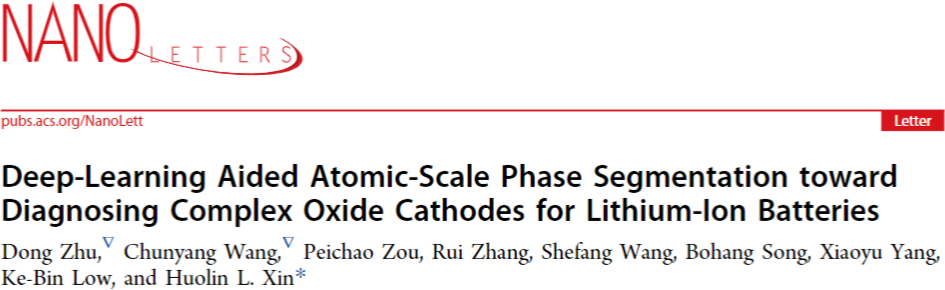
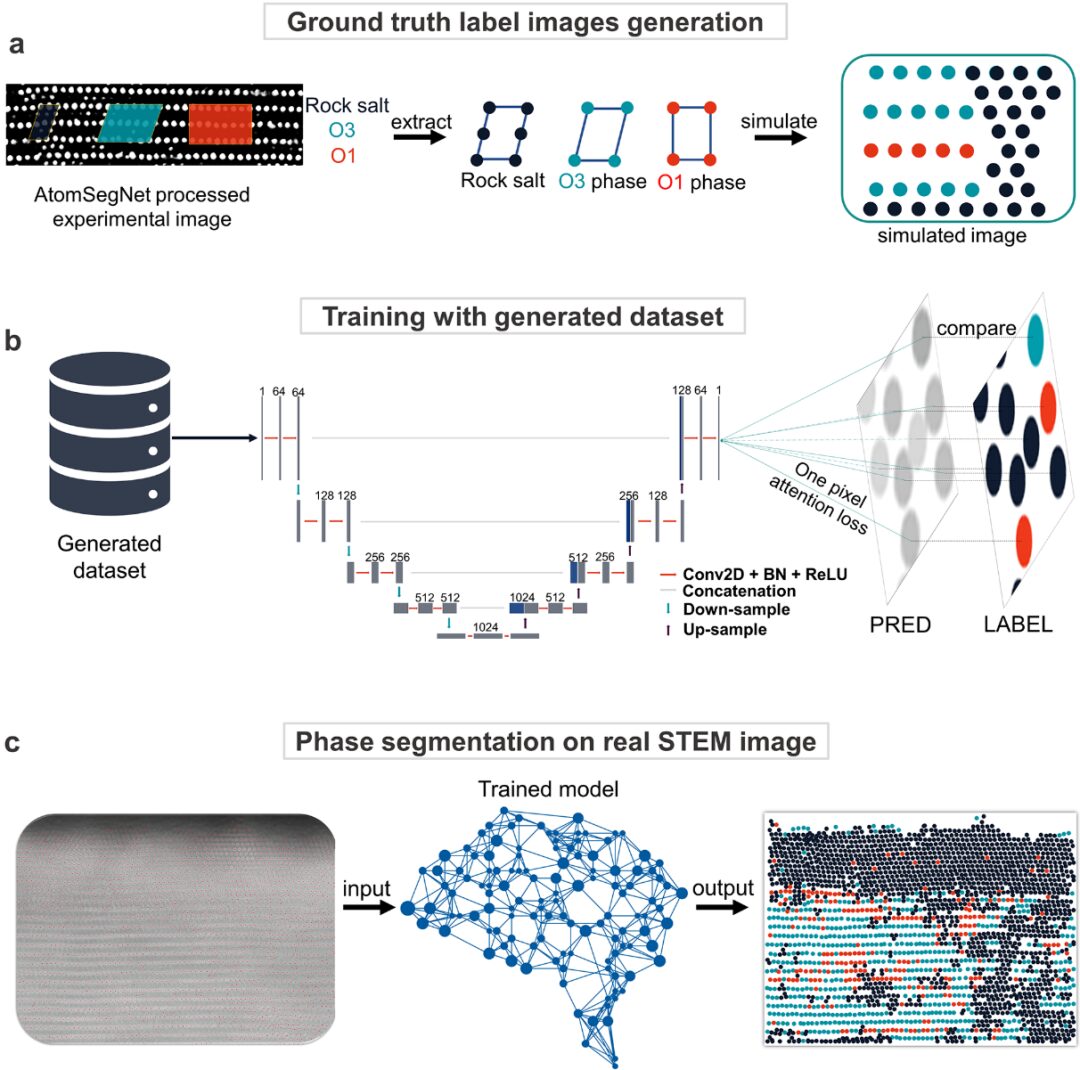
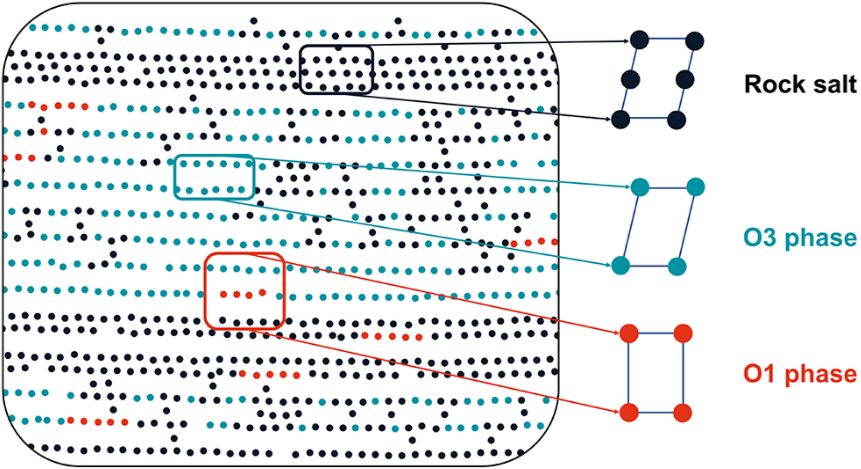
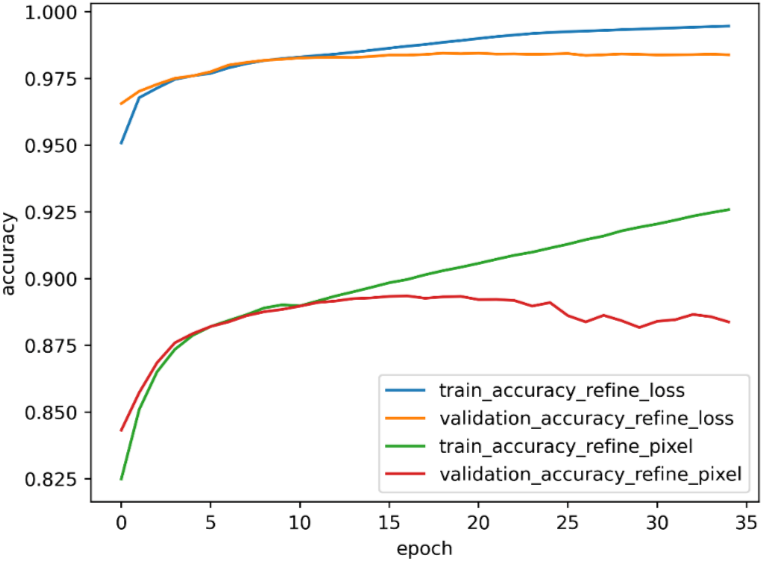
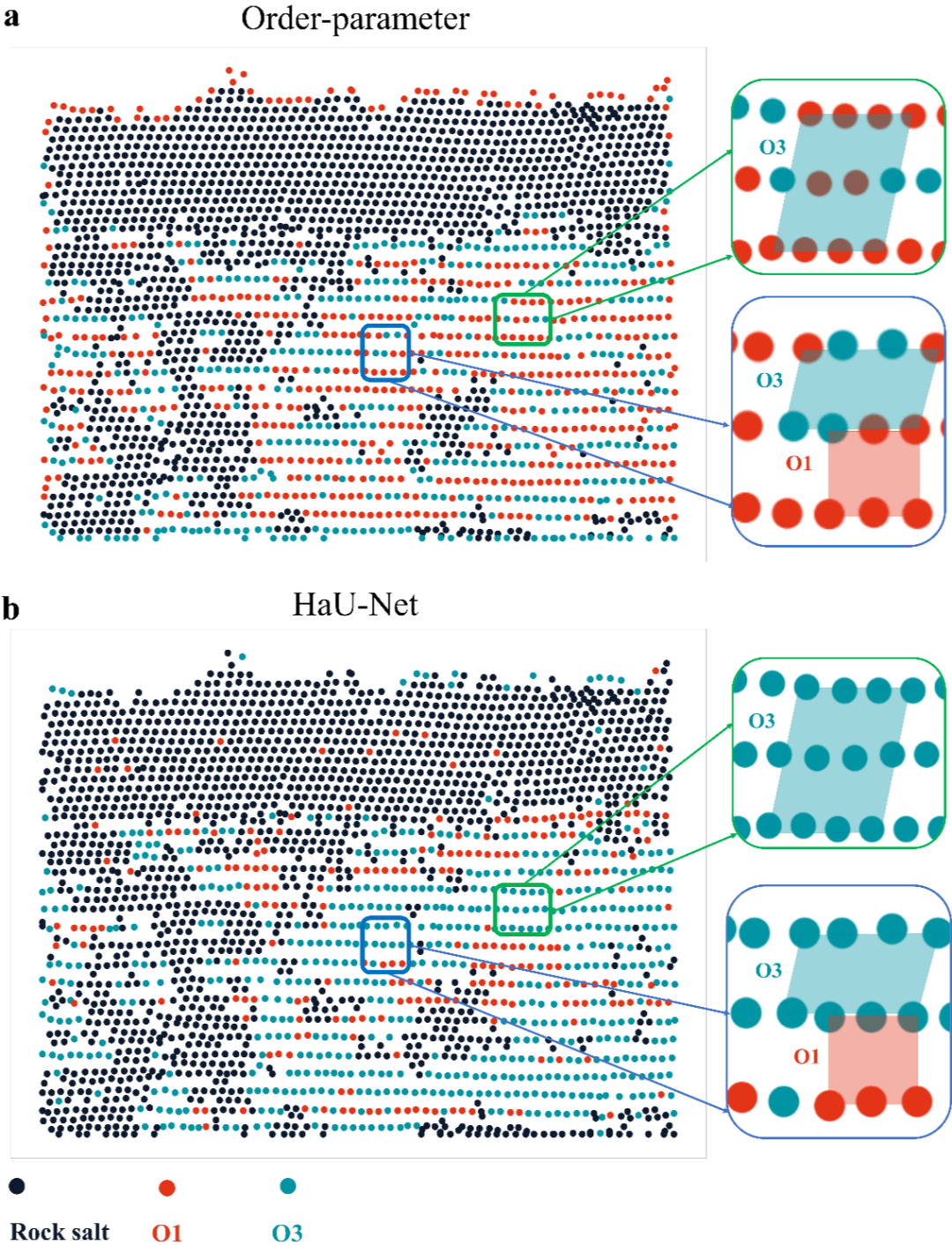
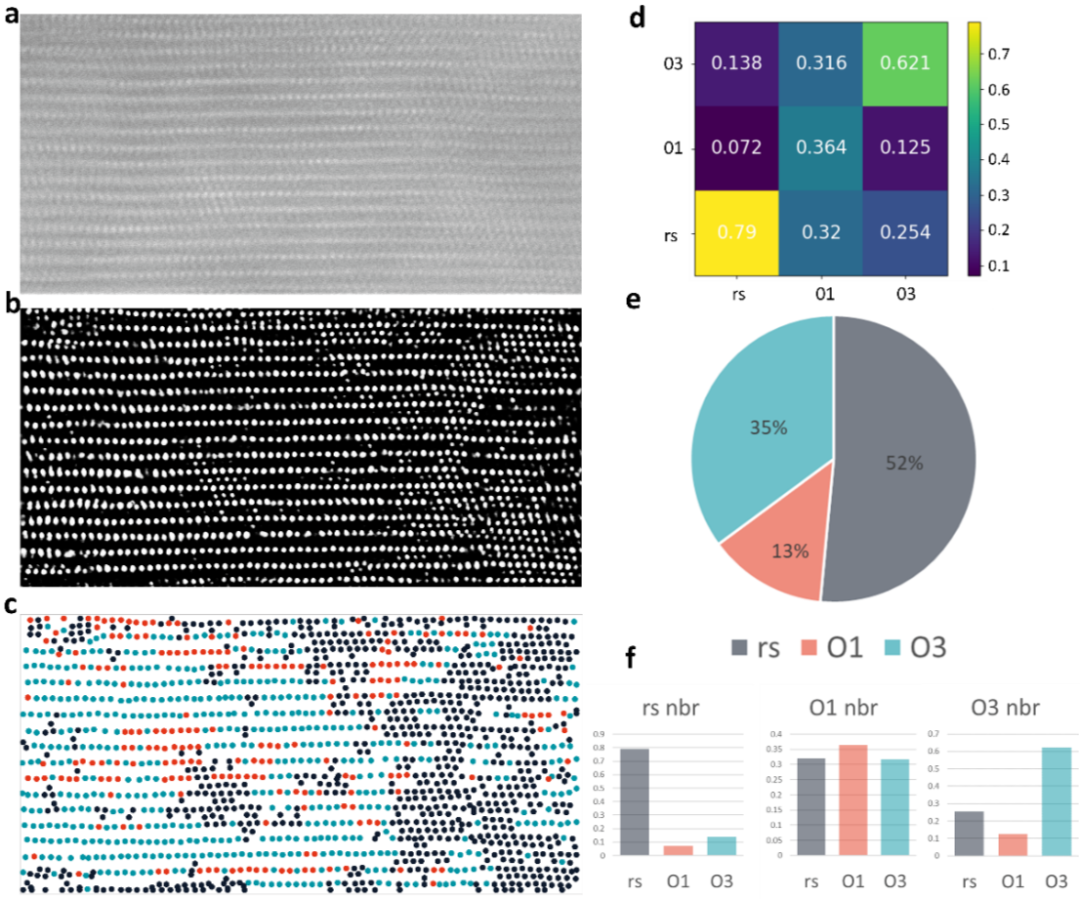
Polysulfide/Manganese Flow Battery: Low Cost, High Specific Energy, Scalable Production
2023-09-03
Researcher Mingjun’s Electrolyte Binder Article: Discussing the