Click the blue text above to follow us
Author Introduction:Yin Rui, PhD, Associate Professor, Master’s Supervisor, School of Educational Information Technology, South China Normal University (Guangzhou, Guangdong 510631); He Shuyin, Master’s student, School of Educational Information Technology, South China Normal University (Guangzhou, Guangdong 510631).
Funding Project:The 2019 Guangdong Higher Education Teaching Reform Project “Design Thinking-Oriented Online Open Course Mixed Teaching Reform and Practice Innovation for Pre-Service Teachers’ Information Technology Application Ability”; South China Normal University “Challenge Cup” Seed Cultivation Project “Research on Evaluation Model of Mixed Learning Input in Colleges and Universities Based on Multi-Source Data Analysis”.
Reference:Yin Rui, He Shuyin (2022). Research Progress and Prospects of Multimodal Learning Analytics Based on Systematic Literature Review[J]. Modern Distance Education Research, 34(6):54-63.
Abstract:Multimodal learning analytics refers to the use of the concept and methods of “multimodal” to deeply interpret learners’ intrinsic learning states, characteristics, and changes, aiming to explore learning patterns, optimize the learning process, and promote precise teaching. Under the research paradigm of educational big data, multimodal learning analytics has emerged and formed many research results. A systematic literature review method was used to sort out relevant research on multimodal learning analytics at home and abroad, revealing that current research focuses on three main aspects: data collection, data fusion, and data modeling. In terms of data collection, thanks to the development of intelligent sensing technology, modalities such as text, voice, motion, expression, eye movement, and physiological data have received much attention, and their acquisition methods have become increasingly convenient; in terms of data fusion, it mainly focuses on data layer fusion, feature layer fusion, and decision layer fusion, with hybrid fusion strategies beginning to emerge due to the development of deep learning algorithms; in terms of data modeling, learner models oriented towards knowledge, cognition, emotion, and interaction states have emerged, and holistic models based on multiple learning states are increasingly valued. Future research on multimodal learning analytics should strengthen situational awareness to achieve mixed collection of scene data; deepen the theoretical foundation to promote scientific precision in data fusion; and emphasize situational dependence to strengthen the contextual applicability of data modeling.
Keywords:Multimodal Learning Analytics; Data Fusion; Data Modeling; Learner Models; Systematic Literature Review
In December 2021, the Central Cybersecurity and Informatization Commission issued the “14th Five-Year National Informatization Plan”, proposing to establish a resource system for efficient utilization of data elements (Central Cybersecurity and Informatization Commission, 2021), stimulating and enhancing the empowering role of data elements to lead and create new demands through innovation-driven, high-quality supply. In the field of education, stimulating and enhancing the innovative driving role of data is a new trend in deepening the reform of education evaluation in the new era. With the vigorous development of new generation information technologies such as big data, the Internet of Things, mobile internet, and artificial intelligence, the empowering role of educational data is gradually becoming popular, leading educational research from “hypothesis-driven” to “data-driven”, giving rise to the “educational big data research paradigm” (Zhao Jiali et al., 2020). Against this background, Multimodal Learning Analytics (MMLA) has quietly emerged and entered people’s field of vision. It breaks the limitations of traditional evaluation that only relies on single-channel data collection mediated by computers (such as online learning management systems and social network environments) to capture single-modal data, advocating the capture, fusion, and analysis of multi-source heterogeneous data such as voice, behavior, expression, and physiology across contexts and spaces, to interpret and predict learners’ behavioral habits, cognitive patterns, psychological states, and emotional changes. This has important value for constructing learner models that connect high-level learning theories with underlying data, achieving scientific, precise, personalized, and panoramic teaching evaluation, and better optimizing learners’ learning experiences, helping learners engage more deeply and effectively in their studies.
Currently, multimodal learning analytics is gaining momentum and becoming a focal topic for scholars to understand and optimize learning. However, how to collect data (Worsley et al., 2015; Spikol et al., 2017), fuse data (Kadadi et al., 2014; Samuelsen et al., 2019), and model data (Mu Zhijia, 2020; Wang Yiyan et al., 2021) remains urgent issues that need to be addressed as multimodal learning analytics transitions from case studies in educational experimental environments to comprehensive perspectives in real educational scenarios. Therefore, this study will focus on sorting out and explaining the three major issues of data collection, fusion, and modeling in multimodal learning analytics, aiming to provide an overall approach for the application of multimodal learning analytics.
This study adopts a systematic literature review method to search, screen, and evaluate relevant literature, ultimately determining the research literature sample.
1. Literature Acquisition Methods
To effectively search for relevant research literature on multimodal learning analytics at home and abroad, this study searched authoritative databases such as Web of Science, Springer Link, and Science Direct, as well as conference proceedings from the International Conference on Multimodal Interaction (ICMI), International Conference on Learning Analytics and Knowledge (LAK), ACM International Conference on Human Factors in Computer Systems (ACM CHI), and ACM International Conference on Multimedia (ACM MM), using keywords such as “Multimodal Learning Analytics”, “Multimodal Data”, and “Multimodal Sensory Data” to search for relevant foreign literature. At the same time, relevant Chinese literature was searched in the CNKI database using keywords such as “多模态学习分析” and “多模态数据”. The publication time of the literature was limited to January 2012 – July 2022, resulting in a total of 2052 articles, including 1801 foreign articles and 251 Chinese articles.
2. Literature Screening Process
To ensure the accuracy of the sample literature included, the study referred to existing systematic literature review screening criteria (e.g., papers must be fully accessible, research themes must revolve around multimodal learning analytics, and empirical research or literature review methods must be used) and used a “snowball” method to search, read, and include more literature that meets the criteria, ultimately screening out a total of 68 articles that meet the standards. The literature screening process is shown in Figure 1.
