▲Click above Lei Feng Network Follow
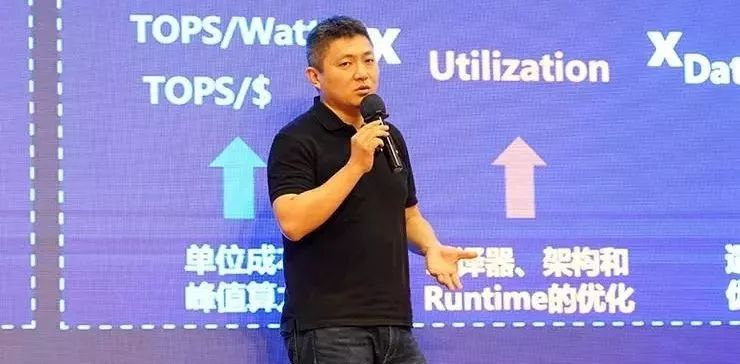
Zhang Yongqian stated that the contradiction between the data generated at the edge and the limited processing capacity of the backbone network is becoming increasingly prominent. To solve this contradiction, a combination of cloud, edge, and terminal is essential.
Written by | Bao Yonggang
The integration of AIoT is on the rise, and the intelligent transformation of industrial manufacturing is imminent.
In order to construct a new understanding of the AIoT industry, analyze the development of the AIoT ecosystem of “cloud, network, edge, terminal” and the intelligent manufacturing industry, and explore the current challenges in the AIoT industry and the development ideas for the industrial internet, the Global AIoT Industry and Intelligent Manufacturing Summit was grandly held in Shenzhen on November 22, 2019. This conference was hosted by Lei Feng Network and supported by the Shenzhen Software Industry Association, Shenzhen Big Data Industry Association, Shenzhen Artificial Intelligence Society, and Shenzhen Artificial Intelligence Industry Association.
As the only annual AIoT event carefully crafted by Lei Feng Network, the summit focuses on the development of key technologies including AI, IoT, 5G, edge computing, and their application scenarios; it focuses on the landing of key industries centered on smart home, smart manufacturing, and smart cities, committed to discussing key issues in the development of AIoT technology and industry. It provides a cutting-edge platform for the collision of ideas in production, learning, and research, and offers a professional platform for communication and interaction among professionals from government and enterprises.
At the morning forum on “AIoT Technological Revolution,” Zhang Yongqian, Vice President of Horizon Robotics and General Manager of the Smart IoT Chip Product Line, delivered a presentation titled “Empowering Industries with Edge AI Chips, Co-creating an Inclusive AI Era.” He stated that the rapid growth in the number of AIoT devices hides huge demands and business opportunities. Among them, the contradiction between data generated at the edge and the limited processing capacity of the backbone network is becoming increasingly prominent. To solve this contradiction, a combination of cloud, edge, and terminal is essential.
On the edge side, having computing power is not enough; it also depends on the effective utilization rate of that computing power. Moreover, AI chips should not just be hardware; they need to integrate both hardware and software. Specifically, regarding AIoT scenarios, Zhang Yongqian believes that the landing of AI faces five challenges: multi-form products, scenario effectiveness, rapid application development, hardware development, and system integration, and he specifically introduced how Horizon Robotics gradually dismantles and addresses these challenges.
The following is a transcript of the speech edited by Lei Feng Network without altering the original intent:
AI is indeed increasingly integrated with our production and life, and it is beginning to create real value. Today, I want to share how Horizon Robotics develops AI chip solutions on the edge and how to integrate the edge with the cloud to enable AI deployment.
First, let’s talk about computation. Computation is increasingly decentralized from the center to the edge. Initially, people used mainframes, then PCs, one per household, then smartphones, one per person, and now in the AIoT era, the trend of smart terminal devices is becoming more apparent. We believe that in the near future, the number of smart devices may exceed the global population by an order of magnitude, or even two. Behind this process lies enormous demand and business opportunities, which is also a significant area of interest for us.
5G is actually preparing for AIoT; it can greatly increase the number of devices connected on the terminal and edge sides, as well as the quality of connections. However, 5G will bring a more significant contradiction: while massive amounts of data are generated on the edge every day, the expansion and cost of building the backbone network and cloud are substantial challenges.
