Recent major reports on trends for 2024 have all mentioned AI Agents.
Accenture’s “Technology Outlook 2024” report indicates that 96% of executives believe that the application of AI Agent ecosystems will bring significant opportunities to their organizations in the next three years.
The report suggests that as artificial intelligence evolves into agents, automation systems will be able to make autonomous decisions and take actions. Agents will not only provide suggestions to humans but also act on their behalf. AI will continue to generate text, images, and insights, while AI Agents will decide how to handle this information independently.
As agents upgrade to become colleagues of humans, it will be necessary for humans and agents to reconstruct the future of technology and talent together.
According to IDC’s “Top Ten Trends in AIGC Applications” report, all companies regard AI Agents as a definitive direction for AIGC development, with 50% of companies already piloting AI Agents in some work, and another 34% devising application plans for AI Agents.
This report also makes two predictions about the development trends of AI Agents:
-
AI Agents make “human-machine collaboration” the new normal, ushering individuals and enterprises into the era of AI assistants. AI Agents can help future enterprises build a new normal of intelligent operations centered around “human-machine collaboration.”
-
AI Agents will transform the organizational form of future productivity, countering organizational entropy increase. In the future, work tasks will become increasingly atomized and fragmented with the support of AIGC, complex processes will be infinitely disassembled, and then flexibly rearranged and combined, with AI continuously mining the efficiency and potential of each link. From the supply side, the efficient collaboration model of “human + AI digital employees” will provide an ideal solution for large enterprises to counter organizational entropy increase.
The report believes that general artificial intelligence is approaching, large models are transitioning to multimodal, and AI agents are expected to become the next-generation platform; edge large models will accelerate deployment and may become a new entry point for future interactions. AI is making significant strides in fields such as mathematical reasoning, new drug development, material discovery, and protein synthesis, with “AI scientists” expected to emerge rapidly.
These three reports predict the future development trends of AI Agents while also mentioning a series of concepts such as multimodal large models, digital employees, and embodied intelligence.
The new concept of digital employees provided by IDC has a very strong connection with AI Agents. Additionally, the report’s mention of “one person plus enough AI tools can become a specialized company” points to the currently hot topic of super individuals.
In fact, AI Agents are not only related to two concepts but have also spawned more research directions. So, what is the connection between digital employees and super individuals with AI Agents? What is the relationship between embodied intelligence and AI Agents? What are the research directions of AI Agents?
Research Direction 1: AI Agents Based on Large Language Models
Large Language Models (LLM) are deep learning models built using artificial neural networks trained on massive amounts of text data. They can not only generate natural language text but also understand text meanings deeply and handle various natural language tasks such as text summarization, Q&A, translation, etc.
In 2023, large language models and their applications in the field of artificial intelligence have become a global research hotspot, particularly notable for their growth in scale, with parameter counts jumping from a few billion to now trillions.
The increase in parameters allows the models to capture the subtleties of human language more precisely and understand the complexities of human language more deeply.
Over the past year, large language models have shown significant improvements in absorbing new knowledge, decomposing complex tasks, and aligning text and images. As the technology matures, it will continue to expand its application range, providing more intelligent and personalized services to improve people’s lives and ways of production.
The wave of large language models has accelerated the rapid development of research related to AI Agents, which is currently the main exploration route to AGI.
The vast training datasets of large models contain a wealth of human behavioral data, laying a solid foundation for simulating human-like interactions; on the other hand, as model sizes continue to grow, large models have exhibited various capabilities similar to human thinking, such as contextual learning, reasoning, and chains of thought.
LLMs provide a new foundation for AI Agents, focusing on automation and humanization as two major directions. The vast training datasets of large language models contain a wealth of human behavioral data, laying a solid foundation for simulating human-like interactions; on the other hand, as model sizes continue to grow, large models have exhibited various capabilities similar to human thinking, such as contextual learning, reasoning, and chains of thought.
Using large models as the core brain of AI Agents allows for the realization of previously difficult tasks such as breaking down complex problems into manageable sub-tasks and human-like natural language interactions. However, since large models still face many issues such as hallucinations and context capacity limitations, constructing intelligent agents with autonomous decision-making and execution capabilities by leveraging one or more agents has become the current main research direction toward AGI.
