Please click on the four words “Si Ying Technology” above to follow us. Si Ying Technology specializes in brain imaging data processing, covering (radiomics, fMRI, gradients, structural images, T1w/T2w, white matter hyperintensity analysis, PVS, brain lymphatic analysis, PET, spectroscopy (CSI/SVS), expression profiling and enrichment analysis, CEST, MTI, DWI (DTI, DKI, NODDI, free water), DTI-ALPS, QSM, myelin water, ASL, IVIM, DCE, DSC, oxygen extraction fraction (OEF) and CMRO2, BOLD-CVR, primate brain imaging, mouse brain imaging, microbiota, EEG/ERP, sleep EEG, TMS-EEG, magnetoencephalography, FNIRS, eye movement, Eprime programming), etc. We hope that professional content can help followers. Feel free to leave comments for discussion, and we also welcome you to participate in Si Ying Technology’s courses. You can add WeChat ID 19962074063 or 18983979082 for consultation. (Click to browse at the end of the article)
Epilepsy is a disorder of brain networks. A better understanding of the structural and dynamic network characteristics may help improve the diagnosis, treatment, and prognosis of epilepsy. Hubs are brain regions that are highly connected to other parts of the brain, usually located on the most efficient communication paths in the brain, supporting large-scale wiring and many higher-order neural functions. Visualization and analysis of hubs provide insights into regional and global network organization and may offer new insights into brain diseases and epilepsy. By supporting interactions between various brain networks, hubs may be related to the spread of seizures and epilepsy-related phenotypes. In this article, we will discuss the growing literature on typical hub organization in common epilepsy syndromes, involving both neuroimaging of brain structure and function, as well as neurophysiological data from magnetic and EEG measurements of neural dynamics. As research increasingly explores the clinical applications of network neuroscience approaches, we emphasize the potential of hub mapping as candidate biomarkers for cognitive impairment and postoperative seizure outcomes. We will conclude this article with a discussion of current limitations and prospects for future research. This article is published in Epilepsia.
Key Points:
-
Changes in brain network organization and dynamics in epilepsy have been linked to clinical features and disease prognosis.
-
Hubs are key nodes in brain networks, supporting efficient and large-scale information flow within networks.
-
We review studies linking typical hub organization in epilepsy to seizure activity, cognitive impairment, and clinical outcomes.
-
This article highlights work conducted across various epilepsy syndromes and imaging modalities.
1 Introduction
The human brain is a complex network where interconnected regions collectively produce macro functions. Within these networks, hubs are brain regions with numerous connections, or located at the intersection of important fiber pathways, crucial for efficient network communication. Hubs play a key role in maintaining network stability and function, serving as key relays for information integration between different subnetworks. However, due to their role as interconnected regions, they may be particularly susceptible to pathological influences, with increasing evidence showing they possess high plasticity and metabolic demands. At the intersection of multiple brain networks, hubs are increasingly recognized as contributors to seizure generation and epilepsy-related functional imbalances. They are more susceptible to structural changes associated with epilepsy, with new results indicating these regions may play significant roles in patient-specific prognosis and disease-modifying therapies. Abnormal network synchronization, due to recurrent excitatory circuits, can facilitate the generation and maintenance of seizures. Therefore, understanding how network changes are associated with epilepsy is a promising new avenue for exploring different epilepsy syndromes. More specifically, the seizure onset zone in focal epilepsy is not only highly excitable but may also be closely connected in terms of structural and dynamic connectivity, representing possible individualized pathways for seizure spread through network hubs. In epilepsy research, hub regions may thus be potential targets for better understanding and modifying disease processes.
In this article, we will first briefly introduce the various techniques available for visualizing structural and functional brain networks (e.g., diffusion-weighted imaging, structural and functional magnetic resonance imaging [fMRI], as well as electroencephalography [EEG] and magnetoencephalography [MEG]) and elucidate the concept of hubs in network neuroscience. An increasing number of techniques can detect the brain as an interconnected network, with mathematical methods identifying hub regions as centers of brain networks. We will discuss work demonstrating spatial associations between network hubness and other neural properties such as metabolic cost, brain plasticity, molecular, and microstructural patterns. The second part will summarize recent findings in multimodal neuroimaging and electrophysiological studies, outlining the role of hubs in epilepsy. Although most literature has focused on temporal lobe epilepsy (TLE), we will also highlight emerging literature on extratemporal and idiopathic/genetic generalized epilepsy syndromes. Specifically, we will discuss advances made in neuroimaging studies showing typical hub organization in epilepsy compared to controls, as well as associations between hub topology and cognitive impairment, and propose potential uses of hub mapping in predicting postoperative outcomes. Despite invasive methods in humans and animals revealing critical network information, they are inherently limited in spatial sampling to selected brain regions. Therefore, this article will focus on non-invasive methods providing a whole-brain perspective to detect network hubs in health and epilepsy. We will conclude our discussion by addressing current knowledge gaps and potential avenues for future research.