Figure 1 Literature Screening Process
3. Data Collection in Multimodal Learning Analytics
Data collection of multimodal data is the foundation and key of multimodal learning analytics. Existing studies have classified multimodal data according to different criteria: Chen Kaiquan et al. (2019) categorized all data generated during the learning process into explicit data, psychological data, physiological data, and basic data based on external and internal levels; Mu Zhijia (2020) classified data into learning characteristic data, human-computer interaction data, learning resource data, and learning context data based on the spatial structure of multimodal learning analytics; Wang Weifu et al. (2021) further subdivided learning characteristic data into controllable action data and accompanying physiological data; and Mu Su et al. (2021) categorized data into digital space data, physical space data, physiological characteristic data, environmental space data, and psychological measurement data based on the field in which the data is generated. If classified according to the interaction between the human sensory system and the external environment, data can be divided into text, voice, motion, expression, eye movement, and physiological multimodal data.
Text data refers to data generated by learners in the learning process represented in written language form, such as thematic speeches, question comments, reflective reports, etc. This data can be collected through online learning management systems. With the rise of smart pens, handwriting recognition technology can achieve simultaneous transcription of learners’ process text data in classroom teaching scenarios. Typically, text data can be used to predict learners’ cognitive states. Some researchers conducted cognitive network analysis on writing texts submitted by 150 pre-service teachers majoring in elementary education in an online learning management system with the topic “My Educational Philosophy” and found that in the comparison of achievement levels, the professional cognitive network of the excellent group of pre-service teachers was more complex and rich; in the gender comparison, female pre-service teachers could organically combine different cognitive contents under the same cognitive skill pattern, while male pre-service teachers often did not adhere to a specific cognitive skill acquisition (Wu Xiaomeng et al., 2021).
Voice data refers to the verbal content of learners in dialogue situations, including both person-to-person and human-computer dialogues. For the former, multi-directional microphones can automatically collect voice data from learners’ interactions in collaborative learning contexts to explain the levels of learners’ collaborative knowledge construction. For the latter, intelligent learning tools are the main tools for obtaining this type of voice data. For example, in language learning scenarios, intelligent language learning tools can automatically collect content from learners’ conversations with intelligent devices to interpret learners’ knowledge acquisition performance. In addition to content-related voice data, prosodic data such as tone, intonation, and speech rate also belong to voice data and can be used to explain learners’ emotional states. Worsley et al. (Worsley et al., 2011) used Praat, a natural language processing software, to collect the verbal thoughts of college students completing electronic and mechanical engineering design tasks, analyzing prosodic features (including pitch, intensity, and speech duration), fluency (including pauses, fillers, and restarts), and emotional expressions, discovering that novice learners tended to use understated language, while expert learners preferred to use affirmative expressions.
Action data refers to data identifying and representing movements of various parts of learners’ bodies, such as head movements, gesture changes, and leg movements. This data can be collected through video from non-contact recording devices (such as cameras) and extracted from the video using relevant algorithms. With the development of motion-sensing technology, the action data that can be captured and used for learning analytics will become increasingly refined, such as angles of head movements, positions of gesture changes, and directions of leg movements. Generally, there are three sensing methods for motion-sensing technology: first, using inertial sensors such as gravity sensors, accelerometers, gyroscopes, and magnetic sensors to sense local body movements; second, using optical sensors to obtain full-body images; and third, combining inertial and optical sensors to sense the direction and displacement of body movements. Action data can not only determine learners’ behavioral trajectories and sequences, reflecting learners’ behavioral characteristics but can also predict the interaction states between learners and their environment and the development of their cognitive states. For example, Andrade (Andrade, 2017) collected data on learners’ hand movements in an embodied interaction learning environment, finding that the sequence of learners’ hand movements was closely related to their understanding of feedback loops in ecosystems.
Expression data refers to data capturing and identifying learners’ facial expression characteristics. This data can be collected through cameras and facial recognition systems, and with sensing technology, subtle changes in expressions can also be tracked and captured. Monkaresi et al. (Monkaresi et al., 2017) used the Microsoft Kinect face tracker to capture all facial expressions of learners completing structured writing activities and allowed learners to annotate their facial expressions’ engagement states in retrospective activity videos, finding that the expression data extracted by the face tracker could effectively measure learners’ engagement states.
Eye movement data refers to data such as fixation trajectories, fixation duration, saccade direction, pupil size, blink frequency, and scanning rate obtained through eye trackers. It is a key indicator of attention (Reichle et al., 2009; Sharma et al., 2019). Some researchers have used eye movement data to determine learners’ decision-making strategies in complex learning tasks (Renkewitz et al., 2012), and other researchers compared eye movement data such as fixation trajectories, fixation duration, and fixation counts of experts and novices when viewing new maps to assess cognitive loads and their differences (Ooms et al., 2012). Due to the high cost of eye movement data collection devices, existing studies are mainly conducted in laboratory environments, with few studies in real environments.
Physiological data refers to basic vital sign data such as learners’ body temperature, blood pressure, heart rate, respiration, blood flow, as well as brain electrical signals (Electroencephalogram, EEG), and skin electrical responses (Galvanic Skin Response, GSR). The acquisition of this data usually requires specific devices (such as EEG headsets, wristbands, etc.). Recently, a new method has emerged that does not require special hardware, only a toolkit developed based on webcams and machine learning algorithms, which can automatically collect physiological data in internet environments without browser or programming experience limitations. Moreover, this method is gradually extending to the collection of eye movement data, significantly reducing the difficulty of multimodal data collection. For example, the EZ-MMLA toolkit developed by Hassan et al. (Hassan et al., 2021) at Harvard University’s Learning, Innovation, and Technology Lab can achieve automatic collection of various modal data. Researchers often use EEG signals to estimate learners’ engagement and cognitive load while completing tasks (Mills et al., 2017; Hassib et al., 2017), and skin electrical responses to assess learners’ emotional arousal states (Pijeira-Diaz et al., 2019). However, while skin electrical responses can monitor emotional arousal, they cannot determine whether the arousal is caused by positive or negative stimuli; therefore, to diagnose the positivity or negativity of emotions, it is best to integrate data from other modalities (such as eye movement data, expression data, and EEG signals) for comprehensive analysis.
