Founder and CEO of Horizon Robotics Yu Kai
From Center to Edge
In the past, big data, big models, and big computing have driven academic research and industrial development. All computing, from PCs to mobile devices, has shown a trend of aggregation towards cloud computing and data centers. However, I believe that in the next ten to twenty years, much of the computing will shift to the edge of the internet, to the device side, and even in situations where there is no internet connection or wireless connection, relying on battery or local power support for local computing. The “Embedded AI” we proposed earlier embodies this concept, achieving real-time environmental perception, human-machine interaction, and decision-making control without an internet connection.
Now, we are witnessing a revolution starting from the edge, similar to the revolution triggered by deep learning since 2012. In April 2012, I gave a lecture on deep learning at a conference in Xi’an, where deep learning was still a marginal topic, but has now become the center of a storm. Most of today’s AI computations are in the data centers of BAT (the three giants of China’s internet: Baidu, Alibaba, Tencent), but we will find that there is a huge opportunity away from data centers, at the edge of the internet.
The Three Major Trends of the Future
I believe there are three major trends in the future. The first trend, which I call the “New Moore’s Law.” Moore’s Law has pushed the global tech community forward over the past two or three decades. Moore’s Law states that the cost of computers halves and performance doubles every 18 to 24 months. According to this rule, by 2045, the computing resources that can be purchased for $1000 will be almost equivalent to the computing power of the human brain, which is currently the computing power of the Tianhe-2 supercomputer, but the Tianhe-2 consumes 1000 watts, while the human brain only consumes 20 watts.
Will this be achieved by 2045? Intel officially announced the end of Moore’s Law last year. The entire Moore’s Law is developing along another track, no longer focusing on the number of transistors per unit area, but rather on architectural improvements that evolve computing from logical operations to AI operations. So will AI operations enhance CPU technology or design a new architecture? That is the question.
We find that if we make a general-purpose CPU, it may be very flexible because it can perform many tasks, but the overall efficiency is not high. However, if we optimize specifically for each task, we can increase efficiency by two to three orders of magnitude.
The second major trend is the shift from center to edge. The demand for AI is increasing from PC internet to mobile internet and then to smart IoT. The PC internet does not have high requirements for AI, but this demand appears in the mobile internet. For example, the news on Toutiao is quite different from Baidu; Baidu is “search and get it immediately,” while Toutiao is “get it without searching.” This is because on mobile devices, Toutiao implements a personalized recommendation mechanism for articles, making the acquisition of human-machine interaction information more intelligent and efficient. In the future, there will be an increasing demand for AI on mobile devices, and much computing will shift from data centers to mobile devices for perception, human-machine interaction, and decision-making.
These computations need to be real-time, low power consumption, and low cost. For example, in a situation where a child suddenly crosses the road in front of a car, it is unimaginable to upload data to the data center, process it, and then send it back. Therefore, local computing and real-time processing without delay are essential. For instance, surveillance cameras in the future will all have dedicated processors to handle real-time video, which is a major trend. Sun Zhengyi, chairman and CEO of SoftBank Group, believes that many computations will occur at the edge, and the computing on edge devices will turn these devices into robots. In 15 years, the number of robots will exceed that of humans, reaching 10 billion.
The third major trend is the competition at the edge. For example, every car currently has a reversing camera, but future autonomous vehicles will have 8 to 12 cameras, with resolutions covering 720P, 1080P, 4K, and other levels. Moreover, in the next 10 to 20 years, the development of autonomous driving technology will increase the average speed of cars on highways from 100 kilometers per hour to nearly its physical limit—200 kilometers per hour. As speed increases, the amount of data processed will continue to grow. Thus, computing at the edge will constantly pursue faster, higher, and stronger capabilities, marking a new competition.
Bringing AI to the Device Side
In the next 5 to 10 years, what will be the most disruptive industrial opportunities? Typically, industrial opportunities are divided into two stages: first, a wave of 2B (business-to-business) opportunities, where these companies themselves develop technology and provide the “guns and ammunition.” The second wave are 2C (business-to-consumer) companies. 2B companies serve 2C companies, which then capture user opportunities. This model has indeed repeatedly occurred in history. For instance, in the late 1990s when the PC internet emerged, the primary task in this phase was to build architecture and networks, so companies like Cisco performed exceptionally well. This phase overall belonged to the “gun and ammunition” manufacturing stage for the B-end, followed by the emergence of large internet companies for the 2C market, such as Google.
