“In our research, we screened several at-risk infants through calibration by professional physicians, and then urgently contacted the parents, advising them to take their infants to a higher-level hospital for a comprehensive examination. At that moment, we truly felt the importance of what we were doing.”
Dr. Zhang Senhao and Dr. Bao Benkun from the Suzhou Institute of Biomedical Engineering and Technology, Chinese Academy of Sciences, still find great significance in a recent study they participated in.
Image | Dr. Zhang Senhao (Source: Dr. Zhang Senhao)
Recently, he and his collaborators developed a flexible sparse sensor network system that can achieve ultra-high accuracy in the automatic classification of infant restless movements.
Currently, the experimental results conducted by the team in collaboration with clinical hospitals indicate that this technology can quickly and effectively conduct large-scale screening for the risk of cerebral palsy in newborns.
In several years, this technology is expected to be promoted to more regions, becoming a new essential screening item for newborns, similar to a “vaccine.”
(Source: Advanced Science)
Due to the low cost and low resource dependency of this technology, it can effectively operate even in underdeveloped medical conditions in the Midwest and other regions, and is expected to greatly promote the development of maternal and child health in our country.
“Especially if it can be popularized in areas with low medical standards, early screening, intervention, and rehabilitation of newborns’ neurological development and behavioral habits can better alleviate the burden on families. Just thinking about this makes me feel that my efforts are worthwhile,” said Dr. Zhang Senhao.
(Source: Advanced Science)
Recently, a related paper titled “Flexible Intelligent Sparse Sensor Network for Automatic Early Evaluation of General Movements in Infants” was published in Advanced Science.
Dr. Bao Benkun and Dr. Zhang Senhao from the Suzhou Institute of Biomedical Engineering and Technology, Chinese Academy of Sciences, are co-first authors.
Dr. Zhang Senhao, Researcher Yang Hongbo from the Suzhou Institute, and Professor Cheng Huanyu from Penn State University serve as co-corresponding authors.
Image | Related paper (Source: Advanced Science)
It is reported that screening for cerebral palsy in newborns has always been a focus in clinical research.
However, previous clinical assessment methods mainly relied on experienced professional doctors observing the autonomous movement patterns of newborns over a period of time to assess whether they are at risk for cerebral palsy, and subsequently determine whether further imaging examinations are needed.
It is undeniable that assessing neurological development through the autonomous movements of newborns has certain convenience and potential for large-scale screening value.
However, the shortage of professional doctors limits its further promotion, especially in regions with low-level medical resources.
Dr. Zhang Senhao stated: “This project originated from a medical-engineering integration project jointly participated in by our researcher Cheng Xiankai, researcher Yang Hongbo, and the First Affiliated Hospital of Jilin University.”
This project aims to promote a number of promising medical-engineering integration and interdisciplinary projects to solve pain points and difficulties in clinical practice, and this project was fortunate to receive support.
Initially, the research team did not have a good solution for wearable methods and data stability.
With the support of Researcher Yang Hongbo’s international collaboration program, Dr. Zhang Senhao began participating in a joint doctoral training program in Professor Cheng Huanyu’s research group at Penn State University (PSU) in 2020.
During this period, after discussions, they believed that using flexible structures and material design methods should be more suitable for this clinical application scenario.
Dr. Zhang Senhao said: “Therefore, we started this research with the PSU team. Under Professor Cheng Huanyu’s guidance, we began to design structures and materials, and optimize networking methods and stability.”
It is understood that previous studies mostly captured movements using the “video method” and then digitized them.
However, this method cannot guarantee privacy, and motion recognition is easily disturbed by environmental factors, leading to a massive amount of background noise that limits its development in clinical settings.
Based on this, the research team envisioned: can we complete the digitization of newborn movements through direct motion information collection?
Considering the fragility of newborn skin, they completed the flexible design of sensor nodes through an “island-bridge” structure. At the same time, the use of highly biocompatible materials further ensures the safety of the newborn’s skin.
To prevent the sensor from influencing the newborn’s autonomous movements, they aimed to minimize the number of sensor nodes.
Through algorithm optimization and other means, they only placed sensors at five points on the newborn’s limbs and head, and built a sparse sensor network using low-power Bluetooth.
