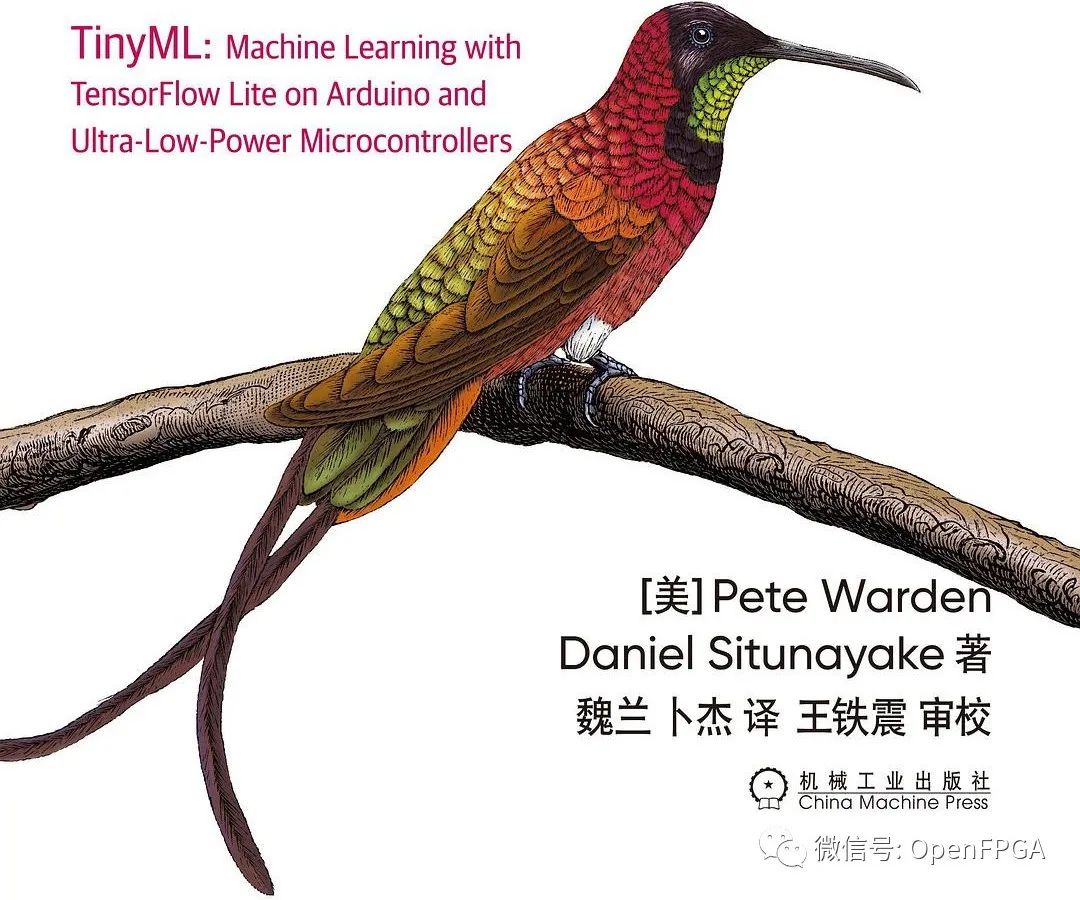
TinyML is a type of machine learning characterized by shrinking deep learning networks for use in micro hardware, primarily applied in smart devices.
Ultra-low power embedded devices are “invading” our world, further promoting the proliferation of AI-driven IoT devices with the help of new embedded machine learning frameworks.
FPGA has been applied across various fields due to its low power consumption and reconfigurability, significantly enhancing IoT application environments. So, is the application of TinyML on FPGA the best use case for FPGA? Let’s analyze the characteristics of TinyML before summarizing.
Next, let’s translate this jargon: What is TinyML? More importantly, what can (and cannot) it be used for?
What is TinyML?
Machine learning is a buzzword that has existed for a while, with many useful applications requiring an understanding of chaotic data. In current applications, it is rarely associated with hardware. Typically, if it must be associated with hardware, it is usually linked to the cloud, which often relates to latency, power consumption, and connection speed.
