Article Overview
1. Research Background and Challenges
– Multi-sensor fusion is crucial for autonomous driving systems.
– Existing methods based on point cloud fusion suffer from semantic information loss.
– The BEVFusion framework is proposed to unify multi-modal features in the Bird’s Eye View (BEV) representation space.
2. Overview of the BEVFusion Method
– Unifies multi-modal features into a shared Bird’s Eye View (BEV) representation space.
– Optimized BEV pooling addresses the efficiency bottleneck of view transformation, reducing latency by over 40 times.
– Supports different 3D perception tasks such as 3D object detection and BEV map segmentation.
3. Efficient Camera to BEV Transformation
– Explicitly predicts the discrete depth distribution for each pixel.
– Optimizes the BEV pooling operation using pre-computation and interval reduction.
– Achieves a 40x acceleration in the camera to BEV transformation.
4. Experimental Results and Analysis
– On the nuScenes and Waymo benchmarks, BEVFusion achieves state-of-the-art performance in 3D object detection and BEV map segmentation.
– Compared to existing methods, BEVFusion reduces computational costs by 1.9 times and latency by 1.3 times.
– BEVFusion demonstrates higher robustness under different weather and lighting conditions.
5. Conclusion
– BEVFusion is an efficient and general multi-task multi-sensor 3D perception framework.
– By unifying feature representation and optimizing view transformations, it significantly improves the performance of multi-sensor fusion.
– It provides a strong and simple baseline for future research on multi-task multi-sensor fusion.

Article link: https://arxiv.org/pdf/2205.13542
Project link: https://github.com/mit-han-lab/bevfusion
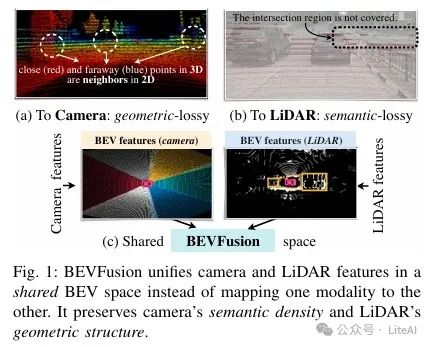
TL;DR
Article Method
1. Unified Representation:
– Bird’s Eye View (BEV) representation: Choosing BEV as the unified representation space because this view retains the geographical structure of LiDAR features while maintaining the semantic density of camera features.
– LiDAR-to-BEV projection: Flattens LiDAR point clouds along the height dimension to avoid geometric distortion.
– Camera-to-BEV projection: Projects each camera feature pixel back to rays in 3D space, generating dense BEV feature maps while preserving the complete semantic information of camera features.
2. Efficient Camera-to-BEV Transformation:
– Depth prediction: Explicitly predicts the discrete depth distribution for each pixel.
– Feature scattering: Scatters each feature pixel into D discrete points along the camera ray and readjusts related features based on depth probability.
– Optimized BEV pooling:
– Pre-computation: Pre-computes the 3D coordinates and BEV grid indices for each point, sorts and records the ranking of points, and a caching mechanism reduces grid association latency.
– Interval reduction: Parallelizes BEV grids through dedicated GPU kernels to compute intervals directly and write back results, avoiding reliance on output and partial sums written to DRAM, thus reducing feature aggregation latency.
3. Fully-Convolutional Fusion:
– Feature fusion: Fuses the transformed multi-modal features together through element-wise operations (like concatenation).
– Convolutional encoder: Applies a convolution-based BEV encoder (with residual blocks) to compensate for spatial misalignment and improve local alignment accuracy.
4. Multi-Task Heads:
– 3D object detection: Uses category-specific center heatmap heads to predict object center locations, and regression heads to estimate object size, rotation, and velocity.
– BEV map segmentation: Frames the problem as multiple binary semantic segmentations, one for each category, using focal loss to train the segmentation head.
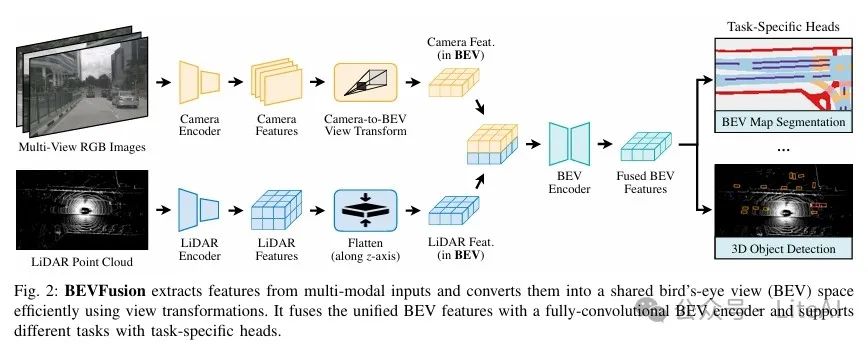
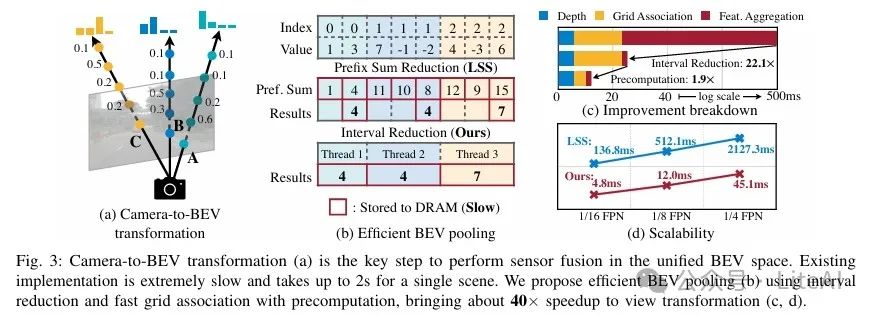
Experimental Results
1. 3D Object Detection:
– nuScenes Dataset:
– mAP and NDS: BEVFusion achieves 70.2% mAP and 72.9% NDS on the nuScenes test set, and 68.5% mAP and 71.4% NDS on the validation set.
