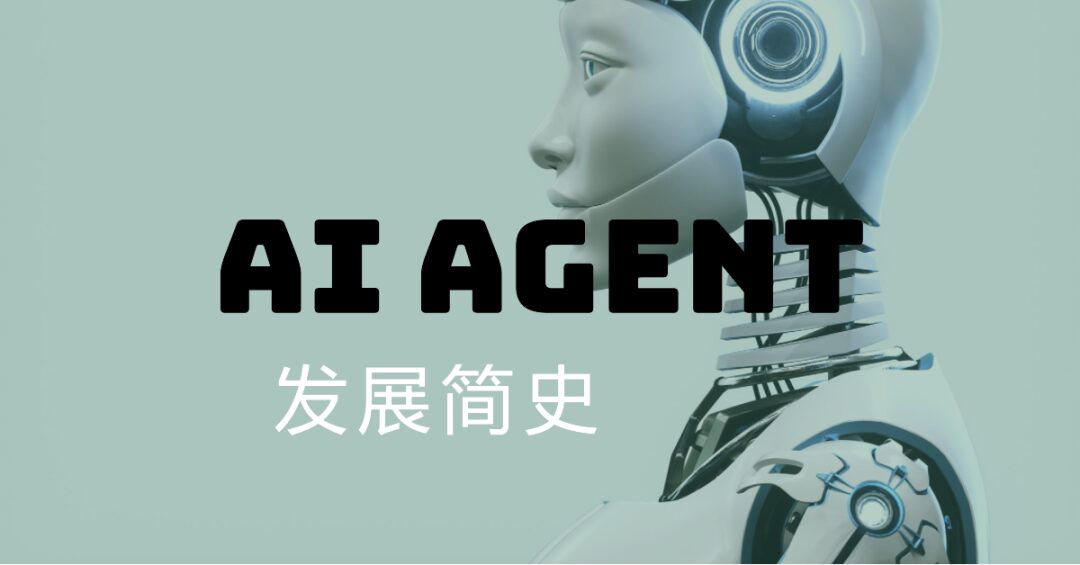
-
A Brief History of AI Agent Development, From Philosophical Enlightenment to the Realization of AI Entities
-
Want to understand the development history of AI agents? This brief history of AI agents is a must-read!
-
Finally, someone has clearly explained the history of AI agents; make sure to save it!
-
A Brief History of AI Agents, Uncovering the Journey from Philosophical Enlightenment to Realized AI
-
To fully understand the development of AI agents, you must read this brief history!
-
You might not believe it, but the earliest appearances of AI agents can be traced back to the “Tao Te Ching” and Aristotle’s philosophy.
Since the emergence of AutoGPT, discussions about AI agents have never ceased in the industry.
Lilian Weng, in her six-thousand-word blog post, provided a systematic introduction to AI agents, redefining contemporary AI agents based on large language models as a new set of four components: “LLM + Memory + Task Planning + Tool Use.”
Once the principles of AI agents are understood, the exploration and communication regarding AI agents naturally increase. Especially after experiencing a period of skepticism about the practical implementation of LLMs, now whenever AI agents are mentioned, entrepreneurs’ eyes light up, investors are enthusiastic, and large enterprises are eager to try.
Thus, AI agents have become a new focus known to all sectors following LLMs.
From everyone discussing large language models to casually mentioning AI agents indicates that attention on LLMs has genuinely shifted to the application layer, where practical implementation has become the main event for exploration across all fields.
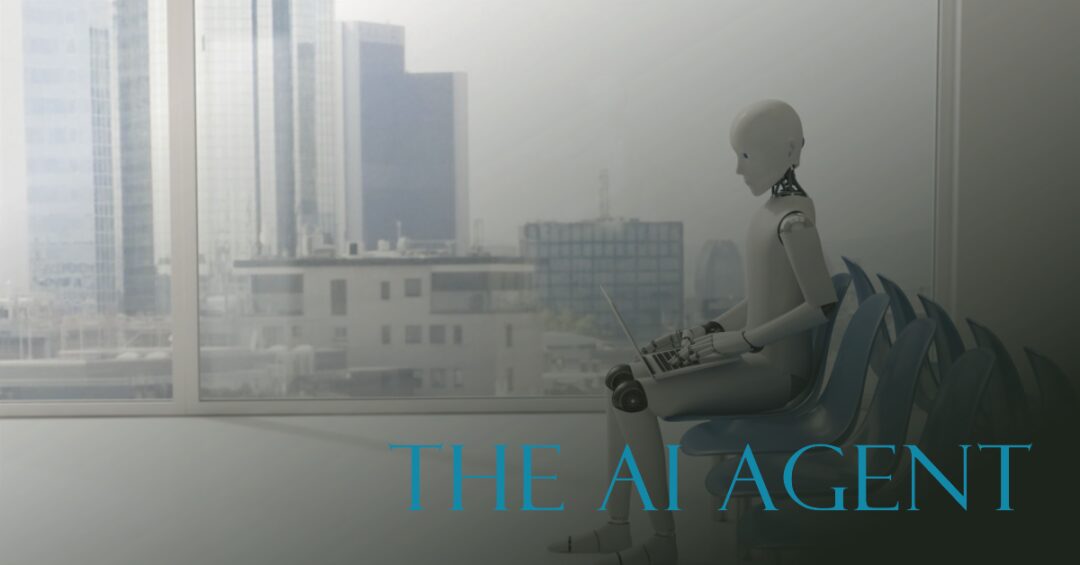
When discussing AI agents, many believe they are products of LLMs, as most people’s first exposure to agents comes from open-source agent programs based on GPT-4, such as AutoGPT, BabyGPT, and GPT-Engineer.
However, those who understand AI agents should know that the concept of agents is not a modern invention; it is the result of the continuous evolution of the concept of intelligent entities alongside artificial intelligence.
Some believe AI agents originated in the 1980s when Wooldridge and others introduced agents into artificial intelligence, while others argue that the earliest concept of agents should trace back to the 1950s when Alan Turing expanded the idea of “highly intelligent organisms” to artificial intelligence.