Before the arrival of the AGI era, the limits of AI Agents’ capabilities will primarily be influenced by their brain, which is the LLM, indicating that LLM will determine the popularity and application of Agents in the future.
Therefore, AI Agents based on LLM will be a long-term research direction for people.
Research Direction 2: Construction, Application, and Evaluation of AI Agents
This is the main direction of AI Agent research.
Building AI Agents requires a deep understanding of their core technologies, including LLM, memory, planning skills, and tool usage capabilities. The application fields of AI Agents are very broad, including games, personal assistants, emotional companionship, etc.
Evaluating the performance of AI Agents is an important part of research, considering how to assess their general language understanding and reasoning abilities under zero-shot conditions.
The construction, application, and evaluation of AI Agents are all important parts of artificial intelligence research.
Construction of AI Agents
The construction of AI Agents mainly includes four parts: large models, planning, memory, and tool usage.
Large Models:Large models (e.g., GPT-4, Wenxin Yiyan, Tongyi Qianwen, etc.) serve as the “brain” of AI Agents, providing reasoning and planning capabilities.
Planning:Agents can decompose large tasks into smaller, manageable sub-goals, thereby better handling complex tasks.
Memory:AI Agents have the ability to retain and recall information over long periods, usually achieved by utilizing external vector storage and rapid retrieval.
Tool Usage:Agents learn to call external APIs to obtain additional information missing from the model weights, including current information, code execution capabilities, access to proprietary information sources, etc.
These four modules are closely related to the improvement of AI Agent capabilities, and many organizations will invest significant and ongoing research efforts to enhance the application and popularization speed of AI Agent capabilities.
Application of AI Agents
AI Agents have applications in multiple fields, including but not limited to education, gaming, online shopping, and web browsing. For example, in the education sector, AI Agents provide personalized, intelligent, and efficient services to optimize the learning experience.
This book will explore the applications of AI Agents in various fields in the second part.
Evaluation of AI Agents
Evaluating AI Agents is a significant challenge that requires quantifying and objectively measuring their intelligence levels. The Turing Test is a common evaluation method used to assess whether an artificial intelligence system exhibits human-like intelligence.
Additionally, there are specialized benchmark tests, such as AgentBench, used to evaluate LLMs as agents in various real-world challenges and different environments. More benchmark tests will be developed for various aspects of agents to promote the healthy development and ecological improvement of the agent ecosystem.
Research Direction 3: Multi-Agent Systems
Multi-Agent Systems (MAS) consist of multiple autonomous agents that cooperate or compete with each other to solve complex problems through collective behavior. In MAS, each agent has a certain degree of autonomy, can perceive the environment, make decisions, and interact with other agents.
Agents can perform various tasks, with their specific nature depending on the system’s goals and application fields. The main tasks of agents typically include perceiving the environment, processing information, making decisions, and interacting with other agents to achieve common goals.
Multi-Agent Systems are an important branch of artificial intelligence that studies how to design and implement mechanisms and methods for cooperation and competition among multiple agents. They have the following characteristics:
1. Composed of multiple autonomous, interactive, heterogeneous agents, each with its own goals, behaviors, beliefs, and preferences, while also being influenced and constrained by the environment.
2. The goal is to achieve a balance of collaboration and competition among agents, allowing each agent to reach its own goals while also promoting the performance and efficiency of the entire system.
3. The challenge lies in how to handle complex interactions and coordination among agents, resolve conflicts and contradictions between agents, assess agent performance and progress, accept human feedback and guidance, and comply with human ethics and laws.
Multi-Agent Collaboration Systems (MACS) are a special type of multi-agent system aimed at enabling multiple agents to effectively collaborate to achieve tasks beyond the capability of a single agent.
Agents can interact with each other in a cooperative or competitive manner. This allows them to make progress through teamwork or adversarial interactions. In these systems, agents can collaboratively complete complex tasks or compete with each other to improve their performance.
For example, they can be used to simulate and optimize complex systems in traffic, energy, logistics, etc., or to design and implement applications such as smart homes, smart cities, and smart factories.