4. Data Fusion in Multimodal Learning Analytics
Research on educational big data follows the paradigm of knowledge discovery driven by data and algorithms (Zhao Jiali et al., 2020). Although multimodal learning data provides diverse information support for comprehensively perceiving learners’ true learning states, to reveal the underlying mechanisms and patterns of learning, it is necessary to utilize the information complementarity mechanism of multimodal data and re-fuse different modalities’ datasets according to certain rules and relationships to fully exploit the hidden information behind multimodal data, objectively and comprehensively revealing learners’ cognitive patterns. The purpose of data fusion is to associate and integrate two or more datasets based on key features, generating a coherent, aligned, and mutually corroborative evidence picture based on multimodal data (Wang Weifu et al., 2021), thereby leading to more robust predictions and providing a basis for subsequent personalized interventions and adaptive feedback (Wu Yonghe et al., 2021). Currently, researchers mainly use machine learning methods for data fusion to achieve integration and layered abstraction of multimodal data’s internal characteristics. According to the level of information abstraction, multimodal data fusion strategies can be divided into three levels from low to high: data layer fusion, feature layer fusion, and decision layer fusion. To maximize the advantages of multimodal data, researchers attempt to combine different levels of fusion strategies to form a hybrid fusion strategy. Figure 2 illustrates the four fusion strategies.
Figure 2 Illustration of Multimodal Data Fusion Strategies
Data layer fusion refers to the direct input of raw data (i.e., unprocessed or simply preprocessed data) from various modalities into the fusion center for completion. The characteristic of this level of fusion is that it retains as much raw information as possible, but it may also reduce model performance due to information redundancy. When using data layer fusion strategies, it is first necessary to find the temporal or spatial correspondence of the same instance in different modal information, align the data, and then use adaptive weighting-based fusion methods, correlation function-based fusion methods, etc. to train the data to obtain the model, and finally evaluate the model’s performance using indicators such as accuracy, precision, recall, P-R curve, root mean square error (RMSE), etc. For example, Song Dan et al. (2020) collected the grades of students from different courses in the first two years and established a mutual prediction model of professional course grades based on the correlation between grades. Additionally, Pardo et al. (Pardo et al., 2017) had learners simulate controlling the relationship between predators and prey in a computer interface through gesture actions while learning the concept of ecosystems, merging gesture action sequences with gaze data to establish a fusion model of learning patterns and learning outcomes.
Feature layer fusion refers to the extraction of features from the original data of each modality, followed by correlation and normalization processing of the extracted data, and then integrating features to complete data fusion. This level of fusion has the following two characteristics:
First, it requires feature extraction before data fusion can be achieved through machine learning algorithms. There are mainly two methods for feature extraction: one is to identify modality features related to learning indicators through theoretical research and empirical assumptions, then extract data features through algorithms or professional software. For example, Spikol et al. (Spikol et al., 2018) obtained multimodal data such as video data, audio data, and programming process data to construct a performance prediction model in group project learning, and then used experience to apply algorithms such as the Viola-Jones algorithm and fast Fourier transform algorithm to extract facial distance from peers, the number of faces gazing at the screen, and audio frequency during conversation, before proceeding to data fusion. The other method is to simultaneously achieve data fusion and feature extraction through deep learning algorithms. For example, some researchers collected data using depth cameras and wearable devices, performed preliminary data extraction through applications associated with hardware devices, and then employed long short-term memory network algorithms for data fusion, thus achieving feature extraction during the fusion process (Di Mitri et al., 2019).
Second, there is a strong correlation between features across modalities, leading to high data redundancy and making it difficult to find complementarity between multimodal data. Therefore, it is necessary to select the extracted features to obtain key features that can represent predictive indicators. Common methods include filtering methods, which eliminate useless features using principal component analysis, correlation analysis, etc., independent of machine learning algorithms; embedding methods, where algorithms like logistic regression and random forests determine which features to retain, which is a method that simultaneously performs feature selection and algorithm training; and wrapping methods, which continuously trim features until the best feature combination for predictive modeling is found, which is a method involving repeated model training. In practical applications, when the data volume is large, it is advisable to first use filtering algorithms to eliminate a large number of features, and then use embedding or wrapping methods for feature selection.
Using this strategy for multimodal data fusion can occur in two scenarios: one is to not consider the semantic relationships between modality features, directly using machine learning algorithms to process the extracted and selected features for fusion, typically employing supervised learning algorithms. For instance, Giannakos et al. (Giannakos et al., 2019) collected eye movement data, EEG data, facial data, and clickstream data from a gamified learning environment, extracted 689 features from these, and then used random forest algorithms to fuse these features, ultimately constructing an optimal modal combination and learning performance prediction model. The second is to consider the semantic relationships between modality features, using simple fusion operations based on concatenation and linear combinations, attention mechanism-based fusion methods, bilinear pooling-based fusion methods, and tensor fusion methods to complete feature fusion. For example, Chen et al. (Chen et al., 2019) extracted features from text data and voice data, and then used attention mechanism-based fusion methods to merge these feature vectors, resulting in a model for emotion state recognition.