2 Non-Invasively Mapping Brain Networks
Recent advances in acquiring and processing neuroimaging and electrophysiological data provide us with a window into understanding the structural and dynamic range of human brain function. These advancements are accompanied by an increasingly adopted approach in neuroscience, which does not view brain regions as isolated modules but considers network-level mechanisms. Network effects reflect the structural and functional connections between regions, and studying them is expected to enhance our understanding of healthy and diseased brains.
Structural connectivity provides a wiring diagram of the brain, a map that limits sustained neural function. A widely used technique for non-invasively approximating human structural wiring is diffusion MRI. Diffusion MRI is sensitive to the diffusion of water molecules in tissue and can be used to measure individual-specific changes in brain microstructure at the voxel level. Furthermore, fiber tracking algorithms applied to diffusion MRI can provide a non-invasive biophysical approximation by tracing continuous paths of preferentially diffusing water molecules throughout the brain, approximating white matter axonal fibers. Over the past few years, with the introduction and continuous improvement of new fiber tracking methods, acquisition and modeling techniques have significantly improved. Additionally, some studies have attempted to cross-validate diffusion MRI fiber tracking, for instance, by comparing with fiber tracking experiments in non-human animals or water phantom data, particularly with very reliable results at the deep white matter level.
Structural networks can also be derived from covariance analysis of morphological MRI markers (e.g., cortical thickness) among individuals in a population. Although this method is merely correlational, it directly compares the gray matter distributions between individuals, unlike fiber tracking-based methods, which are limited to white matter analysis. Structural covariance analysis has been shown to detect sustained functional communication and mature coupling performance, as well as shared developmental genetic influences. Initially limited to the population level, covariance methods are increasingly being adopted at the individual level, particularly through recent extensions of the method that plot morphological similarity networks based on the correlation of multiple morphological and diffusion measures between paired regions within a given individual. Similarly, microstructural profile covariance techniques plot networks based on the similarity of microstructural profiles sampled from entire gray matter bands sensitive to myelin. This approach classifies networks with similar cellular structures, thereby distinguishing sensory and motor regions from integrative association regions, which have more hubs and broader and longer-range connections to other parts of the brain.
Functional network mapping and dynamic network mapping represent other important aspects of network neuroscience. A widely used technique is functional magnetic resonance imaging (fMRI), which assesses brain networks by evaluating statistical associations between hemodynamic signals in the brain, quantifying the interrelatedness of low-frequency oscillations between regions. In recent years, functional connectivity has increasingly been studied through resting-state (also known as task-free) acquisitions, during which participants do not perform any externally constrained tasks. Resting-state networks are relatively reproducible across individuals and correspond to systems that would also be involved in specific tasks. These characteristics make resting-state fMRI a versatile method for detecting multiple functional networks across single low-demand acquisitions.
More directly related to neural function, functional connectivity can also be inferred from electroencephalography (EEG) and/or magnetoencephalography (MEG). Scalp EEG/MEG signals can be analyzed using inverse solution algorithms to analyze inferred cortical and subcortical neural sources. Some EEG/MEG studies validated by intracranial recordings indicate that source imaging can also detect deep sources, particularly in medial temporal structures for EEG and in the thalamus. Compared to fMRI, these two techniques provide higher temporal resolution, thus offering greater precision in studying network dynamics and the temporal organization of functional networks. This high temporal resolution can also be used to infer the directionality of information transfer between sources. Additionally, EEG/MEG provides the possibility of identifying interictal epileptiform discharges and analyzing their network properties.
Despite the rich catalog of human structural and functional network organization provided by the aforementioned techniques, each has its limitations. Diffusion MRI fiber tracking may not be accurate in regions with fiber crossings or complex path anatomy (e.g., regions with elongated, remote, and curved projections). Diffusion MRI fiber tracking also cannot determine the exact termination points of fiber bundles within the cortex, which is a key aspect of cortical wiring, nor can it reliably track inter-regional connections of horizontal fibers in vivo, nor determine the direction of connections. Nevertheless, it remains the only non-invasive technique based on tracking axonal projections.
Cortical MRI markers (e.g., cortical thickness) covariance analysis can provide information about networks during brain maturation, but these analyses remain indirect and correlational. If these analyses are conducted at the population level, they offer limited assistance in decision-making for specific patients. Although fMRI functional connectivity analysis can provide consistent networks for individual subjects, it is also correlational, thus this technique is indirect as well. Additionally, fMRI-based analyses require assumptions about the coupling between neural activity and measured hemodynamic signals, which may vary between different regions and may be further altered in brain diseases.
Scalp EEG and MEG techniques offer better temporal resolution but lower spatial precision, which may be limited to sub-lobe levels even with advanced recording and source reconstruction techniques. Since these techniques measure neural signals not directly from the brain but from the scalp, source reconstruction techniques are needed to approximate signals from cortical and (with higher uncertainty) deeper regions. High-density electrode systems and proper sampling of the temporal region have been shown to improve the accuracy of inverse solution algorithms.
