Click the “Machine Vision” above, and select “Pin/Star” in the upper right corner…Public Account to receive the latest articles!
Source: Translated by Machine Heart
Original article: https://towardsdatascience.com/i-built-a-diy-license-plate-reader-with-a-raspberry-pi-and-machine-learning-7e428d3c7401
Author: Robert Lucian Chiriac, translated by Machine Heart
With some free time, we equipped our beloved car with a Raspberry Pi, added a camera, designed a client, and successfully built a real-time license plate detection and recognition system.
How to create an intelligent car system without changing the vehicle? For some time, the author Robert Lucian Chiriac has been pondering how to give a car the ability to detect and recognize objects. This idea is intriguing because we’ve seen the capabilities of Tesla, and while I can’t buy a Tesla right away (I must mention that the Model 3 is looking more and more attractive), I had an idea to strive for this dream.
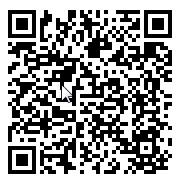
-
Detect the license plate.
-
Recognize the text within each license plate bounding box.
-
A machine learning model that detects license plates using unlabeled images as input;
-
Some hardware. Simply put, I need a computer system connected to one or more cameras to call my model.
-
YOLOv3 – This is one of the fastest models available today and has a comparable mAP to other SOTA models. We use this model to detect objects;
-
CRAFT Text Detector – We use it to detect text in images;
-
CRNN – In simple terms, it’s a recurrent convolutional neural network model. It must be sequential data to arrange detected characters into words in the correct order;
-
First, the YOLOv3 model receives frames from the camera and finds the bounding boxes of the license plates in each frame. It is not recommended to use very precise predicted bounding boxes — having the bounding box slightly larger than the actual detected object is better. If it’s too tight, it may affect the performance of subsequent processes;
-
The text detector receives the cropped license plates from YOLOv3. If the bounding box is too small, it’s likely that part of the license plate text is also cropped out, resulting in poor prediction results. However, when the bounding box is enlarged, we can allow the CRAFT model to detect the positions of the letters, making each letter’s position very precise;
-
Finally, we can pass each word’s bounding box from CRAFT to the CRNN model to predict the actual words.
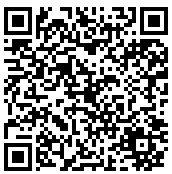
-
On the rearview mirror side, the Raspberry Pi + GPS module + 4G module will be retained. You can check my article about the EC25-E module for the GPS and 4G antennas I used;
-
On the other side, I used a ball-joint arm to support the Pi Camera.
-
Keras implementation: https://github.com/experiencor/keras-yolo3
-
Submit merge request: https://github.com/experiencor/keras-yolo3/pull/244
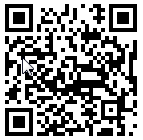
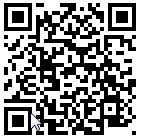
-
Perform all inference locally;
-
Perform inference in the cloud.
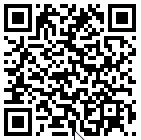
-
Defining a cortex.yaml file, which is our API configuration file. Each API will handle one type of task. I assigned the task of detecting license plate bounding boxes on the given frame to the yolov3 API, while the crnn API predicts license plate numbers with the help of the CRAFT text detector and CRNN;
-
Defining predictors for each API. Basically, all you need to do is define a predict method for a specific class in cortex to receive a payload (all the server part has been handled by the platform), this payload can be used to predict results and then return the prediction results. It’s that simple!
