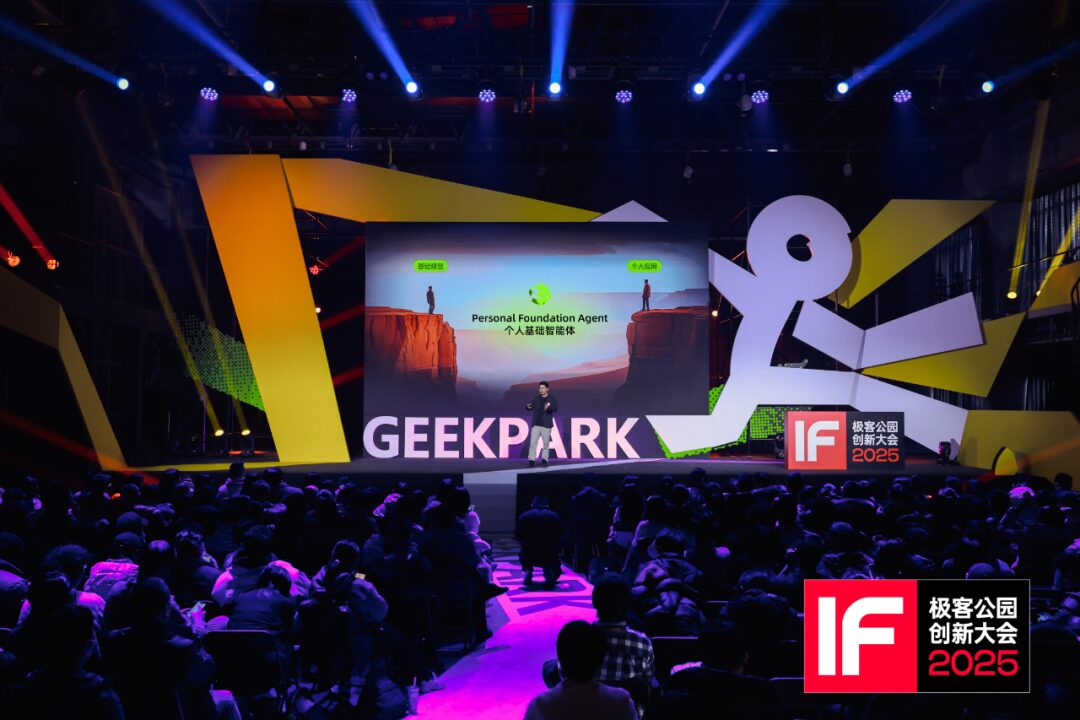
From traditional AI Agents to Personal Foundation Agents.
Compiled by | Lian Ran Edited by | Jing Yu
2024 is seen as the year of AI applications, and the upcoming 2025 is widely anticipated for Agents. Last week, Google officially released its latest large model series, Gemini 2.0, claiming it to be their most powerful artificial intelligence model to date, “designed for the Agent era.” How to leverage “agents” as the core driver to break the limitations of traditional human-computer interaction has become a hot topic in the industry.
At the Geek Park IF2025 Innovation Conference, Zeng Xiaodong, founder and CEO of AutoArk, explored the future development direction of AI Agents in his keynote speech titled “What else can AI do besides finding a ‘partner’?” He delved into how to advance AI from a single-task assistant to a personalized and emotional intelligent partner through Foundation Agents.
From the initial AlphaGo to today’s large language models and specialized agents in vertical fields, the functionality and application scope of AI Agents are rapidly expanding. However, as AI technology enters personal life, agents are no longer just tools for task completion but increasingly become core partners that understand user emotions and meet personalized needs.
In key areas such as interaction, memory, and skills, achieving low latency, visual understanding, and high emotional interaction for real-time feedback, constructing personalized memory systems, and ensuring robust execution capabilities in both virtual and physical environments have become significant challenges for the evolution of agents.
Zeng Xiaodong’s concept of a “Personal Foundation Agent” is proposed against this backdrop. He emphasized that AI Agents in personal domains are not merely assistants for solving isolated problems but long-term partners for users, personalized to meet productivity and emotional companionship needs, enabling AI to truly understand and integrate into users’ lives and work.
Moreover, Zeng Xiaodong pointed out that AI Agents in personal domains will not only exist on current smartphones and computers but will also be present in an increasing number of new hardware terminals. Therefore, AutoArk’s exploration encompasses not only technological breakthroughs but also the incubation of hardware products based on self-developed technological advantages. The intelligent robot “Arki,” set to be released next year, embodies this concept.
Under this new blueprint for AI agents, Zeng Xiaodong and the AutoArk team’s technological exploration is accelerating. Future AI products may become indispensable personalized partners in everyone’s life, further pushing human-computer interaction towards higher levels of intelligence and emotionality.
In Zeng Xiaodong’s view, AutoArk’s specialized agent solutions will also bring unprecedented opportunities to the enterprise-level market. AI Agents have emerged as a necessity, gradually learning and optimizing through interactions with industry experts, thus paving the way for the digital transformation of enterprise business processes.
