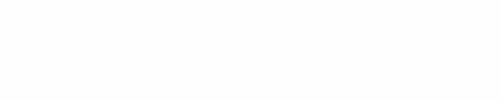
National Certification Remote Forensic Base
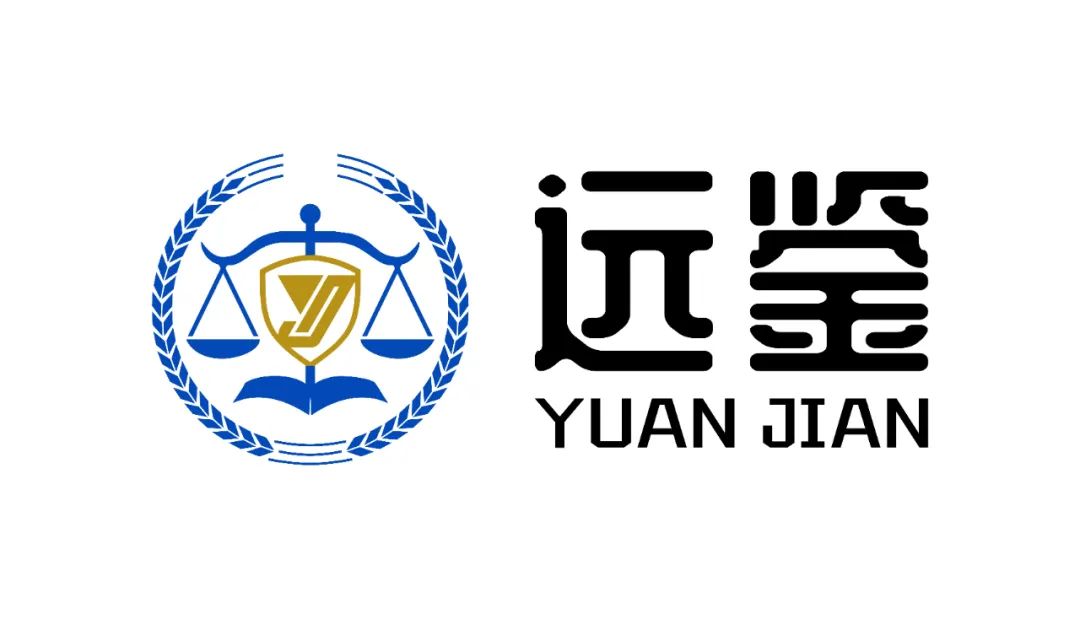
With You

.

.

Learning and Practicing

IntroductionForensic toxicology is an important component of criminal science and technology, studying the properties, sources, routes of entry, mechanisms of action, symptoms of poisoning, metabolism and excretion in the body, poisoning amounts, lethal doses, and pathological changes after poisoning of toxic substances, and separates, extracts, and tests relevant evidence in cases, issuing test reports to provide clues for case resolution and scientific basis for court trials.Although forensic toxicology is a traditional discipline, more than 12,000 new synthetic chemicals are produced globally every day, with new toxins emerging continuously. There are still many challenges in intelligent sampling at poisoning scenes, rapid screening and precise detection of toxic substances, interpreting test results, and understanding poisoning mechanisms.In recent years, the rapid development of artificial intelligence (AI), a branch of computer science, has brought unprecedented opportunities and challenges to scientific and technological research in various fields, and the intersection of forensic toxicology and AI has increasingly become a research hotspot in forensic science. Therefore, this article summarizes the application progress of AI in forensic toxicology, including poisoning scene investigation, toxic substance analysis, toxicology research, target identification, and characterization, exploring its enormous potential, risks, challenges, and development trends, providing references to promote the deep integration of forensic toxicology and AI.