<span># predictor.pyimport boto3</span>import picklelabels = [<span>"setosa"</span>, <span>"versicolor"</span>, <span>"virginica"</span>]<span><span>class</span> <span>PythonPredictor</span>:</span> <span><span>def</span> <span>__init__(<span>self</span>, config)</span></span>: s3 = boto3.client(<span>"s3"</span>) s3.download_file(config[<span>"bucket"</span>], config[<span>"key"</span>], <span>"model.pkl"</span>) <span>self</span>.model = pickle.load(open(<span>"model.pkl"</span>, <span>"rb"</span>)) <span><span>def</span> <span>predict(<span>self</span>, payload)</span></span>: measurements = [ payload[<span>"sepal_length"</span>], payload[<span>"sepal_width"</span>], payload[<span>"petal_length"</span>], payload[<span>"petal_width"</span>], ] label_id = <span>self</span>.model.predict([measurements])[<span>0</span>] <span>return</span> labels[label_id]
curl http://***.amazonaws.com/iris-classifier \ -X POST -H <span>"Content-Type: application/json"</span> \ -d '{<span>"sepal_length"</span>: 5.2, <span>"sepal_width"</span>: 3.6, <span>"petal_length"</span>: 1.4, <span>"petal_width"</span>: 0.3}'
-
Collect frames from the Pi Camera at an acceptable resolution (800×450 or 480×270) with a frame rate of 30 FPS and push each frame into a public queue;
-
In a separate process, I will take frames from the queue and distribute them to multiple workstations on different threads;
-
Each worker thread (or what I call inference thread) will make API requests to my cortex API. First, a request goes to my yolov3 API, and then, if any license plates are detected, another request with a batch of cropped license plates is sent to my crnn API. The predicted license plate numbers will be returned in text format;
-
Each detected license plate (with or without the recognized text) will be pushed to another queue, which will eventually broadcast it to the browser page. Simultaneously, the predicted license plate numbers will be pushed to another queue to be saved to disk in CSV format later;
-
The broadcast queue will receive a set of unordered frames. The consumer’s task is to first place them in a very small buffer (the size of a few frames) and broadcast a new frame to the client for reordering. This consumer runs in a separate process, and it must also try to keep the queue size fixed at a specified value to display frames at a consistent frame rate. Clearly, if the queue size decreases, the frame rate will decrease proportionally, and vice versa;
-
Meanwhile, in the main process, another thread will run to get predictions and GPS data from another queue. When the client receives a termination signal, predictions, GPS data, and timestamps will also be stored in a CSV file.
-
Reduce the width to 416 pixels, which is the required size for the YOLOv3 model, and the scale is clearly lossless;
-
Convert the image to grayscale;
-
Remove the top 45% of the image. The idea here is that license plates won’t appear at the top of the car frame because cars don’t fly, right? As far as I know, removing 45% of the image doesn’t affect the predictor’s performance;
-
Convert the image back to JPEG, but at a much lower quality this time.
Recommended Popular Articles:
001: Research resources and journals, conference introductions in the field of computer vision
002:German kuka robot vs world champion table tennis
003:120 images outline the complete map of the global AI industry!
004:Facebook open-source computer vision system, understanding images at the pixel level (includes papers and code)
005:If you want to become a machine learning engineer? This self-learning guide is worth saving
006: Eleven common filtering algorithms
007: Basics of image processing and computer vision, classic and recent developments
008: In-depth report on the robotics industry (full version)
009: From laundry girl to Google chief scientist, she changed the AI界 with loneliness!
010:Industrial-grade machine vision industry research report
011:Brief description of the principle of dual telecentric industrial lenses
012:How to equip an academic iPad?
013:Overview of machine vision systems
014:German craftsmen: We don’t have “good quality at a low price”
015:Why is the best robotic arm 7 degrees of freedom, not 6?
016:The most powerful technology video ever!
017:Comparison of the top 10 popular programming languages for robots, which one do you master?
018:Novel and complex mechanical principle diagrams!
019: A comprehensive collection of knowledge related to robot control systems
020: The working principles of robots, the most detailed analysis ever!
021: Knowledge points for selecting light sources
022: This is what a mechanical hand is, this is automation, what you have is nothing!
023: Basic knowledge of cameras and lenses
024: The panoramic view of the IoT industry chain (includes another 13 panoramic views of the electronic industry, must collect)
025: How powerful is Japan? Breathtaking! I couldn’t sleep all night after watching it
026: German machinery amazes the world: how lonely is invincibility
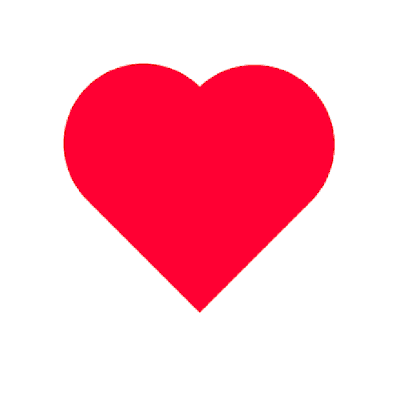
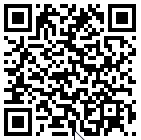