On January 9, AI Analysis successfully held the 2024 AI Analysis · AI and Large Model Summit Forum, inviting experts, scholars, corporate representatives, large model vendors, and practical experts from various fields to share cutting-edge technological advancements and leading enterprise application scenarios and practical experiences.
Today, we present an introduction and interpretation of AI Agent construction and business empowerment.
Featured Speaker|Zhou Jian, Founder & CEO of Lanma Technology
The content has been condensed. For further communication with experts and to access the full video recording, please scan the code.
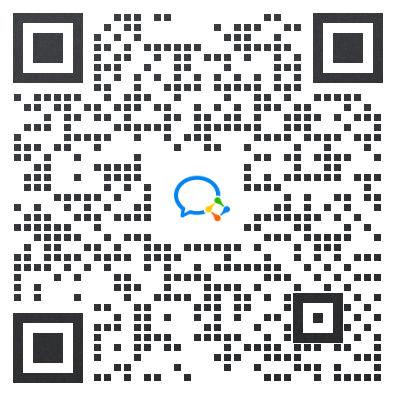
This sharing mainly focuses on our practices and related thoughts regarding AI Agents in the industry during the past year of 2023.
With the rapid development of technology, our understanding of software development models is continuously deepening. In recent years, the emergence and widespread application of large models represent a deep compression of big data, signifying further advancements in machine learning and deep learning, which we can regard as a predictive behavior. Previous second-type big data systems were more like information recording systems capable of recording various processes and IoT device information within enterprises.
Current large models are more about compressing vast amounts of data; they cannot entirely replace the human brain, so their role should not be overstated. Recently, some viewpoints suggest that the emergence of large language models indicates that we can convert computational power into intelligence and regard this intelligence as infrastructure. With strong large language models as support, we only need to directly increase computational power to expand this intelligence into more fields.
Just like cloud computing, by increasing computational power tenfold, intelligence can be “copied” into ten parts, which is the charm of large language models. However, in practical applications, we also face challenges from the third type of system—action systems. Within enterprises, every employee needs to confront various IT environments, using various systems, versions, functions, etc., which itself is an environment. Therefore, we need to use multiple tools to achieve the same goal, requiring us to handle different situations in different environments and to explore and solve related problems.
Before truly realizing this process, it is important to know that AI Agents must not only possess intelligence but also the ability to interact with complex and changing environments, including learning and exploration.
After a year of exploration, many enterprises face various challenges when implementing large language models. The concepts involved are very rich, covering pre-training, vertical industry large model fine-tuning, Prompt, RAG, and even multi-Agent technical terms. In fact, many practitioners are currently discussing the value issues, including the value of large models and what practical business value they can bring to enterprises. How can our enterprises reach the stages of being large model Friendly or AI Ready in the face of these issues?
We might start by re-examining the definition of the AI process. In this process, we selected three different perspectives that might be familiar to the public to explain the AI process. Specifically, we initially defined AI Agents as the process of human-machine collaboration, from the AI 1.0 era to the present, such as facial recognition, OCR, etc., which we consider embedded applications.
In the industry, not all business processes require the use of AI. Microsoft’s Copilot project actually points out that software like Excel, Word, and PPT can assist humans in completing work in certain situations. However, we believe that the main characteristic of an Agent lies in its understanding of the domain model, allowing it to plan autonomously. For example, if instructed to arrange next week’s schedule, the AI Agent might automatically gather schedule information from your calendar system for next week and, based on this, look for suitable flights and hotel options, ultimately determining the itinerary according to personal preferences. We believe this could be the most important characteristic of an AI Agent. Of course, we must pay attention to technical considerations during this process.
Similarly, as mentioned earlier, we believe the key issue is the domain model. Large language models are more used for interaction with humans, enabling machines to adapt to humans, but they cannot solve domain model issues. For example, in recruitment scenarios, common resume evaluations, how to define 985, 211 universities, job stability, etc., are all domain model issues. However, these problems are not suitable for solving through large-scale resume datasets trained on large language models; traditional methods such as vector databases, traditional search technologies, and database technologies may instead better address the aforementioned issues.
The content described above primarily addresses product-level descriptions. In practical operations, we find that implementing AI Agents also requires meeting certain conditions.
First, information technology is the foundation of digitization, and digitization is the foundation of intelligence, with the prerequisite being the need to have accumulated expert knowledge. For example, if we want to build a conversational BI for a banking department, we might expect to first have a metrics database. If a metrics database has not been established, or if there is ambiguity in the definitions of metrics within the enterprise, then the AI Agent’s ability to respond to user inquiries will become very difficult. Similarly, many knowledge consulting firms convert a large amount of knowledge into document products or FAQs, which are prerequisite conditions. If there is a lack of unified consensus on these terms and metrics within the enterprise, teaching the Agent and then empowering different business personnel to complete the task will become extremely challenging.
Second, flexible interaction based on CUI.Everyone today realizes that with large language models, we have the opportunity to let machines adapt to humans rather than making humans adapt to machines. When building AI Agents, we need to ensure that the Agent can meet human needs, with reasonable expectations of human capability, which can be addressed through value alignment and other means.
Third, after completing the aforementioned two tasks, AI Agents can actually participate deeply in key aspects of business processes, providing a decision support process that is comprehensively recorded. This method is essentially an important data source, reflecting the digitization of employee behavior. In fact, employee behavior has not yet been digitized; many still rely on emails, web pages, and other tools to complete work tasks, which is a manifestation of processes not being digitized, making it difficult to conduct in-depth optimization. However, if we can assist business personnel in completing business tasks and activities through automation technologies, we will have the opportunity to accumulate data and further improve expert knowledge.