In other words, in the 5G era, the contradiction between the data generated on the edge and the relatively limited processing capacity of the backbone network will become more pronounced. To solve this contradiction, the future deployment of AI must combine cloud, edge, and terminal, achieving a dynamic balance. At the front end, we must perform intelligent processing; a large architecture involves extensive perception at the front end while the cloud handles more complex cognition, forming a dynamic balance that optimizes the cost-effectiveness of the entire network.
Edge computing has become quite popular in recent years because it has other advantages, such as privacy protection. Compared to transmitting data to the cloud, keeping data at the edge can provide better protection. Of course, the term edge computing has also gained popularity, now standing alongside cloud computing as a key element in the entire architecture.
When discussing edge computing, we cannot overlook edge AI chips. In recent years, many companies in China have been discussing AI chips, and Horizon has been doing this since its inception. From our perspective, edge AI chips differ significantly from traditional chips. Traditional chips primarily focus on PPA, which stands for Power, Performance, and Area (cost). These factors have dominated the rapid development of Moore’s Law over the past two to three decades.
When it comes to AI edge computing, this evaluation becomes much more complex. First, when discussing AI chips, people often mention having several T of edge computing power, but having computing power alone is not enough; the effective utilization rate of that computing power must also be considered. For example, if you have a T of computing power but only achieve a 30% effective utilization rate in practical scenarios, it means that 70% of the costs, including the power consumption required to maintain the chip’s normal operation, are wasted.
Additionally, we must look at the processing scenarios of AI chips and whether the effectiveness indicators of the output results for those scenarios are optimal, as this is one of the most important metrics for evaluating AI chips and algorithms. We combine these points to genuinely assess the situation.
Based on the above analysis, when Horizon was established in 2015, we did not simply become a chip company; we conducted a lot of software work. When designing chips, in addition to the chip architecture, we also designed corresponding instruction levels, compilers, and model structures. These efforts are our predictions for the mainstream edge and AI scenarios in the coming years. When designing the chip architecture and models, we closely integrate these predictions to meet the stringent requirements for cost-effectiveness and energy consumption at the edge.
In our view, AI chips should not just be hardware; they need to integrate both hardware and software. The best practitioner of this is Apple; even after using an Apple phone for a long time, it still feels very smooth because it integrates hardware and software very well. Even though Apple’s clock speed and pure physical computing power may be lower than Qualcomm’s, in fact, after two or three years of running its operating system, the smoothness and performance of an Apple phone exceed those of phones equipped with chips from other manufacturers with the same clock speed. This is an excellent example of the power of hardware-software integration.
This is an assessment conducted by our company; on our self-developed chip, we input a 720p image and run an algorithm for face recognition. If we do not consider software, compilers, and architecture, the single frame consumption is 141.9 MB per frame, with a single frame computation delay of 43.77 milliseconds and a resource utilization rate of 57%. After we open the compiler for optimization, the single frame bandwidth consumption is greatly reduced, and the resource utilization rate can reach 95%, significantly decreasing the exchanges and time with external storage data. This example illustrates the power of hardware-software integration, which has been our ongoing focus since the company was founded. The value we bring to users through our underlying empowerment is the combination of hardware and software.
We are confident in continuing Moore’s Law; the traditional Moore’s Law is process-oriented, and TSMC is preparing a 3nm factory. However, through the combination of hardware and software, we can see that there is still a lot of room for development.
Next, let’s talk about Horizon. Horizon was established in July 2015 and is the first company in China to truly focus on AI chip development with a hardware-software integration approach, leading the way with the hardware-software integrated BPU architecture.
In December 2017, Horizon released China’s first edge AI chip—the Sunrise Generation and the Journey Generation. The Sunrise Generation is aimed at AIoT, while the Journey Generation is aimed at intelligent driving. We are honored to be the first company globally to tape out AI chips with TSMC.
In 2018, our Journey chip was deployed in the top Robotaxi fleet in the United States. By the end of 2018, in the first year of the Sunrise Generation’s deployment, our chip and solution shipment volume reached six figures.