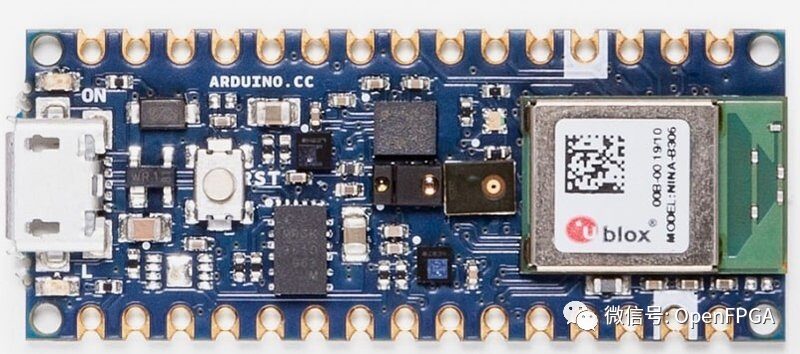
However, applying machine learning on devices is not a new concept. For several years, most of our smartphones have included some form of neural networks. Device music recognition and many camera modes (like night and portrait modes) are just a few examples relying on embedded deep learning. These algorithms can identify applications we are more likely to use again and shut down unnecessary ones to extend phone battery life. However, embedded AI faces many challenges, the most significant being power and space. This is where TinyML comes into play.
Sensor data on devices requires substantial computational power, leading to issues such as limited storage capacity, limited CPU performance, and degraded database performance. TinyML brings machine learning “on-site” by embedding AI into small pieces of hardware. With it, deep learning algorithms can train networks on devices and reduce their size without sending data to the cloud, thus minimizing analysis latency.
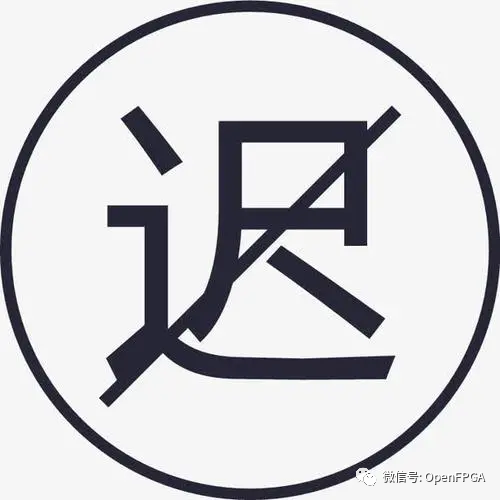
TinyML: Understanding the Basics
Google’s TinyML master and TensorFlow Lite engineering head Pete Warden published a book with Daniel Situnayake. This book, “TinyML: Machine Learning with TensorFlow Lite on Arduino and Ultra-Low-Power Microcontrollers,” has become a reference in the field.
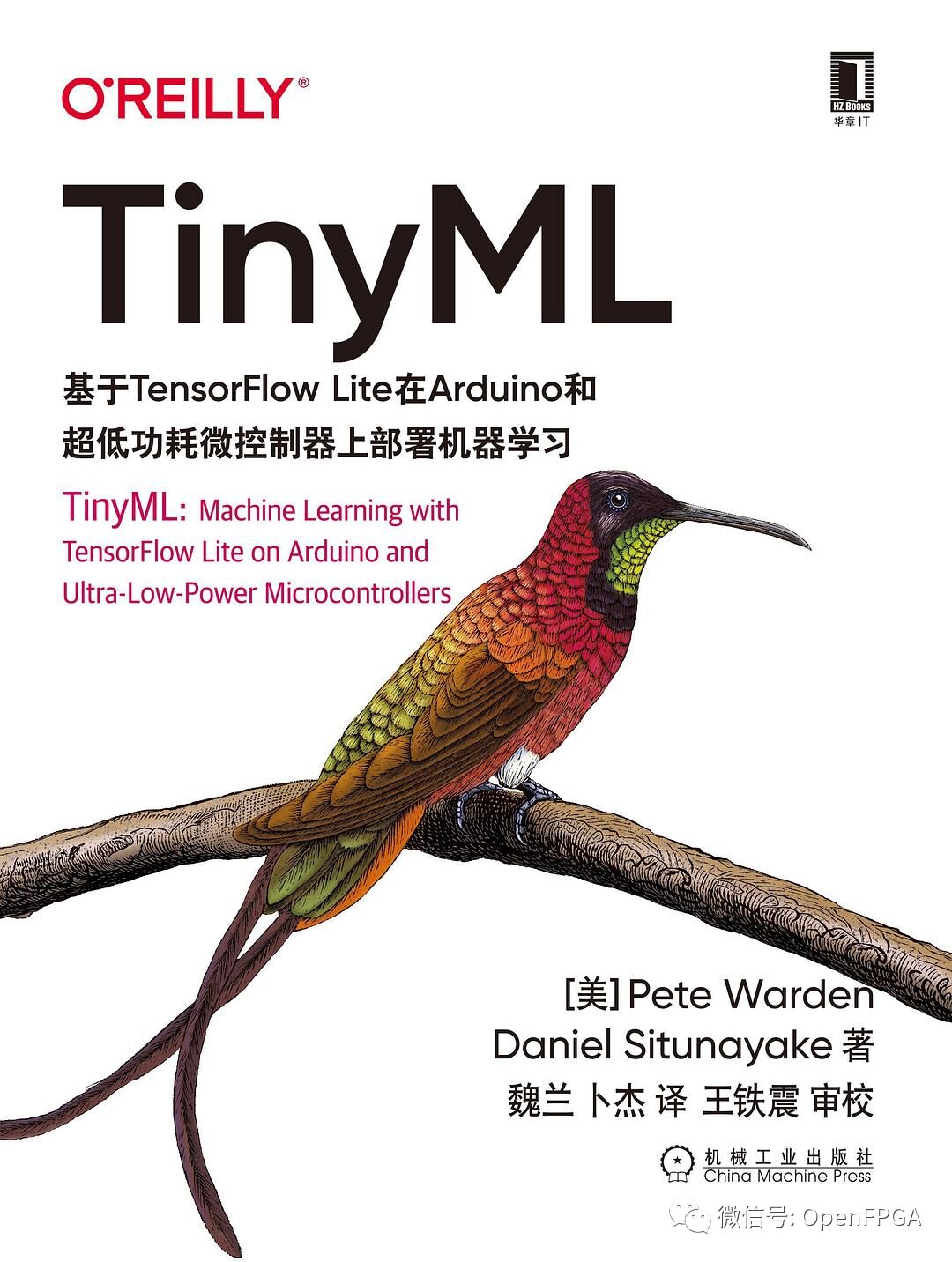
