Click the blue text above
Follow us!

When we drive through communities and towns and see children walking and riding bicycles, we realize the importance of road safety. A study by the National Highway Traffic Safety Administration (NHTSA) in 2021 showed that, on average, 20 pedestrians die in traffic accidents every day in the United States—one pedestrian dies every 71 minutes. A study by the World Health Organization in 2022 found that 1.3 million people die each year due to road traffic accidents, with more than half being pedestrians, cyclists, and motorcyclists. Unfortunately, distracted driving is one of the leading causes of these accidents, and this trend seems to be increasing every year.
Advanced Driver Assistance Systems (ADAS) help mitigate the impact of driver distraction, providing comprehensive protection for drivers, pedestrians, and vulnerable road users. To achieve a five-star safety rating and meet regulatory requirements, additional rearview cameras, front cameras, and driver monitoring systems must be added. As a result, many manufacturers are improving their vehicle architectures to integrate various active safety features into ADAS domain controllers.
Domain controllers typically require:
-
Ability to connect to various sensors: quantity, mode, and resolution.
-
Visual, Artificial Intelligence (AI), and general processing for perception, driving, and parking applications.
-
Connection to low bandwidth and high-speed vehicle networks.
-
Functional safety and assurance to prevent critical components from being compromised.
Processing and System Requirements for ADAS Domain Controllers
The growing demand for system memory, computational performance, and input/output (I/O) bandwidth complicates system design and increases system costs. Today’s high-end ADAS systems use multiple cameras of different resolutions and are equipped with various radar sensors around the vehicle to provide a complete view of the driving environment. For each set of images collected from the sensors, detection and classification algorithms supported by AI and computer vision need to operate at high frame rates per second to accurately interpret the scene. This presents multiple challenges for system and software designers, including connecting these sensors to processing systems, transferring their content to memory, and synchronizing data for real-time processing by classification algorithms.
The Texas Instruments (TI) TDA4VH-Q1 System on Chip (SoC) (as shown in Figure 1) integrates functions such as visual preprocessing, depth and motion acceleration, AI network processing, automotive network interfaces, and safety microcontrollers (MCUs). The TPS6594-Q1 power management integrated circuit is optimized to power the TDA4VH-Q1 in applications requiring compliance with Automotive Safety Integrity Level (ASIL) D, including some functional safety features such as voltage monitoring, hardware fault detection for the TDA4VH-Q1 SoC, and a watchdog to monitor the MCU on the SoC for software errors that could cause a lockup.
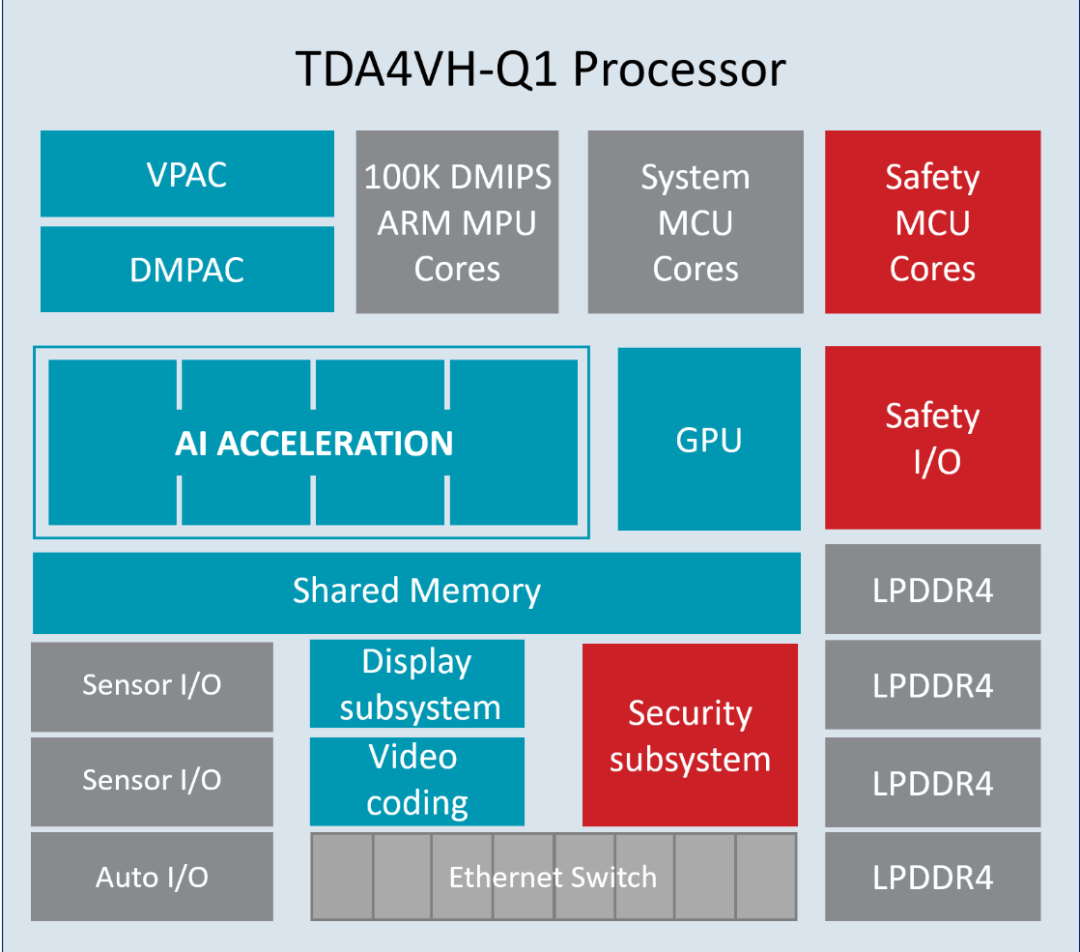
Figure 1: Simplified diagram of TDA4VH-Q1 SoC
Supporting Multi-Camera Vision Perception
One example of ADAS applications requiring enhanced processor performance is multi-camera vision perception. Installing cameras around the vehicle provides a 360-degree field of view, helping to prevent frontal collisions and assisting drivers in monitoring traffic and pedestrian activity in blind spots and adjacent lanes.