The core challenge of multi-agent collaboration systems is how to achieve a balance of collaboration and competition among agents, as well as how to enable agents to adapt and learn based on different tasks and roles.
In recent years, with the development of deep learning, reinforcement learning, natural language processing, and other technologies, significant progress and breakthroughs have been made in the research of multi-agent collaboration systems.
For example, CAMEL is the first large model multi-agent framework that allows multiple agents to learn collaboratively and competitively in a shared environment, while also enabling natural language communication and negotiation between agents. CAMEL has gained 3.6k stars on NeurIPS 2023, showcasing the tremendous potential and prospects of multi-agent collaboration systems.
Additionally, there are several representative multi-agent collaboration systems, such as OpenAI Five, AlphaStar, and DeepMind Quake III Arena Capture the Flag, which have demonstrated superhuman levels of collaboration and competition in games like DOTA 2, StarCraft II, and Quake III.
Multi-Agent Systems are a cutting-edge and hot research field in artificial intelligence, involving multiple disciplines and fields such as computer science, mathematics, economics, psychology, sociology, and biology.
Their research and application are of great significance and value for understanding the essence and mechanisms of human intelligence, improving the level and capabilities of artificial intelligence, and solving various problems in human society.
Research Direction 4: Autonomous Agents
In the field of artificial intelligence, autonomous agents refer to intelligent entities that can perceive, learn, and execute actions in an environment. These entities possess autonomy, meaning they can make decisions and act independently without human intervention.
Autonomous agents have the ability to make decisions and act independently, allowing them to perceive, learn, and make decisions autonomously in a given environment to achieve specific goals. They can continuously adapt and improve their behavior based on changes in the environment and feedback information, achieving better performance and results.
They are typically designed to have the ability to perceive the environment, make rational decisions based on perceived information, and execute corresponding actions to achieve specific goals. In achieving autonomy, techniques such as machine learning and deep learning play key roles.
The design and implementation of autonomous agents involve multiple aspects, including but not limited to the comprehensive application of AI technologies such as machine learning, natural language processing, and computer vision.
They are designed to perform various tasks, such as managing social media accounts, investing in markets, creating children’s books, and in some cases, they can help people free up time for more creative endeavors.
Their research value is primarily reflected in reinforcement learning and robotics, such as DeepMind’s Alphago and OpenAI’s OpenAI Five (an AI for team battles in Dota 2), which are typical examples of reinforcement learning-based agents.
With the explosion of LLMs, research and topics related to agents have surged in the past year, with projects like AutoGPT, BabyAGI, Generative Agents, and MetaGPT amassing tens of thousands of stars on GitHub, becoming hot star projects.
Using autonomous agents generally involves the following steps:
Defining the Problem and Goals:First, clarify the problem and goals, defining the tasks the agent needs to solve and the expected outcomes.
Building the Environment Model:Understand and model the interaction between the agent and the environment, including state space, action space, reward functions, etc.
Selecting the Appropriate Algorithm:Choose suitable reinforcement learning algorithms or other related algorithms to train the autonomous agent based on the nature and characteristics of the problem.
Training and Optimization:Using the selected algorithm and environment model, train the autonomous agent through interactions and feedback with the environment to learn appropriate decision-making strategies that maximize cumulative rewards or achieve specific goals.
Tuning and Evaluation:Based on the performance and outcomes during the training process, tune and evaluate the autonomous agent to enhance its decision-making capabilities and effectiveness.
Compared to previous reinforcement learning-based agent research, current agents primarily refer to intelligent entities that use large model technology (LLM) as the core or brain, capable of automated planning and possessing autonomous decision-making abilities to solve complex problems.
In recent years, there have been many breakthrough advancements in the research of autonomous agents, with previous challenges concerning social interactivity and intelligence being addressed with the development of large language models (LLMs).
For example, some research is exploring how to guide large models in task decomposition through large model prompting methods like Chain-of-Thought, as well as how to enhance precise memory and complex reasoning abilities through memory modules. Overall, the research on autonomous agents is progressing rapidly, showing tremendous potential and prospects.
Research Direction 5: Generative Agents
Generative Agents can be defined as computational software agents capable of simulating credible human behavior. They can store a complete record of the agent’s experiences, integrating these memories over time into higher-level reflections and dynamically retrieving these memories to plan behaviors.