Decision layer fusion refers to processing different modal data through a series of steps (including preprocessing, feature extraction, recognition, etc.), training multiple models from different modal data, and then combining these models to complete data fusion. This level of fusion has two characteristics: one is that the effectiveness of the fusion results is related to the combination of models, and since model performance is independent, the final model formed after fusion has high fault tolerance and anti-interference performance. The second is that the correlation between modalities is not high, thus helping to leverage the complementarity between multimodal data, better reflecting the complementary mechanism of the data.
When using decision layer fusion strategies, one can first label each modality’s data at specific time intervals, then construct predictive models for each modality concerning learning indicators using machine learning algorithms, and finally fuse the models using methods such as maximum value fusion, average value fusion, Bayesian rule fusion, and ensemble learning, obtaining the optimal model after evaluating model performance. Among these, ensemble learning methods include ensemble classifiers based on majority voting mechanisms, bagging ensemble techniques based on the Bagging idea, boosting methods based on the Boosting idea, stacking frameworks for hierarchical model integration, and ensemble learning based on neural networks. For example, Ashwin et al. (Ashwin et al., 2020) collected image data of facial expressions, gestures, and body postures in face-to-face learning environments, then trained posed single and multiple image data using convolutional neural network algorithms to obtain CNN-1 and CNN-2 models, respectively, and finally fused these two models through neural network weight matrices to obtain a classifier model for emotional states such as engagement, boredom, and neutrality in classroom natural contexts. Zhang Qi et al. (2020) collected facial data and biological signal data in online learning environments, performed feature extraction, and then constructed emotional models for happiness, boredom, as well as positive and negative emotions, and finally used hidden Markov models for time series association processing, obtaining optimal feature combinations and emotional state fusion models using recurrent neural networks and long short-term memory networks.
With the continuous emergence of deep learning algorithms, data layer fusion and feature layer fusion are collectively referred to as early fusion, while decision layer fusion is referred to as late fusion. Hybrid fusion is a new data fusion strategy that combines early and late fusion. It involves training multiple models for each modal data, then combining several models to form a multimodal predictor, and finally combining it with unimodal predictors to complete data fusion. For example, Luo et al. (Luo et al., 2022) collected head posture data, facial expression data, and online platform interaction data in online learning environments, where head posture data and facial expression data employed decision layer fusion strategies, while online interaction data used feature layer fusion strategies, ultimately using analytic hierarchy processes to obtain a weight matrix, thereby achieving multimodal data fusion and constructing a learning interest prediction model comprising cognitive attention, learning emotions, and cognitive activities. Although this data fusion strategy integrates the advantages of early and late fusion, it also increases the structural complexity and training difficulty of the model.
Currently, no researchers have explicitly pointed out which algorithms or fusion strategies are more suitable for solving which types of problems in multimodal learning analytics. Therefore, to comprehensively understand learners’ learning issues and predict their potential trends, it is necessary to use multiple algorithms to construct several predictive models and select the best one after comparing the predictive performance of the models.
5. Data Modeling in Multimodal Learning Analytics
Multimodal learning analytics emphasizes using models to achieve mathematical explanatory logic in educational evidence. Among them, learner models are the primary models for learning analytics. From the learning process perspective, by comprehensively collecting and analyzing multimodal data such as learners’ language, actions, expressions, eye movements, and physiological data, it is possible to model learners’ knowledge, cognition, emotions, and interaction states, accurately portraying learners’ learning characteristics and exploring and explaining learners’ learning patterns at a deeper level.
1. Knowledge State Modeling
The knowledge state not only describes the current level of knowledge mastery in a certain knowledge domain by the learner but also includes a description of the previously mastered knowledge level. Knowledge state modeling for learners typically relies on data collected from multiple tests during a certain learning period, supplemented by sketch or concept map data created by learners, to visualize knowledge resources and their carriers using knowledge graph techniques, employing methods such as naive Bayes and convolutional neural networks to display individual knowledge development processes and their structural relationships, thereby providing personalized resource recommendations and learning path planning for learners, making data-driven “personalized teaching” possible.
2. Cognitive State Modeling
Cognitive state describes the internal information processing of learners. Cognitive state modeling for learners can typically leverage learning behavior data and text data. Given the high correlation between physiological data and human brain neural system activities, there is increasing attention on using physiological data to construct cognitive state models. Larmuseau et al. (Larmuseau et al., 2020) measured and collected psychological data, such as performance on different types of tasks, breadth of cognitive operations, basic levels of working memory, and self-reported cognitive load levels, along with physiological data such as heart rate, heart rate variability, skin electrical responses, and skin temperature, to gain insights into learners’ cognitive load during online problem-solving, constructing a cognitive load prediction model comprising learners’ physiological characteristics, breadth of cognitive operations, and performance. The results indicated that when learners completed breadth of operation tests, heart rate and skin temperature were the best predictors of cognitive load levels, implying that learners’ cognitive load increases with the difficulty of problems, although learning skills improve, cognitive load does not significantly decrease. This model describes the internal cognitive state changes of learners when solving problems in online learning environments, providing scientific grounds for teachers to better design online learning tasks based on learners’ cognitive levels.
