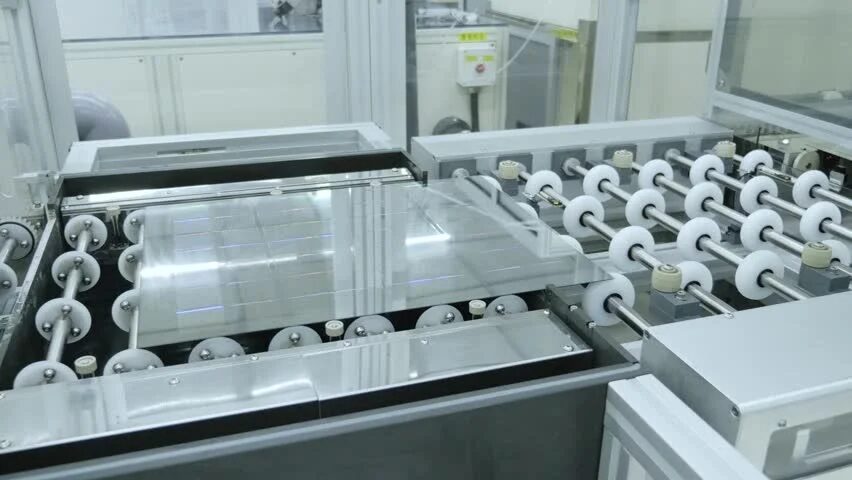
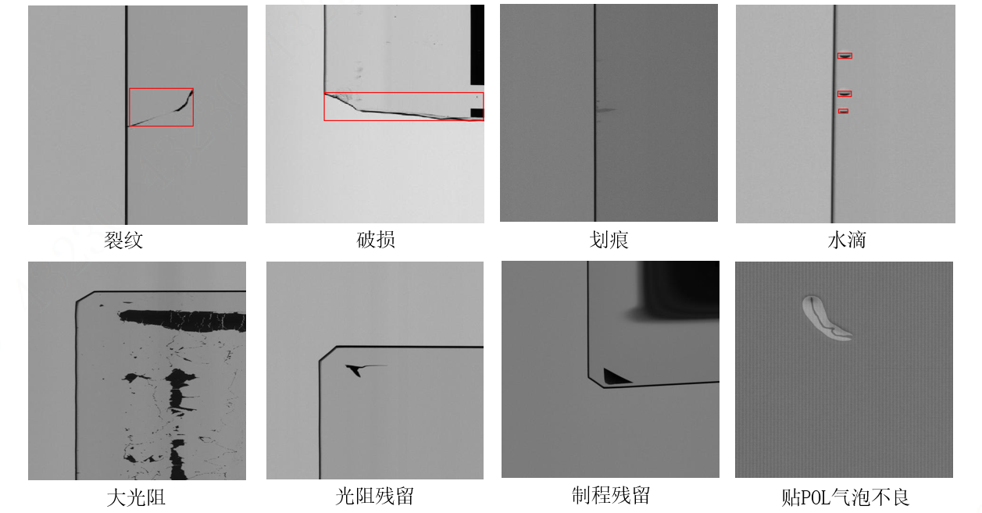
Large product area and high production capacity make it impossible for manual inspection of every panel, posing a risk of missed detections.
Before cutting, LCD panels typically have a large area. For example, a 10.5-generation line can have a panel area of up to 3370mm*2940mm, making manual inspection extremely difficult and prone to fatigue, thus failing to ensure production capacity and accuracy.
Panel defects can easily lead to product breakage.
During the production of LCD panels, defects such as edge chipping, breakage, cracks, and scratches may occur. If not detected and addressed promptly, they can lead to glass breakage within the main production equipment in subsequent processes, necessitating downtime for maintenance and cleanup, resulting in economic losses.
The locations and types of defects cannot be statistically analyzed or digitized.
Manual inspection lacks image documentation, leading to poor consistency in detection standards and hindering product traceability.
Difficulty in recruitment and high labor costs.
As the average wage level continues to rise, labor costs increase annually, and recruitment becomes challenging due to high employee turnover. Additionally, manual inspection poses significant harm to workers’ eyes, resulting in high turnover rates and recruitment difficulties.
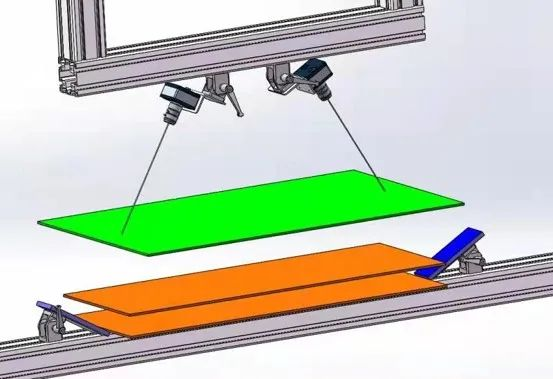
Increase Efficiency
Deep learning algorithms can more accurately identify production hazards such as edge chipping, thus preventing production line downtime caused by glass fragments and eliminating false alarms caused by misidentification, thereby improving production efficiency.
Reduce Costs
Avoid damage to equipment caused by glass breakage, reduce the costs associated with downtime, and lower repair costs for equipment.
Improve Quality
Reduce product quality issues caused by surface scratches and stains affecting circuit production and liquid crystal imaging.
Enhance Processes
By digitizing quality inspection, enhance data traceability and analysis capabilities, providing strong support for improving production processes.