Phantom AI utilizes TI’s J784S4 processor open-source software development kit (SDK) to develop a multi-camera vision perception system for the TDA4VH-Q1. Phantom AI’s PhantomVision™ system provides a complete set of ADAS functionalities for the TDA4VH-Q1 processor, ranging from compliance with EU General Safety Regulations to meeting the U.S. Society of Automotive Engineers (SAE) Level 2 and Level 2+ standards. In addition to basic functionalities such as vehicle, vulnerable road user, free space, traffic sign, and traffic light detection, PhantomVision™ also includes additional features like construction zone, turn signal, and tail light detection, as well as AI-based self-path prediction. Its multi-camera perception system consists of a combination of front, side, and rear cameras that cover the vehicle’s 360-degree field of view, helping to eliminate blind spots (see Figure 2).
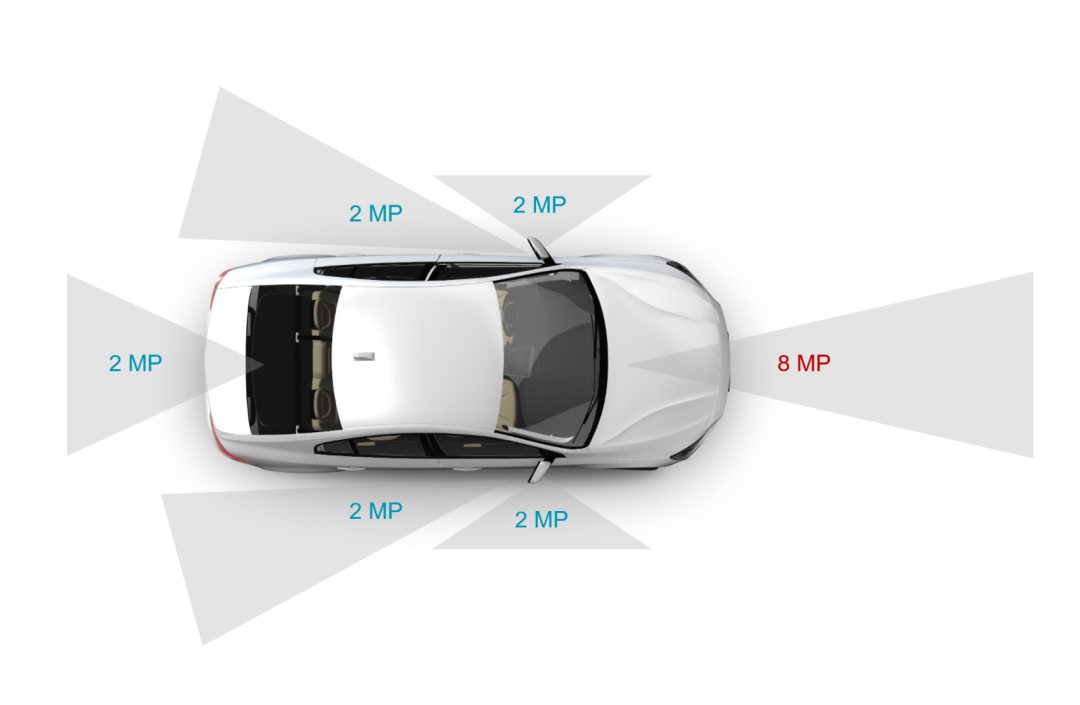
Figure 2: Camera positions for 360-degree view used by Phantom AI
By leveraging the high-performance computing, deep learning engine, and dedicated accelerators for signal and image preprocessing of the TDA4VH-Q1, Phantom AI enables real-time operation. The dedicated visual preprocessing accelerators handle the camera pipeline, including image capture, color space conversion, and multi-scale image pyramid construction. Combined with Texas Instruments’ deep learning libraries, the TDA4VH-Q1’s trillions of operations per second high-speed multicore digital signal processors and matrix multiplication auxiliary engines provide efficient neural networks with fast algorithms and minimal I/O operation scheduling, achieving high accuracy and low latency.