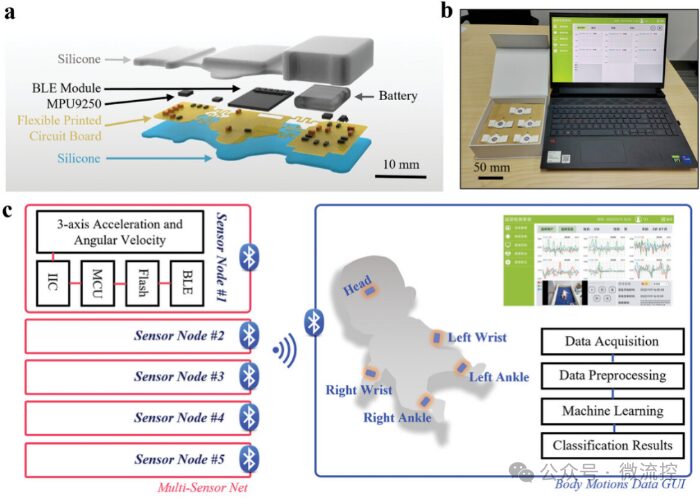
Once the system design was stable, they began clinical validation research. With the support of the First Affiliated Hospital of Jilin University, Suzhou Children’s Hospital, and Quxian County Traditional Chinese Medicine Hospital in Shanxi Province, they successfully obtained a batch of clinical data.
After preliminary data analysis, they found significant differences in time and frequency domains between children with and without risks. However, there was still no direct threshold that could be used as a dividing standard.
Specifically, they found that relying on traditional classification methods could not achieve high accuracy screening, so they began using machine learning to build classification models.
However, the overall model could not be too large, otherwise the computing power requirements would increase, making it impossible to deploy in areas with low medical standards.
Therefore, creating a lightweight and compact classification model was their goal. At the same time, they did not want the algorithm to be a “black box” algorithm.
Thus, researchers tried to extract feature values based on clinical doctors’ diagnostic standards.
At the same time, they ranked the obtained feature values according to their relevance to identify those that are more effective for classification and ensure that these feature values can be clinically interpreted.
Only in this way can they further reduce the dimensionality of the feature values to create a lightweight algorithm model.
During the model construction process, considering the need to occupy as little computational resources as possible, Dr. Bao Benkun from the team optimized the dimensionality reduction of features and algorithm redundancy to obtain a highly accurate small algorithm model.
With the help of artificial intelligence technology, they successfully developed the required classification model. Moreover, as the amount of data increases, this data processing method can also be optimized to achieve a more lightweight, faster, and more efficient classification algorithm.
Considering the application promotion in areas with low medical resources, it is essential to develop low-cost hardware systems and easy-to-deploy small automatic recognition algorithms.
Ultimately, through their efforts, the entire system’s cost is less than 500 yuan. At the same time, the research team reduced the number of feature values and adopted a logistic regression algorithm, achieving the construction of the smallest model with a 99.9% high recognition rate.
Additionally, this flexible physiological sensor network can not only be used for detecting restless movements in newborns but can also obtain more physiological information for other clinical applications.
For example, heart rate and respiratory rate can be collected through accelerometers, as well as swallowing and activity abilities, thus playing a role in ICU critical care.
On the other hand, for the rapid screening of cerebral palsy in newborns, restless movement monitoring is mainly applicable to newborns under 20 weeks.
For older infants who can crawl, they are also conducting analyses of crawling behavior and cerebral palsy to assess their neurological development capabilities.
In terms of applications, they are actively contacting maternal and child health stations in some regions of the Midwest to better promote this system.
At the same time, they are also actively exploring the possibility of constructing a battery-free sensor system.
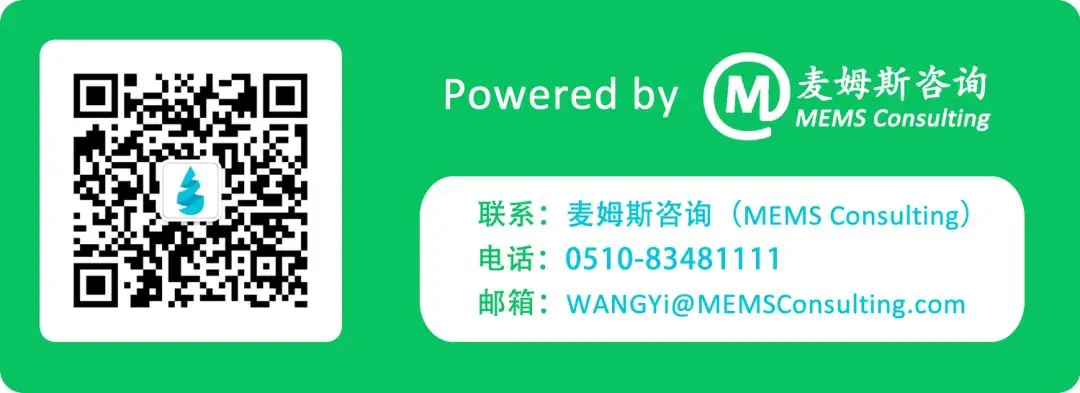