There are also papers (see Fudan University’s “The Rise and Potential of Agents Based on Large Language Models: A Review,” available at the end of the article) that trace the earliest concept of agents back to Denis Diderot’s theory of the “smart parrot.”
From a philosophical enlightenment perspective, the concept of agents can be traced back to the teachings and works of thinkers such as Zhuangzi, Aristotle, and Laozi between 280 BC and 485 BC.
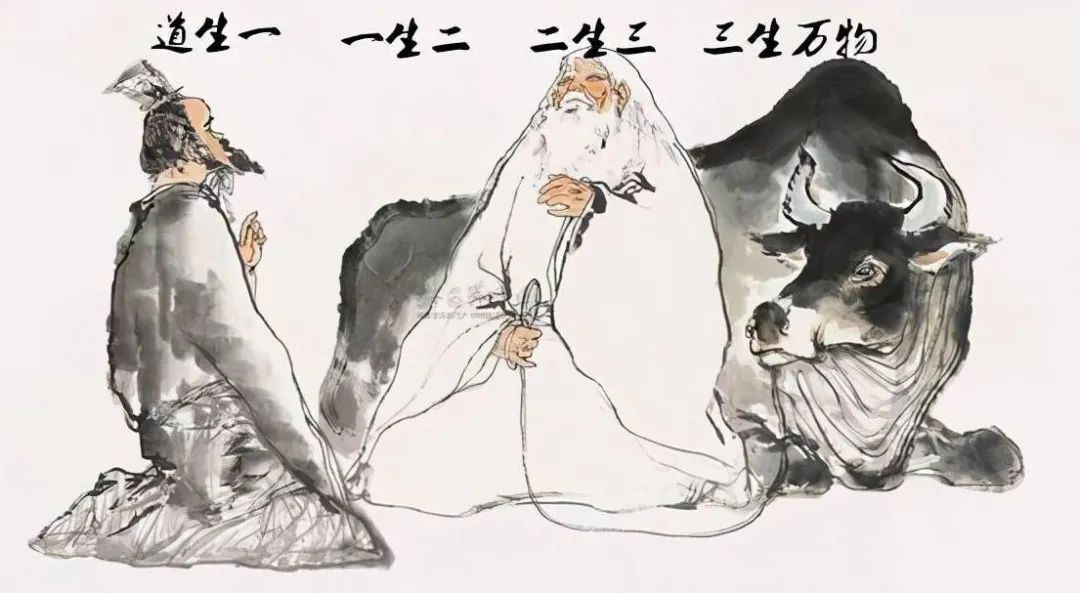
From these viewpoints, teachings, and papers, a general outline of AI agent development can be organized along the direction from philosophical thought to artificial intelligence entities.
On this basis, Wang Jiwei’s channel summarizes this brief history of AI agents to provide a more comprehensive understanding of AI agents.
The full text is approximately 7000 words; the article is somewhat lengthy, so it is recommended to save it for later reading. If you find it useful, don’t forget to like, share, and save it.
Origins: The Philosophical Enlightenment Stage
“Agent” is a concept with a long history that has been explored and explained in many fields.
The earliest origins of AI agents should be explored from the philosophical realm that inspires human thought. Some papers trace it back to around 350 BC during the time of Aristotle, when some philosophers described entities with desires, beliefs, intentions, and the capability to act in their philosophical works.
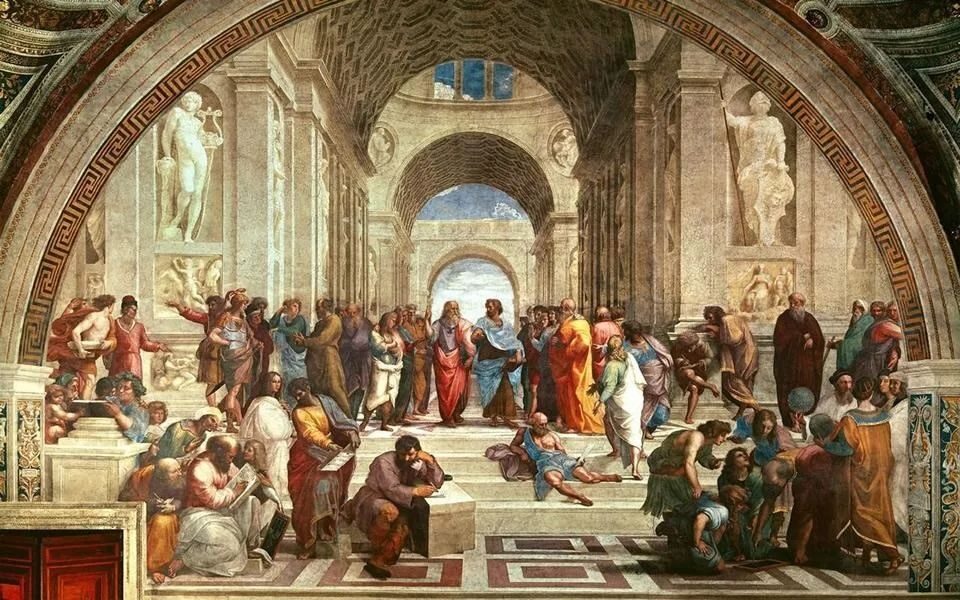
Looking for traces of agents in the writings of ancient philosophers can take us back to around 485 BC during China’s Spring and Autumn period, where the shadow of intelligent entities can also be seen in Laozi’s influential work, the “Tao Te Ching.”
In chapter 42 of this book, it states: “The Dao produces One; One produces Two; Two produces Three; Three produces all things.” From the perspective of modern computational science, the “Dao” it describes might indeed be an entity that is self-generating, encompassing all things, and capable of self-evolution, representing a typical autonomous intelligent entity.
In a later period, Zhuangzi, in his “Zhuang Zhou Dreams of Butterflies,” could not distinguish whether he was Zhuangzi or a butterfly, unable to tell if it was a dream or reality. Viewed through the lens of modern computational technology, this dream can be understood as a metaverse, where the butterfly in the dream includes all living entities, akin to generative intelligent agents in games like “Westworld.”