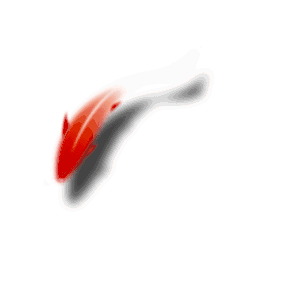
1. Application of AI in Poisoning Scene Investigation
AI is a technology that studies how to enable machines to see, hear, speak, think, and act like humans. Currently, AI is mainly applied in poisoning scene investigations in terms of its “mobility,” particularly in robotics. In poisoning cases, especially in scenes of gas poisoning, explosions, and fires, there may be various toxic and harmful gases present, posing high risks to investigators. Additionally, there are often small tunnels, ventilation ducts, and pipes that are difficult for humans to access. Developing robots and other intelligent equipment for real-time detection of toxic gases on-site to assist investigators in safety assessments, planning investigations, and sample collection is currently the main application of AI in this field.
Thilagar et al. reported a robot designed for detecting toxic gases in sewers, equipped with a gas sensor array and temperature sensor module, capable of detecting harmful gases such as hydrogen sulfide, ammonia, carbon monoxide, carbon dioxide, and methane in sewers, and wirelessly transmitting the detected gas concentration information. If the concentration exceeds a set threshold, it triggers an alarm on a buzzer.Saeed et al. developed a small autonomous robot for gas leak detection, using ultrasonic sensors for obstacle avoidance and infrared sensors for line tracking, and sending wireless signals to a receiving module with an LCD display to monitor gas leak intensity in real-time.Mai Qiuyuan et al. designed a multifunctional integrated toxic substance sampling and detection robot applicable to emergency response at chemical poisoning scenes, capable of detecting toxic gases on-site, remote real-time reconnaissance, remote toxic substance sampling, meteorological detection, obstacle clearance, climbing, and overcoming obstacles, which can be used in emergency scenarios such as chemical spills, reducing personnel safety risks while improving sampling and detection efficiency.
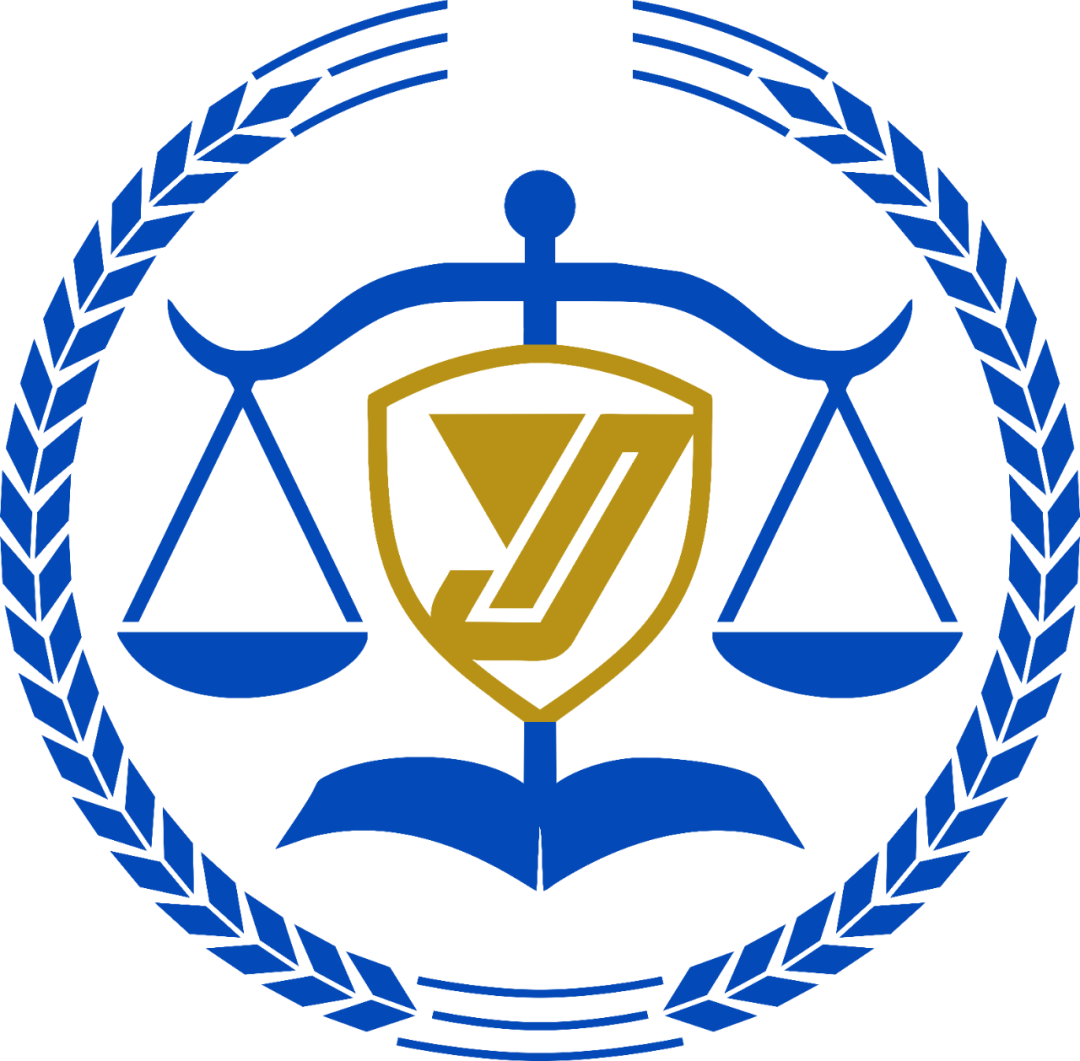
2. Application of AI in Toxic Substance Analysis
The inference and determination of target toxic substances have long been a challenging issue in toxic substance analysis. In cases of suspected poisoning with unclear direction, testing personnel need to infer based on the scene conditions, poisoning symptoms, and findings from forensic autopsies, requiring a high level of interdisciplinary knowledge from forensic medicine, toxicology, and chemistry. Traditional testing primarily relies on expert experience. In recent years, large language models represented by ChatGPT have been able to learn