Finally, a common challenge across all the aforementioned methods is accurately identifying the brain regions that constitute the networks. These regions may be distinguished at different granularity levels based on various features (e.g., neuroanatomy, function, connectivity, transcriptomic features, cellular structure, or combinations of multiple features). Different brain region atlases have different numbers and sizes of regions, and each atlas may be better suited for specific imaging techniques or research purposes. Additionally, multimodal imaging and electrophysiological modalities are often sensitive to head motion and the influence of processing choices, as well as physiological confounding factors and artifacts. Therefore, these data should be carefully scrutinized to ensure sufficient quality, and appropriate techniques should be employed to minimize the impact of noise and confounding factors on connectome features. While this increases the demand for quality control, the combination of multiple modalities and methods may also help mitigate the limitations of specific techniques.
3 Network Hubs in Healthy Connectomes
Connectomes are system descriptions of brain connectivity, encompassing the connections between a range of brain regions, which can be derived from each of the aforementioned network measurements. Network neuroscience is the field that utilizes graph theory concepts to study brain networks, with graph theory being a branch of mathematics used to formalize the organizational structure of complex systems. In graph theory, brain regions are nodes of the network, connected by edges that are obtained through the aforementioned neuroimaging or neurophysiological measurements (MRI fiber tracking, structural covariance, fMRI, EEG, or MEG). Graph theory allows us to calculate topological parameters such as clustering and path length, which capture local and global network efficiency and provide techniques to decompose the entire brain network into a series of intermediate communities. These metrics collectively quantify complementary topological properties, particularly network integration and segregation, affecting the overall or local efficiency of the graph.
The identification of network hubs utilizes graph theory (Figure 1). More specifically, graph theory can be used to identify the relevance or centrality of individual nodes (i.e., regions) within a network. Centrality metrics aim to rank the nodes in the network based on their hubness or importance in facilitating information flow within the network. Different centrality parameters have been proposed, each emphasizing different aspects of network communication, and thus may yield slightly different rankings of nodes. Previously developed centrality metrics include degree centrality (the total number of connections of a given node), eigenvector centrality (a recursive formula where nodes connected to central nodes receive high centrality scores), and betweenness centrality (prioritizing nodes located on the shortest paths in the network; Figure 1A). Importantly, the choice of specific network metrics should be considered based on the goals and questions of the study. While centrality metrics allow quantifying the interrelated aspects of network organization, they each emphasize different properties of the graph, potentially yielding slightly different hub maps. In this regard, knowledge of community structure in brain networks can be used to classify hubs, determining whether they connect nodes within a given community or serve as connector hubs mediating connections between different communities. Furthermore, certain regions in the brain may be more highly interconnected than expected based on their strength of connectivity, i.e., hubs are interconnected in an enriched club analogy. This architecture implies a densely interconnected core as a trunk for signal transmission, receiving connections from more segregated suppliers and peripheral nodes that maintain more specialized functional roles (Figure 1B).
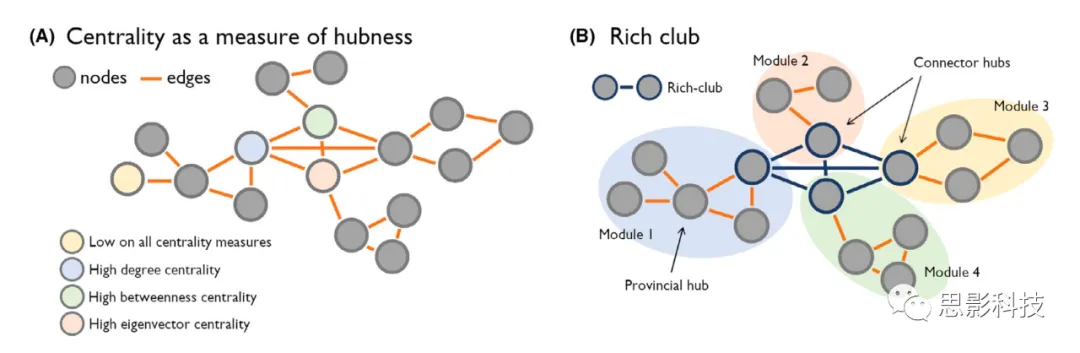
(A) Various methods can be used to characterize network hubs. Notably, hubs are often formalized using topological parameters of networks or graphs, composed of nodes (brain regions) and edges (connections). For example, centrality metrics can be used to identify hub nodes.
(B) On the other hand, an understanding of community structure in the network can be utilized to classify important nodes in the graph, defining them as local hubs or connector hubs. Hubs may form a so-called “enriched club” structure in the graph, where highly connected nodes are densely linked and support integrated information flow across peripheral nodes and modules in the network.