3. Emotional State Modeling
Emotional state modeling aims to achieve precise collection of learners’ voice, expressions, and physiological multimodal data through technologies such as natural language processing, computer vision, and speech recognition, as well as various intelligent sensing devices, thus conducting comprehensive modeling analysis of learners’ emotional external representation patterns and internal mechanisms, to construct multi-layered emotional models that integrate external stimuli, internal physiological states, and psychological statuses, accurately assessing learners’ emotional attitudes and their changes during the learning process, timely identifying factors affecting learners’ emotions, and providing timely emotional encouragement and support. Facial expression data and physiological data are common data sources for emotional models. Ray et al. (Ray et al., 2016) designed and developed a multimodal learning emotion recognition system that collected physiological data such as heart rate, skin electrical responses, blood pressure, and facial expression data from learners during classroom learning, using learners’ self-reported emotional states (including disgust, sadness, happiness, fear, anger, surprise) as outputs, constructing emotional matrices for physiological and facial expression data respectively using the DTREG tool, thus achieving data fusion at the decision level. The results indicated that the emotional model formed by fusing physiological data and facial expression data could achieve higher accuracy than predictions based on single-modal data. Similarly, Pham et al. (Pham et al., 2018) assessed learners’ emotional states during mobile MOOC learning using multimodal learning analytics technology, capturing facial expression data with the front camera and fingertip light pulse image signals (Photo Plethysmo Graphy, PPG) with the rear camera while controlling video playback, and using support vector machine methods for data fusion, also finding that the combination of physiological data and facial expression data was the best model for predicting academic emotions.
Additionally, some researchers have attempted to fuse other modal data to construct emotional state models. Henderson et al. (Henderson et al., 2020) obtained posture data, gameplay data, and emotion state data observed by researchers from learners participating in emergency medical skills training games, and used deep neural networks to fuse these multimodal data, thus constructing emotional models for learners in gamified learning environments. Luo et al. (Luo et al., 2022) collected learners’ head posture data, facial expressions, and interactive learning data using cameras and learning platforms, and constructed a learning interest model predicted by three types of data using a weighted hierarchical fusion method, thus providing support for teachers to measure learners’ learning interest in real-time and make timely interventions.
4. Interaction State Modeling
Interaction state refers to the communication and interaction between learners and teachers or peers. In previous learning analytics research, interaction state models were mainly achieved through social network analysis of learners’ behavior data on learning platforms. With the development of multimodal data collection technologies and fusion algorithms, data reflecting interaction states have become more multidimensional and refined. Spikol et al. (Spikol et al., 2018) collected interaction action data, voice data, and hardware and software types used in programming automatically recorded by Arduino boards, as well as data reflecting the results of planning, implementation, and reflection phases recorded in mobile tools, from learners completing STEM projects collaboratively, and constructed interaction state models using deep neural networks. This study particularly pointed out that the distance between peers’ hands and heads during collaborative completion of complex tasks could predict the quality of collaborative learning.
5. Comprehensive State Modeling
Comprehensive state modeling refers to the establishment of integrative models through comprehensive analysis of multiple learning states of learners. These models focus on the overall performance of learners in behavior, cognition, emotion, interaction, etc., such as learning engagement models, self-regulated learning models, and academic performance prediction models. For instance, Papamitsiou et al. (Papamitsiou et al., 2020) conceptualized learning engagement in adaptive learning activities as behavior, feelings, and thoughts, collecting log records, eye movement data, physiological data, expression data, and questionnaire data related to these dimensions, and obtained the best variable combination explaining academic performance through fuzzy-set qualitative comparative analysis (fsQCA) methods (as shown in Figure 3). This study well demonstrated the complex mapping relationship between different types of learning activities and multimodal data, as well as the predictive analysis of academic performance based on mixed research methods, providing a good analytical framework for researchers and practitioners.
Figure 3 Mapping Relationship Between Academic Performance Prediction Models and Data Types
In addition to considering learners’ own situations as predictive variables, some researchers have also taken the contextual dependence of models into account, incorporating learning environment data and types of learning activities into the predictive variables for academic performance. For example, Mangaroska et al. (Mangaroska et al., 2021) based on cognitive load theory and the model of affective dynamics, obtained EEG data, eye movement data, expression data, and platform log data from learners during reading and coding events in understanding and optimizing code phases on programming platforms. Among these, EEG data was used to represent attention, convergent thinking, and memory load; eye movement data represented learners’ fixation durations and cognitive loads in different areas of the programming platform (coding area, programming problem area, console area); expression data represented emotions such as satisfaction, frustration, boredom, and confusion; and platform log data represented reading and coding events, ultimately employing linear regression algorithms to construct academic performance prediction models based on learners’ gaze on different areas of the programming platform during different learning phases.
6. Discussion and Prospects
Multimodal learning analytics aims to utilize the concept and methods of “multimodal” to deeply interpret learners’ intrinsic learning states, characteristics, and changes, thereby exploring learning patterns, optimizing the learning process, and promoting precise teaching. In the literature review, we found that although multimodal learning analytics has made significant progress in data collection, data fusion, and data modeling, there are still issues such as the difficulty of cross-scenario data collection, weak theoretical foundations for data fusion, and a lack of contextual dependence in data modeling. Future research on multimodal learning analytics can be deepened in the following aspects:
1. Strengthen situational awareness to achieve mixed collection of scene data
Situational context is an important factor representing educational data, capable of reflecting learners’ true learning states in complex teaching environments, playing a key role in constructing learning models. With the development of the Internet of Things and intelligent sensing technologies, how to achieve precise modeling of educational contexts across physical and digital worlds is becoming a research trend (Mu Zhijia, 2020). Based on literature analysis, the current data collection in multimodal learning analytics faces two major issues: first, the collected data mostly consists of simple, fragmented learning performance data, with little coherent process data captured at the time series level, making it difficult to comprehensively and timely reflect the dynamic learning process. Second, the collected data is mainly from single spatial and temporal fields, making it challenging to achieve “seamless connection” across different online and offline scenarios and closely couple with real teaching contexts.