Generative Agents can reason extensively about themselves, other agents, and the environment. When faced with new tasks, they can quickly adjust their learning methods using acquired general knowledge and strategies, reducing reliance on large samples. This technology can be widely applied in interactive applications such as immersive environments, rehearsal spaces for interpersonal communication, and prototype design tools.
The concept of generative agents was first proposed by researchers from Stanford University and Google in the 2023 paper “Generative Agents: Interactive Simulacra of Human Behavior.”
Paper link: https://arxiv.org/abs/2304.03442
To create generative agents, researchers constructed a system architecture that extends the capabilities of large language models, enabling them to store experience records of the agent’s use of natural language. Over time, these memories are integrated into higher-level thoughts and dynamically retrieved to plan the agent’s behavior.
Researchers applied generative agents in an interactive sandbox environment inspired by “The Sims.” In this environment, end-users can interact using natural language with a small town composed of 25 agents.
The behaviors of these agents resemble those of humans: they wake up in the morning, make breakfast for themselves, and then go to work; artist agents create paintings while writer agents write articles; they can form their own opinions, pay attention to other agents, and engage in conversations; when planning their work for the next day, they recall and reflect on past days.
Additionally, these agents can use natural language to store complete records related to the agents, integrating these memories over time into higher-level thoughts and dynamically retrieving these memories to guide their behavior.
Evaluation results show that these generative agents exhibit credible individual and social behaviors. For example, starting from a user-specified concept, such as an agent wanting to host a Valentine’s Day party, these agents autonomously spread invitations over the next two days, meet new friends, agree to attend the party, and coordinate to appear at the party at the right time.
Research findings indicate that the components of the agent architecture, such as observation, planning, and reflection capabilities, play a crucial role in the credibility of agent behaviors.
This research combines large language models with computational, interactive agents, laying the foundation for credible simulations of human behavior.
Furthermore, this research demonstrates that credible human behavior agents can enhance the functionality of interactive applications, from immersive environments to rehearsal spaces for interpersonal communication and prototype design tools.
Research Direction 6: Human-Agent Collaboration
The future of human-agent collaboration brought about by generative AI will present three modes: embedding mode, copilot mode, and agent mode.
Embedding Mode:Users set goals through language interactions with AI using prompts, and AI assists users in achieving these goals.
Copilot Mode:In this mode, humans and AI play respective roles. AI intervenes in the workflow, from providing suggestions to assisting in various stages of the process.
Agent Mode:In this mode, humans set goals and provide resources, usually computational power, while supervising the results. In this case, the agent takes on most of the work.
Agent mode will become the primary mode of future human-machine interaction.
Human-Agent Collaboration (HAC) refers to the collaboration and cooperation between humans and agents (such as robots, virtual assistants, etc.) to accomplish specific tasks or solve problems.
Agents can interact with humans, provide assistance, and execute tasks more efficiently and safely. They can understand human intentions and adjust their behaviors to provide better services. Human feedback can also help agents improve performance.
In agent mode, humans set goals and provide necessary resources (e.g., computational power), while AI independently takes on most of the work, and finally, humans supervise the process and evaluate the final results. This collaborative mode combines human creativity and judgment with the data processing and real-time response capabilities of intelligent agents, aiming to achieve a more efficient and intelligent working method.
In this mode, AI fully reflects the interactive, autonomous, and adaptive characteristics of agents, approaching independent actors, while humans play more of a supervisory and evaluative role. The agent mode is undoubtedly more efficient than embedding and copilot modes, and it may become the primary mode of future human-agent collaboration.
The emergence of AI Agents has evolved large models from “super brains” to “all-purpose assistants” for humans. AI Agents not only need to possess the intelligence to handle tasks and problems but also need to have social intelligence for natural interaction with humans.
This social intelligence includes the ability to understand and generate natural language, recognize emotions, and more. The development of social intelligence will enable AI Agents to better collaborate and communicate with humans, expanding their application scenarios.
Agents based on large models can allow everyone to have a personalized intelligent assistant with enhanced capabilities, and will change the mode of human-agent collaboration, inevitably leading to broader human-agent integration.
