reflect the models’ actual capabilities.
At the same time, as LLM technology progresses, existing datasets often fail to keep pace with the increasingly sophisticated capabilities of models, as these benchmark tests typically maintain fixed complexity levels. To address this challenge, researchers have begun developing dynamic benchmarks that can withstand simple memorization behaviors. Additionally, they are exploring the creation of completely synthetic benchmark tests based on users’ specific environments or use cases to evaluate agent performance more accurately. While these methods may help reduce data contamination, decreasing human involvement may increase uncertainty in evaluation results regarding accuracy and problem-solving capabilities.
Benchmark Scope and Transferability
Many language model benchmarks are designed to solve problems in a single iteration without tool calling, such as MMLU or GSM8K. While these are important for measuring the capabilities of base language models, they are not good standards for assessing agent capabilities, as they do not consider the ability of agent systems to reason over multiple steps or access external information. StrategyQA improves upon this by evaluating models’ reasoning abilities over multiple steps, but the answers are limited to yes/no responses. As the industry continues to shift toward agent-centric use cases, additional measures will be needed to better evaluate agents’ performance and generalization capabilities in tasks involving tools beyond their training data.
Some specific agent benchmarks, such as AgentBench, evaluate language model-based agents’ performance in various environments, such as web browsing, command line interfaces, and video games. This better indicates the generalization capabilities of agents in achieving given tasks through reasoning, planning, and tool calling. Benchmarks like AgentBench and SmartPlay introduce objective evaluation metrics aimed at assessing success rates, output similarity to human responses, and overall efficiency. While these objective metrics are essential for understanding the overall reliability and accuracy of implementations, it is also important to consider more nuanced or subjective performance metrics. Metrics such as tool usage efficiency, reliability, and planning robustness are almost as important as success rates, but are more challenging to measure. Many of these metrics require human expert evaluation, which may be costly and time-consuming compared to LLM-based assessments.
Bias and Fairness in Agent Systems
Language models often exhibit biases in evaluation processes and societal fairness. Moreover, agent systems have been pointed out to lack robustness, potentially displaying inappropriate behaviors and generating more subtle content than traditional language models, posing significant security challenges. Some studies have observed that even when guided to debate from a specific political perspective, language model-driven agents tend to follow inherent biases within the models, which may lead to errors in the reasoning process.
As the tasks undertaken by agents become more complex and their involvement in decision-making processes increases, researchers need to delve deeper into studying and addressing bias issues within these systems. This undoubtedly poses a significant challenge for researchers, as creating scalable and innovative evaluation benchmarks typically requires a certain degree of involvement from language models. However, to genuinely assess the biases of language model-based agents, the benchmarks employed must include human evaluations to ensure accuracy and fairness in assessments.
Conclusion
AI Agents can effectively enhance the reasoning, planning, and tool-calling capabilities of LLM language models. Whether in single-agent or multi-agent architectures, they exhibit the problem-solving capabilities required to tackle complex issues. The most suitable agent architecture depends on the specific application scenario. However, regardless of the architecture, high-performing agent systems typically adopt at least one of the following strategies: clear system prompts, explicit leadership and task allocation, specialized processes from reasoning and planning to execution and evaluation, flexible team structures, feedback mechanisms from humans or agents, and intelligent message filtering capabilities. The application of these techniques allows agents to perform better across various benchmark tests.
Despite the tremendous potential exhibited by AI-driven agents, they still face challenges and areas for improvement. To achieve more reliable agents, we must address issues such as comprehensive benchmark testing, real-world applicability, and the reduction of harmful biases within language models in the near future. Researchers aim to provide a comprehensive perspective on the evolution from static language models to more dynamic and autonomous agents by reviewing this evolution, offering valuable insights for individuals or organizations currently using existing agent architectures or developing customized agent architectures.
Final Thoughts
This article primarily serves as a translation, summary, and study of the paper “The Landscape of Emerging AI Agent Architectures for Reasoning, Planning, and Tool Calling: A Survey” and related literature, intended for learning and exchange purposes only. The original English paper can be viewed by clicking on the Read Original
button in the lower left corner.
References:
-
Masterman, Tula et al. The Landscape of Emerging AI Agent Architectures for Reasoning, Planning, and Tool Calling: A Survey. 2024. arXiv: 2404.11584 [cs.AI]. URL: https://arxiv.org/abs/2404.11584 -
Xudong Guo et al. Embodied LLM Agents Learn to Cooperate in Organized Teams. 2024. arXiv: 2403.12482 [cs.AI]. -
Weize Chen et al. AgentVerse: Facilitating Multi-Agent Collaboration and Exploring Emergent Behaviors. arXiv:2308.10848 [cs]. Oct. 2023. URL: http://arxiv.org/abs/2308.10848.
Recommended Selections
-
Three Methods to Completely Freely Use GPT-4, All Organized for You! -
The Domestic Light of the AI Field, ChatGPT’s Free Alternative: Kimi Chat! -
Kimi Chat, More Than Just Chatting! In-depth Analysis of 5 Major Use Cases! -
I Used AI Tools to Make an Animated Short Film in 5 Minutes! This AI is Now Free! -
As Everyone on the Internet Goes Crazy Over OpenAI’s Sora, What Can Ordinary People Prepare? — What You Need to Know About Sora! -
Which Is Stronger, Wenxin Yiyan 4.0 VS ChatGPT 4.0?! Is the Monthly 60 Yuan Wenxin Yiyan 4.0 Worth It? -
Which Is Better, ChatGPT or Wenxin Yiyan? One Question Tells You the Answer! -
ByteDance Launched “Button”, the Domestic Version of Coze, But I Don’t Recommend You Use It! -
Freely Using GPT-4, Dalle3, and GPT-4V – Initial Experience of Coze Developed by ByteDance! Includes Tutorials and Prompt Keywords! -
In 2024, Are You Still Using Baidu Translate? A Step-by-Step Guide to Using AI Translation! One-Click Translation of Webpages and PDF Files!
If you’ve read this far, please give a thumbs up to encourage me; a little thumbs up can lead to an annual salary of a million! 😊👍👍👍. Follow me, and you won’t get lost on the road to AI; original technical articles will be pushed at the first time 🤖.