Finally, TensorFlow Lite is an embedded machine learning framework created by Google, with a subcategory specifically designed for microcontrollers. In 2019, in addition to TensorFlow Lite, other frameworks began focusing on making deep learning models smaller, faster, and suitable for embedded hardware, including uTensor and Arm’s CMSIS-NN. Meanwhile, many tutorials have emerged on how to use TinyML and similar frameworks on AI-driven microcontrollers to train, validate, and then deploy small neural network sets on hardware via inference engines.
Machine learning is often associated with optimization, but TinyML is not just about optimization: some cloud application programming interfaces (APIs) merely exclude interactivity and are overly constrained from a power consumption perspective. Most importantly, these limitations make edge computing slower, more expensive, and less predictable.
Unlike the previously mentioned machine learning applications based on mobile devices, TinyML enables battery or energy-harvesting devices to operate without needing to manually recharge or replace batteries due to power limitations. Think of it as an always-on digital signal processor. This translates to devices operating at power levels below 1 milliwatt (exaggerated description), allowing the device to either run on batteries for years or use energy harvesting. This also means these devices cannot connect via radio at all, as even low-power short-range radios would use tens to hundreds of milliwatts of power and only allow brief bursts of power. These constraints also lead to the need for code that can run with extremely minimal memory limits, constrained to tens of KB, thus requiring TinyML to be distinguished from code on Raspberry Pi or smartphones.
