Ms. Chloe Ma, Vice President of Business Development at Arm’s IoT Division
Recently, Ms. Chloe Ma, Vice President of Business Development at Arm’s IoT Division, conducted an online interview with industry media, introducing the evolution of edge artificial intelligence (AI) and Arm’s new generation Ethos-U85 AI accelerator and the new IoT reference design platform Arm Corstone-320 in response to market demand.
The Evolution of Edge AI
“We are in an era where everyone carries one or more ‘supercomputers’ in their pockets, and in this evolution, Arm has deployed computing resources at the edge and endpoints, which has obvious advantages in enhancing response speed and reliability, saving bandwidth resources, protecting privacy and data security, and reducing costs,” Ma opened.
She first introduced the evolution of edge intelligence: “The history of edge intelligence can be traced back to early embedded systems, such as home air conditioners and electric meters, which are equipped with embedded processors to achieve simple functions like control and device management. At the beginning of this century, with the advancement of network and internet technology, the Internet of Things (IoT) emerged and connected everything through protocols like Wi-Fi, Bluetooth, ZigBee, Narrowband IoT (NB-IoT), and LoRa. The rise of IoT has greatly promoted the popularity of edge computing devices, which possess stronger processing capabilities and connectivity, bringing data processing closer to the data source. With the development of machine learning (ML) and artificial intelligence (AI) technologies, smart devices can not only perform tasks but also learn and adapt. Especially recently, with the development of Transformer and large models, AI models have made qualitative breakthroughs in universality, multi-modal support, and model fine-tuning efficiency, along with low-power AI accelerators and dedicated chips being integrated into endpoint devices, edge intelligence is becoming increasingly autonomous and powerful.”
Edge AI has enormous potential and is expected to assist multiple fields in continuously evolving and transforming towards intelligence. In this development process, “Arm’s customers and ecosystem partners are constantly innovating in smart home, smart retail, and smart manufacturing fields, thereby achieving a closed loop of perception, decision-making, and action, enhancing automation levels,” Ma said.
Specifically, in smart home applications, people mostly use different applications on their smartphones to manually control various appliance switches and settings. In the foreseeable future, AI models will act as a home “brain,” integrating various sensors, cameras, external weather, family preferences, natural language commands, and more inputs to create a safer and more energy-efficient personalized home environment. AI and large models will also make the retail industry smarter, more personalized, and automated. Through personalized shopping experiences, intelligent inventory management, dynamic pricing strategies, seamless online and offline integration, and automated operations, higher efficiency and superior customer experiences will be achieved. AI and large models are also expected to drive the transition from Industry 4.0 to Industry 5.0, achieving intelligent production lines, precise quality control, customized production, supply chain optimization, autonomous maintenance and remote monitoring, human-machine collaboration, energy conservation, emission reduction, and the development of new materials and processes, bringing profound changes to the manufacturing industry.
With the vigorous development of AI technology, Arm has made significant investments over the past decade. Ma introduced: “Initially, we focused on optimizing embedded processors necessary for various sensors to achieve ultra-low cost, low power consumption, and small footprint, while supporting the development of control-level code. With the development of the Internet of Everything, connectivity has made edge computing power and security increasingly important, leading to the emergence of technologies like Armv8-M and Arm TrustZone, enhancing computing power and security. As the demand for edge and endpoint AI inference grows, Arm introduced Armv8.1-M in the embedded field. Arm Helium technology enables CPUs to execute more compute-intensive AI inference algorithms. Subsequently, the Arm Ethos series AI accelerators were launched to meet higher performance and more complex AI workloads.”
Meanwhile, as systems become more powerful, their complexity also increases. Software and hardware must work together to unleash the maximum potential of AI processing. “Arm focuses not only on processor IP but also strengthens investment in software and toolchains to ensure industry leadership, meeting the development needs for simpler, faster high-performance edge AI systems, supporting the optimization of various AI operators and applications on the Arm computing platform, allowing edge AI to thrive on the Arm platform. Of course, we must work together with ecosystem partners to achieve this goal,” Ma introduced Arm’s market development strategy.
