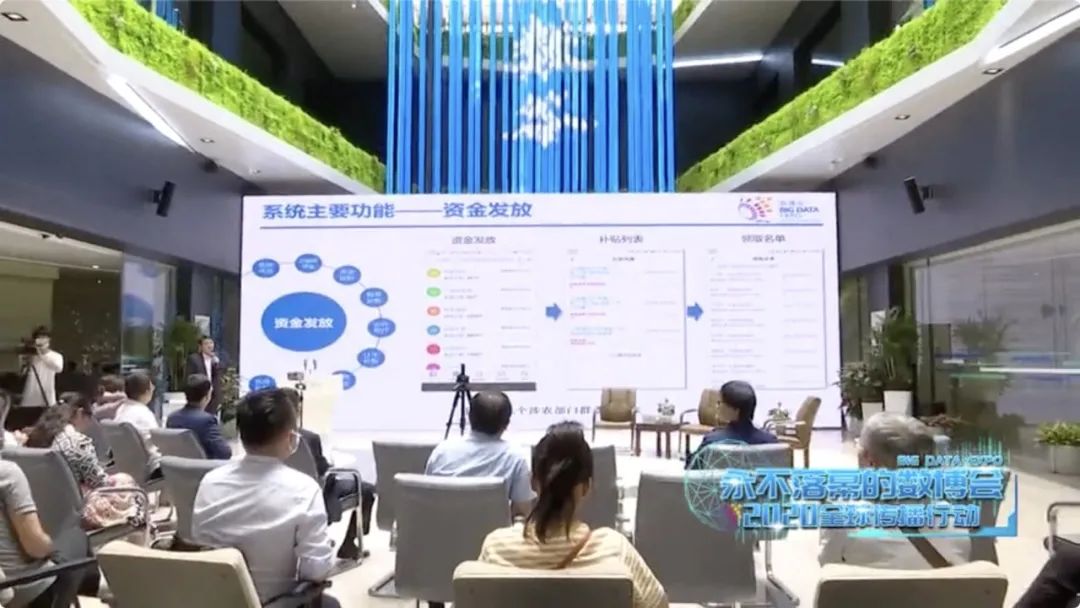
2021 Smart Transformation: New Innovations
Artificial Intelligence
2021/7/26

Real-Time Computing Without Internet Connection


Reducing Massive Calculations to Small Terminals

END
Source: Science and Technology Daily
Reporter: Ma Aiping
Editor: Lin Xianxian
Related activities of the Digital Expo
Vice Premier Liu He attended the 2021 Digital Expo online “News Broadcast” focuses on the 2021 Digital Expo “Focus Interview” focuses on the 2021 Digital Expo The 2021 Digital Expo concluded successfully “Digital Salon” enters Guizhou Renhe Data on April 9 “Digital Salon” enters AIRLOOK on April 15
“Digital Salon” enters Xiangshu Technology on April 23
“Digital Salon” enters Guizhou Haohuo on April 27
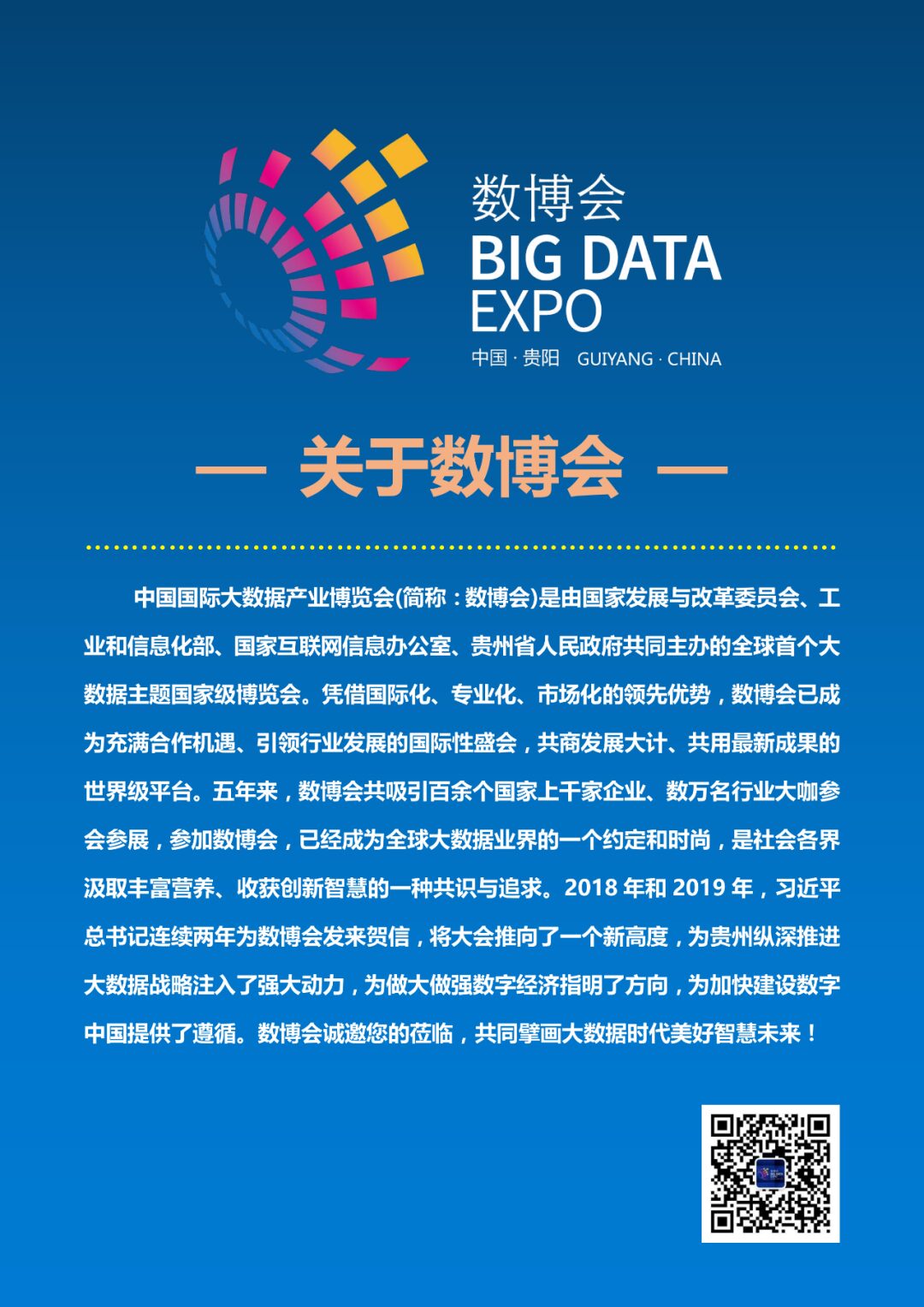