We believe that today’s large language models have significant limitations, especially in scenarios where privatized deployment is necessary. In situations where GPT-4 cannot be used, we can only choose open-source models or privatized deployment versions of domestic large models. To this day, a reasonable expectation is that under the premise of having a large number of employees in certain single positions, skills can be imparted to the Agent by experts, who in turn empower grassroots business units, teaching these skills to grassroots employees, helping them progress from beginner to intermediate levels of competence.
Now let’s discuss a practical implementation case.One of our benchmark clients, the Jinguang Group, aims to evaluate the financial statements of suppliers. Whether it is suppliers, supplier distributors, customers, or banks’ credit reviews or fund investment companies, they all have clear requirements for financial statements, including equity structure, accounts receivable profit margins, and other situations, which essentially involve financial health evaluation.
First, we need to conduct sampling selection work, which includes various sources such as Word, PPT, PDF, etc. Our task is to extract key indicator data from key areas. Next, professionals guide how to understand and interpret these indicators. For example, we may not be clear about the relationship between accounts receivable and operating income ratios or other related indicators. At this point, guidance from professionals becomes crucial.
Once the indicators and corresponding sampling are completed, frontline business employees conducting financial health condition audits can propose what they consider reasonable suggestions based on relevant regulations, eventually giving green, red, or yellow light recommendations. This will enable us to leverage computational power to create a simulated expert effect that achieves similar outcomes even without an expert present, allowing us to quickly and steadily achieve goals in business processes.
What we mentioned here is the entire financial risk management method of the supply chain. Currently, we have implemented and successfully landed a case that involves information extraction, decision support, risk assessment, knowledge input, execution process feedback, and overall core risk control indicator monitoring. In fact, this is the Augmented-Connected Workforce, which encapsulates the knowledge of professionals through AI Agents to process various formats of three financial statements on a large scale and digitizes the execution process based on pre-set business rules and personalized business rules, presenting the discussion results in text form, providing references, and conducting conversational data analysis.
Finally, to summarize, within the entire framework structure of Agents, problems can be addressed through multiple levels. Business experts will focus on standard operating procedures (SOP) for business processes, focusing on four categories: data, documents, applications, and processes. We will establish knowledge centers for different types of business objects and apply capabilities based on large language models. Meanwhile, in the task center, we will integrate relevant content using the embedded capabilities mentioned earlier, which is also the basic logic of our product design.
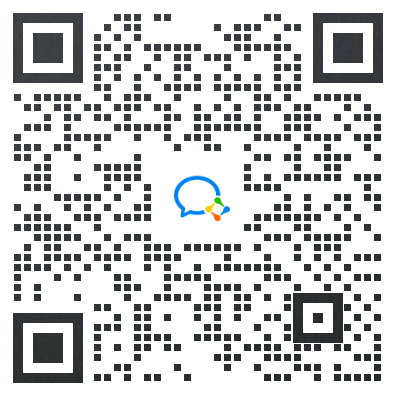
⩓
Long press the QR code to receive the complete video recording and courseware
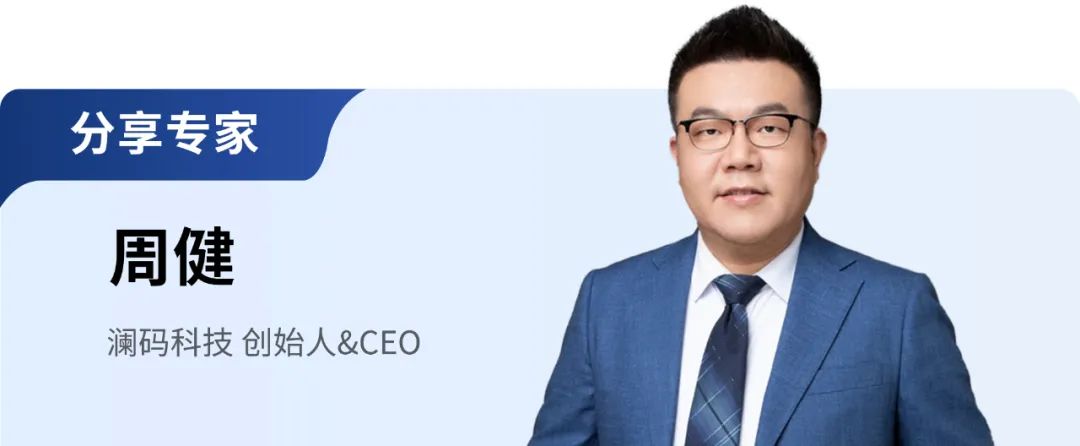
Bachelor’s and Master’s degrees from the Department of Computer Science, Shanghai Jiao Tong University. In 2002, won the ACM International Collegiate Programming Contest World Championship, being the first Asian team member to win this competition. Joined Google headquarters in the US in 2006, responsible for optimizing search quality for Chinese websites. Later served as R&D director and CTO in companies like Alibaba Cloud, MediaV, Yitu Technology, and Hongji RPA.
Ten years of continuous entrepreneurial experience, as the 10th employee of Yitu, and CTO of Hongji RPA, possessing rich experience and successful project cases in AI, big data, and enterprise services.
Note:Click the bottom left corner“Read the original text” to receive the expert’s complete recording..