vast amounts of knowledge through unsupervised, semi-supervised, or self-supervised methods and provide responses, creations, summaries, and analyses based on user needs.Abdel-Messih et al. explored the application potential of ChatGPT in target toxic substance inference. They queried ChatGPT (released on February 13, 2023, available through OpenAI) about possible toxic substances and subsequent disposal methods in a simple poisoning case. The case description is as follows: A 28-year-old male truck driver was found unconscious in a parking lot 30 minutes ago, with a heart rate of 110 beats per minute, blood pressure of 150/90 mmHg, respiratory rate of 22 breaths per minute, body temperature of 36.8℃, and blood oxygen saturation of 88% RA, with a suspected solvent smell on his chest and torso, sweating, and pupils at 1 mm, with profuse salivation.Based on the provided information, ChatGPT suggested that the truck driver might have been poisoned by organophosphates, which was consistent with subsequent investigations revealing the driver had been exposed to the pesticide chlorpyrifos. Additionally, ChatGPT recommended further diagnostic tests, including complete blood count, liver function tests, serum electrolytes, arterial blood gas analysis, and chest X-rays, to assess the severity of poisoning, determine the presence of complications, and monitor the patient’s response to treatment. However, the application of AI in target toxic substance inference is still in the exploratory stage, requiring more research in the future while also considering safety, ethical, and legal issues involved.
2.2 Toxic Substance Detection
In toxic substance analysis and detection, AI can assist in compound screening, peak identification, and result determination, enhancing the positive detection capability, structural prediction capability, and accurate quantification of toxic substance testing, enabling rapid spectral library retrieval, accurate quantitative analysis, and sensitive compound detection.For example, Woldegebriel et al. reported a Bayesian probability algorithm for toxic substance screening that effectively combines all possible evidence fragments in chromatography to assist in determining positive results.Romain et al. processed data from liquid chromatography-high resolution mass spectrometry detecting tramadol in human urine and plasma using molecular network technology, discovering 25 previously unreported metabolites.Yang Qiong et al. established a million-scale computer-simulated mass spectrometry database and proposed an ultra-fast and accurate spectral matching method (FastEI), greatly improving the accuracy and speed of compound identification.