To address this, future schools need to construct intelligent educational environments that integrate situational awareness technologies, imaging technologies, tracking and evaluation technologies, and platform data collection technologies, thereby supporting the online and offline scenario collection of multimodal learning data from the perspective of learning space construction, and achieving comprehensive and detailed data collection through a four-layer framework of “situation-activity-event-data”. Teaching situational data can be classified and collected from both online and offline temporal and spatial dimensions, including information related to users, time, tasks, physical environments, and equipment related to learners. Since data is merely symbolic records used to describe things, it does not provide judgments or meanings on its own; the meaning of data acquisition needs to be associated with specific business contexts (Wang Dongqing et al., 2018). Therefore, by establishing connections between online and offline cross-temporal and spatial teaching scene data, the learning activity data can be integrated to reflect the learning process in time series, providing a detailed portrayal of learners’ overall learning process and helping to uncover learners’ learning change patterns throughout the learning process; it can also achieve dynamic descriptions of learning activities in blended teaching scenarios, aiding in constructing diversified blended learning activity paths. Learning event data refers to the multimodal datasets generated by learners when completing specific learning tasks during the learning process. As previously mentioned, to understand learners’ programming learning performance, Mangaroska et al. (Mangaroska et al., 2021) conducted real-time data collection based on online programming platforms, obtaining data chains when learners completed different activity events in different areas of the programming platform. This provides valuable insights for deepening research on situational data collection in multimodal learning analytics.
2. Deepen theoretical foundations to promote scientific precision in data fusion
Data fusion is a complex and labor-intensive process (Liu et al., 2019). Existing research indicates that data fusion methods mainly fall into three categories (Mu Su et al., 2021): first, using multi-dimensional, multimodal data measurements to explain a single learning indicator; second, using multi-dimensional, multimodal data measurements to explain multiple learning indicators; and third, using multiple data to mutually corroborate and comprehensively and deeply explain a specific issue. Based on literature analysis, feature selection is key to data fusion in multimodal learning analytics. As the measurement potential of each type of data is gradually explored, the feature information contained increasingly enhances the explanatory power for the same learning indicator. Although optimizing or innovating algorithms can achieve feature filtering, having a clear learning theory to support this will make feature extraction more rational.
In the future, data fusion in multimodal learning analytics will gradually shift from supporting single feature aggregation in data layer fusion to supporting high-dimensional spatial mapping in feature layer fusion, and even to optimal decision support in decision layer fusion and hybrid fusion. Therefore, it is urgent to explore the value implications of foundational theories related to behavior, cognition, emotion, and interaction in multimodal data fusion research, enriching the complex mapping relationships between different modalities and learning, thus providing strong theoretical support for data fusion. As mentioned earlier, some researchers have begun to focus on establishing dimensions for feature extraction from learning theories such as cognitive load and affective dynamics, thereby constructing learner models. In order to achieve scientifically precise data fusion, it is also essential to track the latest theories in educational neuroscience, brain science, and learning science.
3. Emphasize contextual dependence to strengthen the contextual applicability of data modeling
Temporal and contextual characteristics are fundamental features of education. Due to limitations in cross-scenario and temporal data collection technologies, comprehensive, multimodal learning data collection for specific contexts of individual learners throughout the learning process remains inadequate. Consequently, the learner models constructed in existing research are somewhat de-contextualized, making it challenging to accurately analyze the cognitive, emotional, and interaction development patterns and mechanisms of learners in specific contexts, leading to difficulties in generalizing the constructed models.
Strengthening the contextual dependence of data modeling will become one of the key focuses of future multimodal learning analytics. On one hand, in the temporal dimension, time series analysis methods can be introduced to serialize the cognitive, emotional, and interaction development status of learners, eliminating the uncertainties represented by single temporal data, and deeply exploring learners’ periodic learning patterns while predicting their developmental trends (Huang Tao et al., 2020). On the other hand, in the spatial dimension, it has been noted that learners’ cognitive, emotional, and interaction states may vary with changes in learning space fields; for instance, the learning states exhibited by learners in online learning spaces differ from those in intelligent learning spaces or even in hybrid physical-virtual spaces. Therefore, it is necessary to quantify the spatial fields of learning and construct learning models tailored to different spatial fields. This is crucial for analyzing learners’ cognitive development, emotional changes, and interaction states in different learning contexts, thereby assisting teachers in making precise teaching decisions and activity designs. Additionally, in the task dimension, there is an urgent need to explore learners’ learning patterns, cognitive characteristics, emotional and physiological states, and social preferences in complex task contexts across different subjects, constructing cognitive, emotional, and interaction models that adapt to task contexts, or even comprehensive models, to expand and enrich case studies in multimodal data modeling, thus providing a repository of learner models for multimodal learning analytics systems tailored to different task contexts, further promoting the development of multimodal human-computer interaction.
References:
[1] Chen Kaiquan, Zhang Chunxue, Wu Yueyue et al. (2019). Multimodal Learning Analytics, Adaptive Feedback, and Human-Machine Collaboration in Educational Artificial Intelligence (EAI) [J]. Distance Education Journal, 37(5):24-34.
[2] Huang Tao, Wang Yiyan, Zhang Hao et al. (2020). Research Trends in Learner Modeling in Intelligent Educational Fields [J]. Distance Education Journal, 38(1):50-60.
[3] Mu Zhijia (2020). Multimodal Learning Analytics: A New Growth Point in Learning Analytics Research [J]. Research on Educational Technology, 41(5):27-32,51.
[4] Mu Su, Cui Meng, Huang Xiaodi (2021). A Comprehensive Perspective on Data Integration Methods in Multimodal Learning Analytics [J]. Modern Distance Education Research, 33(1):26-37,48.
[5] Song Dan, Liu Dongbo, Feng Xia (2020). Research on Course Grade Prediction and Course Warning Based on Multi-Source Data Analysis [J]. Higher Engineering Education Research, (1):189-194.
[6] Wang Weifu, Mao Meijuan (2021). Multimodal Learning Analytics: A New Direction for Understanding and Evaluating Real Learning [J]. Research on Educational Technology, 42(2):25-32.
