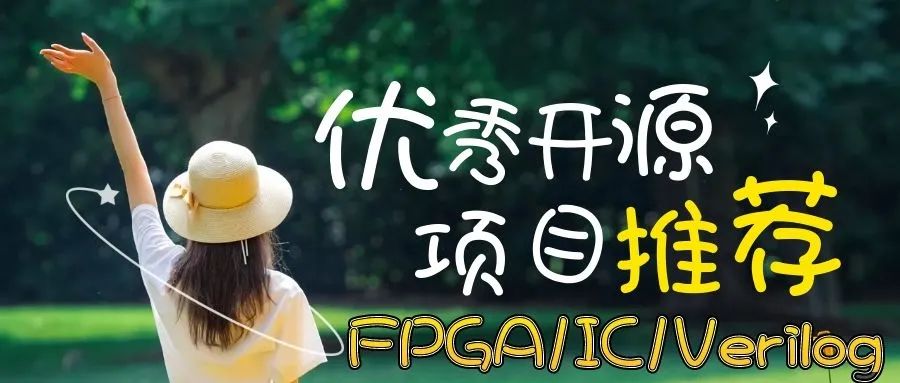
Introduction
Check out Why FPGA/ADC Communication Prefers GPMC Interface in Industrial Applications? to learn about TinyML~
Today, I will introduce several open-source projects related to TinyML.
TinyML Cookbook
https://github.com/PacktPublishing/TinyML-Cookbook
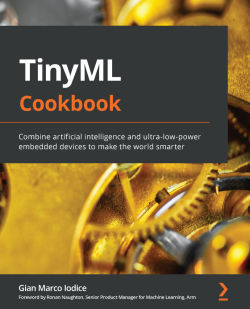
Overview
This book is about TinyML, a rapidly evolving field that lies at the unique intersection of machine learning and embedded systems, enabling AI to be applied in ultra-low-power devices such as microcontrollers.
TinyML is an exciting field full of opportunities. With a minimal budget, we can bring life to objects that interact cleverly with the surrounding world, improving our way of life. This book aims to eliminate barriers through examples, allowing developers without embedded programming experience to get started with TinyML. Each chapter will be an independent project to learn how to use some core technologies of TinyML, interface with electronic components like sensors, and deploy ML models on memory-constrained devices.
License
MIT license
Lattice tinyvision & tingyml
https://github.com/tinyvision-ai-inc
https://www.latticesemi.com/Products/DevelopmentBoardsAndKits/HimaxHM01B0
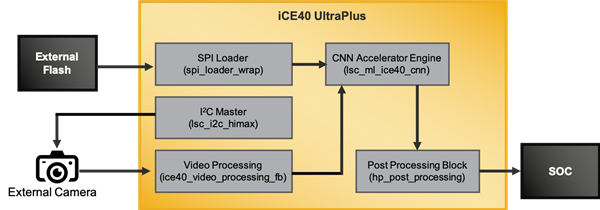
The above links are references for Lattice’s related AI routines implemented on the low-power FPGA Up5k. Interested parties can search for more information; the official website has relevant introductions. Although the GitHub repository doesn’t seem official, it also contains many complete reference designs.
Efinix TinyML
https://github.com/Efinix-Inc/tinyml/tree/661ae30f2bf5b083ab88c7a4e54f0185a859f9b8
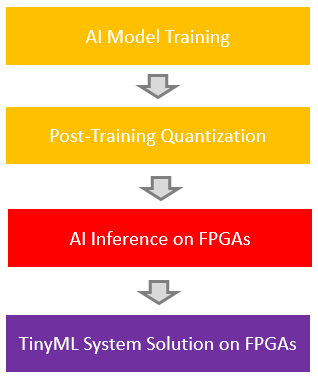
Efinix provides a TinyML platform based on the open-source TensorFlow Lite for Microcontrollers (TFLite Micro) C++ library, which runs on RISC-V and includes the Efinix TinyML accelerator. This site offers an end-to-end design process that aids in deploying TinyML applications on Efinix FPGAs. It covers the design process from training AI models, post-training quantization, to running inference on RISC-V using the Efinix TinyML accelerator. Additionally, it demonstrates the deployment of TinyML on Efinix’s highly flexible domain-specific framework.