Numerous studies have mapped the hub regions in healthy adults. Although results may vary due to mapping techniques and processing choices (e.g., diffusion MRI fiber tracking vs. resting-state fMRI/EEG/MEG connectivity analysis), high centrality regions are typically identified in the medial and lateral parietal cortices, as well as in the anterior insula and cingulate cortex, occasionally including medial prefrontal regions. Additionally, information transfer directionality measured using EEG (i.e., time-varying Granger causality modeling) indicates that the strongest information flow is from the posterior cingulate to the outside, followed by medial temporal regions and anterior cingulate, leading to global information transfer from posterior to anterior cortical regions. Although sensory and motor networks may also exhibit very high connectivity, particularly with nearby nodes, multimodal nodes more frequently serve as connector hubs, facilitating communication across distant networks. In adults, multimodal networks have also been shown to participate more frequently in enriched clubs,where they preferentially mediate long-distance communication in the brain. In addition to neocortical regions, increasing literature highlights the importance of heteromodal regions such as the hippocampus and subcortical targets (particularly the thalamus) in the overall organization of brain networks, which may serve as important nodes for maintaining integrated brain function and cross-community organization.
Developmental imaging studies emphasize significant changes in the spatial arrangement of brain networks and hub regions over the first few decades of life. For example, studies show that the topography of enriched clubs transitions from more sensory-motor characteristics shortly after birth to more heteromodal/integrative characteristics in adulthood. This process may reflect relative enhancements in long-range connectivity, particularly those connections mediating communication between higher-order systems (e.g., the default mode network) during childhood. Other studies indicate that cross-modal and subcortical hubs may undergo more disruptive changes in connectivity during adolescence, characterized by significant reorganization of their functional connectivity configurations, while sensory and motor regions appear more stable. Complementing these developmental effects, several studies have shown that hub regions may exhibit different microcircuits compared to non-hub regions. For example, heteromodal hub regions typically show greater cortical thickness and lower myelin content, compared to sensory and motor regions that are thinner and heavily myelinated. These findings may reflect increased synaptic density and more complex dendritic arborization in hub regions relative to non-hub regions. This may also relate to higher synaptic plasticity in unimodal regions compared to cross-modal regions with heavy myelination. Hubs have also been shown to exhibit higher metabolic activity, such as increased glucose metabolism. These observations are further complemented by transcriptomic and cellular structure associations, showing that highly connected hubs exhibit tightly coupled transcriptional activity associated with metabolic processes, synaptic activity, and cellular structure similarities. Collectively, these findings underscore potential mechanisms of the different developmental trajectories of hub and non-hub connectivity configurations and contrasting cellular structure and genetic characteristics.
If you are interested in brain networks and brain imaging, please browse the following links from Si Ying (click directly to browse), thank you for your support in sharing.(You can add WeChat ID 19962074063 or 18983979082 for consultation):
The 135th MRI Brain Imaging Basic Class (Guangzhou, 1.20-24)
Nanjing:
The 132nd MRI Brain Imaging Basic Class (Nanjing, 1.8-12)
The 30th Advanced Brain Network Data Processing Class (Nanjing, 1.18-23)
Si Ying Technology Brain Structural MRI (T1) Imaging Data Processing Business
Si Ying Technology Rodent (Mouse and Rat) Neuroimaging Data Processing Business
Si Ying PET Data Analysis Business
Si Ying Magnetic Resonance Spectroscopy (MRS) Data Analysis Business
Si Ying Expression Profiling Analysis Business
Si Ying Technology Deep Learning Radiomics Data Processing
Si Ying Technology Primate Brain Structural Analysis Business (T1 and DWI)
Si Ying Sleep EEG Data Processing Business
Eyepiece Functional MRI Stimulation System Introduction
4 Atypical Network Hubs in Common Epilepsy Syndromes
Focal epilepsy, such as temporal lobe epilepsy (TLE) associated with medial temporal sclerosis or extratemporal epilepsy associated with cortical malformations (e.g., focal cortical dysplasia [FCD]), has traditionally been understood as a “location-related” disease. However, in recent years, focal epilepsy syndromes have undergone significant reconceptualization, emphasizing network pathology. Network mechanisms are now recognized as participating in the generation and propagation of refractory seizures, as well as cognitive and emotional difficulties, and their study may aid in surgical planning and prognosis estimation. Hub reorganization is often accompanied by changes in global network topology, supporting the utility of hub mapping as a compact form to index macro anomalies. Nevertheless, some studies have also pointed out additional benefits in considering the spatial distribution of hubs in terms of sensitivity. For instance, some studies comparing mixed/extratemporal focal epilepsy patients with controls did not identify measurable differences in global network organization but noted atypical hub reorganization. These results may be interpreted as the emergence of potential compensatory mechanisms in patients, wherein overall network topology may be maintained through plasticity mechanisms involving reorganization of node-level connectivity. Connectivity of specific regions may increase to balance the loss of connectivity affecting other regions. However, this possible compensatory contribution of hub reorganization remains to be studied, and other perspectives should also be considered, as changes in hub connectivity may reflect the pathological effects of seizure activity. Given their central role in large-scale connectome organization, hubs thus appear to be viable candidates for better understanding, diagnosing, and treating focal epilepsy.