When we entered the market, the United States had already matured its foundational technologies. From 2000 until today, we have been enjoying the opportunities brought by the technological growth of the 1980s and 1990s, which then led to user-oriented enterprises. However, in the current entrepreneurial environment, the cost of innovation for 2C companies has become extremely high, such as Didi raising over a hundred billion dollars, and Meituan also raising tens of billions. Meanwhile, Google only raised twenty million dollars before going public, and Baidu and Tencent were similar, at that time there were numerous opportunities to capture. But now, the capabilities brought about by the technological innovations of the 1980s and 1990s have almost been fully explored, leading to increasingly heavy innovation burdens.
So where are the future opportunities? We find that during the overall downturn of the high-tech market, one company—NVIDIA—has seen its stock price rise fivefold in the past year, making it the fastest-growing company in the high-tech market. The core logic behind this is simple: “manufacturing guns and ammunition.” We also value this aspect very much, thus we are committed to developing processors for deep neural networks, providing integrated hardware and software solutions.
From a more macro perspective, among the top 20 high-tech companies by market capitalization this year, 8 are hard-tech companies, with the two fastest-growing being NVIDIA and AMD. We develop processors for deep learning because we see this trend, that the combination of software algorithms, AI, and semiconductors will spur explosive growth. Our focus is on accelerating inference efficiency, creating low-power, high-performance solutions to bring AI to the device side.
We are not a traditional hardware manufacturer; we are actually a soft-hard integrated 2B company. We first optimize from a system perspective, designing a dedicated process for software algorithm experiments, then designing hardware architecture based on this software algorithm, and finally using hardware architecture to optimize software. Through soft-hard optimization, we can enhance performance by two to three orders of magnitude.
Why do I believe system optimization is important? Take autonomous vehicles as an example. To this day, whether it is Baidu or Google’s self-driving cars, they need to stop for cooling every two hours. Why is the heat dissipation problem difficult to solve? Why can’t power consumption be reduced? It is precisely because there has been no optimization at the system level. Software frameworks continue to iterate, and hardware architectures also need to iterate accordingly, so that perceptual capabilities can be greatly enhanced. Our goal is to create a chip that consumes only a fraction of the power and cost of the top processors on the market but performs two to three times better.
Steve Jobs removed keyboards and mouse controls from phones and tablets; we aim to remove all remote control tools in five years, achieving complete human perception. To achieve this goal, we need to completely reconstruct software and hardware, ensuring that the power consumption and size of the processors and entire systems are low enough. We are not only focused on software algorithms but are more concentrated on three vertical markets: autonomous driving, smart homes, and public safety, which will experience explosive growth in the coming years.
Finally, AI changes the world, but what truly changes AI and the world is talent. The most lacking resource in AI today is talent. I encourage young people to intern and work at Horizon. I believe that what Horizon is doing is at the forefront of the times, providing young people with exciting growth and learning opportunities. We are not interested in incremental innovations; what we want to do is disruptive innovation.
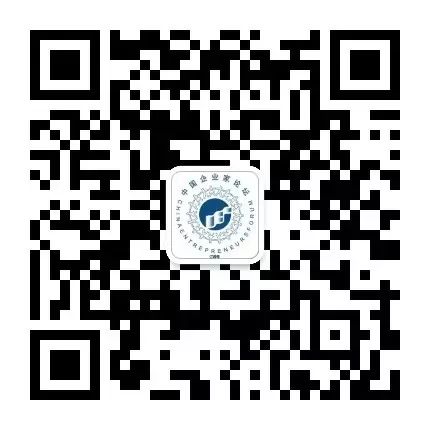
Business Cooperation
Please Contact
”Contact Number: 8610-66426315
Email: [email protected]
Mobile and WeChat: 15010075732