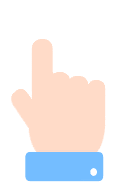
Click the blue text above to follow me
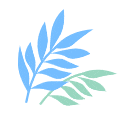



Breakthroughs in Technical Solutions
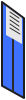
In traditional cognition, deep learning models require powerful computing support. However, in recent project practices, I found that TinyML is changing everything. Through model compression and quantization techniques, real-time inference has been successfully achieved on STM32H7 series MCUs.
After repeated testing, we finally adopted the TensorFlow Lite Micro framework and optimized the model through the following steps:
// 1. Select appropriate quantization parameterstypedef struct { int8_t* data; // Quantized weight data float scale; // Quantization scale int32_t zero_point; // Zero point offset} QuantParams; // 2. Implement quantized matrix multiplicationvoid quantized_matmul(const int8_t* input, const int8_t* weights, const QuantParams* params, int32_t* output, const int rows, const int cols) { for (int i = 0; i < rows; i++) { int32_t acc = 0; for (int j = 0; j < cols; j++) { // Use SIMD instructions for acceleration acc += (int32_t)input[j] * weights[i * cols + j]; } // Dequantize back output[i] = (acc * params->scale) + params->zero_point; }}
The key points in this optimization process are:
-
Using symmetric quantization to reduce computational complexity
-
Utilizing CMSIS-DSP’s SIMD instruction acceleration
-
Assembly optimization targeting performance hotspots
In memory management, we implemented an efficient circular buffer:
typedef struct { float* buffer; // Data buffer uint32_t size; // Buffer size uint32_t head; // Write position uint32_t tail; // Read position uint32_t count; // Current data amount} CircularBuffer; // Thread-safe data writingbool buffer_write(CircularBuffer* cb, float data) { __disable_irq(); // Enter critical section if (cb->count == cb->size) { __enable_irq(); return false; } cb->buffer[cb->head] = data; cb->head = (cb->head + 1) % cb->size; cb->count++; __enable_irq(); // Exit critical section return true;}
Through this design:
-
Achieved a lock-free producer-consumer model
-
Avoided memory fragmentation
-
Optimized data access efficiency
To ensure the real-time nature of inference tasks, we implemented priority scheduling based on FreeRTOS:
// Task priority definitions#define SAMPLING_TASK_PRIORITY (tskIDLE_PRIORITY + 3)#define INFERENCE_TASK_PRIORITY (tskIDLE_PRIORITY + 2)#define MONITOR_TASK_PRIORITY (tskIDLE_PRIORITY + 1) // Create sampling taskxTaskCreate( sampling_task, "Sampling", SAMPLING_STACK_SIZE, NULL, SAMPLING_TASK_PRIORITY, &sampling_task_handle);
The key scheduling strategies are:
-
Sensor sampling task has the highest priority
-
Inference task has medium priority
-
Monitoring task has the lowest priority
Experience Summary
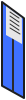
Several important engineering experiences:
-
Algorithm Optimization:
-
Matrix operations must use SIMD acceleration
-
Avoid dynamic memory allocation
-
Properly use DMA to reduce CPU load
System Design:
-
Use a layered architecture for easier functional expansion
-
Implement a watchdog mechanism to ensure system reliability
-
Add a logging system for easier problem location
Thoughts on Future Development
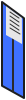
Thoughts on Future Development
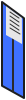
I believe that the development of embedded AI will show the following trends:
-
Heterogeneous computing will become mainstream
-
The combination of RISC-V and AI accelerators will become more common
-
Reconfigurable computing architectures will be more widely used
Compiler optimization is crucial
-
Automated model compression and quantization techniques
-
Code auto-optimization for specific hardware
Development toolchains will become smarter
-
Automated performance analysis and optimization suggestions
-
Integrated debugging and performance monitoring tools
Learning Suggestions
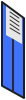
If you also want to develop in this direction, I suggest:
-
Technical Preparation:
-
Deeply study the ARM CMSIS library, especially the DSP section
-
Master the task scheduling mechanism of FreeRTOS
-
Understand the working principle of TensorFlow Lite Micro
Practical Improvement:
-
Start with simple microcontroller projects
-
Gradually try to deploy small neural networks
-
Focus on performance optimization and power control
Finally, I want to share a saying I often tell my team: Embedded AI is not just about moving the model to the MCU, but about making the best system design based on understanding business needs.
True technological innovation is not simply piling up functions, but achieving extreme performance optimization in resource-constrained environments.
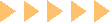
“Previous Recommendations”
Future of Embedded Technology: Connectivity, Intelligence, and Innovation
Embedded Development: From Circuit Boards to Unlimited Creativity
FPGA Development: Exploring the Infinite Possibilities of Embedded Systems