Various methods have been implemented to study network hub changes associated with epilepsy, partly because hubs may be formalized in different ways. As mentioned above, quantifying hubs using centrality metrics allows researchers to investigate relative differences in node-level connectivity between patients and controls. Different thresholding techniques can be applied to node data, enabling comparisons of hub topographies across different groups. From this perspective, studies identifying changes in global metrics of network efficiency associated with epilepsy, such as overall path length and clustering, are also considered to reflect changes in the patterns and properties of hub connectivity, given the critical role these nodes play in facilitating efficient network communication. Although several studies have investigated regional connectivity changes in epilepsy, these findings have not been systematically embedded within the context of quantifying the large-scale network efficiency or properties of integration and segregation. In these cases, it remains unclear how the observed changes in connectivity impact the hubness and communication efficiency of a given node. While some studies may be discussed, particularly when changes in connectivity are found in established communication hubs in healthy connectomes (e.g., in nodes of multimodal associative networks, such as the default mode and fronto-parietal systems, as well as marginal brain regions), this article focuses on studies highlighting group differences, including local hubness and more global network efficiency. Given the heterogeneous modalities and methods applied in the reviewed studies to map brain networks and hubs, we present the findings organized by epilepsy syndromes and imaging modalities while briefly summarizing the general methods and main results of each study.
Previous studies using different imaging modalities have shown that the hub topography of refractory focal epilepsy patients differs compared to healthy controls. In patients with the most common refractory epilepsy in adults—TLE—cortical thickness and volume covariance analyses reported atypical spatial distributions of hubs compared to controls, accompanied by overall increases in network path length and clustering (Figure 2A). The overall increase in network path length indicates a reduction in global efficiency, which in turn may affect the overall signal transmission process in TLE. Diffusion MRI studies of TLE have shown widespread reductions in regional efficiency affecting the ipsilateral temporal region, bilateral frontal regions, and bilateral parietal regions. Hubs identified in healthy connectomes, such as the left precuneus, also exhibited reduced node efficiency. A high-angular-resolution fiber tracking study revealed changes in global and regional connectivity, along with hub structural abnormalities, with right TLE being more pronounced than left TLE. Microstructural analyses in the same study indicated that changes in axonal density were stronger than changes in fiber orientation, providing a potential microstructural basis for the connectivity changes observed in that study. Utilizing network controllability analysis, a simulation-based method to detect dynamic functional properties of structural connectomes, previous studies showed significantly reduced controllability in bilateral default mode and more ipsilateral medial temporal regions, particularly in patients with pronounced hippocampal structural abnormalities (Figure 2B). Collectively, these findings suggest the presence of global and regional structural network changes in TLE, with changes in local hubness associated with global and local network efficiency.
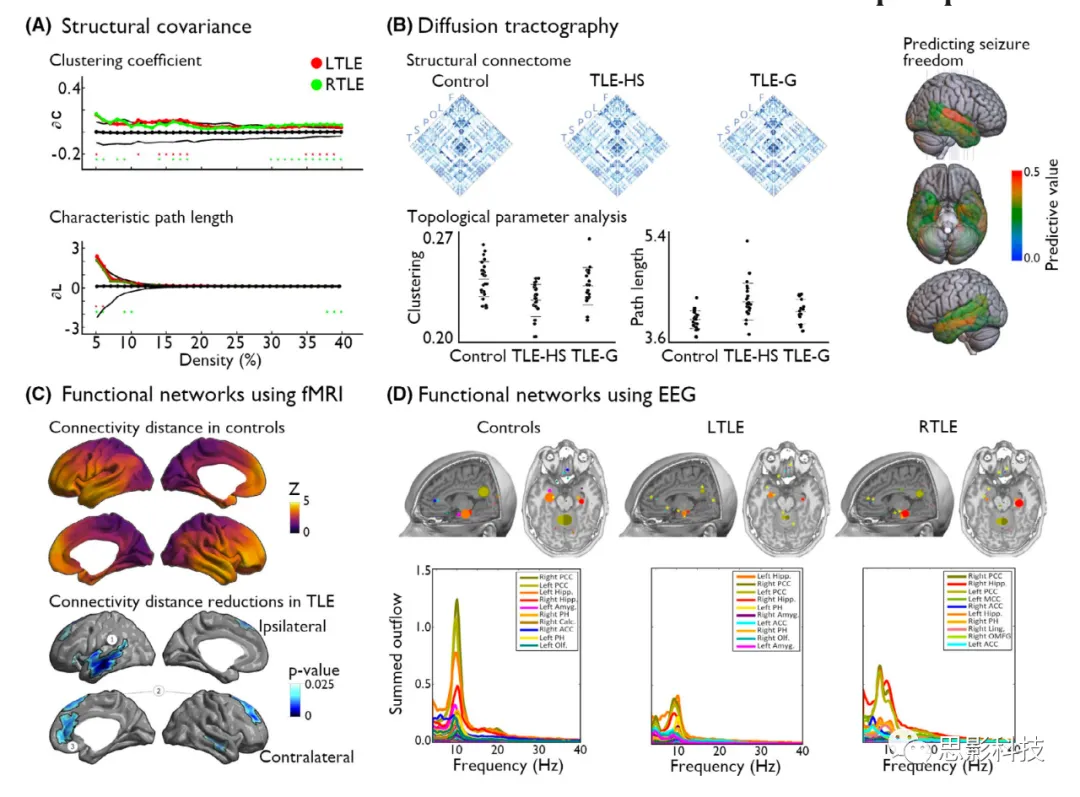
Figure 2 Altered Network Topology in Temporal Lobe Epilepsy (TLE).