▲ Image generated by Bing, keywords: Zhuang Zhou Dreams of Butterflies
Moving to the 18th century, during the French Enlightenment, Denis Diderot also proposed a similar viewpoint: if a parrot can answer every question, it can be considered intelligent. Although Diderot wrote about parrots, everyone can understand that the “parrot” here does not refer to a bird but highlights a profound concept: that highly intelligent organisms can possess intelligence similar to that of humans.
Isn’t it interesting that what we think of as modern ideas about AI agents were actually contemplated and explored by ancient thinkers long ago?
Perhaps it is this line of thought that has led humanity to pursue various tools to an extreme, giving rise to creations such as the “Wooden Magpie” crafted by Lu Ban during the Spring and Autumn period, which could fly for three days and nights, the mechanisms of the Mohists, the wooden oxen and flowing horses of the Three Kingdoms, the guide car, the wooden person “waitress” from the Tang dynasty, and the various wooden figures that helped people in the Ming dynasty.
These early automated tools created by humanity do not possess the analytical and reasoning capabilities to act as intelligent agents. However, these ideas and practices that have existed from ancient times to the present reflect humanity’s enduring pursuit of intelligent entities or automation.
Through these thoughts, we can also learn that the philosophical concept of agents broadly refers to entities or concepts with autonomy, which can be artificial objects, plants, animals, or even humans.
Development: The Realization of Artificial Intelligence Entities
No matter where the earliest descriptions of agents originated, these philosophical ideas have inspired the development of modern agents to varying degrees.
In the 1950s, Alan Turing expanded the concept of “highly intelligent organisms” to artificial entities and proposed the famous Turing Test. This test is fundamental to artificial intelligence, aimed at exploring whether machines can exhibit intelligent behavior comparable to humans.
These artificial intelligence entities are typically referred to as “agents,” forming the basic building blocks of artificial intelligence systems. From this point on, agents mentioned in the field of artificial intelligence generally refer to artificial entities that can perceive their surrounding environment using sensors, make decisions, and then use actuators to take responsive actions.
With the development of artificial intelligence, the term “agent” found its place in AI research, used to describe entities that exhibit intelligent behavior and possess qualities such as autonomy, reactivity, proactivity, and social ability. Subsequently, the exploration of agents and technological advancements became the focus in the field of artificial intelligence.
The late 1950s to the 1960s was a creative period for artificial intelligence, and the programming languages, books, and films that emerged during this time continue to influence many people today.
After experiencing the first AI winter, a wave of interest in artificial intelligence emerged in the 1980s. During this time, various research breakthroughs occurred, and investments from government and other institutions began to increase, leading researchers to gradually explore AI agents.
However, this wave of enthusiasm lasted only seven years, leading to a second AI winter by 1987.
This cold wave persisted for many years; despite the lack of funding support for most institutions during this period, artificial intelligence continued to develop along existing technological paths.
Among them, AI agents were defined in 1995 by Wooldridge and Jennings as a computer system that operates in an environment, capable of acting autonomously within that environment to achieve its design goals. They also proposed that AI agents should possess four basic attributes: autonomy, reactivity, social ability, and proactivity.
Once AI agents were officially accepted in economics, they were further defined as systems capable of perceiving their environment and taking actions to maximize their chances of success. According to this definition, simple programs that can solve specific problems also qualify as “AI agents,” so robots that can play chess against humans are also considered a type of AI agent.
The AI agent paradigm defines AI research as “intelligent agent research,” which studies various forms of intelligence, surpassing the study of human intelligence.
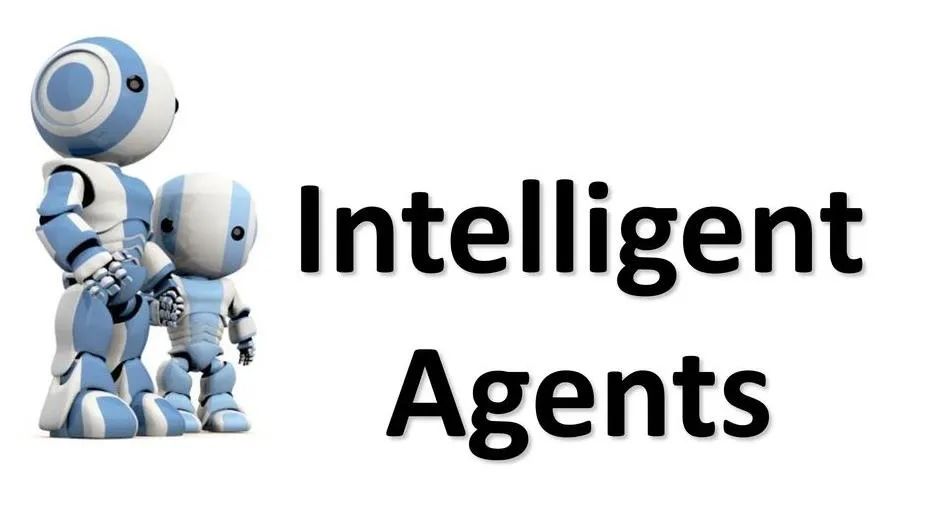
During the period when AI agents were endowed with “four basic attributes,” from 1993 to 2011, many impressive agent-based projects emerged based on the AI technologies of the time.
The timeline and brief descriptions of these projects are as follows:
-
1997: Deep Blue (developed by IBM) defeated world chess champion Garry Kasparov in a widely publicized match, becoming the first program to defeat a human chess champion.
-
1997: Windows released speech recognition software (developed by Dragon Systems).
-
2000: Professor Cynthia Breazeal developed the first robot capable of simulating human emotions through facial expressions, equipped with eyes, eyebrows, ears, and a mouth, called Kismet.
-
2002: The first Roomba was released.
-
2003: NASA landed two rovers (Spirit and Opportunity) on Mars, which navigated the Martian surface without human intervention.
-
2006: Companies like Twitter, Facebook, and Netflix began utilizing AI as part of their advertising and user experience (UX) algorithms.
