

Click the blue text above to follow us

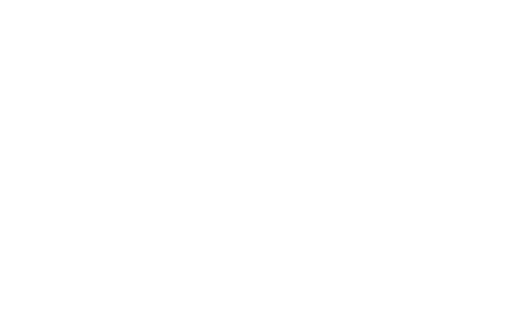

Gift for readers
👨💻 Doing research involves a profound system of thought, requiring researchers to be logical and meticulous, but effort alone is not enough; often leveraging resources is more crucial. One must also have innovative points and inspirations. When a philosophy teacher asks you what science is or what electricity is, do not find these questions amusing. Philosophy is the mother of science, it pursues ultimate questions and seeks those self-evident questions that only children ask but you cannot answer. It is suggested that readers browse in order to avoid suddenly falling into a dark maze without finding their way back. This may not reveal all the answers to your questions, but if it can raise clouds of doubt in your heart, it might create a beautiful sunset scene. If it brings you a storm in your spiritual world, take the opportunity to dust off the ‘lying flat’ mindset that has been resting there.
Perhaps, after the rain, the sky will be clearer…🔎🔎🔎
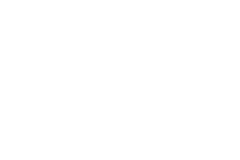
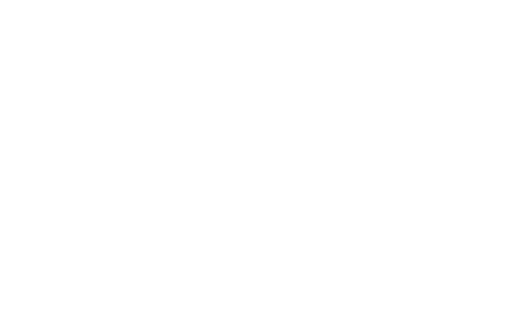

1 Overview
1. Introduction
With the acceleration of urbanization and the widespread application of distributed energy, the power system faces unprecedented challenges. Especially in the case of large-scale distributed photovoltaic grid connection, the coordinated autonomy of the power system becomes particularly important. Therefore, this study aims to optimize the power line planning strategy among multiple buildings through clustering methods to improve the efficiency and stability of the power system.
2. Research Background
-
Development of Distributed Energy: With the continuous advancement of renewable energy technology, the proportion of distributed energy (such as solar and wind energy) in the power system is gradually increasing. These distributed energies are intermittent and uncertain, bringing new challenges to the scheduling and control of the power system.
-
Complexity of Building Spatial Layout: The spatial layout of buildings in urban areas is complex and diverse, with different electrical distances, load characteristics, and photovoltaic generation capacities among buildings. These differences make the clustering planning of the power system more complicated.
3. Research Method
-
Modularity Index Based on Electrical Distance: Modularity is an important indicator for measuring the strength of network structure. In the distribution network, the edge weight of modularity is represented by electrical distance. Therefore, this study uses a modularity index based on electrical distance to measure the structural strength of clustering. By calculating the electrical distance between nodes within different clusters, the rationality of clustering can be assessed.
-
