Every time a new industry term emerges, we media professionals face the challenge of understanding what a company aims to achieve, especially when they use these terms to fulfill their marketing goals. A recently popular term is Edge Artificial Intelligence (AI), or Edge AI.
Due to the rapid development of the Internet of Things (IoT) and the increased computing and processing power needed to enable IoT devices to be intelligent, the scope of ‘edge’ can be quite broad, referring to any device from the ‘edge of a gateway’ to ‘endpoints.’ So, is there a consensus in the industry regarding the definitions of edge vs. endpoint? Who needs Edge AI? How smart can edge devices be? Let’s explore.
First, what is the difference between edge and endpoint? Well, everyone has their own opinion—everything outside the cloud can be referred to as edge.
Definition: Edge can refer to many devices, while endpoint is just that—an endpoint.
The clearest definition may come from Wolfgang Furtner, Senior Director of Concept and System Engineering at Infineon (Figure 1). He believes that the term ‘Edge AI’ is as ambiguous as ‘edge.’ Some refer to vehicles as edge devices, while others use the term to refer to small, low-power, wireless-connected energy-harvesting sensors. Edge is relative; it distinguishes local from cloud. However, it is indeed necessary to differentiate the various devices at the edge; sometimes terms like ‘edge-of-the-edge’ or ‘leaf nodes’ are used. Edge AI can refer to many devices, including computational servers in vehicles.
Figure 1 Wolfgang Furtner from Infineon believes that the term ‘Edge AI’ is as ambiguous as ‘edge.’
But he emphasizes that ‘endpoint AI sits at the intersection of the virtual network world and the real world, where sensors and actuators are very close together.’
Markus Levy, Machine Learning (ML) Technology Director at NXP Semiconductors, believes this is actually a semantic understanding, depending on how the boundaries are drawn. ‘Edge ML is the same as endpoint machines, except that Edge ML can also include ML in gateways or even fog computing environments. Endpoint ML is typically used in distributed systems; for example, our customers have even implemented intelligence in the sensors of these distributed systems. Another example is home automation systems, which include ‘satellite’ devices (like thermostats, doorbell cameras, security cameras, or other types of connected devices) that can independently perform ML functions and also send data to the gateway for higher-level ML processing.’
Regarding the increasing intelligence of edge servers and endpoints, Chris Bergey, General Manager and Vice President of Arm’s Infrastructure Business, expresses a slightly different viewpoint (Figure 2). He states, ‘Basic devices like bridges and switches are no longer suitable; instead, powerful edge servers have replaced them, which bring data center-level hardware to the gateways between endpoints and the cloud. These new edge servers, in combination with 5G base stations, are very powerful and can perform complex AI processing—not just ML inference but also training.’
Figure 2 Chris Bergey from Arm holds a different view on the intelligence of edge servers and endpoints.
What is the difference between edge servers and endpoint AI? Bergey says, ‘Smartphones contain powerful hardware, making them fertile ground for endpoint AI for a long time. With the integration of IoT and AI technologies, and the continuous maturation of 5G, although devices are smaller and cheaper, they are smarter and more powerful, and due to less reliance on the cloud or the internet, they also offer better privacy protection and reliability. As endpoint devices become more intelligent, the boundaries of where AI is executed are beginning to merge from endpoints to edges, creating an urgent need for heterogeneous computing infrastructure.’
Some people believe that everything outside the cloud is edge. For example, Jeff Bier, founder of Edge AI and the Vision Alliance, states, ‘We define Edge AI as all or part of AI executed outside of data centers. Intelligence may be right next to the sensor of a smart camera; or a little further away, in a grocery store’s equipment cabinet; or even further, at a cellular base station; or it could be some combination or variation of these.’
Nick Ni, Director of AI, Software, and Ecosystem Product Marketing at Xilinx, holds a similar view (Figure 3). He states, ‘Edge AI is fundamentally intelligence that does not rely on data centers and can meet its own needs in applications. This is crucial for applications that require real-time responses, security (for example, not sending confidential data to data centers), and low power (for most devices). Humans do not rely on data centers every day to make countless decisions; in the coming years, Edge AI will dominate market applications such as semi-autonomous vehicles and smart retail systems.’
Figure 3 Nick Ni from Xilinx believes that Edge AI is fundamentally intelligence that does not rely on data centers and can meet its own needs in applications.
Andrew Grant, Senior Director of AI at Imagination, also agrees with this view. ‘We know that everyone is talking about the edge now. How it develops will depend on the customers. There will definitely be a hybrid approach in the future, with cloud computing and data centers absolutely having a place in it,’ he adds, ‘The market is rapidly moving towards the edge, and there is currently a wave of movement towards edge intelligence, but for many applications, the implementation of specific chips takes time.’ Grant further explains, ‘After communicating with a traffic management company in China, we learned that they were still transferring data back and forth from the cloud. I told them what we were doing, and they immediately realized that if traffic lights could determine whether vehicles were moving, there would be no need to send data to the cloud, which would be greatly beneficial.’