-
2010: Microsoft launched the Xbox 360 Kinect, the first gaming hardware designed to track body movements and translate them into game directions.
-
2011: A natural language processing computer named Watson (created by IBM) was programmed to answer questions, defeating two former champions in the televised quiz show “Jeopardy!”.
-
2011: Apple released Siri, the first popular virtual assistant.
Evolution: The Transformation of AI Agents
With the advancement of AI technology, by around 2000, agents had diversified into several types.
Based on the varying degrees of perceived intelligence and capabilities, Russell, Norvig, Peter, and others categorized AI agents into five types in the book “Artificial Intelligence: A Modern Approach”:
Simple Reflex Agents: A simple type of agent that bases its actions on current perceptions rather than the history of perceptions. This type of agent is limited in intelligence, unaware of non-perceptual aspects of the state, generates and stores massive amounts of data, and cannot adapt to environmental changes.
Model-Based Agents: These agents operate using conditional action rules, working by searching for rules that satisfy conditions based on the current situation, typically consisting of two important factors: a model and an internal state. They can update their state by acquiring information about how the world evolves and how their actions affect the world.
Goal-Based Agents: This type makes decisions based on its goals or ideal situations, allowing it to choose actions that achieve the desired goals. These agents can confirm their ability to achieve goals through searching and planning, demonstrating proactivity.
Utility-Based Agents: The ultimate purpose of utility agents is their building blocks, used when the best action and decision must be taken from multiple alternatives. They consider the agent’s well-being and provide insights into how happy the agent is due to utility, thus taking actions that maximize utility.
Learning Agents: These agents have the ability to learn from past experiences and make decisions or take actions based on their learning capabilities. They gain foundational knowledge from the past and use this learning to act and adapt automatically. Generally composed of four parts: a learning element, a critic, a performance element, and a problem generator.
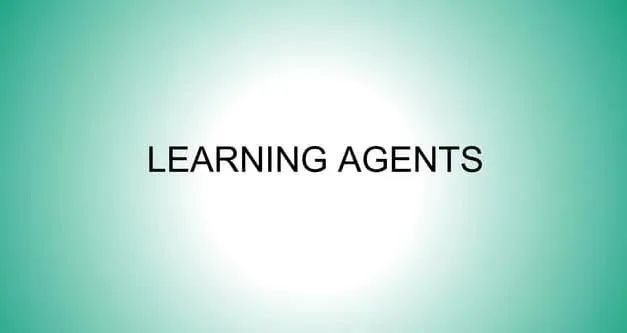
From these classifications and basic definitions, many AI tools and early intelligent programs can be categorized as a type of agent. This includes early AI tools like IBM’s Deep Blue used in chess competitions and later programs like AlphaGo, which were based on the latest AI technologies of their time.
Contemporary: LLM-Based Agents
In the 2012 ImageNet computer vision challenge, the AlexNet convolutional neural network deep learning model won first place, marking the true emergence of deep learning in the field of artificial intelligence.
In 2016, AlphaGo (an AI agent specializing in Go games) defeated European champion Fan Hui and world champion Lee Sedol, and was soon surpassed by its successor, AlphaGo Zero.
In 2017, Google introduced the transformer model.
In 2018, Google released BERT, based on the Transformer model, marking the dawn of large language models.
In 2019, Google AlphaStar reached Grandmaster level in the video game “StarCraft II,” outperforming all human players except for the top 0.2%.
In 2019, OpenAI released the natural language processing model GPT-2, followed by GPT-3, DALL·E 2, and GPT-3.5 in 2020 and 2022, with the popularity of ChatGPT providing new opportunities for the development and application of AI agents in the era of large language models.
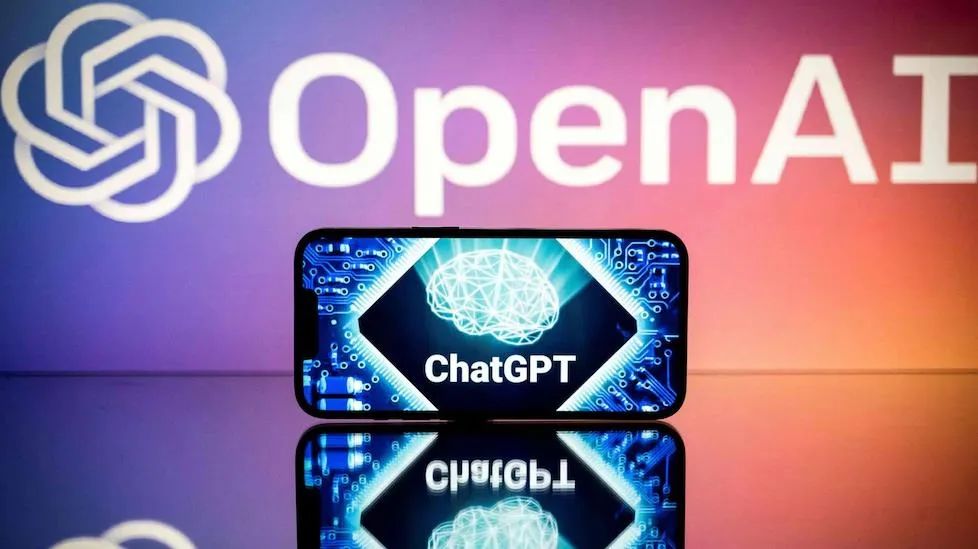
Starting from January 2023, global vendors have released multiple LLMs, including LLaMA, BLOOM, StableLM, ChatGLM, and many other open-source LLMs.
At the same time, the thousands of LLMs launched by global tech companies provide a broader foundation for the diversified applications of AI agents across various fields.
On March 14, 2023, OpenAI released GPT-4. By the end of March, AutoGPT emerged and quickly gained worldwide popularity.
AutoGPT is a free open-source project launched by OpenAI on GitHub, integrating GPT-4 and GPT-3.5 technologies to create complete projects through APIs.
Unlike ChatGPT, where users need to continuously ask questions to get corresponding answers, in AutoGPT, users simply provide an AI name, description, and five goals, and AutoGPT can complete the project by itself. It can read and write files, browse the web, review the results of its prompts, and combine them with its prompt history.