Conclusion
Building complex multi-sensor ADAS systems for SAE Level 2 and Level 2+ driving does not require water-cooled supercomputers. With TI’s well-designed TDA4VH-Q1 SoC and the expertise of automotive engineers like Phantom AI, market-ready systems that meet functional safety requirements can be developed at a competitive price. While we are excited about the future of autonomous driving, the true goal of designing cost-effective systems that meet functional safety requirements is to make our world safer. Making ADAS technology accessible to more automotive market segments (equipping more vehicles with more ADAS) will provide a better and safer experience for drivers and pedestrians.

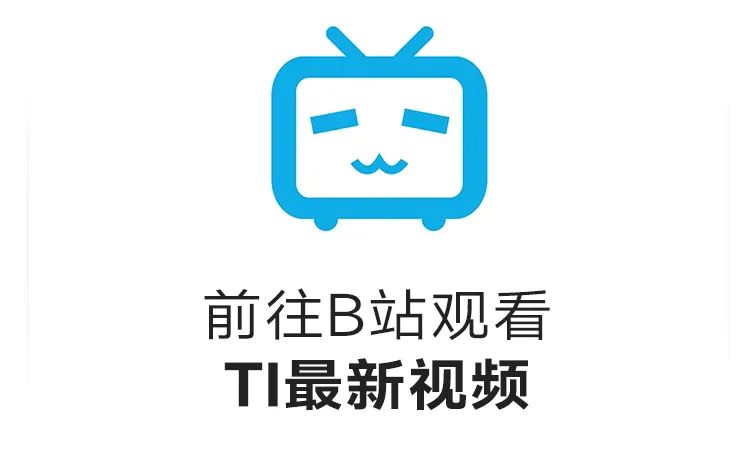
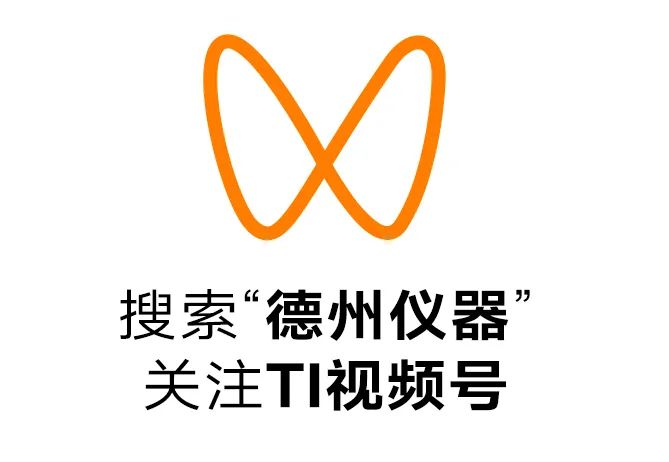

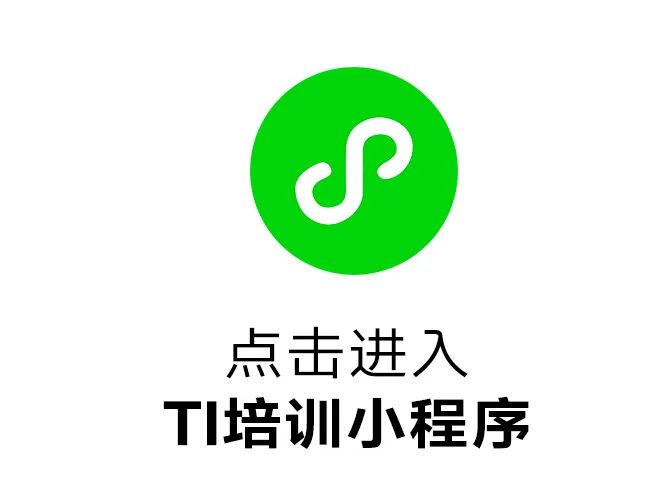
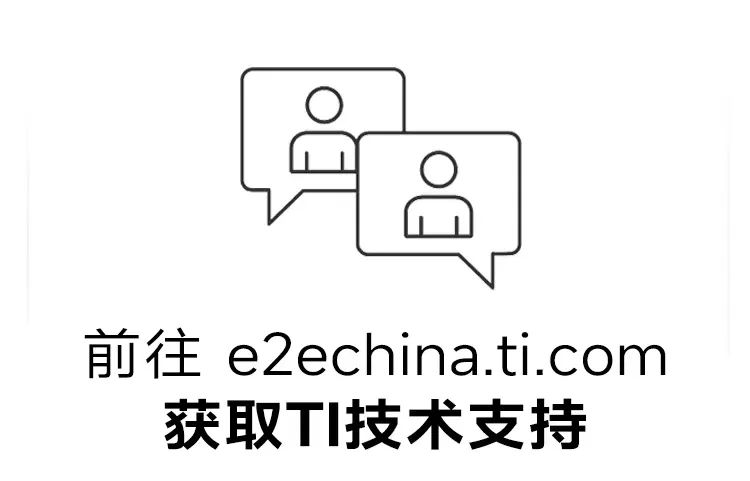

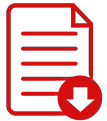
Click “Read the Original” to learn more about TI TDA4VH-Q1 products!