The theme of today’s conference is “Intelligent Manufacturing,” so here I will introduce our AI chips aimed at AIoT. Horizon just released the second generation of Sunrise at the recently concluded AIBO conference. This is an edge chip processor for AIoT, with equivalent computing power of 4T.
In addition to the previously mentioned hardware-software integrated chip concept, I will now discuss how our edge AI chips empower the entire industry.
On the basis of our hardware-software integration, we will provide a foundational toolchain for very capable customers in the industry. These customers have excellent algorithm teams and rich industry scenarios and data, and they can use our chips and toolchains to train their own models.
This training differs from ordinary computing chips; we have made significant predictions about the algorithm evolution trends for future mainstream scenarios. In our chips, we have further accelerated many algorithms so that when customers use our processors and toolchains combined with their data for training, it is like standing on our shoulders. If it were an ordinary AI processor, it might require several experienced algorithm engineers to spend a long time training, but on our platform, a few relatively entry-level or less experienced engineers can quickly train models. This is beneficial for many new applications and distributed edge-side applications, greatly reducing enterprise investment and time to market.
Over the past four years since 2015, there has been a problem in the AIoT deployment landscape on the edge side: very few companies have strong AI capabilities, including algorithm and data capabilities, accounting for less than 1% of the total.
Many enterprises have rich industry experience and may have some industry data, but knowing how to effectively implement AI on the edge is a significant challenge for them. This is a system-level issue, and as you can see, it faces many challenges:
The first is the various forms of AI products, such as cameras, smart panels, various types of robots, smart appliances, etc. With so many forms of AI products, how to productize them is a very challenging process.
The second is the intelligent effects in scenarios. You can purchase an AI chip and find the desired algorithm from an algorithm company, but then you have to integrate all these elements yourself, considering the quality of input images and truly creating a product that can perform intelligent perception and analysis at the edge to meet scenario-specific needs. The resource investment required to create a demo-level product versus a fully functional product is significantly different. Achieving excellent AI and product performance at the edge requires not only computing power but also effective outcomes, which is difficult.
The third challenge is how to quickly develop upper-layer intelligent applications. Given the complexity of scenarios where edge deployment occurs, even with chips, algorithms, and good image input, it is still insufficient. The strategies for the entire front-end scenario are complex. You may have a basic algorithm that outputs structured information, but this structured information is often far from the useful information you desire. How to quickly develop effective software that integrates with applications is a significant gap. Moreover, you will find that strategies may vary across different scenarios, making it essential to develop upper-layer intelligent applications rapidly.
The fourth challenge is hardware-related. We have found that AI is integrated with scenarios, not like traditional industry chain divisions where product makers make products, hardware makers make hardware, and then sell to those who implement in the industry. In fact, many customers in various industries and applications are closest to the scenarios and know how to use AI. They want to own an intelligent product rather than procure it from a third party; they hope to directly obtain a high-quality intelligent product. However, hardware development is a headache for them, as many companies have never engaged in hardware development before.
The fifth challenge is system-level integration. Even a company that makes AI chips, such as Horizon, which excels in hardware-software integration and provides toolchains, finds that 99% of users cannot utilize it effectively. It is too complex; the division of labor in the industry has not formed, and the industry chain has not matured enough, requiring us to take further steps to empower and overcome these challenges.
Next, I will detail how we empower, which is to provide chip-level solutions tailored to edge scenarios, further empowering our customers.
AIoT is a vast concept. When we provide solutions, we dismantle the challenges step by step. First, regarding various forms of AI products, we mainly focus on three categories: the first category is cameras, which are widely applicable in various scenarios. The second is smart products with screens, used for interactive panel products, mainly for close-range interactions. The third is edge-side computing box solutions, which flexibly connect various front-end perception data. These are currently based on Horizon’s first-generation Sunrise chip and the second-generation Sunrise chip’s edge AI solutions.