[7] Wang Dongqing, Han Hou, Qiu Meiling et al. (2018). Dynamic Generative Data Collection Methods and Models in Smart Classrooms Based on Context Awareness [J]. Research on Educational Technology, 39(5):26-32.
[8] Wang Yiyan, Wang Yangchun, Zheng Yonghe (2021). Multimodal Learning Analytics: A New Trend Driven by “Multimodal” in Intelligent Education Research [J]. China Educational Technology, (3):88-96.
[9] Wu Xiaomeng, Niu Qianyu, Wei Ge et al. (2021). Exploration of the Characteristics of Professional Cognition of Pre-Service Teachers Majoring in Elementary Education: Based on Cognitive Network Analysis [J]. China Educational Technology, (6):135-143.
[10] Wu Yonghe, Guo Shengnan, Zhu Lijuan et al. (2021). Research on Multimodal Learning Fusion Analysis (MLFA): Theoretical Elucidation, Model Patterns, and Application Paths [J]. Distance Education Journal, 39(3):32-41.
[11] Zhang Qi, Li Fuhua, Sun Jinang (2020). Multimodal Learning Analytics: Moving Towards the Era of Computational Education [J]. China Educational Technology, (9):7-14,39.
[12] Zhao Jiali, Luo Shengquan, Sun Ju (2020). The Connotation, Characteristics, and Application Limits of the Educational Big Data Research Paradigm [J]. Modern Distance Education Research, 32(4):57-64,85.
[13] Central Cybersecurity and Informatization Commission (2021). Central Cybersecurity and Informatization Commission Issued the “14th Five-Year National Informatization Plan” [EB/OL]. [2022-03-05]. http://www.cac.gov.cn/2021-12/27/c_1642205312337636.htm.
[14] Andrade, A. (2017). Understanding Student Learning Trajectories Using Multimodal Learning Analytics Within an Embodied Interaction Learning Environment [C]// Proceedings of the 7th International Conference on Learning Analytics & Knowledge. New York: ACM:70-79.
[15] Ashwin, T. S., & Gusseti, R. M. R. (2020). Automatic Detection of Students’ Affective States in Classroom Environment Using Hybrid Convolutional Neural Networks [J]. Education and Information Technologies, 25(2):1387-1415.
[16] Chen, F., Luo, Z., & Xu, Y. et al. (2019). Complementary Fusion of Multi-Features and Multi-Modalities in Sentiment Analysis [EB/OL]. [2022-10-14]. https://arxiv.org/abs/1904.08138.pdf.
[17] Di Mitri, D., Schneider, J., & Specht, M. et al. (2019). Detecting Mistakes in CPR Training with Multimodal Data and Neural Networks [EB/OL]. [2022-02-15]. https://www.mdpi.com/1424-8220/19/14/3099.
[18] Giannakos, M. N., Sharma, K., & Pappas, I. O. et al. (2019). Multimodal Data as a Means to Understand the Learning Experience [J]. International Journal of Information Management, 48:108-119.
[19] Hassan, J., Leong, J., & Schneider, B. (2021). Multimodal Data Collection Made Easy: The EZ-MMLA Toolkit [C]// Proceedings of the 11th International Conference on Learning Analytics & Knowledge. New York, ACM:579-585.
[20] Hassib, M., Schneegass, S., & Eiglsperger, P. et al. (2017). Engage Meter: A System for Implicit Audience Engagement Sensing Using Electroencephalography [C]// Proceedings of the 35th International Conference on Human Factors in Computing Systems. USA, Denver CO:1-6.
[21] Henderson, N., Min, W., & Rowe, J. et al. (2020). Enhancing Affect Detection in Game-Based Learning Environments with Multimodal Conditional Generative Modeling [EB/OL]. [2021-07-28]. https://www.intellimedia.ncsu.edu/wp-content/uploads/henderson-icmi-2020_v3.pdf.
[22] Kadadi, A., Agrawal, R., & Nyamful, C. et al. (2014). Challenges of Data Integration and Interoperability in Big Data [C]// Proceedings of the 2014 IEEE International Conference on Big Data. USA, Washington DC:38-40.
[23] Larmuseau, C., Cornelis, J., & Lancieri, L. et al. (2020). Multimodal Learning Analytics to Investigate Cognitive Load During Online Problem Solving [J]. British Journal of Educational Technology, 51(5):1548-1562.
[24] Liu, R., Stamper, J., & Davenport, J. et al. (2019). Learning Linkages: Integrating Data Streams of Multiple Modalities and Timescales [J]. Journal of Computer Assisted Learning, 35(1):99-109.
[25] Luo, Z. Z., Chen, J. Y., & Wang, G. S. et al. (2022). A Three-Dimensional Model of Student Interest During Learning Using Multimodal Fusion with Natural Sensing Technology [J]. Interactive Learning Environments, 30(6):1117-1130.
[26] Mangaroska, K., Sharma, K., & Gasevié, D. et al. (2021). Exploring Students’ Cognitive and Affective States During Problem Solving Through Multimodal Data: Lessons Learned from a Programming Activity [J]. Journal of Computer Assisted Learning:1-20.
[27] Mills, C., Fridman, I., & Soussou, W. et al. (2017). Put Your Thinking Cap On: Detecting Cognitive Load Using EEG During Learning [C]// Proceedings of the Seventh International Learning Analytics & Knowledge Conference. Vancouver, BC:80-89.
[28] Monkaresi, H., Bosch, N., & Calvo, R. A. et al. (2017). Automated Detection of Engagement Using Video-Based Estimation of Facial Expressions and Heart Rate [J]. IEEE Transactions on Affective Computing, 8(1):5-8.