The new year is approaching, and the next phase of the AI industry will soon begin. It is expected that by 2025, the application market for AI Agents will reach hundreds of billions of dollars, making 2025 a potential year of commercial explosion for AI Agents.
The following is the transcript of Zeng Xiaodong’s speech at the Geek Park IF2025 Innovation Conference, compiled by Geek Park.
How has AI Agent developed?
Zeng Xiaodong: Good afternoon, everyone! I am Zeng Xiaodong, the founder of AutoArk.
Over the past two years, my team and I have been adhering to a direction—AI Agents, and we are currently transitioning from traditional AI Agents to Foundation Agents.
Let’s first look at the development path of AI Agents from a practical perspective.
Development path of AI Agents | Image source: AutoArk
In fact, Agents first appeared in human view about 20 years ago with AlphaGo, which learned to play Go through reinforcement learning by interacting with the environment in numerous games. However, these agents could only handle single tasks, so after AlphaGo, agents received little attention for a long time until the emergence of large models.
Taking language foundation models as an example, they can handle many tasks, including medium to long-tail tasks. Many foundational Agent frameworks quickly emerged on LLMs, and we have also seen many Prompt Agents, which give agents specific roles through writing prompts, including configuring callable tools. According to incomplete statistics, there are currently over 700,000 Prompt Agent applications worldwide. Whenever anyone opens any large model app, there is bound to be a tab about agents. We collectively refer to these agents as Prompt Agents or Baby Agents because they essentially still possess certain general capabilities of large language models, just visualized through the way prompts are written.
I believe AI Agents will have two deep water zones in the future: Expert Agents and Personal Agents.
When Agents enter the first deep water zone, the vertical field, they require higher specialization. Directly applying general model Prompt Agents cannot meet the professional requirements of vertical fields; in previous professional cases, the success rate of general models in vertical field tasks often fell below 50%. Therefore, we need Expert Agents that deeply integrate models with vertical field data and professional business processes to form highly specialized AI Agents.
For highly complex tasks, we can even assemble a multi-agent team to tackle particularly difficult problems. One product example is a product we launched in the first half of the year to tackle challenges in pharmaceutical research and development. In this product, we have 18 specialized agents, each with a different underlying model. The 18 agents can communicate naturally with each other, write code, call medical tools and models, and automatically correct errors to handle highly complex issues.
For the level of Expert Agents, the key to generating a business model is the agent’s specialization in that vertical field. Whether using a single-agent or multi-agent solution, it must effectively achieve the ideal cost reduction and efficiency improvement.
AutoArk’s multi-expert agent product AgentStudio | Image source: AutoArk
When Agents enter the second deep water zone, the personal domain, they will not only help users improve productivity but will also provide more emotional value. Personal domain Agents will exist not only on smartphones or computers but will also be integrated into more terminals, such as glasses, smart speakers, future humanoid robots, and more new types of smart hardware. There is a significant gap in this area; whether in hardware AI products or software AI applications, there are still many core issues to be resolved between foundational models and applications, such as interaction experience, personalized memory, execution capabilities, etc.
Our team has been exploring what kind of agent is needed in the personal domain for a long time. We believe that what is needed in the personal domain is not a traditional agent but a foundational agent, which we term Personal Foundation Agent.
The gap between foundational models and AI applications needs to be addressed through Personal Foundation Agents | Image source: Geek Park
Three Elements of Foundation Agents: Interaction, Memory, Skills
The Personal Foundation Agent is backed by three foundational capabilities, which we aim to elevate to a high level, making the implementation of personalized applications more efficient.
Three elements of Personal Foundation Agents | Image source: AutoArk
The first dimension is interaction, which includes not only text interaction but also real-time interaction involving voice and visual comprehension.
The second dimension is memory, which involves how to build a personalized memory system beyond the foundational model.
The third dimension is skills, which refers to the execution capabilities of AI Agents.
If we plot these three elements in the same coordinate system, we see that all the agents we’ve discussed, whether AlphaGo, Prompt Agents, or expert agents, are located in the lower-left corner of the coordinate system, while our goal is to create a Personal Foundation Agent located in the upper-right corner, which is extremely challenging. Over the past two years, we have achieved some milestones in each dimension, which I will introduce one by one.
Let’s first look at the interaction dimension. When developing a personal AI application, whether it’s software or hardware, many scenarios require not just the pure text interaction capability of LLMs but human-like, real-time voice and visual understanding interaction capabilities, as shown in the middle part of the diagram.
Traditional methods generally implement audio-video interaction using a “three-stage” serial link, which involves first connecting a speech recognition ASR, then a large model LLM, and finally a speech synthesis service TTS, but this approach has three fatal flaws: 1) high latency; 2) stiff interaction; 3) lack of emotion.
Traditional voice agent interaction link | Image source: AutoArk
We can cite several common cases, such as various voice-interactive toys on the market, which have a feedback delay of about 6 seconds, a problem typically encountered using traditional “three-stage” links. Their interaction is not open-ended and cannot be interrupted by voice at any time; many products require pressing a physical button to converse, leading to poor product experiences and high return rates.