Research Direction 7: Super Individuals
Based on the human-agent collaboration model, every ordinary individual has the potential to become a super individual.
A super individual is an organic system composed of many organisms, typically a social unit of a truly social animal, where the social division of labor is highly specialized, and individuals cannot survive alone for long.
In modern society, super individuals can also refer to talents proficient in one or more professional skills, achieving commercial monetization, ultimately breaking away from traditional employment relationships.
AI Agents can empower super individuals with more opportunities, allowing individuals to showcase their talents in broader fields and engage in creative work through AI empowerment, sufficient to create a team and company of one.
Super individuals possess their own AI teams and automated task workflows, establishing more intelligent and automated collaborative relationships with agents and other super individuals. There is no shortage of positive explorations of one-person companies and super individuals within the industry.
On the GitHub platform, several automation team projects based on agents have emerged.
GPTeam utilizes large models to create multiple agents endowed with roles and functions, collaborating to achieve predetermined goals.
Dev-GPT is a multi-agent collaboration team for automated development and operations, including roles such as product manager agent, developer agent, and operations agent. This multi-agent team can meet and support the normal operation of a startup marketing company, which is a one-person company.
There is also NexusGPT, claimed to be the world’s first AI freelancer platform, which integrates various AI-native data from open-source databases and has over 800 AI agents with specific skills.
On this platform, you can find experts in various fields, such as designers, consulting advisors, sales representatives, etc. Employers can choose an AI agent to help them complete various tasks at any time.
Many people are now using AI tools to enhance labor or production skills, automating personal production processes, allowing one person to replace the work of an entire company, which can be seen as a preliminary form of super individuals.
In the future, everyone can choose diverse collaboration methods, becoming super individuals through collaboration with different personal assistants or intelligent agents.
The core operations of future companies will be automated, with tasks decomposed into modular processes for automated execution. This means that one person can manage multiple different companies, as long as the business systems are set up.
As a result, the operations of companies will increasingly rely on super individuals, specialized models, and the construction of AI teams.
Research Direction 8: Digital Employees
Digital employees typically refer to automation tools and applications that combine artificial intelligence and robotic process automation (RPA) technology, representing a new type of digital worker that is highly anthropomorphized from a human resources perspective.
They can automatically execute a large number of repetitive and rule-based tasks without the need for direct human involvement, thereby improving work efficiency and quality.
Digital employees leverage modern technology and data analysis capabilities, integrating AI, RPA, big data analytics, digital humans, and robots through automation and intelligence, providing enterprises with a new tool for enhancing workforce and work efficiency.
Digital employees can replace us in executing many repetitive tasks; however, they do not “replace” humans but help us complete work more efficiently.
The concept of digital employees includes the following characteristics:
Software rather than physical robots:Digital employees are implemented through software, rather than being physically existing robots.
Applicable to specific scenarios:Digital employees are particularly suitable for environments with clear rules and strong repetition.
Wide application:Digital employees have been widely applied in various industries and fields, including finance, manufacturing, and retail.
Digital employees are viewed as an innovative form of labor, capable of helping enterprises reduce costs and improve efficiency while decreasing reliance on human labor. Many industries are gradually introducing this technology, with high application penetration rates already achieved in finance, government, telecommunications, energy, and other fields.
Digital employees rely on AI technology, for instance, their foundational technology RPA is built on AI products, while other applications such as chatbots and digital humans are also applications of AI.
The recent explosion and application of large language models have brought significant technological changes to digital employees, especially their integration with AI Agents is giving rise to a new form of digital employee called RPA Agents.
RPA Agents are generally AI Agents built based on RPA launched by RPA/super-automation vendors, or AI Agents that use RPA as a UI automation tool in agent construction. They combine API and user interface (UI) automation, greatly enhancing the execution capabilities of AI Agents.
Upgrading the RPA technology in digital employees to RPA Agents, or constructing digital employees based on AI Agents using RPA as a tool, will significantly leapfrog the capabilities of digital employees.
Agent digital employees powered by large language models and AI Agents possess higher intelligence and autonomy, can plan tasks and invoke various tools to complete a large amount of work in a unit of time, and can communicate and coordinate with humans using natural language.