(A) In TLE, the structural covariance network based on cortical thickness measurements highlights an overall reduction in network communication efficiency, characterized by increased clustering and path lengths, along with changes in local hub distributions, in left TLE (LTLE; red) and right TLE (RTLE; green). The black line shows the mean and 95% confidence interval of the zero distribution obtained from 1000 permutation tests (shown only for the LTLE group).
(B) Studies using diffusion fiber tracking have investigated changes in hubs in epilepsy using both global and more local hubness metrics. A structural connectome study based on diffusion fiber tracking showed abnormal network topology in TLE patients with hippocampal sclerosis (TLE-HS) and those with only glial proliferation (TLE-G) compared to controls. However, changes in network efficiency, characterized by reduced clustering and increased path lengths, were significant in patients with pronounced HS (hippocampal sclerosis) and subtle in those with only glial changes in the hippocampus. Additionally, node hubness metrics derived from diffusion fiber tracking have shown potential as individualized biomarkers for surgical prognosis. In particular, this study revealed the importance of betweenness centrality in the hippocampus, superior temporal gyrus, and entorhinal cortex in predicting seizure-free outcomes in TLE patients post-surgery.
(C) Disruptions in the organization of functional networks reflecting changes in local hub properties can also be observed in TLE compared to controls. A recent study showed a reduction in physical distances of functional connectivity in TLE, affecting the ipsilateral temporal-limbic and contralateral medial and lateral frontal regions. In subsequent analyses, the reduction in connection distances was greatest in enriched club nodes, which are highly interconnected and play hub roles in the network.
(D) Utilizing the temporal precision of high-density EEG, previous studies reported network reconfigurations affecting the intensity-driven nodes of information flow in TLE compared to controls. Specifically, it was found that the strongest outflow of information occurred in the ipsilateral hippocampus in patients, rather than in the posterior cingulate cortex observed in controls, indicating relative changes in inter-group hubness.
ACC, anterior cingulate cortex; Amyg, amygdala; Calc, calcified cortex; fMRI, functional magnetic resonance imaging; Hipp, hippocampus; Ling, lingual gyrus; MCC, medial cingulate cortex; Olf, olfactory cortex; OMFG, orbitofrontal gyrus; PCC, posterior cingulate cortex; PH, parahippocampal gyrus.
In temporal lobe epilepsy (TLE), the atypical organization of structural connectome hubs has been complemented by functional studies in TLE. Resting-state fMRI studies have shown disruptions in hubs of medial temporal regions and default mode networks, including the emergence and disappearance of hubs compared to healthy controls, as well as some differences in hub topography between left and right TLE patients. A study investigating dynamic and directional network changes during interictal spikes found asymmetric effects in TLE, with right TLE exhibiting more bilateral and extratemporal outflow changes. Other studies have shown reduced functional connectivity between anterior and posterior default mode hubs or disruptions of default mode connectivity at both structural and functional levels. Additionally, studies have shown increased functional connectivity within the marginal medial temporal region during fMRI, while reduced connectivity of distal cortical networks. These findings are consistent with the “normalization” of networks in TLE patients, showing increased local connectivity and disruptions in remote connectivity associated with increased local segregation and decreased overall network integration. A recent analysis highlighted similar findings, outlining the distance of functional connectivity patterns, noting reduced remote connections of distributed cortical networks and increased local connectivity in the temporal-limbic circuits adjacent to the seizure focus. Interestingly, this study also noted that functional network reconfigurations did not strongly covary with gray matter atrophy. Rather, the findings indicated that the contraction of functional connectivity reflected potential microstructural changes in white matter. EEG studies have also shown significant network changes in epilepsy, even in the absence of interictal spikes during recordings, which may reflect deep connectivity changes not visible on the scalp, supported by intracranial EEG studies. In TLE, the major outflow from the ipsilateral medial temporal structures seems to replace the major posterior cingulate outflow seen in healthy subjects. Complementing the findings of reduced structural network efficiency, studies of altered functional connectivity in TLE highlight the disrupted hub topography of patients. Notably, significant connectivity changes can be found in the temporal-limbic disease center, accompanied by disruptions of remote connectivity in extratemporal regions, a characteristic of connectome hubs.