TinyML: Current Application Overview
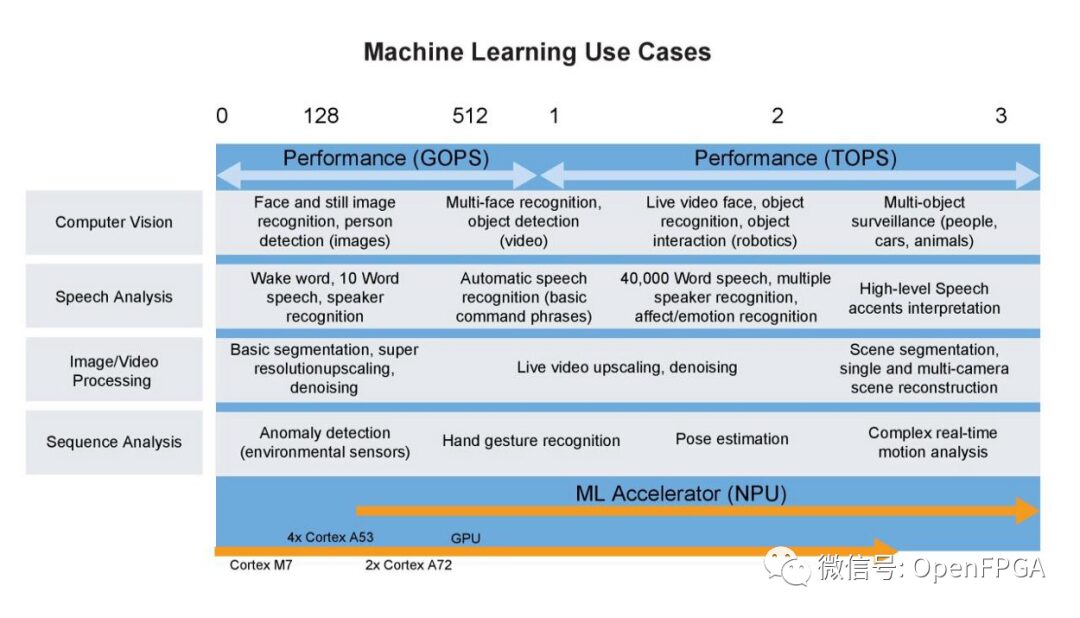
According to Emerging Spaces’ comments on Pitchbook, TinyML has attracted $26 million in investment since January 2020, including venture capital from accelerators, early investors, and later-stage investors. Compared to other more mature branches of AI and ML (like data labeling), this is relatively small. In the trend, the number of transactions competes with other hot topics like cognitive computing, next-generation security, and AIOps.
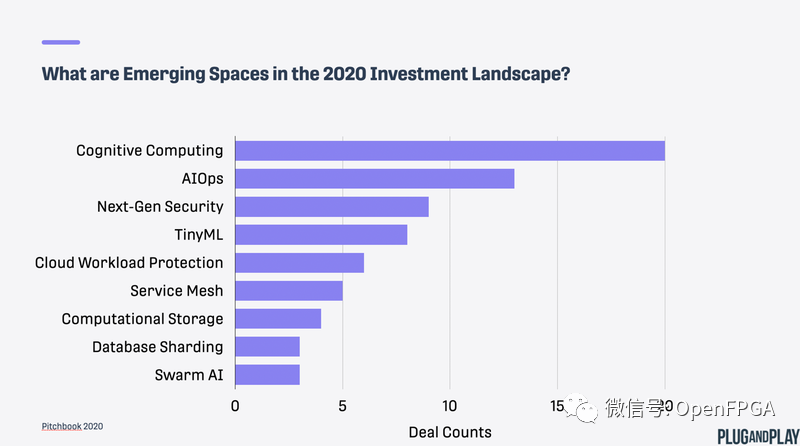
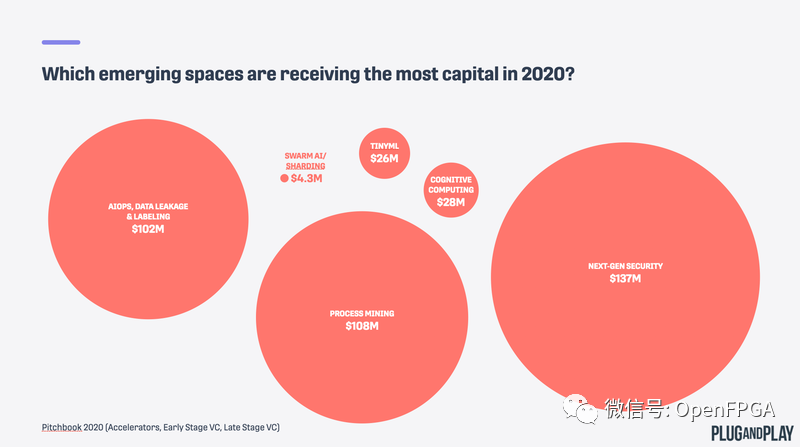
TinyML: How It Works
The working mechanism of TinyML algorithms is almost identical to traditional machine learning models, usually involving training the model on the user’s computer or in the cloud. The real power of TinyML comes into play during post-processing, often referred to as “deep compression.”