Arm’s Third-Generation NPU Products for Edge AI
— Ethos-U85
“Previously, in response to market demand, Arm launched the industry’s first AI micro-accelerator Ethos-U NPU, with companies like Infineon, NXP Semiconductors, Himax, Alif Semiconductor, and Synaptics already launching mass production chips equipped with Ethos-U,” Ma introduced. Now, Arm has launched the new Arm Ethos-U85, “which brings a fourfold performance improvement and a 20% increase in energy efficiency for high-performance edge AI applications, while maintaining a consistent toolchain with previous generations of Ethos U series products, enabling a seamless developer experience. It supports configurations from 128 to 2048 MAC units, providing 4TOPs of AI computing power at maximum performance configuration, sustainably supporting future application scenarios,” Ma said. “We look forward to seeing Ethos-U85 continuously deployed in emerging edge AI application scenarios such as smart homes, retail, and industry, meeting the demand for higher performance computing and supporting the latest AI frameworks.”
Image: Arm Ethos-U85 provides excellent neural network acceleration capabilities
In AI, Transformer-based models have excellent generalization capabilities, which will drive the development of new AI applications faster. In visual and generative AI use cases, such as video understanding, image-text integration, image enhancement and generation, image classification, and object detection, Transformers are highly valuable. The attention mechanism of Transformer architecture networks easily utilizes parallel computing to improve hardware utilization, enabling these models to be deployed on edge devices with limited computing resources. Developers can explore new opportunities in edge AI by choosing designs optimized for Transformer architecture networks, allowing these applications to enjoy faster inference, optimized model performance, and scalability.
To this end, the Ethos-U85 has increased support for Transformer architecture networks, which is a key feature for optimizing the fine-tuning time of large models at the edge and enhancing model generalization. Ethos-U85 not only provides the operations required for weight matrix multiplication in convolutional neural networks (CNNs) but also supports matrix multiplication, which is a fundamental component of Transformer architecture networks. Developers can explore new opportunities in edge AI by choosing designs optimized for Transformer architecture networks, allowing these applications to enjoy faster inference, optimized model performance, and scalability.
Furthermore, Ethos-U85 supports AI acceleration in low-power MCU systems and high-performance edge computing. “In high-performance edge computing systems, we increasingly see application processors and standard operating systems like Linux deployed along with advanced development languages. This model is more conducive to cloud-native development and cloud-edge load scheduling. This trend is particularly pronounced in AI application systems that process ever-increasing amounts of data. Ethos-U85 can well support these needs, combined with Arm’s leading Armv9 Cortex-A CPU, accelerating AI tasks running on application processors in intelligent IoT platforms. This will enable Ethos-U85 to bring efficient edge inference in applications like industrial machine vision, edge gateways, wearable devices, and consumer robots,” Ma said.
New Intelligent IoT Reference Design Platform
— Arm Corstone-320
To help partners simplify system development and accelerate time to market, Arm has also launched the latest edge AI reference design platform Arm Corstone-320 equipped with Ethos-U85, which is a brand new intelligent IoT reference design platform.
It is reported that the new reference design platform includes the highest performance embedded processor in the Cortex-M series, the Cortex-M85, and the new Ethos-U85 NPU AI accelerator. Additionally, as vision plays a key role in multi-modal perception, many edge MCUs and sensor systems increasingly rely on visual and image perception, Corstone-320 also includes the Arm Mali-C55 ISP to achieve low-power systems optimized for visual applications.
This reference design is developed for real workloads, with reference use cases including battery-powered camera systems deployed in smart homes and low-frame-rate network cameras in industrial and retail systems. Meanwhile, the Corstone-320 reference design provides a secure hardware-software combination, enabling partners developing based on this reference design to smoothly pass PSA Certified Level 2 certification, achieving compliance with regional and global security standards. “Through the pre-integrated and pre-validated reference design template of Corstone-320, Arm can help partners reduce the cost and time of developing edge intelligence chips,” Ma said.