In other focal epilepsy syndromes, some studies have also proposed atypical connectome organization and hub topographies. In temporal lobe epilepsy (TLE) and extratemporal epilepsy, resting-state network analyses have shown increased efficiency in somatosensory, ventral attention, and default mode networks. Studies related to extratemporal epilepsy associated with cortical developmental malformations have previously supported the normalization of structural covariance networks and functional randomization, indicating modality-specific transitions in network integration and segregation. Although the changes in structural and functional network topology differ, both indicate that changes in local hub properties affect the overall network efficiency of these patients. Interestingly, the authors observed that the findings of large malformations were characterized by atypical cortical organization (as seen in multiple microgyria and focal cortical dysplasia [FCD] type I), closely related to intermediate findings associated with atypical cortical migrations (heterotopia), and subtle findings in FCD type II, a type of lesion indicating atypical glial proliferation. In patients with FCD type II lesions, another study utilized connectome fingerprinting to perform in vivo lesion tissue biotyping based on resting-state fMRI analyses, focusing on the functional connectivity of lesion patches with their primary functional communities. Besides showing variable functional connectivity configurations of FCD type II lesions, this study also demonstrated associations between functional connectome embedding and postoperative outcomes and histopathological grading. In particular, patients with more disconnected lesions (i.e., reduced functional connectivity within and between communities) were more frequently observed in the default mode network, which is a functional community containing several typical hub nodes in healthy connectomes. Therefore, despite the heterogeneity of the pathophysiology of cortical developmental malformations, previous studies indicate consistency in the disruption of network topology associated with the developmental stage at which these malformations occur.
In idiopathic/genetic generalized epilepsy, a functional connectivity study using fMRI observed atypical distributions of modular degree and participation coefficients, which characterize local and connector hubs, respectively. The findings in patients pointed in opposite directions in thalamic and cortical subregions, with thalamic connectivity configurations being more constrained, while cortical inter-network organization was more dispersed compared to controls. The overall degree of hub changes in patients was found to correlate with the level of drug-related seizure control, with abnormalities being more pronounced in patients who experienced seizure recurrence upon follow-up. Moreover, these changes were already visible shortly after the diagnosis of these patients, indicating an inherent functional imbalance in the thalamocortical networks of these patients. Early reports indicated complex patterns of changes in node efficiency in idiopathic/genetic generalized epilepsy compared to controls, suggesting that the structural-functional relationships in this condition may be decoupled. These results are complemented by a recent report showing changes in diffusion MRI, fMRI, and microcircuits in idiopathic/genetic generalized epilepsy, indicating a reconfiguration of thalamocortical networks affecting multiple organizational levels of the brain. Although the changes in idiopathic/genetic generalized epilepsy syndromes are generally subtler than those in TLE, they remain measurable compared to healthy controls. In untreated children during absence seizures, magnetoencephalography (MEG) studies identified pathological hubs in the thalamus, precuneus, and cingulate cortex. These findings contribute to understanding the rapid involvement of cortical and subcortical regions during absence seizures. Recently, multi-layer network analyses of absence seizures based on simultaneous EEG-fMRI and MEG revealed strong cross-frequency and within-frequency coupling in the prefrontal cortex and anterior precuneus regions, with a major hub cluster in the left prefrontal cortex. These findings highlight significant structural and functional changes in idiopathic/genetic generalized epilepsy, characterized by unique network alterations in cortical and thalamic network organization.
Understanding the spatial topography of hubs may also highlight the impact of epilepsy on the brain. In neurodegenerative and psychiatric diseases, previous studies have shown that highly interconnected hubs typically exhibit greater gray matter atrophy compared to more locally connected peripheral nodes. This increased sensitivity to structural damage is thought to stem from the heightened metabolic activity and plasticity of brain hubs, as well as their intrinsic associations with multiple brain networks. Recent multi-site studies from the ENIGMA-Epilepsy consortium have associated normative maps of functional and structural connectome hubs with patterns of gray matter atrophy plotted in hundreds of epilepsy patients relative to healthy controls. The study observed significant atrophy in hub regions compared to non-hub regions, confirming their sensitivity to structural damage related to epilepsy. The study also highlighted differences between temporal lobe epilepsy and idiopathic/genetic generalized epilepsy, noting that the former’s patient group was more closely related to cortical hubs, while the latter’s pathological findings were better co-localized with subcortical-cortical hubs, possibly emphasizing different pathophysiological bases in focal vs. generalized epilepsy.
5 Hub Mapping as a Clinical Tool
Some studies have also explored the value of network neuroscience approaches in providing clinically relevant metrics related to epilepsy, primarily involving localization of cognitive phenotypes, localization of seizure onset zones, and prediction of postoperative outcomes. A previous MRI covariance analysis observed different hub topographies indexed by betweenness centrality in TLE patients with varying degrees of cognitive impairment. Even in patients without cognitive impairment based on standardized neuropsychological tests, differences in hub spatial distribution were observed compared to controls. Using diffusion MRI fiber tracking, several studies identified associations between language and memory impairments and white matter network organization, particularly regarding more global measures of network efficiency. Interestingly, reduced local efficiency of language networks was observed only in TLE patients with limited language function, particularly in the superior temporal gyrus. A previous study identified associations between network topological metrics (including clustering and path length, as well as node degree centrality) and multidimensional cognitive phenotypes in TLE. The study found that patients with increased path lengths, reduced clustering, and reduced connectivity exhibited more pronounced cognitive impairments in behavioral measures of performance. In particular, network-based metrics capturing global and local efficiency appear promising for better understanding functional impairments in TLE.