[29] Ooms, K., De Maeyer, P., & Fack, V. et al. (2012). Interpreting Maps Through the Eyes of Expert and Novice Users [J]. International Journal of Geographical Information Science, 26(10):1773-1788.
[30] Papamitsiou, Z., Pappas, I. O., & Sharma, K. et al. (2020). Utilizing Multimodal Data Through fsQCA to Explain Engagement in Adaptive Learning [J]. IEEE Transactions on Learning Technologies, 13(4):689-703.
[31] Pardo, A., Han, F., & Ellis, R. A. (2017). Combining University Student Self-Regulated Learning Indicators and Engagement with Online Learning Events to Predict Academic Performance [J]. IEEE Transactions on Learning Technologies, 10(1):82-92.
[32] Pham, P., & Wang, J. (2018). Predicting Learners’ Emotions in Mobile MOOC Learning via a Multimodal Intelligent Tutor [C]// Nkambou, R., Azevedo, R., & Vassileva, J. (Eds.). Proceedings of the Intelligent Tutoring Systems. Switzerland, Cham: Springer International Publishing:150-159.
[33] Pijeira-Diaz, H. J., Drachsler, H., & Jarvela, S. et al. (2019). Sympathetic Arousal Commonalities and Arousal Contagion During Collaborative Learning: How Attuned Are Triad Members? [J]. Computers in Human Behavior, 92:188-197.
[34] Ray, A., & Chakrabarti, A. (2016). Design and Implementation of Technology Enabled Affective Learning Using Fusion of Bio-Physical and Facial Expression [J]. Educational Technology & Society, 19(4):112-125.
[35] Reichle, E. D., Warren, T., & Mcconnell, K. (2009). Using E-Z Reader to Model the Effects of Higher Level Language Processing on Eye Movements During Reading [J]. Psychonomic Bulletin & Review, 16(1):1-21.
[36] Renkewitz, F., & Jahn, G. (2012). Memory Indexing: A Novel Method for Tracing Memory Processes in Complex Cognitive Tasks [J]. Journal of Experimental Psychology: Learning, Memory, and Cognition, 38(6):1622-1639.
[37] Samuelsen, J., Chen, W., & Wasson, B. (2019). Integrating Multiple Data Sources for Learning Analytics Review of Literature [EB/OL]. [2021-01-16]. https://link.springer.com/article/10.1186/s41039-019-0105-4.
[38] Sharma, K., Papamitsiou, Z., & Giannakos, M. (2019). Building Pipelines for Educational Data Using AI and Multimodal Analytics: A “Grey-Box” Approach [J]. British Journal of Educational Technology, 50(6):3004-3031.
[39] Spikol, D., Prieto, L. P., & Rodríguez-Triana, M. J. et al. (2017). Current and Future Multimodal Learning Analytics Data Challenges [C]// Proceedings of the Seventh International Learning Analytics & Knowledge Conference. Canada, Vancouver British Columbia:518-519.
[40] Spikol, D., Ruffaldi, E., & Dabisias, G. et al. (2018). Supervised Machine Learning in Multimodal Learning Analytics for Estimating Success in Project-Based Learning [J]. Journal of Computer Assisted Learning, 34(4):366-377.
[41] Worsley, M., & Blikstein, P. (2011). What’s an Expert? Using Learning Analytics to Identify Emergent Markers of Expertise Through Automated Speech, Sentiment and Sketch Analysis [EB/OL]. [2021-01-10]. https://www.researchgate.net/publication/2ted_Speech_Sentiment_and_Sketch_Analysis.
[42] Worsley, M., Chiluiza, K., & Grafsgaard, J. F. et al. (2015). Multimodal Learning and Analytics Grand Challenge [C]// Proceedings of the Fourth International Conference on Multimodal Interaction. USA, New York:525-529.
Submission Date: 2022-05-28 Editor: Liu Xuan
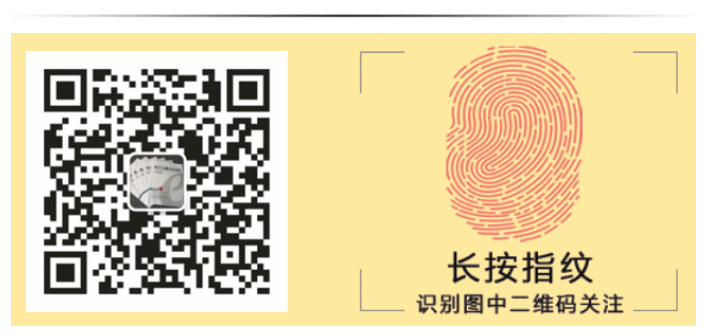
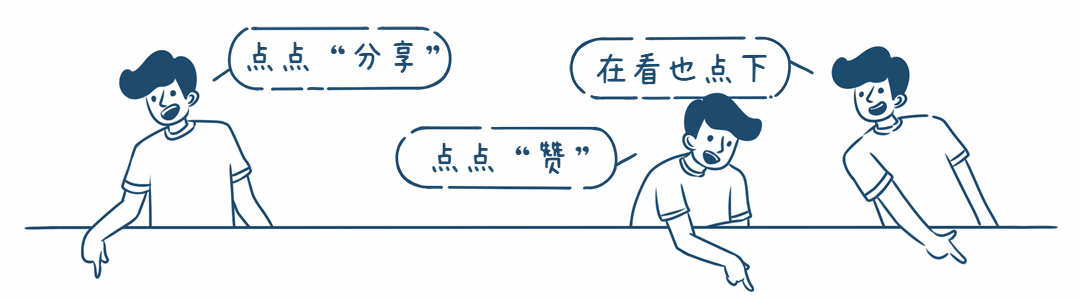
Welcome to reprint, please contact 18980806833 (WeChat)