First, let’s discuss the most concerning aspect of intelligence. We have a full-stack intelligent algorithm capability, mainly targeting human intelligence, as the human intelligence market appears to be the first to land. Level one structuring can perform atomic intelligent detection; level two structuring can conduct recognition, including attribute analysis and voice; and finally, level three structuring can perform further semantic-level analysis of the time and space movement of the analyzed target, such as Re-ID. Based on the Sunrise chip, Horizon possesses full-stack capabilities to empower our customers. This includes 3D modeling of dense crowds, real-time tracking, and analysis of five key points, laying a solid edge-side foundation for tracking, Re-ID, and behavioral analysis in time and space.
All spatiotemporal analyses of dense crowds are completed on an edge AI chip with around 2 watts of power, significantly improving cost-effectiveness compared to traditional servers, while also greatly reducing energy consumption.
With good algorithms, good image input is also a technical task; it is not enough to select a particular ISP from a chip. For different scenarios, extensive micro-tuning is necessary to overcome disturbances caused by image input in the scene. For example, if an intelligent perception device is deployed outdoors, the angle of the sun changes from morning to noon to evening, and from winter to summer, with strong light, dim light, backlight, and counter-light, all constantly changing. How to ensure that this device can automatically obtain the best image effect throughout the day requires extensive image tuning.
Next, let’s discuss engineering, how to quickly develop different intelligent products on the edge and terminal sides. We have made unified planning in our software architecture to quickly launch these solutions and allow customers to rapidly develop upper-layer applications based on the foundational work we provide. We package all these intelligent capabilities, including hardware, OTA upgrades, and cloud SDK integrations, into our solutions, allowing users to quickly develop their applications.
In addition to completing the full algorithm design related to our chips to ensure image recognition effectiveness, we have also designed the modules for intelligent cameras, so customers can directly use the already debugged core parts of the hardware, plus their expandable peripherals, to quickly complete hardware design.
When deploying to scenarios, even after completing all the previous steps, the effect may still be significantly impacted by some minor factors, such as how to supplement light in low-light conditions or when visible light is insufficient, requiring the addition of other light sources, such as infrared light.
The position and power of the supplemental light will directly affect image input and ultimately the effect. We will ensure that all these reference designs are well-prepared, allowing customers to quickly complete the productization process.
In addition to these, we will also assist our customers in conducting industry inspections in some critical sectors. After launching the first generation of AI chips, we gradually explored how to empower our vast customer base, clarifying the tasks and thought processes needed. Currently, we have launched three series of solutions: the first is a solution for smart panel machines, the second is for smart IPCs, and the third includes access control, attendance, and visual intercom, mainly targeting facial recognition. Face recognition in cameras is currently the most widely used, including customer flow analysis in commercial scenarios and structured analysis of personnel in relatively complex scenarios, as well as behavioral analysis. These can also be integrated into our edge-side analysis units.
Finally, I would like to summarize: Horizon is a company focused on edge AI chips and solutions. We empower at the foundational level through hardware-software integration and a complete set of solutions. Currently, very few companies in the industry can make such significant investments and possess such comprehensive capabilities, as this path is indeed quite challenging, requiring chip development, algorithm work, and solution provision.
Our service philosophy is to never engage in industry applications; we will not compete with our customers nor rush to the downstream market when we see a certain industry booming, making products and projects while undermining our partners’ businesses. We adhere to our principles, focusing on foundational technological empowerment.
Our ultimate goal is to make AI more inclusive, not just something that only a few powerful organizations can utilize. Through our hardware-software integration and solutions, we aim to bring AI to industry clients, allowing them to better develop applications in their industries and create excellent intelligent perception inputs and filtration at the edge. AI at the edge will then combine with AI in the cloud, ultimately enabling the technology to truly land in the industry.
Follow the “Lei Feng Network” public account and send the keyword “2019 AIoT Summit – Horizon” to obtain the PPT of this exciting speech.
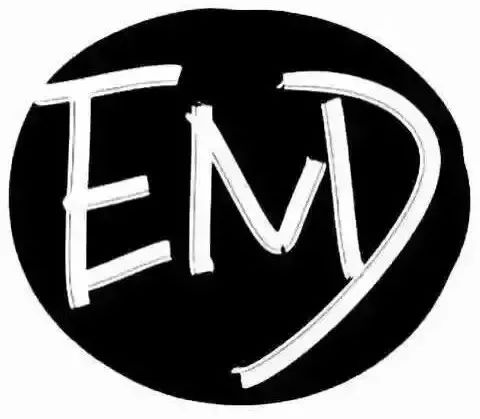
Previous Recommendations
▎QQ’s anxiety has been written into WeChat Mini Programs.