– Computational cost: MACs are 253.2G, and latency is 119.2ms.
– Comparison: Compared to TransFusion, BEVFusion improves 1.3% on mAP and NDS on the test set while reducing MACs by 1.9 times and latency by 1.3 times.
– Waymo Open Dataset:
– mAP and mAPH: BEVFusion achieves 85.7% mAP/L1 and 84.4% mAPH/L1 on the Waymo test set.
– Comparison: Superior to existing multi-modal detectors like DeepFusion.
2. BEV Map Segmentation:
– nuScenes Dataset:
– mIoU: BEVFusion achieves an average mIoU of 62.7% on the validation set.
– Comparison: Improves by 13.6% compared to LiDAR-only models and by 6 mIoU compared to existing fusion methods.
3. Robustness:
– Under different weather and lighting conditions:
– Rainy days: BEVFusion improves mAP by 10.7% in rainy scenarios compared to LiDAR-only models.
– Night: BEVFusion improves mIoU by 12.8% in nighttime scenarios compared to LiDAR-only models.
4. Different Object Sizes and Distances:
– Small and distant objects: BEVFusion shows significant improvements over LiDAR-only models for small and distant objects.
5. Sparse LiDARs:
– 16-beam LiDAR: BEVFusion outperforms MVP, reducing MACs by 1.6 times.
– 4-beam LiDAR: BEVFusion outperforms MVP, reducing MACs by 1.6 times.
– 1-beam LiDAR: BEVFusion outperforms MVP, reducing MACs by 1.6 times and improving NDS by 12%.
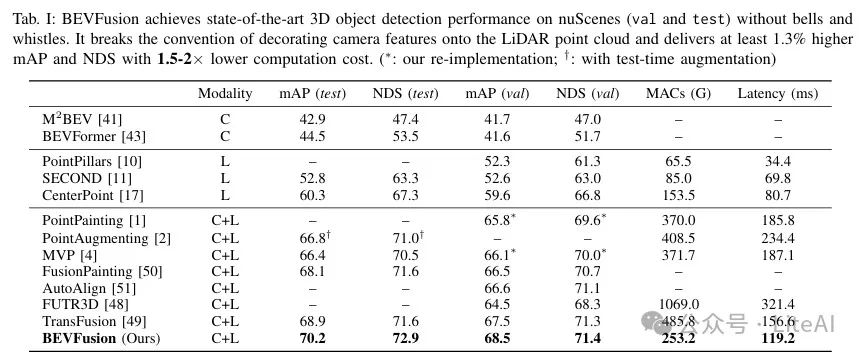
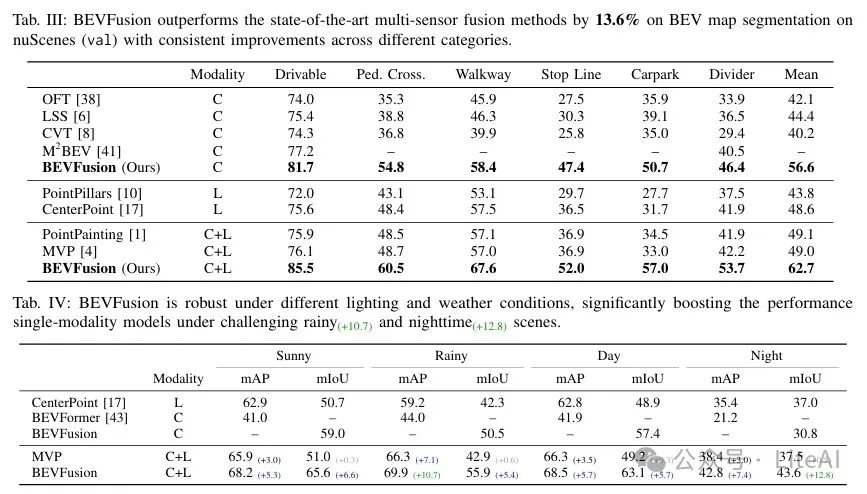
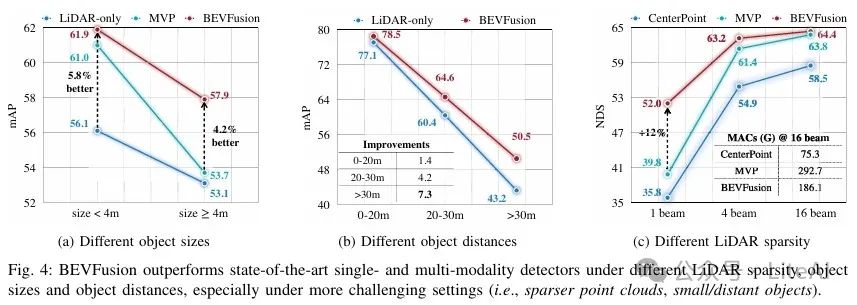
Final Thoughts
Scan to add me, or add WeChat (ID: LiteAI01), for technical, career, and professional planning discussions. Please note “Research Direction + School/Region + Name”
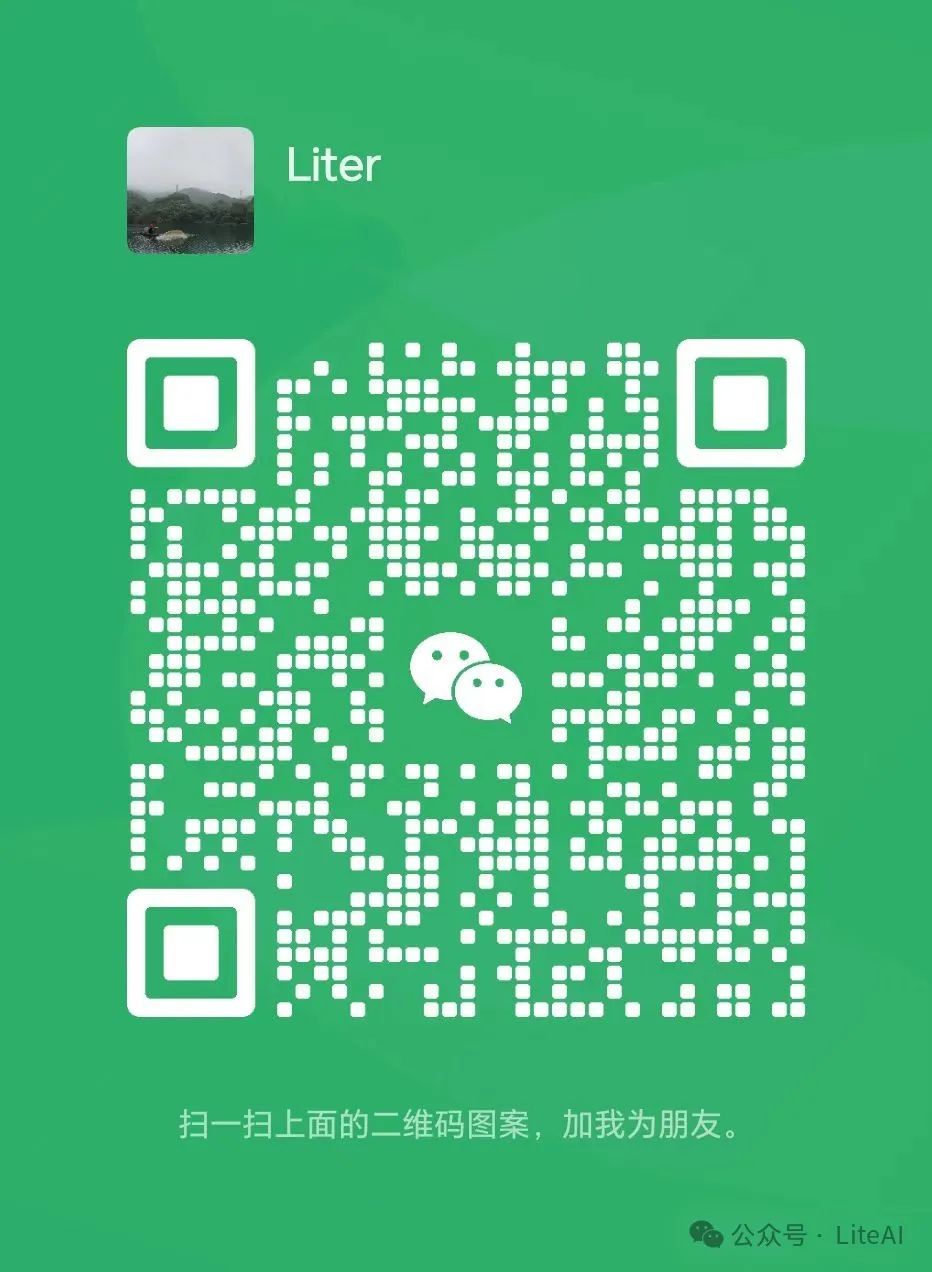