The most commonly used methods for toxic substance testing are gas chromatography-mass spectrometry (GC-MS) or liquid chromatography-mass spectrometry (LC-MS). In traditional methods, the selection of chromatographic conditions usually relies on empirical judgment and repeated experimentation, gradually accumulating analytical knowledge through trial and error. The introduction of AI brings innovative solutions to this field, as AI possesses model prediction capabilities based on data learning, enabling rapid virtual screening of chromatographic conditions, effectively reducing the frequency and cost of trial and error; simultaneously, machine learning algorithms are used to process and analyze chromatographic datasets, identifying patterns and correlations in complex data, facilitating the qualitative and quantitative analysis of compounds in complex samples. This research is highly valuable for studies in toxicology and metabolomics, significantly enhancing analytical accuracy and speed.Torigoe et al. utilized training data from 203 polar metabolites to rank feature importance using random forests among 12,420 molecular descriptors (MDs) and constructed a retention time prediction model containing 26 selected MDs. Evaluating the retention time model’s accuracy using test data from 51 polar metabolites resulted in 86.3% of ΔRTs (the difference between measured and predicted values) within ±1.50 min, with an average absolute error of 0.80 min, indicating high retention time prediction accuracy. Using the developed retention time model and computer-simulated MS/MS, they analyzed non-targeted metabolomics data of frozen human plasma metabolites, successfully predicting the structures of 216 polar metabolites in addition to the 62 metabolites identified based on standards.
The studies above demonstrate the significant potential of AI in detecting all possible peak features in given samples and in high-throughput screening tasks, but different algorithms each have their advantages and characteristics, as shown in Table 1, which compares various algorithms in toxic substance detection.
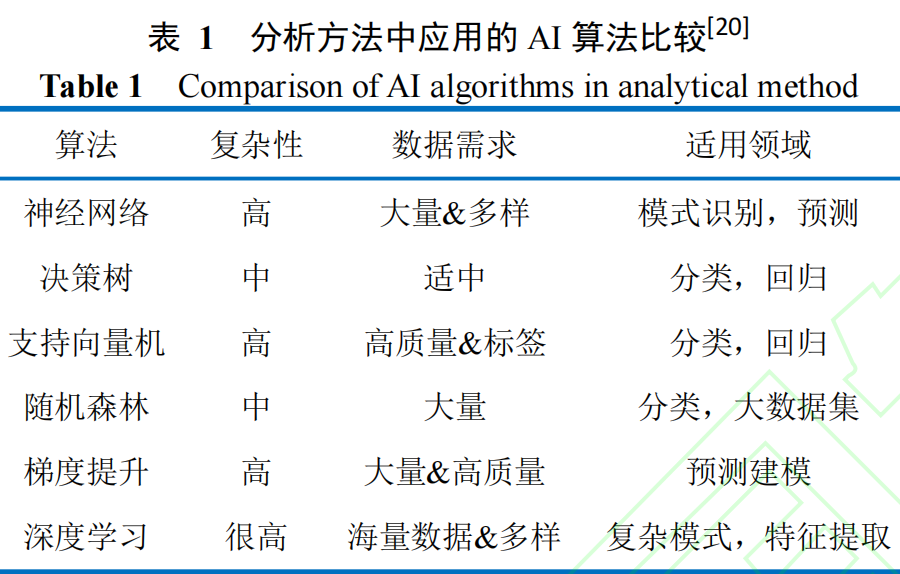
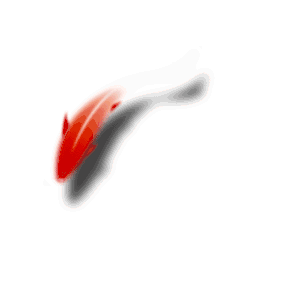
3. Application of AI in Toxicology Research
3.1 Toxicity Prediction
Traditional animal-based toxicity prediction for chemical substances faces challenges such as high costs, time consumption, and ethical issues. Increasingly, researchers are committed to conducting toxicity predictions through computational toxicology methods, and the rapid development of big data and artificial intelligence has strongly supported the development of various toxic substance prediction models. Tan Zhuangsheng et al. established a toxicity prediction model based on the BP neural network module on the Matlab platform, selecting 20 common organophosphate pesticides, with 17 as the training set and 3 as the validation set, capable of predicting the 50% inhibitory concentration of organophosphate pesticides on acetylcholinesterase activity. This model can provide references for risk assessment and toxicity prediction of chemical substances lacking toxicological information in poisoning cases. Studies have shown that quantitative structure-activity relationship (QSAR) or read-across structure-activity relationships (RASAR) models based on big data and machine learning may achieve similar or even better reproducibility than animal experiments.Luechtefeld et al. trained two types of RASAR models (i.e., simple RASAR and data fusion RASAR) based on a large database containing over 866,000 chemical properties/hazards using unsupervised and supervised learning steps. The results indicated that the simple RASAR model achieved a sensitivity of over 80% for animal reproducibility experimental results, with specificity ranging from 51% to 69%. The data fusion RASAR further increased sensitivity to 80% to 95%, while the probability of obtaining the same result in repeated animal experiments is about 78% to 96%. This research demonstrates the advantages of AI models in toxicity prediction. However, predicting the toxicity of compounds is a highly complex field that requires comprehensive consideration of various factors in the selection of modeling methods to achieve optimal results.
