Original: Dear Data
(1)
If we say that developing applications for large models is like gold mining,
then the frameworks, tools, and platforms that accelerate application development are the “shovels for mining gold.”
Where there are gold miners, there will always be shovel manufacturers.
Opportunities will definitely be seized by leading vendors.
And open-source products always take the lead.
LangChain is an open-source framework for large model applications.
In March 2023, LangChain integrated with systems such as Amazon Cloud, Google Cloud, and Microsoft Azure.
Of course, the difficulty of developing large model applications depends on the developer’s level.
Some developers sigh: “LangChain is truly delightful.”
While some novice developers complain: “I struggled with LangChain for several hours just to use its two functions.”
LangChain can enhance the efficiency of developing applications for large language models,
providing tools and modules for building applications.
For example, suppose there is a mobile APP called “Green or Not.”
When enhancing the “Green or Not” APP with a large model,
LangChain would participate in a Pipeline (the process of executing tasks):
First, the “Green or Not” APP sends the question to the large model.
Second, the large model understands and answers the question.
Third, the answer from the large model is sent back to the “Green or Not” APP.
In this Pipeline, aside from the work of the large model, the remaining tasks can be handled by LangChain.
LangChain is a software framework that facilitates the development of various native applications for large models.
Those who have played with large models know that the out-of-the-box approach is to input questions in that window.
Only being able to ask and answer one question at a time is too limiting.
People quickly began to think about how to turn the large model into a super component and combine it with other elements to play.
However, sometimes there can be minor troubles.
Deploying in large enterprises can be even more troublesome, involving many APIs and other issues (authentication, traffic control, permission verification).
This is when large model plugins are needed.
Let me give another example:
An AI Agent powered by a large model consists of three parts.
First, signal collection,
Second, processing end,
Third, execution end.
First, let’s talk about where the large model is.
The large model is part of the second processing end.
At this point, the large model is part of the Agent, while the engineering connection work exists in the first and third parts. This work can be automated and delegated to the large model plugin.
In other words, the needs that combine signal collection and execution can all be delegated to the large model plugin.
Aside from the large model doing its job well, the remaining tasks are handled by the large model plugin.
The plugin receives external requests, the large language model generates answers, the plugin converts the answers into responses, and returns the responses to the API. API is a means of interaction between software systems. API defines how software systems call each other and provides a standard interface, automatically generating an API for the large language model service.
This example of an AI Agent at work is not complex; the plugin certainly acts as a convenient tool.
The conclusion is that to realize rich functionalities, large models must call on “plugins.”
Plugins are a broad concept, with many types and usage methods.
Plugins can be tools that automatically call APIs,
or they can be a RAG framework that only implements a Q&A function.
AI Agents themselves can be plugins, and AI Agents can also call another AI Agent as a plugin.
In cases with multiple AI Agents, one AI Agent acts as a plugin for other AI Agents.
Teacher Tan quickly says, no Russian nesting doll jokes, please.
Thus, the business volume of large model “plugins” can be quite considerable.
(2)
So, what are the differences between early AI Agents and AI Agents powered by large models?
In the early days of reinforcement learning, there were many examples of Agents:
In robot games, Agents are the robots themselves.
Agent‘s goal: win the game.
Agent‘s state: the current state of the game.
Agent‘s action: the next operation in the game.
Actions can be moving left, moving right, or jumping.
First, the Agent perceives the state of the environment, such as the robot’s current position.
Next, based on the perceived state, the Agent (robot) uses a policy function to select an action.
The policy function is a mapping from state to action,
which can be deterministic or probabilistic.
Then, the Agent executes the selected action, changing the state of the environment.
Finally, the Agent receives rewards from the environment.
In the game, robots either score zero for dying or score points for winning.
Early AI Agents could only perceive limited environmental information and could only automate a limited number of predefined responses,
their adaptability was not opened up.
In this context, Teacher Tan summarizes: at that time, AI Agents had a limited perspective.
Agents powered by large models can plan autonomous behaviors for open environments.
Moreover, within the scope they can operate and control, they utilize as many tools as possible and respond to information from this open environment.
Completing this process requires four basic capabilities of the Agent: understanding, generating, logic, and memory.
These represent the AI Agent‘s perception of the external world, processing of information, decision-making, and accumulation of experience.
Suppose there is an AI Agent helping a user find all the rumors about a celebrity and summarize them into a storyline.
The AI Agent first needs to understand the user’s needs, which requires understanding ability.
Then, the AI Agent needs to search the internet and understand what happened.