Objective Function: The objective function consists of three parts: line laying cost, cluster purchasing cost, and modularity. By optimizing the objective function, the best clustering scheme can be found. Among them, the line laying cost reflects the construction cost of power lines between buildings; the cluster purchasing cost reflects the ability to reduce purchasing costs from the external network through energy complementarity within the cluster; and modularity is used to measure the structural strength of clustering.
-
Solution Method: This study uses particle swarm optimization for solving. Particle swarm optimization is an optimization algorithm based on swarm intelligence, with advantages such as fast convergence speed and strong global search capability. By continuously iterating and updating the position and speed of particles, the optimal clustering scheme can be found.
4. Research Case
Taking 20 randomly generated buildings as an example, the number of buildings, load, and photovoltaic data can all be adjusted in applications. Through modular programming and clear comments, the research is facilitated. In the running results, the variations of line laying cost, cluster purchasing cost, and modularity under different clustering schemes can be observed.
5. Research Results and Discussion
-
Effect of Clustering: Through reasonable clustering, significant reductions in line laying costs and cluster purchasing costs can be achieved. At the same time, the electrical distances between nodes within the cluster are relatively close, and the modularity is high, indicating that the clustering has strong structural integrity.
-
Analysis of Influencing Factors: The load characteristics and photovoltaic generation capacity of buildings have significant impacts on the clustering effect. Buildings with similar load characteristics are more likely to be clustered together, while buildings with strong photovoltaic generation capacity can reduce cluster purchasing costs through energy complementarity.
-
Optimization Suggestions: To further enhance the clustering effect, more constraints and optimization objectives can be considered. For example, geographical location, electricity price differences, and environmental factors can be considered as constraints; simultaneously, system stability, reliability, and economic efficiency can also be integrated as optimization objectives.
6. Conclusion and Outlook
This study optimized the power line planning strategy among multiple buildings through clustering methods. By comprehensively applying the modularity index based on electrical distance, the objective function, and particle swarm optimization, reasonable clustering solutions were obtained. The research results indicate that reasonable clustering can significantly reduce line laying costs and cluster purchasing costs, improving the efficiency and stability of the power system. Future research may further consider introducing more constraints and optimization objectives to enhance the effectiveness and practicality of clustering.
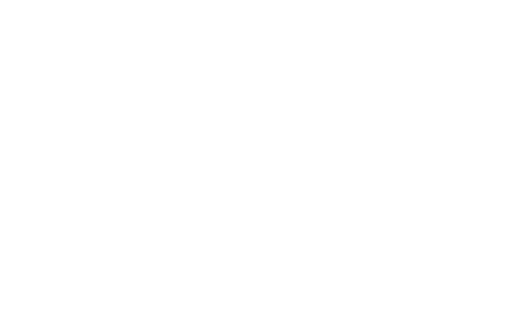