AutoGPT is also an experimental project by OpenAI to showcase the powerful capabilities of the GPT-4 language model. This led to more people becoming aware of and experiencing AutoGPT, gradually recognizing AI agents.
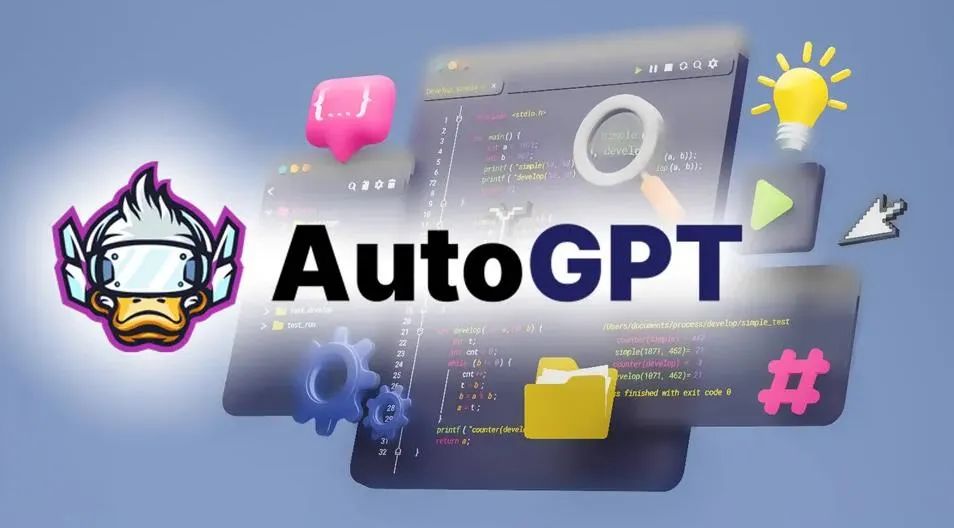
Since then, LLM-based AI agents have sprung up like mushrooms, with projects such as Generative Agent, GPT-Engineer, BabyAGI, and MetaGPT emerging. These projects have ushered in a new phase of development and application for LLMs, directing entrepreneurship and implementation towards AI agents.
In May, after OpenAI secured a new round of $300 million funding, founder Sam Altman revealed a greater focus on how to use chatbots to create autonomous AI agents, with plans to deploy related features into the ChatGPT assistant.
In June, Zuckerberg announced a series of technologies at an all-hands meeting that are at different stages of development, one of which is to release AI agents with different personalities and capabilities to help or entertain users.
At the end of June, Lilian Weng, head of OpenAI’s Safety team, published an article titled “LLM Powered Autonomous Agents,” detailing LLM-based AI agents and considering them one of the pathways for LLMs to evolve into general problem-solving solutions.
At this point, people finally gained a comprehensive understanding of AI agents, and the mysterious veil surrounding AI agents was lifted.
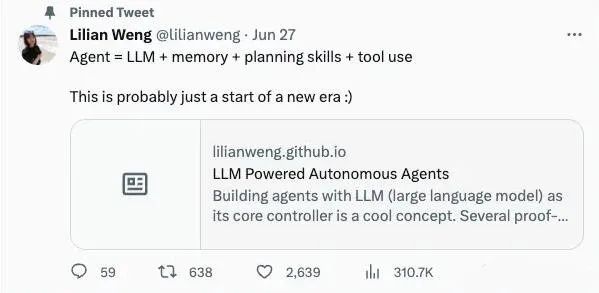
The exploration of AI agents in the field of artificial intelligence has never stopped. After each new breakthrough in AI technology, organizations have incorporated their exploration and application into new topics. For instance, with the emergence of deep learning and neural network technologies represented by AlphaGo, agent applications based on deep learning and neural networks have been developed in various fields such as gaming and healthcare.
In recent years, as large language models have made breakthroughs, many organizations have begun collaborating to create LLM-based agents following the release of BERT by Google and GPT-2 by OpenAI.
While we are still discussing AI agents, many AI agent frameworks and products have already emerged overseas. For example, Voiceflow, which recently completed $15 million in funding at the end of August, has become one of the most popular platforms for developers to build AI agents, with over 130,000 teams collaborating efficiently to create their AI agents.
From these AI agent building platforms, it is evident that many organizations are currently building or have built their own AI agents, and each organization can have multiple agents tailored to different business scenarios.
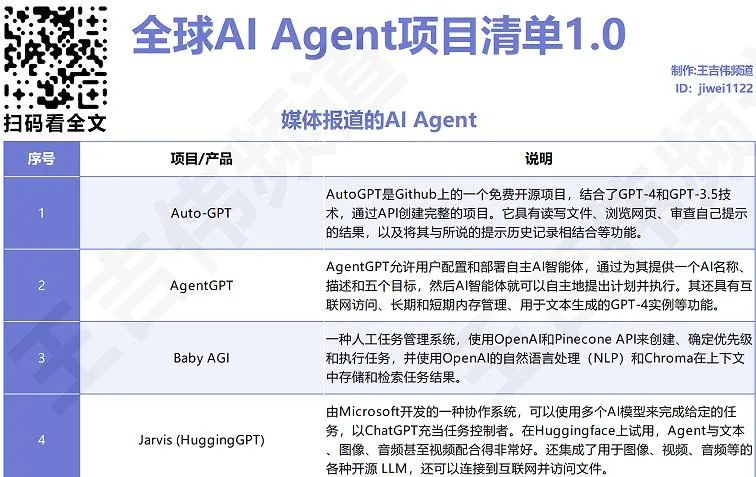
Wang Jiwei’s channel previously reviewed 60 AI agent projects worldwide, and a project list 1.0 has been compiled. This list will continue to be updated, and teams that have launched AI agents or enthusiasts of AI agents are welcome to collaboratively improve this list. (PS: Reply with agent+ to obtain the complete list image.)