LCD Screen Appearance Defect Detection Scheme
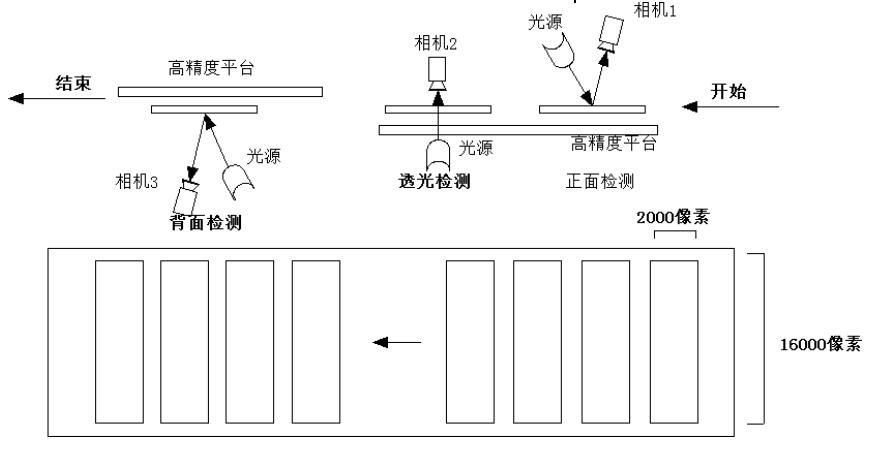
1. Open the product to 180°, placing the LCD screen horizontally on the stage; 2. Start detection: the stage moves, and the camera begins to capture images; 3. After capturing, the stage resets; 4. Detection software analyzes the quality of the LCD screen; 5. Based on the analysis results, provide OK or NG judgment results for the product; 6. Operator: performs sorting and re-inspection based on the results;
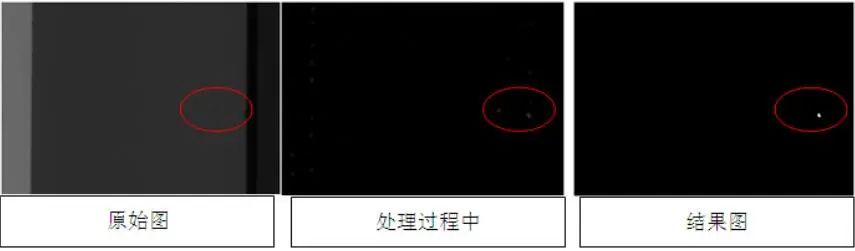
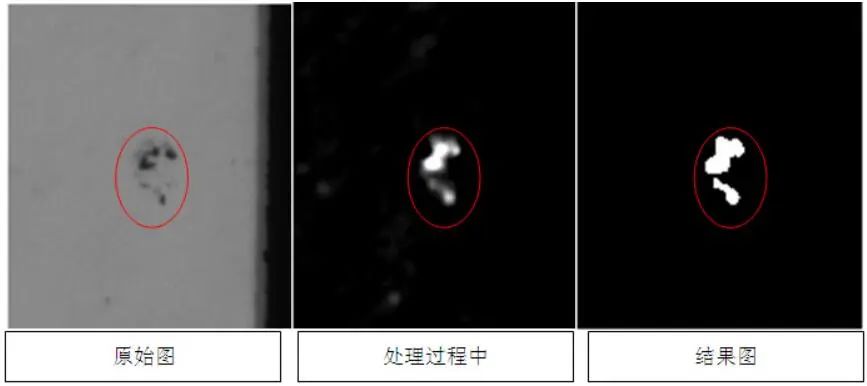
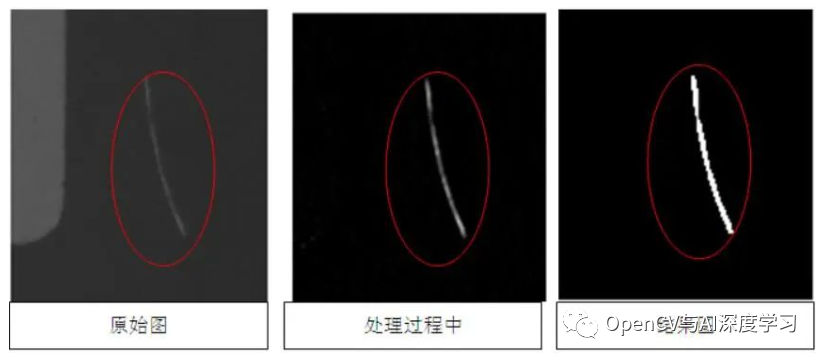
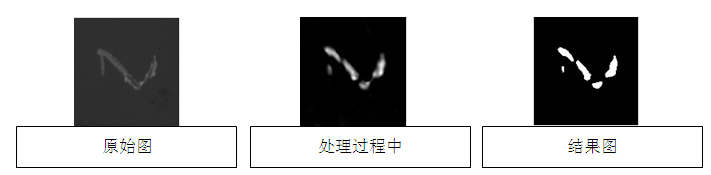
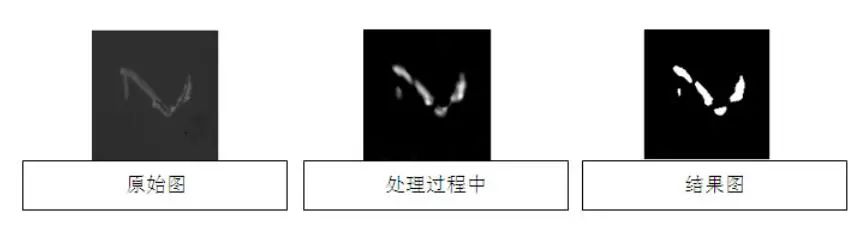