2Running Results
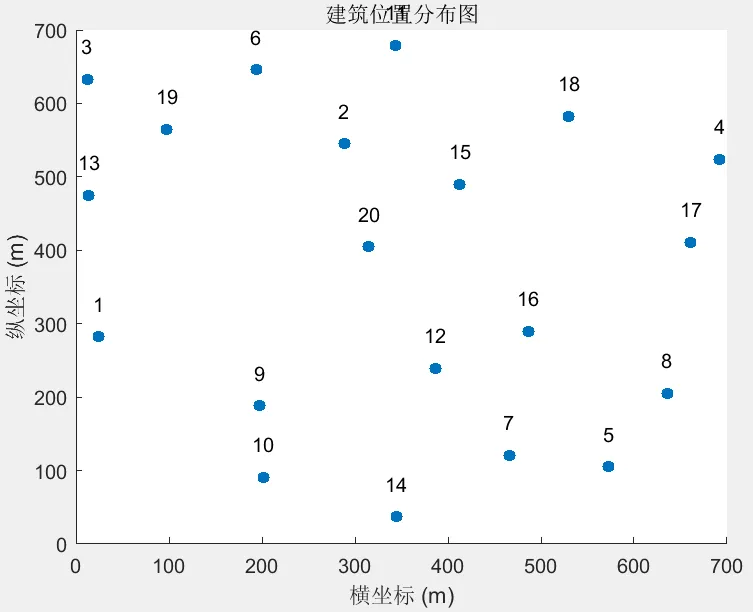
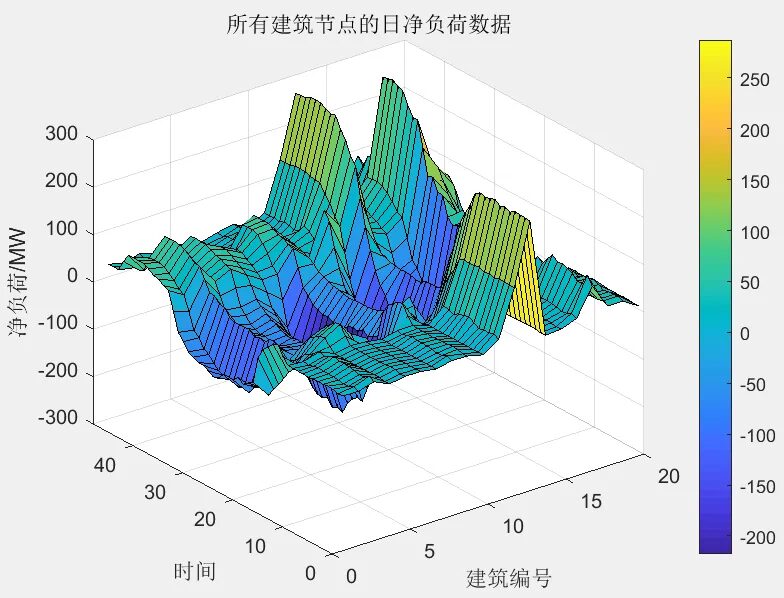