3.2 Toxicokinetics
Toxicokinetics, which refers to the dynamics of toxic substance metabolism, applies principles of drug metabolism kinetics in toxicology, primarily studying the rules of absorption, distribution, and elimination of toxic substances in the body. In recent years, the application of AI in pharmacokinetics research has brought new ideas to toxicokinetics research. Exploratory studies have been conducted using deep learning for predicting the absorption, distribution, and metabolism of drugs and toxins. For example, Li et al. established a multi-layer deep belief network (DBN) model based on semi-supervised learning to predict the water solubility of compounds, discussing the impact of feature dimensionality on prediction accuracy and analyzing model parameters for predicting drug solubility, comparing shallow and deep machine learning methods. The results showed that the model can accurately predict the water solubility of drugs and toxins, achieving a prediction accuracy of 85.9%. Although the application of AI in predicting toxicokinetic parameters is still limited, the methods from pharmacokinetics provide good reference models for toxicologists, and future toxicologists can conduct more beneficial explorations in this field.
3.3 Toxic Interactions
In poisoning cases, the phenomenon of multiple drugs and toxins existing simultaneously often occurs, but the toxic data for drugs and toxins in relevant literature and databases mostly comes from single toxic substance experiments, and research on the interactions between toxic substances is scarce. In practical work, it is often faced with the dilemma of lacking reference data for poisoning amounts and lethal doses under multiple drug and toxin interactions, complicating the determination of poisoning causes in related cases. Utilizing text mining technology to extract drug-drug interaction (DDI) research from biomedical literature provides new insights for exploring toxic interactions. For example, Sahu et al. proposed three long short-term memory network models (LSTM), namely B-LSTM, AB-LSTM, and joint AB-LSTM, and validated the models on the SemEval-2013 DDI extraction dataset, with results indicating that even with a simple architecture, the joint AB-LSTM model can achieve reasonable performance (f-value of 69.39%). Zhao et al. proposed a DDI extraction method based on syntactic convolutional neural networks (SCNN), establishing a new word embedding method – syntactic word embedding, with experimental results on the DDI Extraction 2013 dataset showing that SCNN outperformed other methods (f-value of 0.686).
AI’s application in target recognition and characterization
Methods for toxic substance testing based on GC-MS and LC-MS not only serve traditional poisoning case testing and identification but also aid in target recognition and characterization of involved persons, providing clues for investigations. This includes inferring the living and physiological characteristics of donors through detecting drug and toxin components in biological fluids such as blood stains and saliva stains; analyzing hair segments to characterize the drug use behavior of donors; and analyzing volatile substances in human odors for individual identification and characterization. AI can be widely used in constructing recognition and characterization models in such research, and the literature has detailed the application of machine learning in human odor recognition, which will not be elaborated here.
Conclusion and Prospects
AI has demonstrated good application potential in various branches of forensic toxicology, including poisoning scene investigation, toxic substance screening and qualitative and quantitative analysis, toxicology research, and individual identification and characterization of involved persons, providing new opportunities for the development of forensic toxicology, but it also brings new challenges. Specific challenges include:
1) Data issues. High-quality large datasets are crucial for training robust AI models. Currently, the data formats and types obtained in toxic substance analysis are diverse, making it difficult to obtain a large amount of unified and compatible data; at the same time, although there is a wealth of toxicological data, the quality is difficult to guarantee. Before selecting data to develop models, data quality, completeness, reliability, and relevance must be strictly checked. In the future, establishing systematic and authoritative toxicity data assessment and review methods can promote data quality improvement.
