According to reports from Electronic Enthusiasts (Written by Li Wanwan), in the era of artificial intelligence, more and more AI applications need to extend from the cloud to the edge, such as smart headphones, smart cameras, smart bracelets, logistics robots, etc. Deploying AI at the edge has become a trend. With the rapid development of large AI models, deploying large AI models on the edge has also become a focus of industry attention.
How to Deploy AI Models at the Edge
First, hardware and software compatibility is necessary. On the hardware side, the more compatible AI chips there are, the better, as this reduces the difficulty of adapting and migrating models to the edge for engineers. Even if the device changes, deployment can still be smooth. On the software side, the main operating systems need to be compatible, including Linux, Windows, Android, and iOS, so that deployment can occur on both mobile devices and PCs. Framework compatibility is also better when more frameworks are supported, such as PaddlePaddle, TensorFlow, PyTorch, Caffe, and MXNet.
Secondly, model compression is required. While ensuring high accuracy, it is best to make the model run faster and use less memory. This requires employing model compression techniques, such as model quantization, pruning, and distillation. Especially with the rapid development of large AI models, future deployment of large models on the edge will inevitably require compression technology.
It can be said that model compression technology is the core technology for deploying large AI models at the edge. Model compression technology can reduce the demand for inference computing power while maintaining the original performance and accuracy of the large model.
Specifically, quantization involves converting floating-point calculations into low-bit fixed-point calculations; network pruning involves removing redundant channels, neurons, and nodes in the neural network; knowledge distillation involves using a large model as a teacher model to train a simpler model that closely mimics its output.
For example, the 130 billion parameter model GLM-130B released by Professor Tang Jie’s team at Tsinghua University in August 2022 originally required inference on an A100 40G*8 or V100 32G*8 server. However, after quantizing the model to INT 4 precision, the required GPU memory was reduced by 50% compared to INT 8 precision, and inference could be performed on a server with 4×RTX 3090 (24G) or 8×RTX 2080Ti (11G).
Many Manufacturers Achieving Edge Deployment of Large AI Models
Manufacturers such as Google, Qualcomm, and Huawei have already achieved the deployment of large AI models at the edge. In May of this year, at the Google I/O developer conference, Google announced a new version of TensorFlow optimized specifically for mobile devices, called TensorFlow Lite. This software library, known as TensorFlow Lite, is a deep learning tool that can run on mobile devices, allowing developers to run AI applications in real-time on users’ mobile devices.
This software library is designed for high speed and small storage, supporting both iOS and Android systems. If developers use other systems, they can compile TensorFlow into a software library supported by mobile operating systems through a series of complex and lengthy compilation processes, without changing TensorFlow’s functionality.
TensorFlow Lite also provides a limited number of pre-trained AI models, including MobileNet and InceptionV3 object recognition models, as well as SmartReplay natural language processing models. Custom models created by developers using their own datasets can also be deployed on it. TensorFlow Lite uses the Android Neural Networks API, allowing direct CPU processing without acceleration hardware, ensuring compatibility across different devices.
Qualcomm’s Senior Vice President of Product Management and AI Head Ziad Asghar previously stated that with the rapid proliferation of generative AI, the importance of heterogeneous processing has become unprecedentedly prominent. The importance of heterogeneous processing in AI is highlighted, just as traditional computing has evolved from large mainframes and thin clients to a model combining cloud and edge terminals, AI processing must be mixed between the cloud and terminals to maximize its potential.
According to Qualcomm’s demonstration, setting a phone to “airplane mode” and then performing full-stack AI optimization on the phone allows the model to run entirely on the terminal, completing 20 steps of inference in 15 seconds and generating detailed images. Importantly, even in airplane mode, these AI capabilities can be realized, such as integrating the capabilities of Stable Diffusion into camera applications, allowing users to take photos anywhere and request AI to change the photo’s background to the Great Wall under the sunset.
Ziad Asghar revealed that running a generative AI model with over 1 billion parameters in the cloud could require hundreds of watts of power, while running it on the terminal only requires a few milliwatts. This gives Qualcomm a unique advantage in the field of generative AI. In the near future, models with 10 billion or more parameters will be able to run on terminals.
During the spring flagship product launch in March this year, Huawei introduced a new smart image search function. Based on multimodal large model technology, it performs miniaturization of models on the mobile terminal, achieving an industry-first accurate natural language search experience in mobile photo galleries. Users can wake up Xiao Yi with voice, using natural language to search for photos in their mobile gallery that match descriptions like “watching the sunrise from the mountaintop,” “boiling tea by the fire,” and “clownfish in blue coral.”
Compared to traditional photo galleries that use tags for photo searches, which often suffer from low accuracy and slow response times, smart image search is much “smarter.” Combining multimodal large model technology, smart image search pre-trains on hundreds of millions of image-text data, enhancing its understanding of generalized semantic meanings, and supports natural language searches that include combinations of information such as color, shape, object, behavior, time, and place, while also achieving lightweight applications on the edge.
Due to the many advantages of deploying AI at the edge or terminal side, the penetration rate of AI applications at the edge has been increasing in recent years. With the rapid development of large AI models, deployment on terminals is also an inevitable trend. Many manufacturers have explored this and made breakthroughs, and we look forward to large AI models being able to genuinely empower various industries in the future.
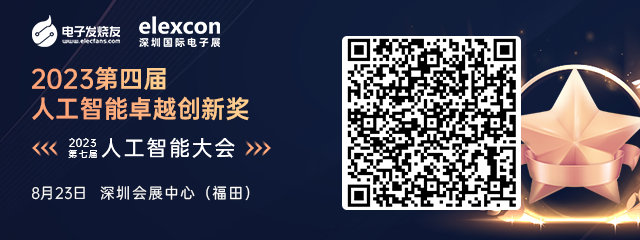
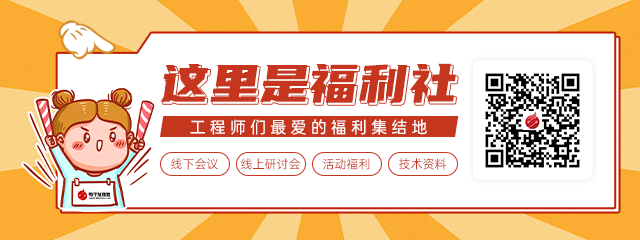

Disclaimer: This article is original from Electronic Enthusiasts, please indicate the source above if reprinted. If you want to join the group for communication, please add WeChat elecfans999, for submission, reporting, and interview requests, please send an email to [email protected].
More Hot Articles to Read
-
AI Drives a Trillion Future, Various Giants Hunt the Chip Market
-
How is SiC Applied in the Charging Pile Market Driven by 400kW High Power Demand?
-
Main Chip Players and Patent Breakthroughs in MicroLED
-
Intel Invests $50 Billion! Global Expansion of IDM 2.0 Strategy Begins to Show Results
-
“Zombie Charging Piles” Will Become History! Policies Drive the Increase of Charging Piles, High-Power Charging Piles Boost the Industry Chain!