Gideon Intrater, CTO of embedded systems supplier Adesto Technologies, states that they provide products for both IoT edge servers and IoT edge devices, so there is no need to distinguish between edge and endpoints (Figure 4). ‘We don’t use the term endpoint much; the definition of endpoint may be similar to that of edge devices. The AI in these devices is usually some local inference, using dedicated accelerators, processing through near-memory processing or in-memory computing, with algorithms executed on processors.’
Figure 4 Gideon Intrater from Adesto Technologies states that they provide products for both IoT edge servers and IoT edge devices, so there is no need to distinguish between edge and endpoints.
He adds, ‘Almost all applications will achieve Edge AI. AI can enable predictive and preventive maintenance, quality control in manufacturing, thus benefiting industries like industrial, construction, and many others, creating huge opportunities. This industry is just getting started, and we expect AI to do more every day. Old devices without AI capabilities often cannot understand our requests, which frustrates us, while some devices can directly understand us. End users are not clear about how AI works; they just want AI to be useful.’
Therefore, the definition in this article is clear: you either agree that edge is everything outside the cloud, or you agree that endpoints are the intersection of the real world and the digital world, where most are sensors. However, as the boundaries between edge and endpoint become increasingly blurred, along with the development of heterogeneous computing architecture, where AI is executed is actually determined by specific applications.
The next question is who needs it, and what are the market expectations for Edge AI? ‘We are all still working on it,’ Levy explains, ‘Large companies in the industry are actively implementing Edge AI, and many of our customers are also implementing various ML at the edge. However, from the ‘technology adoption cycle’ perspective, I still believe that most companies in the industry have not even entered the ‘early adoption phase’; real development will wait until the end of 2020.’
Figure 5 Markus Levy from NXP states that the real development of Edge AI will wait until the end of 2020.
Customers are still learning about the cool evolutions that ML can bring. And Levy is considering:
1. Can it save money? For example, if AI replaces inspectors, making factory production lines operate faster and more efficiently, can it save costs?
2. Can it make money? For instance, adding a special feature to a product to make it more practical. One application scenario is barcode scanners; if the barcode on the outer packaging is wrinkled, it cannot be scanned accurately, but using ML can eliminate the impact of wrinkles.
Furtner believes this actually raises a question, ‘What benefits will Edge AI bring?’ He says, ‘The edge has a huge advantage, which is that we can turn its limitations or ‘weaknesses’ into strengths. Usability, functionality, privacy, security, cost, and the sustainable use of climate or resources are all benefits that Edge AI can bring. We believe that applying AI in the right places can improve our quality of life; there are many real-world applications of endpoint AI. Edge AI can be used for predictive maintenance, further automation or robotics, home automation, or smart agriculture, etc. We have developed low-power sensors that utilize AI, making intuitive sensing more prevalent, giving rise to new applications in homes or cities that make life easier, safer, and more environmentally friendly. The unreliability of the cloud has prompted industries or homes to adopt entirely new application models to meet privacy and security requirements.’
Additionally, Furtner states that Edge AI can utilize massive IoT data more efficiently and sustainably, which is especially important in the context of climate change.
Figure 6 Jeff Bier from Edge AI and the Vision Alliance believes that five key application demands will drive the development of Edge AI.
Bier says that the following five key application demands will drive the development of Edge AI:
Bandwidth:Even with 5G, there may not be enough bandwidth to send all raw data to the cloud.
Latency:Many applications require faster response times, even shorter than the time it takes for users to receive data from the cloud.
Cost-effectiveness:If an application uses the cloud, even if it can technically solve bandwidth and latency issues, it may be more cost-effective to execute AI at the edge.
Reliability:If an application uses the cloud, even if it can technically solve bandwidth and latency issues, the network connection to the cloud is not always reliable, and the application may need to operate continuously. In this case, Edge AI is needed. For example, for a facial recognition door lock, if the network connection is interrupted, you would want the door lock to continue functioning normally.
Privacy:If an application uses the cloud, even if it can technically solve issues like bandwidth, latency, reliability, and cost, many applications may still require local processing for privacy reasons. For example, baby monitors or bedroom security cameras.
The Intelligence of Edge AI Depends on Memory Capacity
This question may seem obvious, but it must be clarified that each application is different.