Definition: Contemporary AI Agent Characteristics
Lilian Weng, in her blog post “LLM Powered Autonomous Agents,” provided a systematic overview of LLM-based AI agents. (PS: If you find it troublesome to search, reply with agent+ to get the article and its translation.)
Original article link: https://lilianweng.github.io/posts/2023-06-23-agent/
She defines agents as a combination of LLM, memory, task planning, and tool use, where LLM is the core brain, and memory, planning skills, and tool use are the three key components of the agent system. She also provides detailed pathways and explanations for the implementation of each module.
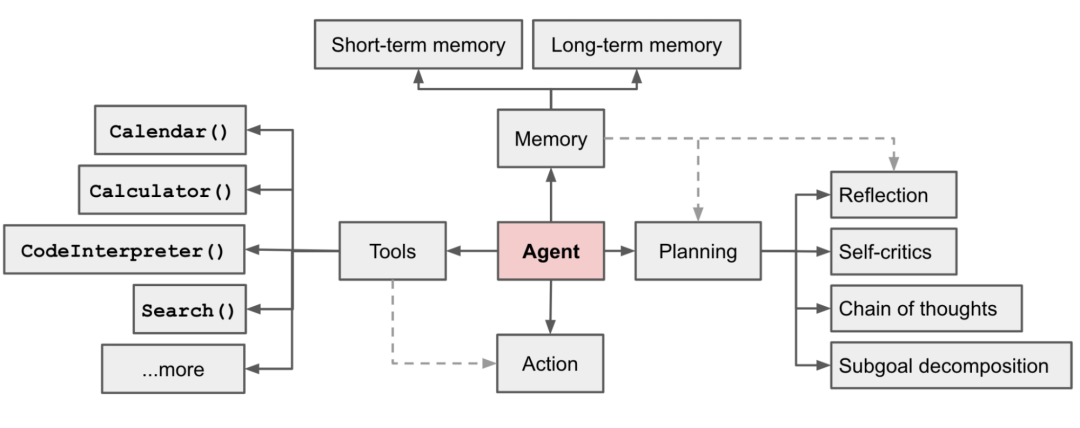
From the article, it is clear that the AI agents we refer to today are essentially a control system for LLMs to solve problems. The core capabilities of LLMs are intent understanding and text generation; if LLMs can learn to use tools, their capabilities will greatly expand. The AI agent system is such a solution that allows the “super brain” of LLMs to truly become a “universal assistant” for humans.
As AI has evolved into the era of large language models, many AI tools appear to have preliminary agent capabilities. Although AI tools, including robots and agents, are designed to automate tasks, specific key features distinguish AI agents as more complex AI software.
Industry experts believe that when AI tools possess the following characteristics, they can be regarded as AI agents:
-
Autonomy: AI virtual agents can independently perform tasks without human intervention or input.
-
Perception: Agents perceive and interpret their environment through various sensors (such as cameras or microphones).
-
Reactivity: AI agents can assess their environment and respond accordingly to achieve their goals.
-
Reasoning and Decision-Making: AI agents are intelligent tools that can analyze data and make decisions to achieve goals. They use reasoning techniques and algorithms to process information and take appropriate actions.
-
Learning: They can learn and improve their performance through machine, deep, and reinforcement learning elements and techniques.
-
Communication: AI agents can communicate with other agents or humans using various methods, such as understanding and responding to natural language, recognizing speech, and exchanging messages through text.
-
Goal-Oriented: They are designed to achieve specific goals, which can be predefined or learned through interaction with the environment.
Under this broader definition, the environments in which AI agents exist will become more extensive, and their varieties will increase.
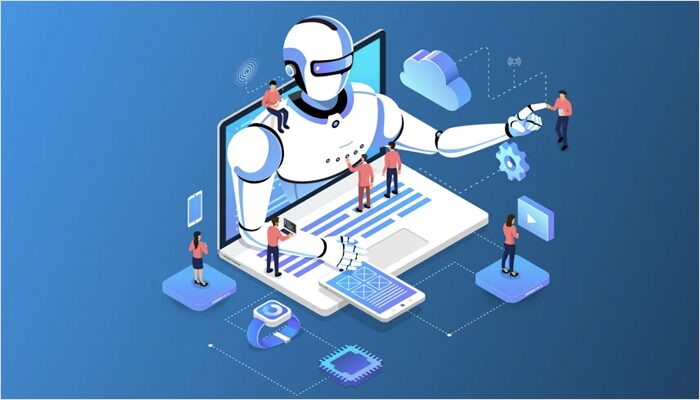
Meanwhile, supported by large language models, AI agents have gradually given rise to autonomous agents and generative agents.
Autonomous agents, such as AutoGPT, can automatically execute tasks and achieve expected results based on natural language requests from people. In this collaborative model, autonomous agents primarily serve humans, acting more like efficient tools.
Most of the agents currently being discussed are LLM-based autonomous agents, which are considered one of the most promising paths toward artificial general intelligence (AGI).
If AutoGPT opened the curtain on autonomous agents, the “Westworld simulation” created by researchers from Stanford and Google in April this year has paved the way for generative agents. In this town, 25 AI agents joyfully walk, date, chat, dine, and share the day’s news.
▲ Screenshot of the generative agent Westworld simulation running
Generative agents are built on LLMs such as GPT-3 and BERT, storing complete records of agent experiences in natural language. The architecture of generative agents includes three main components: observation, planning, and reflection. These components work together to enable generative agents to generate realistic and consistent behaviors that reflect their personalities, preferences, skills, and goals. Additionally, this architecture allows for natural language communication between users, agents, and other agents.
In simple terms, generative agents are like humanoid robots in the TV series “Westworld” and NPCs in “Free Guy”; they live in the same environment, have their own memories and goals, and interact not only with humans but also with other robots.
Future: AI Agents Everywhere
The core of agents lies in LLMs, meaning that the capabilities of large language models determine the capability radius of AI agents. Therefore, currently, agents based on GPT-4 appear more intelligent. In the future, as more large language models are perfected, iterated, and optimized, the capabilities of agents built on these LLMs will naturally become stronger.