2) Model interpretability and adaptability. Machine learning and other AI algorithms are often perceived as lacking interpretability, which poses some resistance to the application of machine learning models in forensic toxicology. Developing interpretable AI models will help researchers better understand the internal processes of algorithms, increasing users’ trust in AI results. Furthermore, a fundamental principle in toxicology is that “dose determines toxicity.” The toxicity of a substance depends on various factors, including exposure dose, time, species, in vitro and in vivo experiments, etc. Most existing computational toxicology models are based on biological activity classification, i.e., whether they possess biological activity/toxicity, and cannot predict the intensity of toxic effects, dose-response relationships, or time dependencies. Models suitable for complex real-world conditions still need to be developed.
3) Safety and regulation. Since much of the research and conclusions in forensic toxicology are related to cases, data security and privacy must be considered. Establishing clear safety protection and regulatory plans is crucial.
4) Team personnel. The integration of forensic toxicology and AI involves multiple disciplines, including chemistry, forensic medicine, toxicology, and computer science. Currently, most forensic toxicology teams lack support from professionals with AI technical expertise. Building and developing interdisciplinary research teams that emphasize the integration and innovation of knowledge from different fields is an important direction for future development.
In summary, AI shows broad application prospects in various branches of forensic toxicology, but existing work is mostly exploratory and requires researchers to continue advancing in-depth studies and validating the feasibility of methods, forming safe, standardized, and reliable methods to better meet the needs of public security practice.
Ren Xinxin, Zou Bo, Dong Linpei, Song Ge, Wu Xiaojun, Chang Jing, Zhang Yunfeng
Criminal Technology2024Year
Forensic Science and Technology
New Media Graphic Planning, Editing/Hongyan
Supervisor, Producer / “Zhe Yi Jian Dao” Ma Yun
Copyright and Disclaimer
-
The articles published in this public account aim to disseminate excellent humanistic and medical education concepts. The above images and texts are valuable for sharing and do not represent the views of this public account;
-
Except for articles specifically marked as “original” authorized for reprinting, other articles are reprints, and the copyright belongs to the original author. If there is any infringement, please contact us in a timely manner;
-
We welcome insightful individuals and colleagues in the industry to communicate and discuss cooperation,
-
When reprinting related articles from this public account, please indicate the source: “Zhe Yi Jian Dao”.


Previous Issues Review Classic Case Analysis Discussion
Philosopher’s Medicine | Forensic Assessment of Rib Fractures
Jian Dao | Case | Causal Relationships in the Assessment of Human Injury Severity and Standardized Expression in Assessment Opinions
Jian Dao | Forensic Analysis Case of Lumbar Transverse Process Fractures
Philosopher’s Medicine | Illustrated | Difficult Issues in Forensic Mental Disability Assessment – Ideas and Methods
Jian Dao | Exploration of Forensic Clinical Expert Consultation System
Philosopher’s Medicine | Illustrated | Forensic Toxicology Laboratory Operation Standards and Risk Management
Jian Wen | Ministry of Justice Releases “Environmental Damage Judicial Appraisal White Paper (2024)”
Jian Xue | Supreme Court Seeks Opinions: Embezzlement Constitutes Fraud, Operators in Fitness, Hairdressing, Education, and Training Must Bear Punitive Compensation Liability for Fraudulent Consumers
Philosopher’s Medicine | Misjudgment Cases | Reflections on Two Cases of Rib Fracture Disability Level Assessment
Jian Dao | Common Issues in the Review of Forensic Clinical Evidence
Jian Xue | 2024 Latest Calculation Rules for Expert Witness Fees