Currently, in addition to RPA/super-automation vendors conducting research and launching related products, large model vendors and some research institutions are also exploring this area.
For instance, Tsinghua University’s natural language processing laboratory and other institutions have jointly released a new generation of process automation paradigm called Agentic Process Automation (APA, with the related project ProAgent), which achieves automation of workflow construction and dynamic decision-making during workflow execution, revealing the feasibility and potential of large model agents in automation through experiments.
Paper link: https://github.com/OpenBMB/ProAgent/blob/main/paper/paper.pdf
Research Direction 9: Embodied Intelligence
Embodied intelligence refers to the ability of robots or agents to adapt to environments and execute tasks through perception, understanding, and interaction. Unlike traditional rule-based or symbolic artificial intelligence, embodied intelligence emphasizes the combination of perception and action, enabling agents to better understand their surrounding environments and interact with them.
AI systems equipped with perception and action capabilities can acquire knowledge and experience through interactions with the environment. AI Agents are a special form of embodied intelligence systems that can understand and respond to user needs, providing personalized services and suggestions.
The combination of AI Agents and embodied intelligence can effectively promote the application of AI large models, mainly including the following points:
1. Enhancing Comprehensive Abilities.AI large models themselves do not possess the ability to perceive the environment and execute actions, while embodied intelligence can provide these capabilities to AI large models, allowing them to better understand the environment, make decisions, and execute actions.
2. Achieving Real-Time Decision-Making and Execution.The training and inference of AI large models usually require a long time and significant computational resources. By assigning computational tasks to cloud-based AI Agents and perception and execution tasks to embodied intelligence, real-time decision-making and execution can be achieved.
3. Providing Personalized and Adaptive Services.AI large models can provide personalized services by learning a large amount of user data and behavior patterns. Combined with embodied intelligence, AI Agents can extend personalized services into the physical world based on user needs.
4. Protecting User Security and Privacy.AI large models typically require large amounts of training data, which may involve user privacy. By combining with embodied intelligence, sensitive user data can be retained locally, transmitting only necessary information to cloud-based AI Agents for processing, thus providing higher security and privacy protection.
The role of embodied intelligence is not limited to robotics; it also involves other fields.
In robotics, embodied intelligence enables robots to better perceive their surrounding environments, make intelligent decisions, and execute corresponding actions to achieve various tasks and goals.
In the field of autonomous vehicles, embodied intelligence allows cars to better perceive the road, assess traffic conditions, and make safe driving decisions.
In the field of drones, embodied intelligence allows drones to better perceive the aerial environment, avoid obstacles, and execute precise flight tasks.
Many companies are exploring embodied intelligence; for instance, OpenAI not only emphasized the importance of AI Agents at its first developer conference but also invested in the Norwegian humanoid robot company 1X Technologies to promote the integration of large models and embodied intelligence.
Embodied intelligence is considered an important pathway to general artificial intelligence, and there have been many breakthrough advancements in research related to it, such as the VoxPoser system developed by AI scientist Li Feifei’s team.
Beijing University of Aeronautics and Astronautics’ intelligent drone team has also proposed a multimodal large model-based embodied intelligent agent architecture called “Agent as Cerebrum, Controller as Cerebellum.”
Paper link: https://arxiv.org/abs/2311.15033
This architecture treats the agent as the brain, focusing on generating high-level behaviors, while the controller acts as the cerebellum, focusing on converting high-level behaviors (such as desired target points) into low-level system commands (such as rotor speeds).
In the future, the combination of AI Agents and embodied intelligence will integrate the powerful capabilities of AI large models with the perception and execution capabilities in specific scenarios, promoting the application of AI large models in practical applications and enriching application scenarios.
Research Direction 10: Agent Society
Agent Society is a term in computer science technology announced in 2018, referring to a multi-agent system defined by roles and role relationships, as well as concepts such as obligations, commitments, and ethics.
Based on the application of large language models, this concept refers to artificial intelligence agents created using LLMs that interact with each other in simulated environments, allowing these agents to act, make decisions, and engage in social activities like humans.