‘Intelligence is usually not the limiting factor—memory capacity is,’ Levy believes. ‘In fact, memory capacity limits the size of the ML models that can be used, especially in the MCU domain. For example, an ML model for a visual application requires more processing power and larger memory capacity. When real-time response is required, processing power becomes more important.’
‘Take a microwave oven as an example; it has a camera inside to determine the type of food placed inside, and a response time of 1 or 2 seconds is sufficient, so a processor like NXP’s i.MX RT1050 can be used. Memory capacity determines the size of the model, which in turn determines the types of food the microwave can recognize. However, what if the microwave cannot recognize the food placed inside? Data can be sent to the gateway or cloud for identification, and that information can then be used to retrain the intelligent edge device.’
Levy’s answer to ‘How smart can Edge AI be?’ is, ‘Simply put, it is a trade-off between performance, accuracy, cost, and energy. Additionally, NXP is developing an application that uses autoencoders to implement another form of ML, namely anomaly detection. In short, autoencoders are very efficient; an example we implemented used only 3KB of data, completing inference in 45-50μs—making it easier for MCUs to handle.’
Furtner also endorses this practical approach. Edge AI is greatly limited in terms of energy consumption, space, and cost. In this case, the question is not ‘How much intelligence should we provide at the edge?’ but ‘How much intelligence can we provide at the edge?’ The next question is ‘Can we simplify an existing AI technology to make it small enough to be applicable at the edge?’ Power consumption undoubtedly limits the degree of endpoint intelligence. These endpoints are often powered by small batteries and sometimes rely on energy harvesting, and data transmission also consumes a lot of energy.
Furtner adds, ‘Take a smart sensor, for example. Under these conditions, for local AI to function properly, certain attributes and behaviors of the sensor must be optimized. Additionally, some new sensors can only be realized through embedded AI, such as liquid and gas environmental sensors. There are many reasons for needing endpoint AI, including the use and reduction of intelligent data, or rapid real-time local responses, which are two of them. Data privacy and security are also two reasons; massive raw data from sensors can be processed where it is generated, while intensive computational tasks still occur in the cloud. With advances in low-power neural computing (such as edge TPUs and neuromorphic technologies), this boundary is starting to shift towards edge nodes and endpoints.’
Figure 7 Andrew Grant from Imagination Technologies advocates implementing as much intelligence as possible at the edge, then optimizing the software over the device’s lifecycle.
Grant states, ‘We believe that as much intelligence as possible should be implemented at the edge, and then software should be used to optimize it over the device’s lifecycle.’ He compares it to the gaming console industry; when a vendor releases a new console, it is optimized through software updates over the entire lifecycle of the hardware. He adds that from a cost or size perspective, adding a neural network accelerator to a system-on-chip (SoC) is not important; ‘thus, the opportunities for edge acceleration are truly enormous.’
Bergey states, ‘As heterogeneous computing becomes more prevalent in infrastructure, we must be able to determine where it is best to process data, which varies by application and can even differ at different times of the day. The solutions the market needs are those that allow different layers of AI to take on different roles to understand the big picture, thus driving real business transformation. At the edge, AI plays a dual role. At the network layer, it can analyze data flows and intelligently send data to the most appropriate processing point, which could be the cloud or other nodes, achieving network prediction and network function management.’
Intrater adds that how smart the edge should be depends on specific applications, how much latency can be handled (not much for critical real-time applications), how much power range is available (very small for battery-powered devices), security and privacy issues, and whether connectivity is available. Even if connectivity is available, you would not want to send all data to the cloud for analysis, as that would incur significant bandwidth overhead. Defining intelligent areas between the edge and cloud is to balance all these issues.
Bergey continues, ‘AI can also be executed on local edge servers, but training and analysis are usually done in the cloud. Deciding where AI should execute is not that easy; ‘intelligence’ is often distributed, with some executed in the cloud and some in edge devices. A typical AI system is divided into local execution and remote execution. Alexa/Siri is a good example, where the device has algorithms to perform voice/keyword recognition, while interaction occurs in the cloud.’
What Are Enabling Technologies?
‘Implementing Edge AI requires many key enabling technologies. The first that comes to mind is high-performance, low-power, inexpensive processors suitable for executing AI algorithms,’ Bier states, along with many other technologies, among which the most important include:
Software tools: to facilitate the effective use of these processors;
Cloud platforms: to gather metadata from edge devices and manage the provisioning and maintenance of edge devices.
Most of the companies interviewed can provide a range of devices and IP for Edge AI. Infineon claims to provide sensors, actuators, MCUs (including neural network accelerators), and hardware security modules for IoT. Furtner states, ‘We have significant advantages in power efficiency, security, and safety. Using our products allows for connecting the real world with the digital world, providing secure, reliable, and energy-efficient AI solutions at the edge.’