Next, it needs to generate a factual storyline with cause and effect, which requires generating, logic, and memory abilities (historical news).
Throughout this process, all four capabilities play an important role.
It seems that the premise of opening up the perspective still relies on the strong capabilities of large models.
Of course, the help of large model plugins is also indispensable.
(3)
Since the scope of large model plugins is so broad, with a variety of software frameworks,
finding commonalities among them and forming a platform could make things more convenient.
I mentioned earlier that this opportunity to enhance development efficiency and accelerate the development of native applications for large models
will undoubtedly be seized by leading vendors.
I see that Baidu is doing just that; on December 20, 2023, Baidu Intelligent Cloud’s Qianfan AppBuilder officially opened.
Earlier, on October 17, 2023, at the Baidu World Conference,
a demonstration of “developing a customer service for Sany Heavy Industry in three minutes” also “revealed” progress in this direction.
Moreover, I believe that Baidu’s AppBuilder not only designed the product of the “large model plugin platform” but also an ecosystem.
First, product ecosystem.
Li Yanhong said that only by having millions of AI applications can large models be considered successful.
Baidu aims for a million-level application ecosystem, and there must be good tools to support application development on the base.
Baidu’s launch of such products also aligns with Li Yanhong’s frequent statement that “the value of AI lies in native applications.”
Second, technology ecosystem.
Baidu’s focus on millions of developers and openers can drive the usage of Baidu’s large models.
Third, industry ecosystem.
Developing an industry ecosystem requires lowering development thresholds, promoting collaborative innovation, and accelerating implementation, all of which large model plugins can assist with.
In addition to the above three points, one more thing is to boost orders from B end enterprise clients.
Currently, many B end enterprises are eager about large model technology but struggle to know how to use it.
At present, Baidu’s AppBuilder has three types (supporting knowledge-enhanced application framework RAG, Agent framework with thinking chain and tool usage capabilities, generative data analysis framework GBI), all reflecting strong customer demand.
Customers can quickly get started with AppBuilder, after which whether imitating or inspiring, it helps
to find more uses for large models and serves as a good sales entry point.
With these thoughts in mind, in the last few days of 2023, I met Dr. Sun Ke, chairman of the Baidu Intelligent Cloud Technical Committee, at Baidu Tower. As he was last seen, he still wore a dark hoodie.I wanted to delve into the topic, and he was very candid.
Sun Ke: If technology really develops to a state where multiple AI Agents exist, it is possible for one AI Agent to always be picked up by another AI Agent and used as a plugin. In this state, you can think of my components as potentially being picked up by a larger AI Agent to be used as its plugin. This is one of the developmental directions we foresee for AI Agents, and everyone is currently exploring and developing.
Tan Jing: With the enhancement of large model capabilities, will the Agent architecture be eliminated?
Sun Ke: Some strategies will definitely become obsolete. However, as an important tool for developing native applications for large language models, the Agent architecture can keep pace with trends, adjust strategies, and aim to make it easier for people to use.
Tan Jing: Will the Agent architecture disappear one day?
Sun Ke: One day, if large models expand to fill the entire AI Agent, it will still ultimately be an AI Agent that exhibits these behaviors (signal collection, processing, execution).
Sun Ke: The Qianfan large model platform is at the PaaS layer of Baidu Cloud. Essentially, you can think of it as an A (application) PaaS that grows on top of the Qianfan large model platform, which we internally code-named APaaS.
AppBuilder is coupled with the Qianfan large model platform. The coupling point lies in billing logic, underlying computing resources, and large model calls. Of course, it includes the interfaces of the series of capability engines PaaS we previously launched, so you can think of the previous AI PaaS layer as being more focused on the underlying PaaS.
Tan Jing: What is the relationship with the previous MLOPs?
Sun Ke: If you are familiar with the previous MLOPs layer, I think it is built on that foundation and can also be said to add a layer between large model applications and large models.
Tan Jing: What were the representative products of the previous generation A-PaaS on public clouds?
Sun Ke: Previously, due to model performance limitations, we focused on PaaS for model training and deployment. Components of this kind of form are not easily integrated into real software engineering systems.
Tan Jing: Compared to the old, how can we summarize this change in one sentence?
Sun Ke: We have encapsulated commonly used capabilities of large models into APIs.
Tan Jing: Can you talk about the timeline for the emergence of AppBuilder?
Sun Ke: Earlier, our team was already very familiar with various frameworks like RAG, and later found the possibility of generalizing this framework.
So, we decided to implement this, and after realizing it, we found it to be a very general framework, and we have continued to pursue this until the current form of AppBuilder.