▎ The world’s fastest 5G! MediaTek releases Dimensity 1000, AI benchmark refreshes records.
▎How was Wang Jian, an academician of the Chinese Academy of Engineering, cultivated?
▎ Xiaomi responds to the decline in mobile phone sales; Lei Jun criticizes competitors for overpriced 5G phones; Apple will switch to Qualcomm baseband next year; Kuaishou responds to abandoning Pinduoduo to team up with Alibaba.
Lei Feng Network Annual Selection
Looking for the Best AI Implementation Practices in 19 Major Industries
Founded in 2017, the “Annual List of Best AI Gold Mining Cases” is the first evaluation activity for artificial intelligence business cases in the industry. Lei Feng Network seeks the best implementation practices of artificial intelligence across various industries from a commercial perspective.
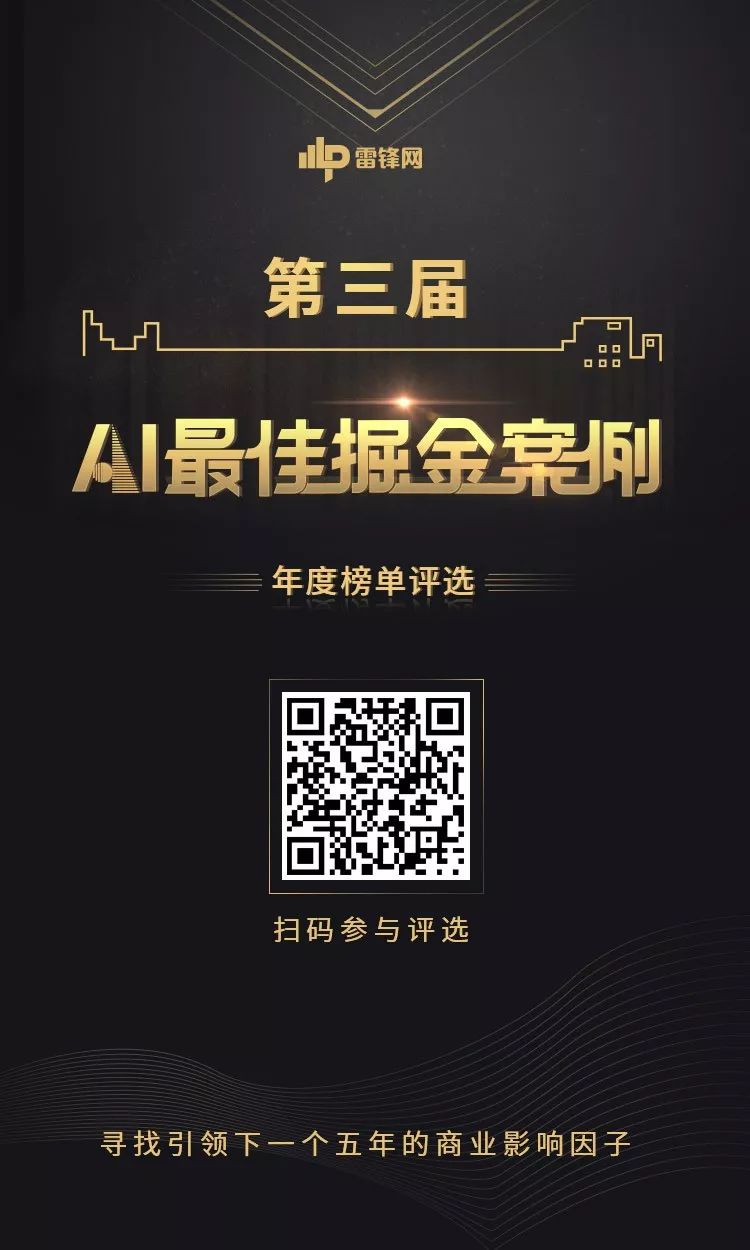