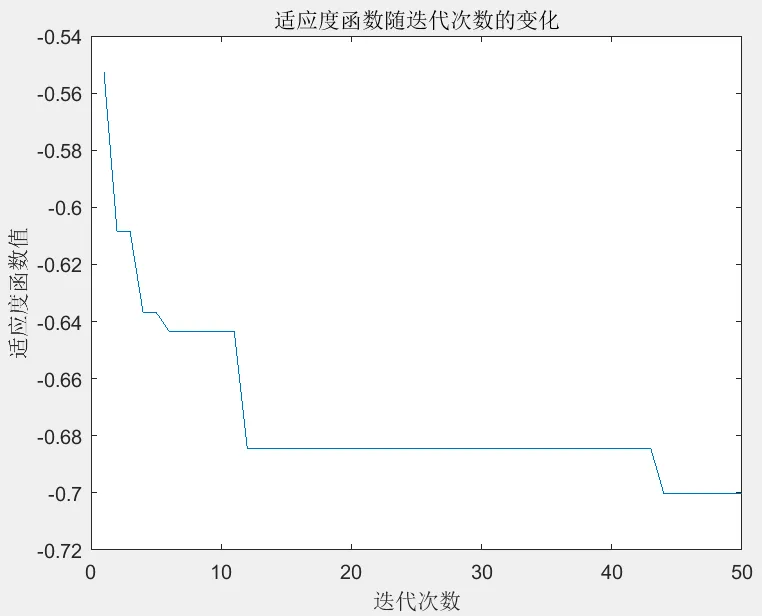
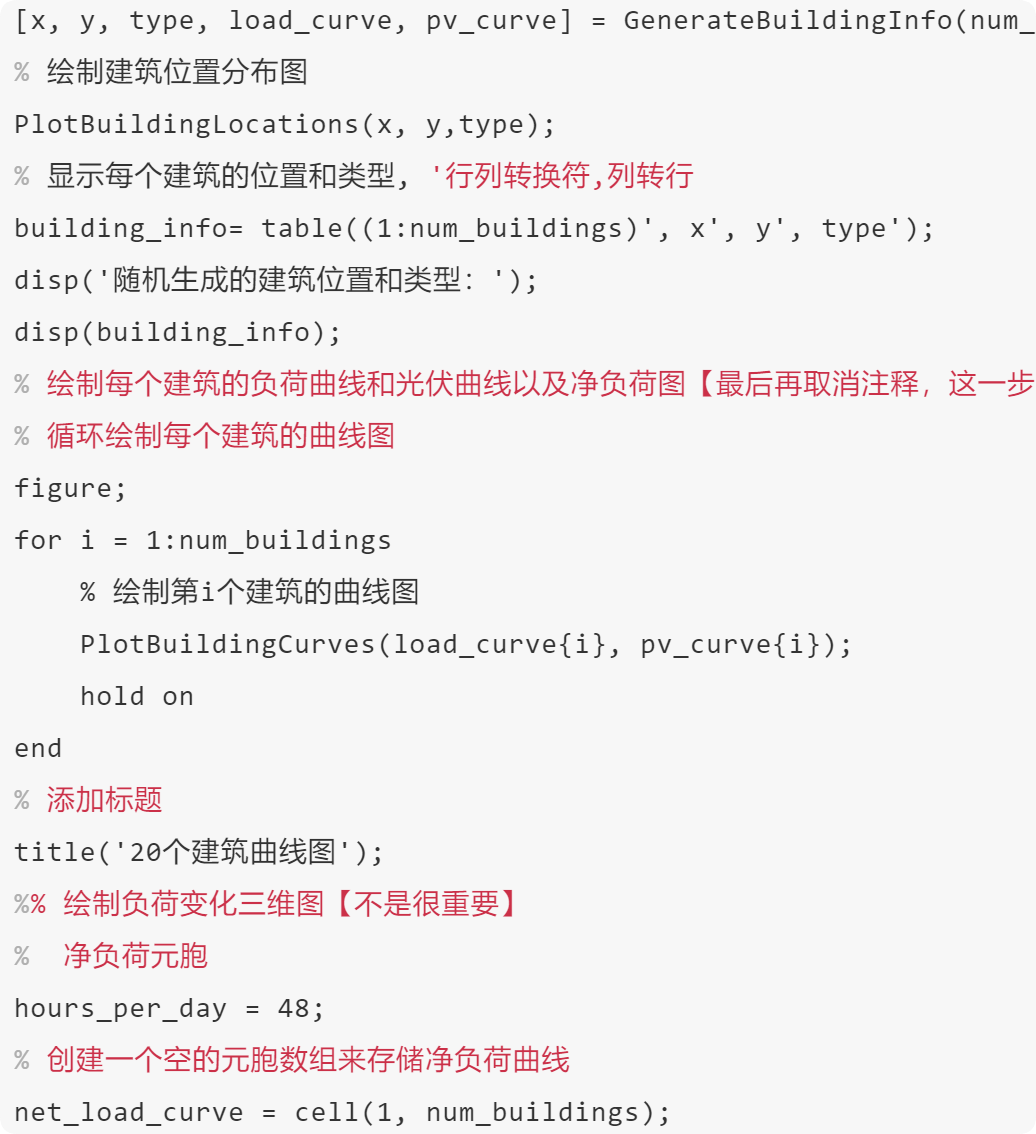
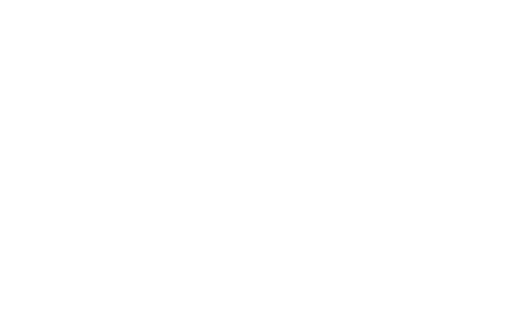