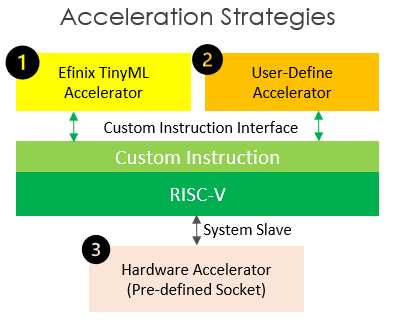
RISC-V SoC:
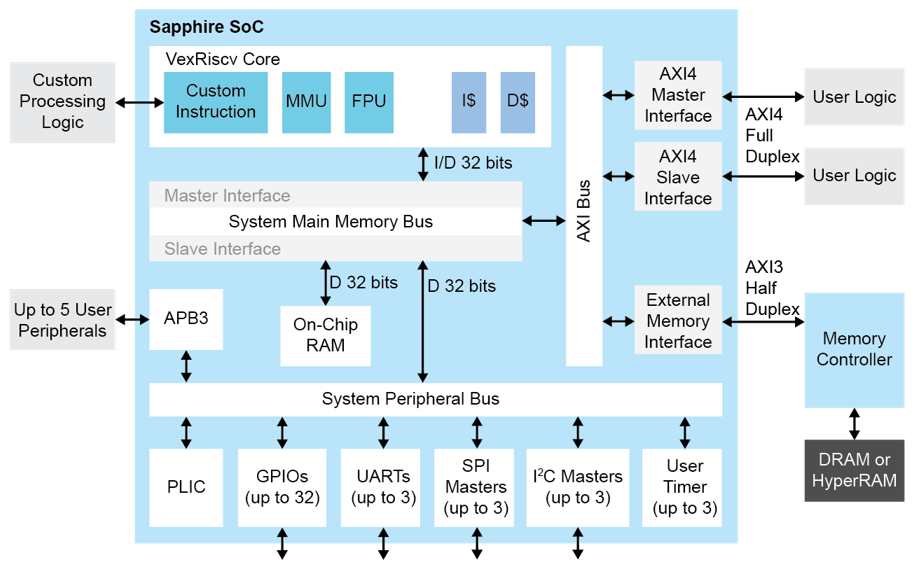
Design Process:
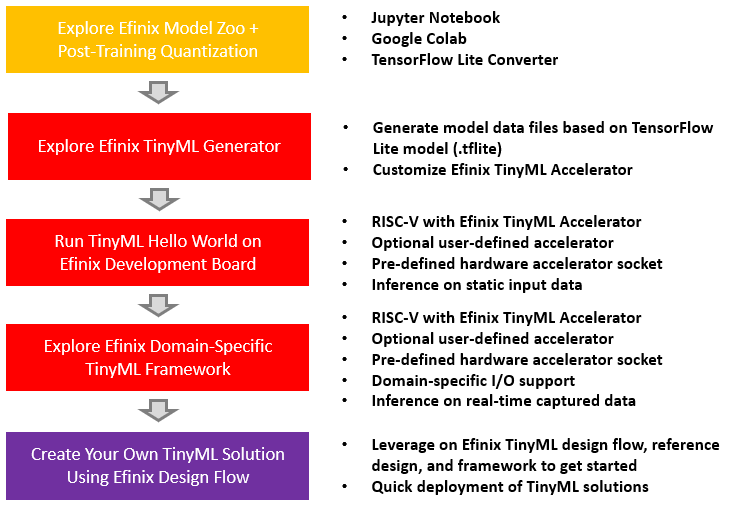
TinyAcc
https://github.com/kksweet8845/TinyAcc
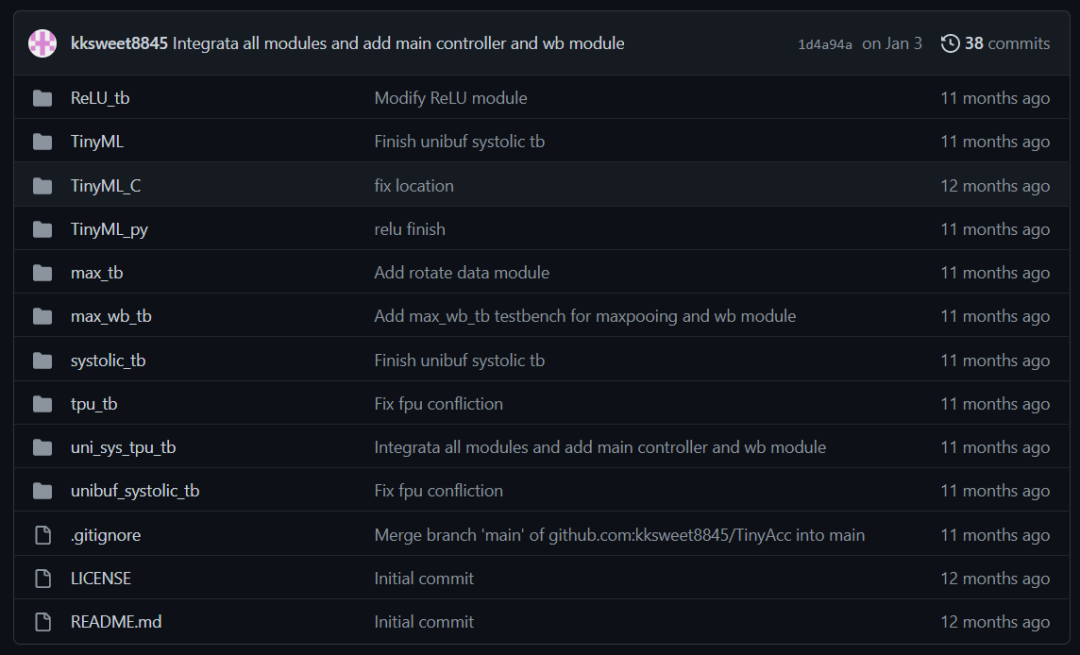
This is a project implementing a neural network model with dropout functionality.
Conclusion
The TinyML projects introduced today are just a few. Current application scenarios are still relatively inclined towards embedded microprocessors, with only Lattice and Efinix FPGAs launching their own IP and example programs in this area. Lattice’s development leans more towards open-source promotion, so whether this application area is a