Ni states that bringing AI edge products to market is not easy, as it requires engineers to integrate ML technologies with sensors and combine traditional algorithms like computer vision and signal processing. ‘To achieve end-to-end responsiveness, all workloads need to be optimized, which requires an adaptable domain-specific architecture that allows both hardware and software to be programmable. Xilinx SoCs, FPGAs, and ACAP provide such self-adjusting platforms that can sustain innovation while meeting end-to-end product demands.’
NXP states that its enabling technologies include both hardware and software. Levy states, ‘Some customers use our low-end Kinetis or LPC MCUs to implement some intelligent functions. At our i.MX RT cross-processor level, things start to get more interesting; we offer MCUs that integrate Cortex-M7 cores, with operating frequencies of 600-1,000MHz. Our new RT600 includes an M33 and HiFi4 DSP, which accelerates various components of neural networks through heterogeneous mode, thus achieving medium-performance ML. With the spectrum raised, the latest i.MX 8M Plus integrates four A53 cores with a dedicated neural processing unit (NPU), which can provide 2.25 TOPS computing power, improving inference performance by two orders of magnitude, with power consumption of less than 3W. This high-end NPU is crucial for applications like real-time voice recognition (i.e., NLP), gesture recognition, and real-time video and object recognition.
Regarding software, Levy states that NXP provides the eIQML software development environment, implementing open-source ML technologies across the NXP product range (from i.MX RT to i.MX 8 application processors and beyond). ‘With eIQ, customers can execute ML on their chosen processing units (including CPU, GPU, DSP, or NPU) as needed. There may even be some heterogeneous implementations, such as running voice applications for keyword detection on DSP, facial recognition on GPU or CPU, high-performance video applications on NPU, or any combination of them.’
Bergey states, ‘The global IoT device count is approaching 1 trillion, and our infrastructure and architecture face unprecedented challenges. To seize this massive opportunity, we need evolving technologies. Arm primarily provides configurable and scalable solutions to meet performance and power requirements, thus making AI ubiquitous.’
Adesto also provides edge AI enabling technologies, including ASICs with built-in AI accelerators, NOR flash that stores weights in voice and image recognition AI chips, and smart edge servers that send new and old data to the cloud (like IBM Watson and Microsoft Azure) for analysis.
Figure 8 Adesto uses RRAM technology to develop in-memory AI computing, where a single memory unit serves as both a storage element and a computing resource. (Image Source: Adesto)
Intrater adds, ‘We are also developing in-memory AI computing using RRAM technology, where a single storage unit serves as both a storage element and a computing resource. In this paradigm, deep neural network matrices become arrays of NVM units, and matrix weights become the conductivity of NVM units. Applying input voltage to RRAM units and summing the resulting current completes the dot product operation. Since there is no need to move weights between computing resources and memory, this model can achieve a perfect combination of power efficiency and scalability.’
In the author’s view, there is a very clear distinction between Edge AI and Endpoint AI. Endpoints are the intersection of the physical world and the digital world, while the definition of edge is quite flexible. The statements of suppliers also vary; some believe everything outside the data center is edge (including gateways, network edges, and vehicles), while others define endpoints as a subset of edges.
How to define it is not important. Ultimately, it comes down to specific applications and how much intelligence can be implemented at endpoints or edges. This requires a trade-off between available memory capacity, performance requirements, costs, and energy consumption to determine how much inference and analysis can be achieved at the edge, how many neural network accelerators are needed, and whether they are part of SoCs or used alongside CPUs, GPUs, or DSPs. Of course, in addressing these challenges, do not forget to use innovative methods and technologies, such as in-memory computing and AI.
We all agree that ‘how smart the edge should be’ is determined by specific applications, and practical methods should be found based on available resources.
This article is original to EET Electronics Engineering Magazine. For reprints, please leave a message.
↓↓ Scan the QR code to subscribe to the magazine for free ↓↓
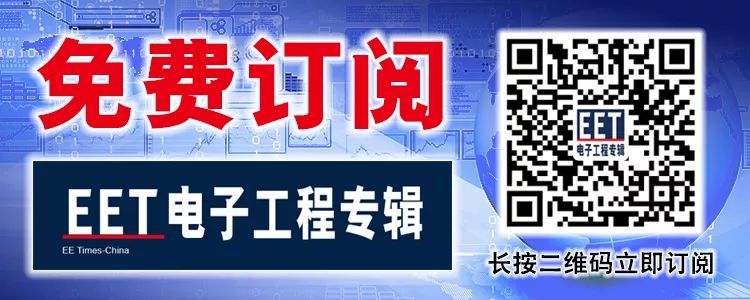