(End)
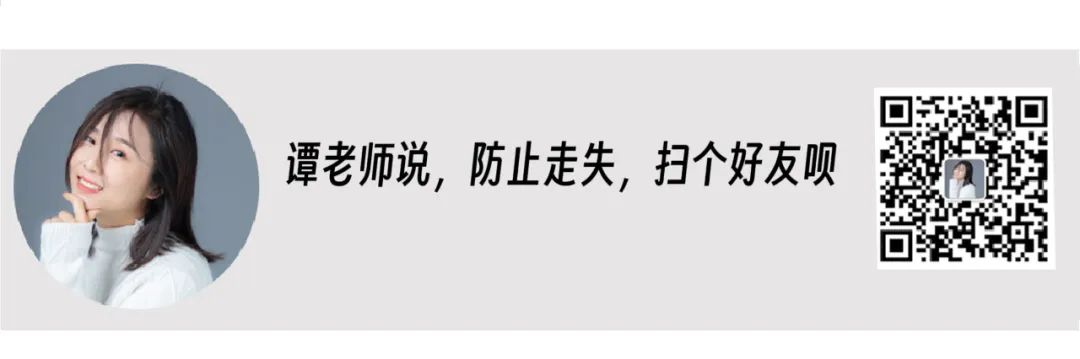
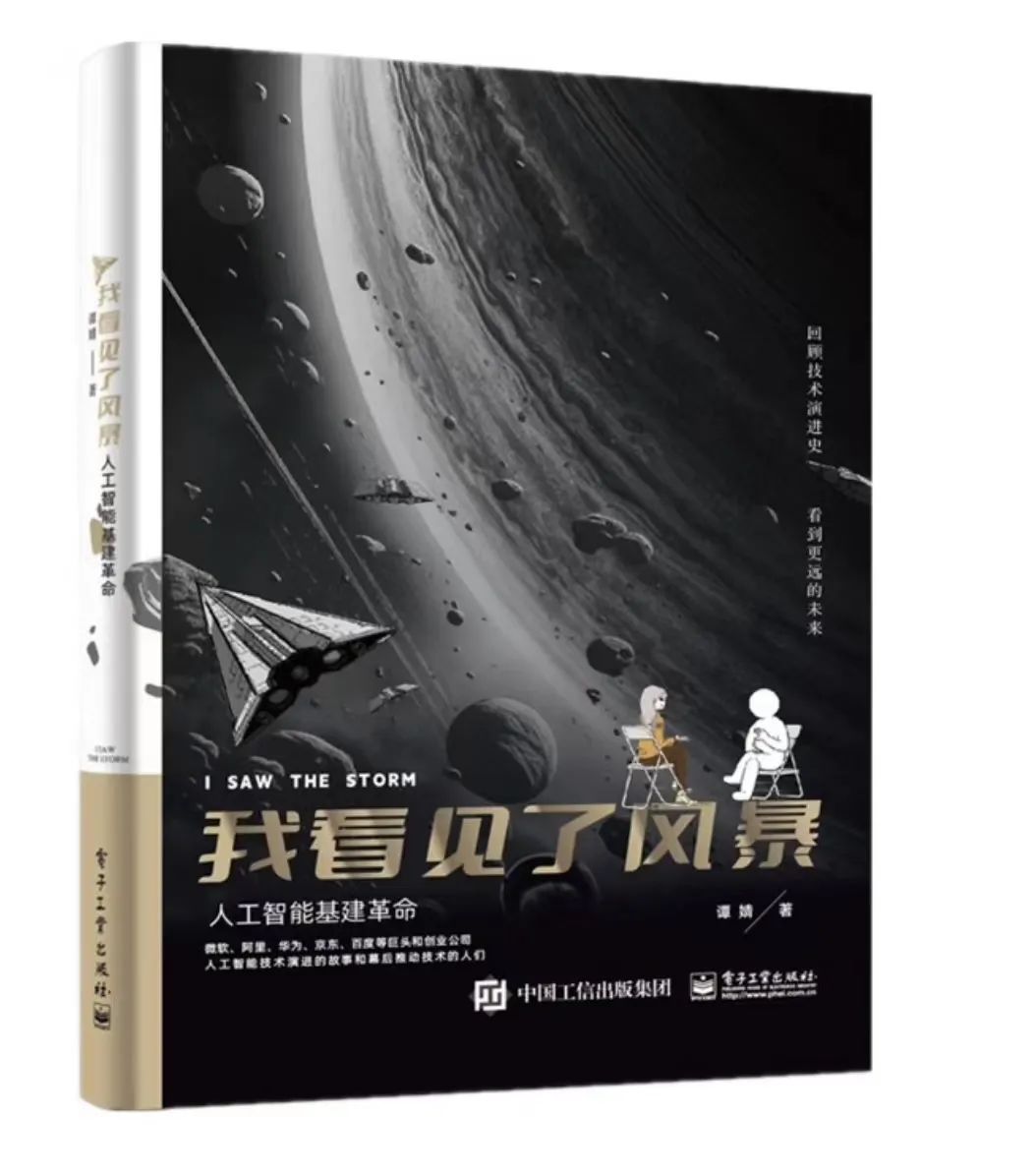
AI Large Models and ChatGPT Series:
1. With ChatGPT’s popularity, how to establish an AIGC company and make money?
2. ChatGPT: Never bully liberal arts students
3. How does ChatGPT’s learning ability come from?
4. Exclusive | From the great Alex Smola and Li Mu’s departure from AWS to start a business successfully, reflecting on the evolution of “underlying weapons” during the ChatGPT large model era
5. Exclusive | Former Meituan co-founder Wang Huiwen is “acquiring” the domestic AI framework OneFlow, aiming to add a new player from light-years away
6. Can large models used for criminal investigation only be fictional stories?
7. The power game of the “cloud economy” of large models
8. CloudWalk Technology’s calm large model: What is the relationship between large models and AI platforms? Why create industry large models?
9. Deep discussion with Fourth Paradigm’s Chen Yuqiang | How to open up the trillion-scale traditional software market with AI large models?
10. Deep discussion with JD Technology’s He Xiaodong | A “departure” nine years ago: laying the foundation for multimodality, competing for large models
11. Old stores welcoming new customers: selection and betting on vector databases, things no one tells you
12. Fine-tuning is delightful; a comic technology blogger is actually using domestic large models to generate a series of comic heroines
13. Large models “stirring the pot”: which will be eliminated first in the selection of data lakes and data warehouses?
14. What are the difficulties of using large models for Tencent advertising?
15. How to squeeze every bit of computing power from large models?
Long Articles
1. Deep discussion with iFlytek’s Liu Cong | If you don’t grasp large model algorithms, missing one thing can cost you three months
2. Deep discussion with Zhang Jiajun from Wuhan Artificial Intelligence Research Institute | What papers are worth reading behind the “Zidong Taichu” large model?
3. Deep discussion with Wang Jinqiao from Wuhan Artificial Intelligence Research Institute | How many high-quality papers are needed to create a domestic large model?
4. Why focus on prompt engineering?
5. How much can your salary increase if you switch to work on domestic large models?
6. After AI roared, how could a Data company with a valuation of $43 billion emerge?
7. Seizing large models, seizing public cloud orders, what “yangmou” did Databricks and Snowflake use?
8. The next battle of large models, why is it AI Agents?
9. If your large model is still a fool, you don’t have to worry about AI safety like Ilya does
10. Instruction data: the “invisible support” for training large models
11. What is the correct posture for open-sourcing large models?
Comic Series
1. Is it joy or sorrow? AI actually helps us finish the Office work
2. AI algorithms are brothers; AI operations are not brothers?
3. How did big data get social anxiety?
4. Is AI for Science really “scientific”?
5. Want to help mathematicians, how much does AI count?
6. Those cheering for Wang Xinling are actually the magical intelligent lake and warehouse
7. It turns out that knowledge graphs are the cash cows for “finding relationships”?
8. Why can graph computing directly challenge the black industry?
9. AutoML: Should I buy a “tuning robot”?
10. AutoML: The hotpot base you love is automatically restocked by robots
11. Reinforcement learning: How many steps can AI chess players see when they move?
12. Time series databases: So close, yet so far from the high-end manufacturing sector
13. Active learning: Has AI been PUA’d?
14. Cloud computing Serverless: A single arrow through the clouds, a thousand troops meet
15. Data center networks: Data reaches the battlefield in 5 nanoseconds
16. Data center networks: Being late is not scary; what’s scary is that others are not late
17. Comic: What are the difficulties of using large models for Tencent advertising?
AI Framework Series:
1. The people working on deep learning frameworks are either madmen or frauds (1)
2. The people working on AI frameworks | The blazing fire, Jia Yangqing (2)
3. The people working on AI frameworks (3): The fervent AlphaFold and the silent Chinese scientists
4. The people working on AI frameworks (4): The prehistory of AI frameworks, stories of big data systems
Note: (3) and (4) are only included in “I See the Storm.”