Like previous Arm IoT reference designs, Corstone-320 not only provides a combination of chip computing subsystem IP but also includes software, AI model libraries, and development tools for software reuse. Leveraging Arm’s strong ecosystem, it also includes virtual hardware for simulating the complete Corstone-320 system, as well as fixed virtual platform (FVP) models for individual CPUs and NPUs, to simplify development and accelerate product design, supporting parallel collaborative development of software and hardware. This comprehensive approach to providing edge AI and intelligent IoT computing subsystem complete hardware and toolchain allows Arm partners to quickly develop differentiated value across a range of performance points.
Image: Arm accelerates the deployment process of edge AI devices
Ecological System
“Simplicity and ease of use are more suitable for widespread promotion, which is why, with the continuous expansion of edge AI, chip and system suppliers, algorithm software developers, and integrators in the upstream and downstream of the IoT ecosystem are increasingly converging on the Arm computing platform,” Ma introduced. After years of expansion and deepening of the ecological chain, the Arm computing platform can provide the features and functions required for AI from cloud to edge, modern agile development and deployment processes, achieving a consistent architecture based on mass production verification, and adopting a unified toolchain for AI transformation. The AI research and development community, including developers, data scientists, and academia, is building an increasingly growing software and tool ecosystem around the Arm computing platform, as well as open-source software libraries and AI frameworks.
“For example, the well-known PyTorch open-source ML framework is widely used to build and train neural network models. We are pleased to see the PyTorch Foundation invest in edge AI, releasing the ExecuTorch inference toolkit for mobile and edge devices, providing a lightweight runtime and operator registry covering various models in the PyTorch ecosystem. Additionally, due to Arm’s unique IP licensing model and open ecosystem, OEMs and ODMs can have multiple chip and module choices based on Arm architecture and computing platforms, flexibly developing system solutions for final applications,” Ma said.
Opportunities and Challenges
“Edge AI brings both opportunities and challenges. When designing edge AI chips and systems, it is necessary to find the right balance between computing power and energy efficiency. High-performance processing capabilities often come with higher power consumption, while edge devices often have strict limitations on power consumption and costs. At the same time, as more and more data is processed at the edge, data security and privacy protection become particularly important. This requires chip designs to include encryption and security features. Edge use cases are diverse, and the traditional IoT market is fragmented, making it essential to have software-defined and software-portable standards to better unify diverse application requirements and achieve economies of scale,” Ma stated.
Edge AI will also rise with the emergence of large models and generative AI. The continuous improvement of user experience, the explosion of data volume, and the recognition of data value by enterprises will bring about significant changes in the industry, such as achieving real-time language translation on devices. “As large models continue to be optimized through quantization, pruning, and clustering techniques, making large models suitable for deployment on edge and super terminal devices, we see the combination of large and small models at the cloud-edge-end becoming an important trend in the future development of AI products, and also an important direction for AI applications empowering industry development. We have already seen developers in the ecosystem evaluating running large models, including LLaMA, on Raspberry Pi devices. The deployment of large models and generative AI use cases at the edge is imminent, and Arm is ready to challenge the performance and efficiency limits of the Internet of Things and large models, multi-modal AI,” Ma said.
Intelligence is ubiquitous, and cloud, edge, and end are indispensable. Currently, about 90% of AI runs on CPUs based on Arm architecture, and these continuous investments have made Arm one of the most prevalent AI computing platforms globally, attracting more and more developers to join. “From the Helium vector-enhanced instruction set of Cortex-M embedded processors to the optimization for vector and matrix operations of SVE, SVE2, and SME in Cortex-A application processors, to the Ethos-U AI accelerators, with the continuous development of edge AI, Arm remains at the core of driving market and technological advancements, but we will not stop there,” Ma stated.
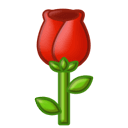