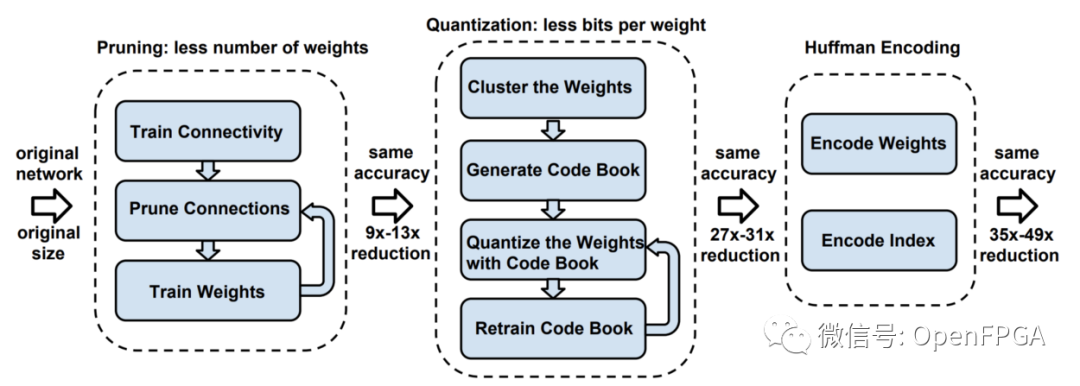
TinyML: Looking to the Future
This fall, Harvard University launched a course CS249R: Micro Machine Learning, mentioning that “the explosive growth of machine learning and the ease of use of platforms like TensorFlow (TF) make it an indispensable learning topic for modern computer science students.”
Today, there are over 250 billion embedded devices running worldwide, expected to grow by 20% annually. These devices are collecting vast amounts of data, and processing this data in the cloud poses significant challenges. Among these 250 billion devices, about 3 billion currently in production can support the TensorFlow Lite being produced today. TinyML can bridge the gap between edge hardware and device intelligence.
Conclusion
With the increase of IoT devices, the fusion of multiple sensors, and the substantial data processing requirements, low-power FPGA will stand out in this area.
In this regard, FPGA manufacturer Lattice has long launched a TinyML development platform based on ultra-low power (the up5k used in iPhones) FPGA and has open-sourced sound and facial recognition solutions. The links are as follows:
https://github.com/tinyvision-ai-inc
https://www.latticesemi.com/Products/DevelopmentBoardsAndKits/HimaxHM01B0
As the number of new smart devices and IoT devices increases, the application of TinyML on low-power FPGA is likely to be widely applied in ubiquitous, cheaper, scalable, and more predictable edge-embedded AI devices, thus changing the paradigm of ML applications.
– -THE END- –
Previous Highlights

[Free] FPGA Engineer Talent Recruitment Platform
FPGA talent recruitment, HR from enterprises, take note!
Selected System Design | Real-time Image Edge Detection System Design Based on FPGA (with code)
Design of Gigabit Ethernet RGMII Interface Based on Primitives
Theory of Timing Analysis and Use of TimeQuest – Chinese Electronic Edition
Job Interview | Latest Compilation of FPGA or IC Interview Questions
New Vivado Content Added to FPGA Image Processing Special Course, Online and Offline Registration Available
New Vivado Content Added to FPGA Timing Analysis and Constraints Special Course, Online and Offline Registration Available
Resource Compilation | FPGA Software Installation Packages, Books, Source Code, Technical Documents… (Updated on 2022.09.24)
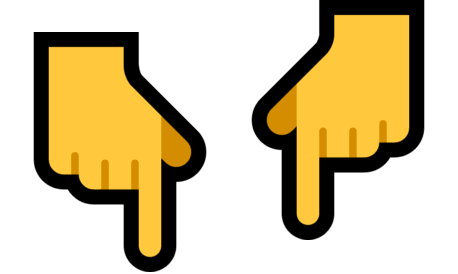
FPGA Technology Community Announcement
Ad-free, pure mode, providing a clean space for technical exchanges, from beginners to industry elites and big names, covering various directions from military to civilian enterprises, from communication, image processing to artificial intelligence.
FPGA Technology Community WeChat Group
Add the group owner’s WeChat, note your name + company/school + position/major to join the group
FPGA Technology Community QQ Group
Note name + company/school + position/major to join the group