Future AI agents will primarily exhibit the following characteristics:
-
More Intelligent, Autonomous, and Adaptable: They will be able to learn and improve their behaviors, make optimal decisions based on different contexts and users, and handle uncertainty and complexity.
-
More Human-like, Friendly, and Trustworthy: They will be able to understand and express emotions, establish and maintain relationships with users, and adhere to ethical and social norms.
-
More Diverse, Specialized, and Collaborative: They will be able to provide specialized services or assistance for different fields and tasks, and collaborate and coordinate effectively with other AI agents or humans.
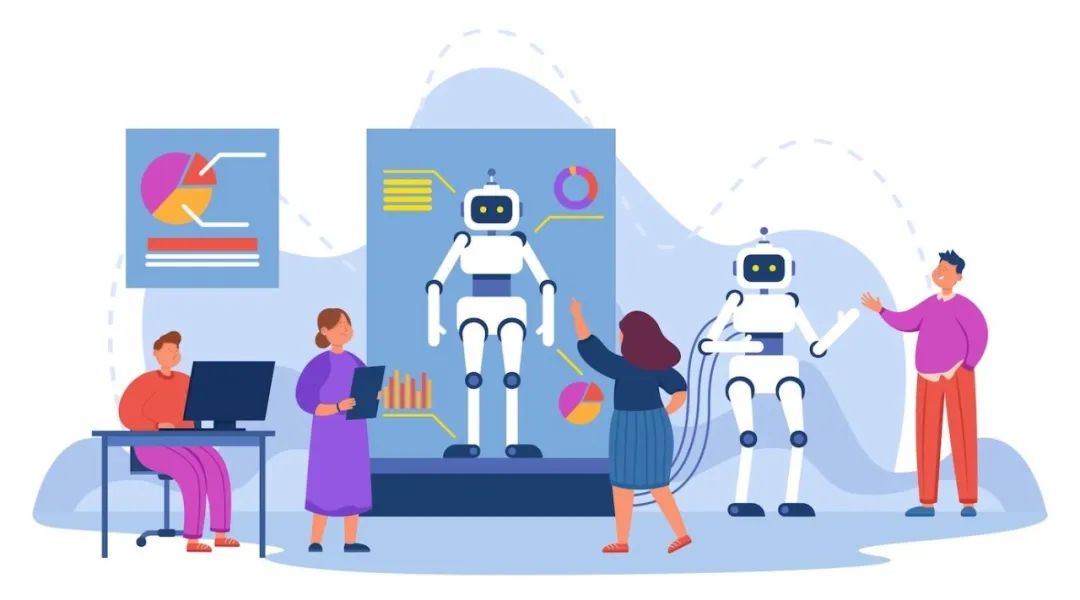
Agents will become the main form of application for large models across various industries and fields. The development and application of LLMs will revolve around agents presented as tools or assistants. As agents emerge in standardized product forms, organizations will find it easier to adopt and apply AI agents.
Related enterprises and organizations can build domain-specific agents based on the large language models or vertical models they introduce, helping clients efficiently unleash the capabilities of LLMs. They can also create AI agent platforms and communities for internal or client-facing use, facilitating the on-demand construction of required agents.
More AI agent building platforms will encourage the emergence of numerous agents, making it easier for individuals to build and apply agents. In the future, everyone will be able to create personalized agents through various agent platforms, enhancing communication and collaboration through more personalized features and services, expanding knowledge and skills.
It may even be possible to build multiple different agents for various business scenarios, allowing these agents to work together; multi-agent systems can produce more accurate results and accomplish more complex tasks.
AI agents disregard industry and business scenarios; wherever LLMs can be applied, corresponding agents can be constructed. They can be applied across various industries, such as education, healthcare, finance, manufacturing, and entertainment, helping to improve efficiency, reduce costs, and create value.
In the future, AI agents may become more intelligent, adaptive, and diverse, capable of addressing more complex problems and scenarios, forming closer collaborations and symbiosis with humans.
As Lilian Weng mentioned in her blog post, AI agents can evolve LLMs from “super brains” to “universal assistants” for humanity, which means that LLM-based agent assistants will serve more people and organizations in the future.
With the widespread application of AI agents, human-computer interaction in the era of large language models will upgrade to an automated collaboration system between humans and AI agents. This new form of human-computer collaboration can be termed human-machine agents, which will promote further upgrades in the production structure of human society, subsequently influencing various aspects of society.
At the same time, an intelligent network capable of communication and autonomously/automatically executing tasks will become the next phase of the internet, with AI agents serving as intelligent tools for humans to interact with and execute tasks.
The future trend suggests that AI agents will likely appear in various scenarios of human work, learning, life, and entertainment, and everyone will be equipped with an intelligent assistant based on the AI agent system. The collaborative scenes between humans and machines depicted in movies like “Iron Man,” “Interstellar,” and “Star Wars” will truly become a reality.
This will also represent a multi-scale market.
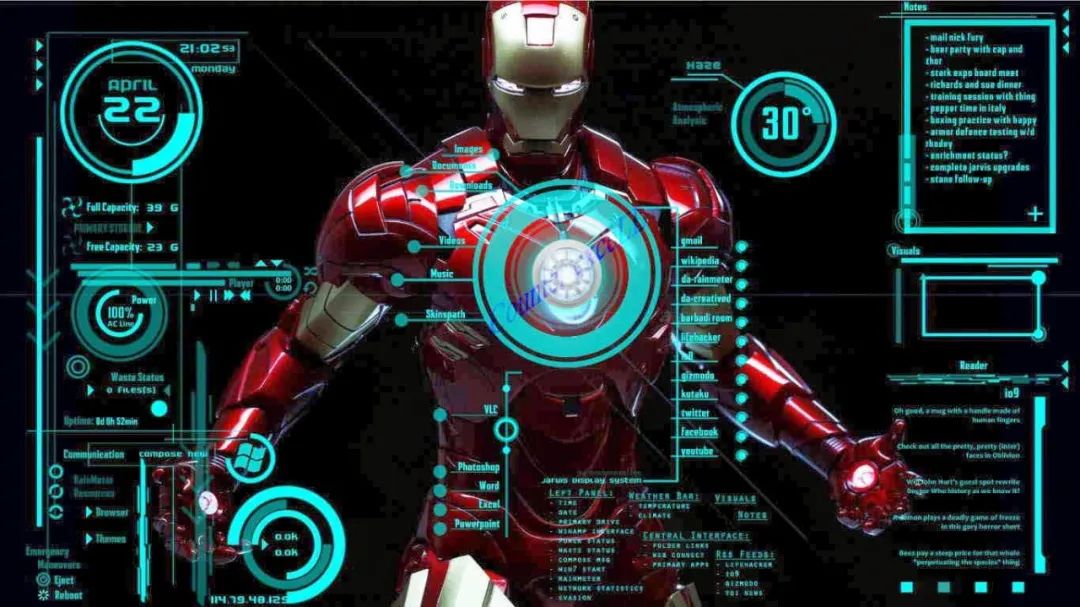
In Conclusion: I originally intended to discuss the evolution of “agent games” and the “boundaries between humans and agents” in this article. Due to space limitations and the relevance of these two topics to this article, I can only explore them in a new topic with everyone. For those interested in these topics, feel free to add me on WeChat mcjave for discussion.