3References
Some content in this article is sourced from the internet, and sources will be indicated or cited as references. If there are any inaccuracies, please feel free to contact us for deletion. (The content of this article is for reference only, specific effects are subject to running results)

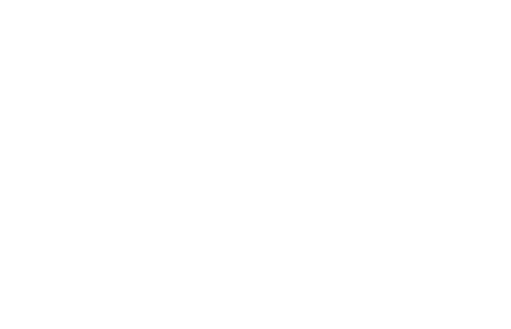

4 MATLAB Code Implementation
1. Introduction
With the acceleration of urbanization and the widespread application of distributed energy, the power system faces unprecedented challenges. Especially in the case of large-scale distributed photovoltaic grid connection, the coordinated autonomy of the power system becomes particularly important. Therefore, this study aims to optimize the power line planning strategy among multiple buildings through clustering methods to improve the efficiency and stability of the power system.
2. Research Background
-
Development of Distributed Energy: With the continuous advancement of renewable energy technology, the proportion of distributed energy (such as solar and wind energy) in the power system is gradually increasing. These distributed energies are intermittent and uncertain, bringing new challenges to the scheduling and control of the power system.
-
Complexity of Building Spatial Layout: The spatial layout of buildings in urban areas is complex and diverse, with different electrical distances, load characteristics, and photovoltaic generation capacities among buildings. These differences make the clustering planning of the power system more complicated.
3. Research Method
-
Modularity Index Based on Electrical Distance: Modularity is an important indicator for measuring the strength of network structure. In the distribution network, the edge weight of modularity is represented by electrical distance. Therefore, this study uses a modularity index based on electrical distance to measure the structural strength of clustering. By calculating the electrical distance between nodes within different clusters, the rationality of clustering can be assessed.
-
Objective Function: The objective function consists of three parts: line laying cost, cluster purchasing cost, and modularity. By optimizing the objective function, the best clustering scheme can be found. Among them, the line laying cost reflects the construction cost of power lines between buildings; the cluster purchasing cost reflects the ability to reduce purchasing costs from the external network through energy complementarity within the cluster; and modularity is used to measure the structural strength of clustering.
-
Solution Method: This study uses particle swarm optimization for solving. Particle swarm optimization is an optimization algorithm based on swarm intelligence, with advantages such as fast convergence speed and strong global search capability. By continuously iterating and updating the position and speed of particles, the optimal clustering scheme can be found.
4. Research Case
Taking 20 randomly generated buildings as an example, the number of buildings, load, and photovoltaic data can all be adjusted in applications. Through modular programming and clear comments, the research is facilitated. In the running results, the variations of line laying cost, cluster purchasing cost, and modularity under different clustering schemes can be observed.
5. Research Results and Discussion
-
Effect of Clustering: Through reasonable clustering, significant reductions in line laying costs and cluster purchasing costs can be achieved. At the same time, the electrical distances between nodes within the cluster are relatively close, and the modularity is high, indicating that the clustering has strong structural integrity.
-
Analysis of Influencing Factors: The load characteristics and photovoltaic generation capacity of buildings have significant impacts on the clustering effect. Buildings with similar load characteristics are more likely to be clustered together, while buildings with strong photovoltaic generation capacity can reduce cluster purchasing costs through energy complementarity.
-
Optimization Suggestions: To further enhance the clustering effect, more constraints and optimization objectives can be considered. For example, geographical location, electricity price differences, and environmental factors can be considered as constraints; simultaneously, system stability, reliability, and economic efficiency can also be integrated as optimization objectives.
6. Conclusion and Outlook
This study optimized the power line planning strategy among multiple buildings through clustering methods. By comprehensively applying the modularity index based on electrical distance, the objective function, and particle swarm optimization, reasonable clustering solutions were obtained. The research results indicate that reasonable clustering can significantly reduce line laying costs and cluster purchasing costs, improving the efficiency and stability of the power system. Future research may further consider introducing more constraints and optimization objectives to enhance the effectiveness and practicality of clustering.
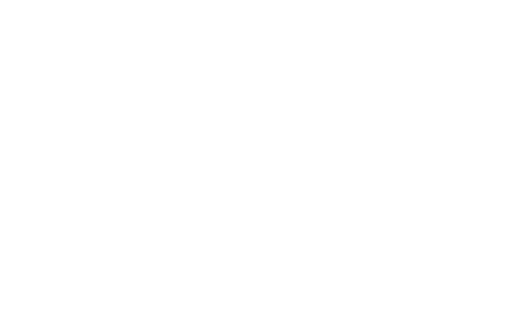

