New imaging applications are booming, from collaborative robots in Industry 4.0 to drone firefighting or agriculture, to biometric facial recognition, and handheld medical devices in homes. A key factor in the emergence of these new application scenarios is that embedded vision is more prevalent than ever. Embedded vision is not a new concept; it simply defines a system that includes a vision setup that controls and processes data without an external computer. It has been widely used in industrial quality control, with the most familiar example being the “smart camera.”
In recent years, the development of cost-effective hardware components in the consumer market has significantly reduced the bill of materials (BOM) costs and product size compared to previous computer-based solutions. For example, small system integrators (OEMs) can now procure single-board computers or module systems like NVIDIA Jetson in small quantities; larger OEMs can directly obtain image signal processors like Qualcomm Snapdragon. On the software level, available software libraries can accelerate the development speed of dedicated vision systems and reduce configuration difficulties, even for small batch production.
The second change driving the development of embedded vision systems is the emergence of machine learning, which allows neural networks in laboratories to be trained and then directly uploaded to processors so that they can automatically recognize features and make decisions in real-time.
Providing solutions suitable for embedded vision systems is crucial for imaging companies targeting these high-growth applications. Image sensors play an important role in large-scale adoption as they can directly influence the performance and design of embedded vision systems, with their main driving factors summarized as: smaller size, weight, power, and cost, abbreviated in English as “SWaP-C” (decreasing Size, Weight, Power, and Cost).
1
Reducing Costs is Crucial
The accelerator for new applications in embedded vision is the price that meets market demand, and the cost of vision systems is a major constraint to achieving this requirement.
1
Saving Optical Costs
2
Cost-Effective Sensor Interfaces
3
Reducing Development Costs
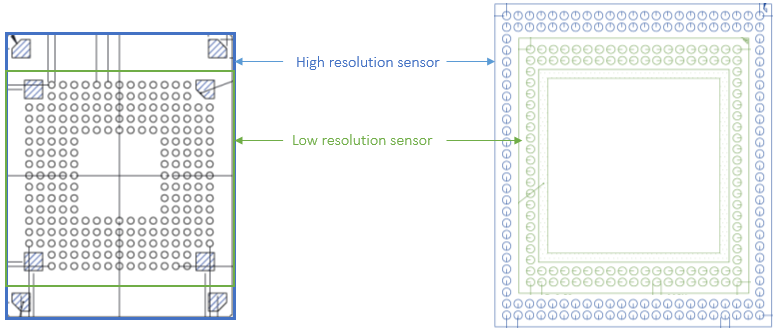
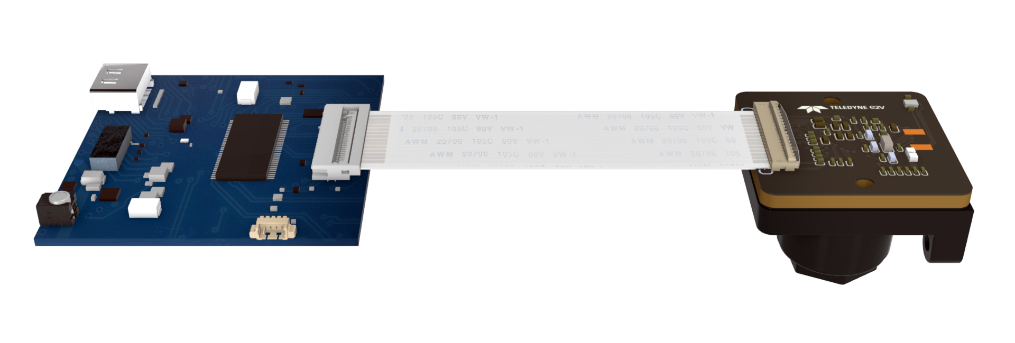
2
Improving Autonomous Energy Efficiency
3
On-Chip Functions Pave the Way for Vision System Programming
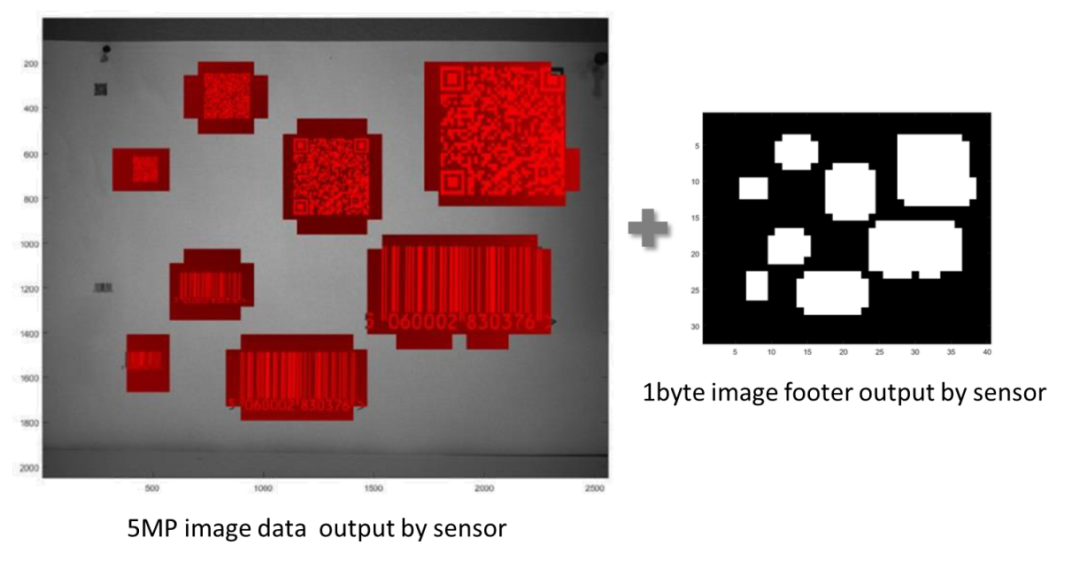