Philosopher’s Medicine | Analysis and Insights from 98 Cases of Tarsal Bone Fractures
Philosopher’s Medicine | Misjudgment Cases | Reflections on Two Cases of Rib Fracture Disability Level Assessment
2024: Guidelines for Evidence in Traffic Accident Damage Compensation
Jian Xue | “200 Articles You Should Know About the Civil Code”
Philosopher’s Medicine | Ministry of Justice Key Laboratory | Forensic Psychiatric Assessment Content Structure and Evaluation Points Analysis
Jian Fa | Forensic Psychiatry Mental Examination Guidelines (2021) GA/1971-2021
Jian Xue | Research Institute of Evidence Science, China University of Political Science and Law, Releases “Expert Consensus on the Authenticity of Medical Records”
Jian Dao | Can Drinking Water Cause Poisoning? A Case Analysis of Severe Injury from Excessive Water Consumption
Jian Xue | Public Course | Heavyweight | Teacher Wang Xiaoming & Analysis of Causal Relationships in Disability Assessment – Including Cases
Philosopher’s Medicine | Case | Analysis of Rib Fracture Assessment
Guidelines for Evidence Collection for Victims of Domestic Violence
[2024] Comprehensive Overview of 483 Crimes and Sentencing Table
2024 Personal Injury Compensation 12 Items + Calculation Methods
Jian Fa | Issues and Handling Processes in Injury Assessment Cases
Jian Xue | Video Highlights | Common Issues in Ethanol Testing and Uncertainty Assessment
Philosopher’s Medicine | Key Points in Forensic Clinical Assessment Technology Management and Risk Control
Philosopher’s Medicine | Forensic Assessment of Death by Asphyxia – Including Cases
Philosopher’s Medicine | Forensic Analysis of a Case of Disguised Homicide Scene
Philosopher’s Medicine | Forensic Examination Case of Female Asphyxia Death in Fujian Province
Philosopher’s Medicine | Forensic Review Analysis of 73 Cases of Death Related to Asphyxia
Philosopher’s Medicine | Forensic Analysis of 364 Cases of Subcutaneous Tissue Contusions
Philosopher’s Medicine | Forensic Review of 1900 Cases of Medical Malpractice
Philosopher’s Medicine | Forensic Kinship Identification Methods and Research Hotspots
Philosopher’s Medicine | Forensic Analysis of Ethanol Detection Results in Blood in Traffic Accidents
Philosopher’s Medicine | Forensic Analysis and Re-evaluation of 54 Cases of Neonatal Death due to Aspiration Pneumonia
Philosopher’s Medicine | Forensic Analysis of 57 Cases of Positional Asphyxia from a Forensic Perspective
Philosopher’s Medicine | Forensic Analysis of 72 Cases of Medical Malpractice in Obstetrics and Gynecology
Philosopher’s Medicine | Forensic Analysis of 113 Cases of Eye Injuries
Philosopher’s Medicine | Forensic Analysis of 89 Cases of Pulmonary Thromboembolism
Philosopher’s Medicine | Forensic Analysis of Sudden Death Related to Sexual Activity
Philosopher’s Medicine | Forensic Analysis of a Case of Incest
Jian Dao | Forensic Analysis of 80 Cases of Swallowing Dysfunction after Brain Injury
Jian Dao | Forensic Analysis of Death from Pulmonary Thromboembolism after Dog Bite
Jian Dao | Forensic Analysis of 59 Cases of Rib Fracture Disability Assessment from Traffic Accidents
Jian Dao | Forensic Analysis of 56 Cases of False Disability Assessment in Traffic Accident Injuries
Jian Dao | Forensic Analysis of Handwriting Identification Cases on a Wall in a Murder and Arson Case
Jian Dao | Forensic Analysis of a Disguised CO Poisoning Death Case
Jian Dao | Forensic Assessment of Multiple Cases of Female Asphyxia Death
Jian Dao | Forensic Analysis of Handwritten Will Cases
Jian Dao | Forensic Analysis of Over 1700 Cases of Medical Malpractice in Zhejiang Province
Jian Dao | Key Points and Challenges in Forensic Clinical Assessment of Nasal Bone Fractures
Jian Wen | After Drinking, the “Activity Trajectory” of Alcohol in the Body
Jian Dao | How to Identify the Authenticity of Company Seals?
Jian Xue | Six Major Laws and Seven Classic Theories
Jian Xue | National Standard | “One Picture to Understand”: GB/T 42430-2023 Testing of Ethanol, Methanol, Propanol, Acetone, Isopropanol, and Butanol in Blood and Urine