Agent Society represents the highest form and goal of artificial intelligence agents, consisting of a complex, dynamic, self-organizing, adaptive, cooperative, competitive, and evolving system made up of multiple artificial intelligence agents. It can perform complex and flexible actions and tasks based on its own goals and environmental changes while engaging in high-level and multidimensional interactions and collaborations with humans and other agents.
It can help us understand how artificial intelligence agents work and behave in social-like environments. This simulation can provide insights into collaboration, policy-making, and ethical considerations. Overall, Agent Society helps us explore the social aspects of artificial intelligence agents and their interactions in real and controlled environments.
In this social system, agents can execute complex and flexible tasks based on goals and environmental changes, while engaging in high-level, multidimensional interactions and collaborations with humans and other agents. Agent Society not only helps humans explore and expand the physical and virtual worlds but also enhances and extends human capabilities and experiences.
Agent Society is an important way for humans to explore and expand the physical and virtual worlds, enhance and extend human capabilities and experiences, and create and enjoy novelty and interesting things. It can help humans achieve their own or others’ values and happiness.
Typical applications of Agent Society include artificial intelligence entities, virtual communities, and distributed systems, which can execute complex and flexible actions and tasks based on their own goals and environmental changes while engaging in high-level and multidimensional interactions and collaborations with humans and other agents.
For a long time, sociologists have conducted social experiments to observe specific social phenomena in controlled environments. Notable examples include the Hawthorne experiment and the Stanford prison experiment.
Subsequently, researchers began using animals in social simulations, with the mouse utopia experiment being an example. These experiments invariably used living beings as participants, making it difficult to conduct various interventions, lacking flexibility and time efficiency.
Researchers and practitioners have been envisioning an interactive artificial society where human behaviors can be realized through credible agents.
From sandbox games like “The Sims” to the Metaverse concept, we can see the definition of “simulated society” in people’s minds: an environment and individuals interacting within it. Behind each individual can be a program, a real human, or an agent based on LLM.
The interactions between individuals are also one of the reasons for the emergence of sociality. Multi-agent collaboration can form the highest form of the technical social system, the Agent Society, which has complex, dynamic, self-organizing, and self-adaptive characteristics, enabling cooperation, competition, and continuous evolution.
Breaking through the development difficulties of multi-agents is an important prerequisite for establishing the future Agent Society.
References:
1. “A Survey on Large Language Model-based Autonomous Agents”
2. “The Rise and Potential of Large Language Model-Based Agents: A Survey”
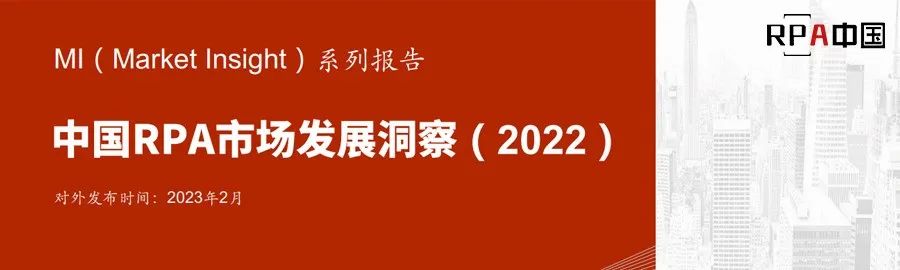
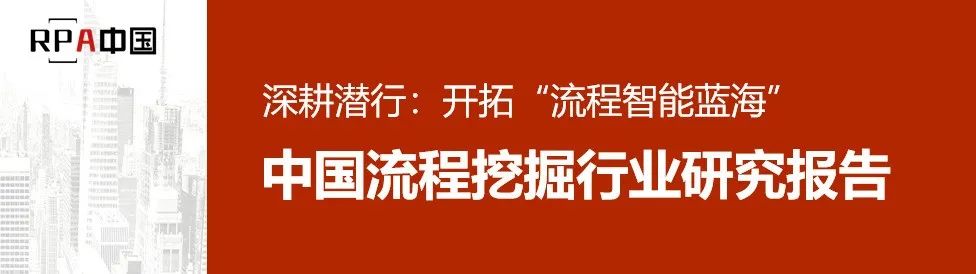