Impact of Binder Functional Groups on Li+ Desolvation Processes
2023-09-02
Mechanism Study on Improving Silicon Anode Performance by Mixing Different Sized Silicon Particles
2023-09-02
Southern University of Science and Technology Xu Qiang Team Chemical Society Reviews Review: Progress, Challenges, and Prospects of Sustainable Zinc-Air Battery Chemistry
2023-09-02
Harbin Institute of Technology Zhang Naiqing Advanced Energy Materials: N Backfill to Guard Oxygen Vacancies, Achieving High Stability Zinc Ion Batteries
2023-09-02
Academician Pan Fusheng’s Group EnSM: Chlorine-Free Low-Cost Mg(SO3CF3)2 Electrolyte Strong Solvation Effect Induces Formation of Gradient SEI Interface Layer, Achieving Efficient Metal Magnesium Deposition/Dissolution
2023-09-02
Hu Xile Latest Nature Catalysis: PtRu Synergy & NC Carrier Promote H2 Oxidation Reaction
2023-09-01
Multi-School Cooperation Nature Catalysis: Atomic-Level Metal-Non-Metal Catalysis for Achieving Efficient HOR
2023-09-01
A New Concept of In Situ Multifunctionalization of Electrolytes for Lithium Metal Batteries
2023-09-01
Journal of Energy Chemistry: V6O13/VO2 Electrode Materials Synergistic Application in Long-Lifespan Aqueous Zinc-Ion Batteries
2023-09-01