References: Fudan University’s NLP team paper “The Rise and Potential of Agents Based on Large Language Models: A Review”
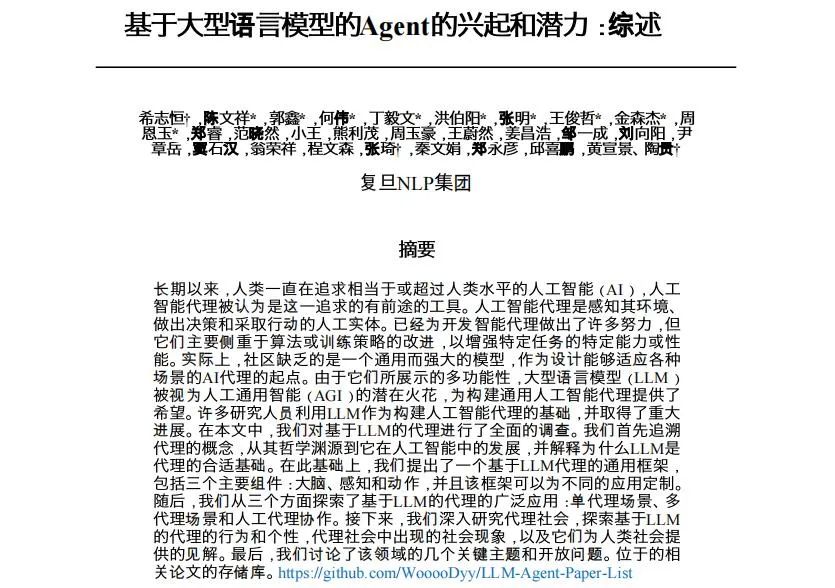
1. The Lifecycle of Modern RPA Built on AI: How Long Will It Last Under the Influence of Generative AI?
2. Multiple vendors integrating ChatGPT, with the integration and fusion of generative AI becoming a new trend in RPA technology.
3. The new business logic on large model APIs, how generative AI transforms organizational operations.
4. Digital employees, human-machine collaboration, hyper-automation: 10 digital tags on RPA.
AIGC Research Series Articles
Integrating ChatGPT with RPA, generative AI + automated processes enhance AIGC value.
Business processes will be transformed by generative AI, with ChatGPT leading the way in changing organizational operations.
More organizations are integrating ChatGPT and other generative AI, making generative automation the new standard for enterprise operations.
The AIGC model is affecting more organizations; ten cases help you deeply understand generative AI.
Multiple vendors integrating ChatGPT, with the integration and fusion of generative AI becoming a new trend in RPA technology.
The lifecycle of modern RPA built on AI: How long will it last under the influence of generative AI?
From the ChatGPT data leak incident, the importance of organizational security and stable automation is highlighted.
The new business logic on large model APIs, how generative AI transforms organizational operations.
What is the relationship between generative AI and customer experience? How does it affect customer experience? This article explains it clearly.
From various business scenarios and actual cases, see the application of generative AI in the financial sector.
Generative AI sweeps PPT production; office productivity is undergoing a major transformation, along with 20 popular AI PPT production tools.
From RPA + AI to RPA x AI, Hongji embarks on a new path of LLM integration.
With the arrival of the LLM era, will generative AI become a catalyst for hyper-automation to flourish?
AIGC continues to thrive, with large models being launched in succession, creating a massive market that evolves the computational supply model.
From “human + RPA” to “human + generative AI + RPA,” how does LLM influence human-machine interaction in RPA?
Generative AI is disrupting the decoration and renovation field [with 28 AI decoration design tools attached at the end].
From the characteristics of LLM and the essence of digital transformation, see how large language models impact digital transformation.
Global RPA financing overview for the first half of 2022-2023: Overseas projects account for 67%, with a total of 16.5 billion yuan.
From integrating and consolidating multiple LLMs to releasing self-developed models, how is the progress of RPA and LLM integration?
Upstream and downstream industries are working together as LLM advances to the edge, accelerating the landing of large language models that benefit hyper-automation.
From AI models changing outfits to AIGC empowering operations, generative AI is penetrating the entire e-commerce industry chain.
[Ten Thousand Word Long Article] A comprehensive overview of global AI agents, a must-reference for entrepreneurship in the era of large language models.
The ultimate development direction of RPA focuses on AI agents; the era of hyper-automation agents has already begun.
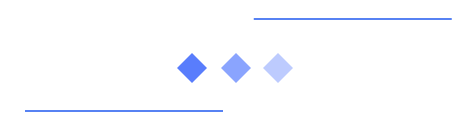
-
Looking forward to likes, views, comments, and shares; your support is my motivation. -
Encouraging active comments; your messages can become topics of discussion.
-
Welcome to read other articles, which may inspire more of your thoughts.
Click the lower left corner “Read the Original” to view the AIGC research series articles; reply with [Join Group] to apply to join the AIGC industry application exchange community.