2Running Results

2Running Results
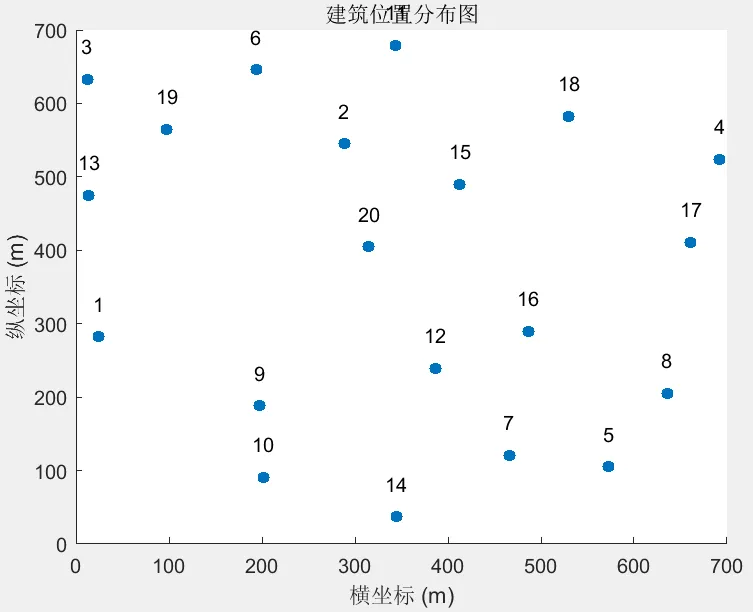
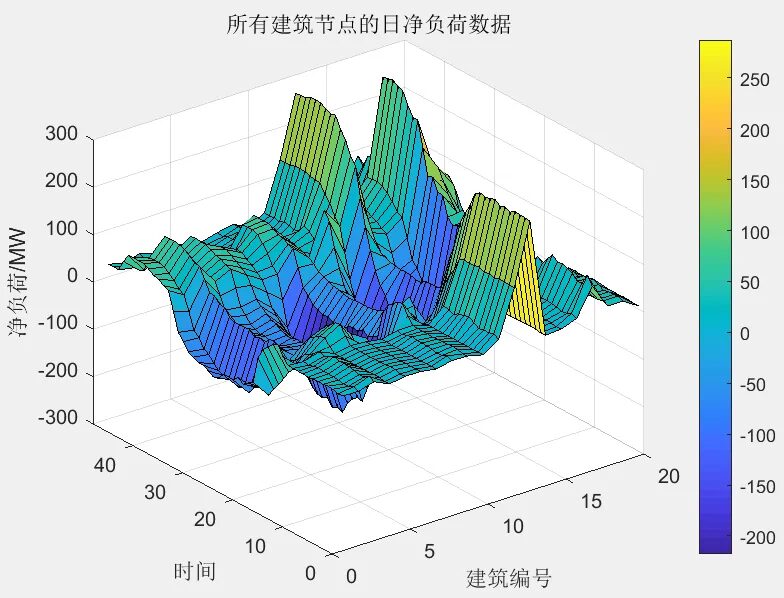
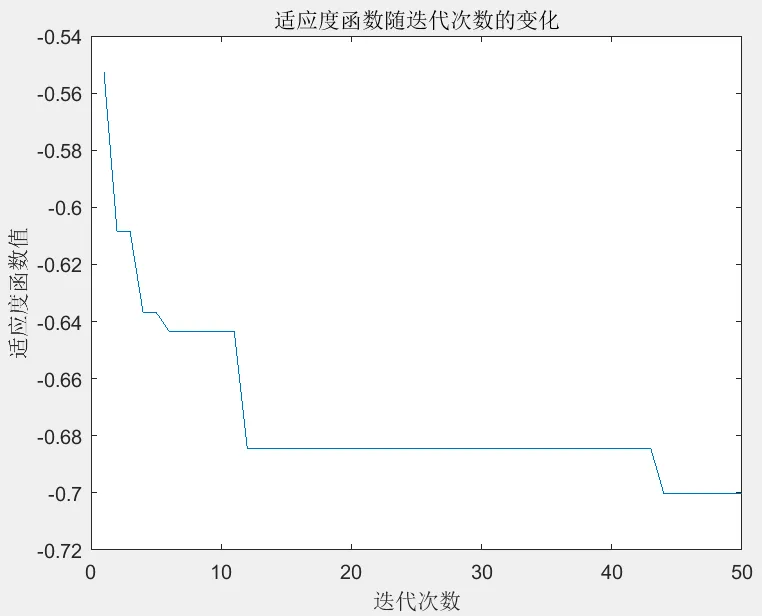
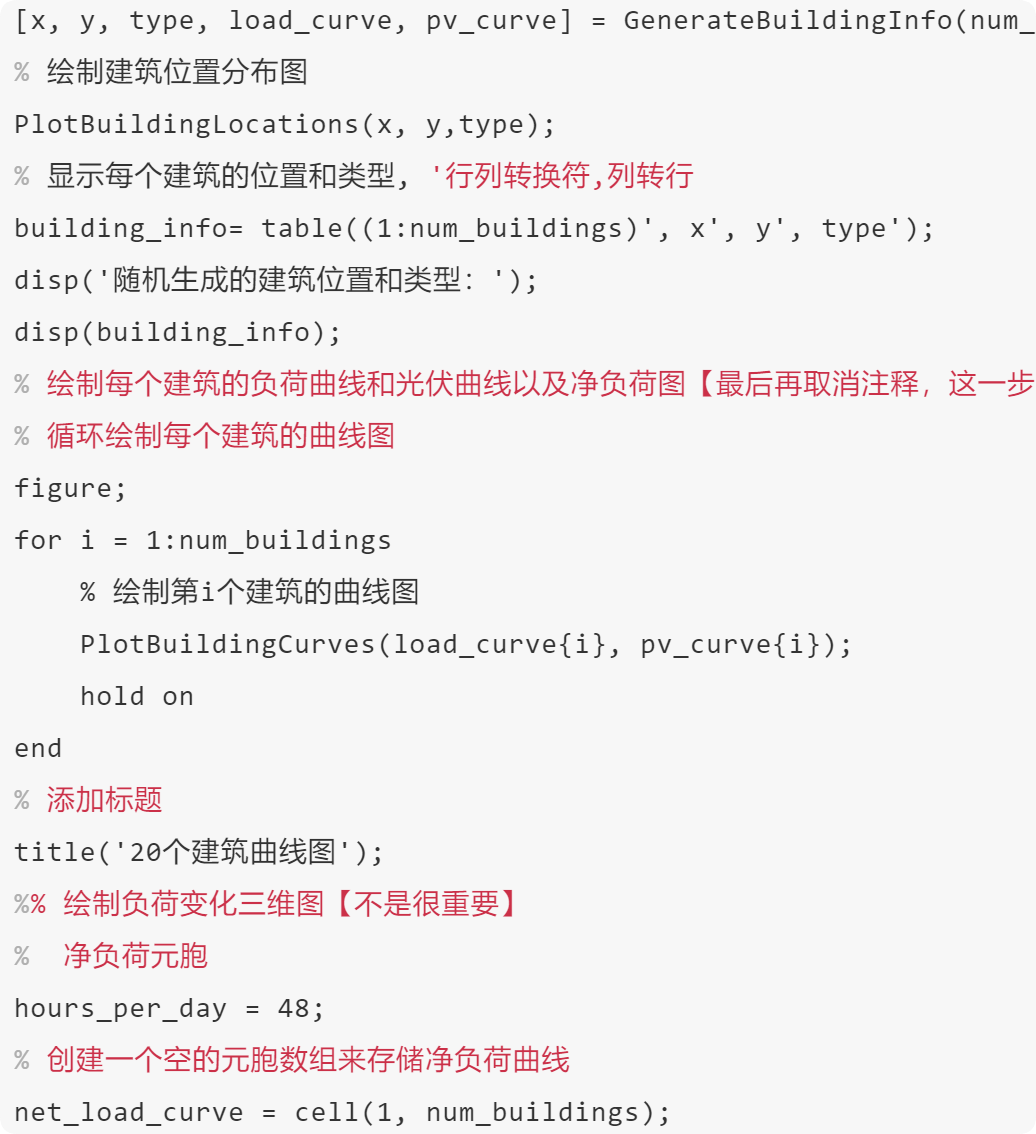
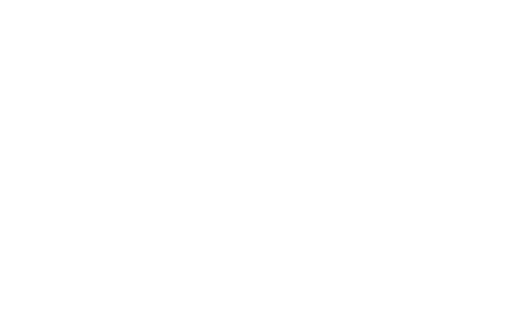

3References

3References
Some content in this article is sourced from the internet, and sources will be indicated or cited as references. If there are any inaccuracies, please feel free to contact us for deletion. (The content of this article is for reference only, specific effects are subject to running results)

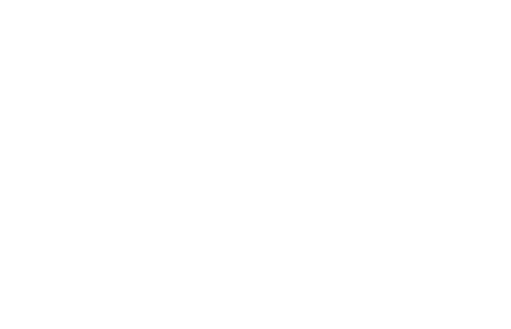

4 MATLAB Code Implementation
For more resources, follow us for MATLAB|Simulink|Python resources