A series of studies have also emphasized the involvement of hubs in the clinical manifestations of epilepsy electrophysiology. A recent study combining intracranial EEG investigations with diffusion MRI connectomics suggested the role of white matter fiber bundles in the spatiotemporal evolution of seizures. Meanwhile, a series of studies explored the extent to which hubs and network properties might provide useful metrics to outline surgical targets. A previous approach developed a new tool for identifying brain regions that facilitate the synchronization of epilepsy networks by identifying regions that may play a role in regulating the propagation of seizures. However, a recent study examining hemisphere networks based on resting-state MEG found different types of hub changes in left vs. right focal epilepsy patients when comparing different centrality metrics (i.e., betweenness centrality, eigenvector centrality, node efficiency, and clustering coefficient). These findings underscore the importance of selecting and comparing appropriate metrics to study changes in network organization related to epilepsy, as well as their limitations for potential clinical use. By providing insights into atypical network organization in focal epilepsy, these studies may ultimately improve surgical prognosis in the context of cognitive and emotional function as well as seizure control.
Numerous studies indicate that preoperative metrics of brain network organization and hubs are associated with postoperative outcomes. Overall, findings from fMRI, structural MRI, diffusion MRI, and EEG/MEG may support the topological isolation of surgical targets in patients with favorable seizure-free outcomes compared to those with poor postoperative seizure outcomes. In a network analysis based on EEG, lower spike-related network integration quantified using global network efficiency metrics was associated with postoperative seizure freedom in patients with TLE and extratemporal epilepsy. In this study, the pathological activity primarily observed in patients with ongoing seizures was characterized by the propagation of pathological activity through the overall higher efficiency of the entire brain. Resting-state MEG connectivity patterns have also been shown to differ between seizure-free and seizure patients. The former subgroup typically exhibited weaker connectivity with the regions generating interictal epileptiform discharge compared to the rest of the brain, relative to non-seizure patients. The differences were primarily located in the alpha band and were mainly mediated by remote connectivity differences. Therefore, EEG/MEG studies seem to emphasize that less widespread epilepsy networks are associated with better outcomes, supporting reduced “propagation.”
In a series of diffusion MRI fiber tracking studies, preoperative structural connectome and hub metrics combined with machine learning techniques have been used to predict seizure outcomes after epilepsy surgery. In particular, a multi-site study showed that nodes contributing most strongly to models predicting seizure outcomes from local metrics of betweenness centrality involved bilateral parahippocampal gyri and superior temporal gyri. This study indicated that patients presenting atypical integrative network regions are less likely to achieve seizure freedom. Other node-level topological metrics, such as node strength and clustering coefficient, did not reflect the predictive accuracy of metrics of network integration. Nodes beyond the parahippocampal region were also associated with surgical outcomes. For example, a previous resting-state functional connectivity study emphasized that thalamic hubness may also be related to outcomes. This study found that patients with ongoing seizures post-surgery exhibited higher degree and eigenvector centrality in bilateral thalami compared to seizure-free patients. In the same condition, thalamic network involvement was also found to be associated with the transition from focal to bilateral seizures. Thus, thalamic network involvement may be critical for seizure control and cognition in TLE. Another study combining preoperative MEG and postoperative structural imaging showed an association between the removal of hubs and surgical outcomes. In this study, patients who became seizure-free post-surgery removed more hubs than those who experienced seizure recurrence, a finding consistently observed across a randomly selected series of time periods. Broadband MEG studies have also suggested the effects of hub removal, although subsequent studies using multiple network metrics have not found clear associations with surgical outcomes. Another study assessed changes from preoperative to postoperative, investigating the impact of TLE surgery on resting-state fMRI connectivity, revealing reconfigurations of multiple network integration metrics post-surgery in TLE patients, with these findings moderated by the type of resection and seizure outcomes. Hub mapping may also be useful for patients with refractory epilepsy unsuitable for surgery, such as in the context of neuromodulation therapies. This treatment shows promise in the thalamic cluster stimulation for idiopathic/genetic generalized epilepsy and may potentially serve as a possible treatment method for addressing epilepsy-related memory deficits in the future. Collectively, these findings emphasize the importance of considering network configurations, particularly the involvement of hub nodes in epilepsy networks and achieving seizure freedom.
Furthermore, the high temporal resolution of electrophysiological studies and the increasing availability of long-term EEG recordings provide the ability to capture seizures and investigate transient changes in network properties during the generation and evolution of seizures. Scalp EEG contains rich data on seizures, but due to the typically short recordings collected using these modalities, seizures are rarely recorded with fMRI or MEG. Localizing seizure sources also becomes challenging due to dynamic patterns and frequent seizure-related artifacts. Early work indicated that localizing network nodes with the strongest outflow of information was consistent with seizure source lesions or single-photon emission computed tomography images of seizures, although without invasive validation. However, more studies with different postoperative outcomes and more extratemporal epilepsy patients are needed to confirm this promising network-based localization and outcome prediction tool.
6 Conclusion and Future Perspectives
Researching network mechanisms in epilepsy holds promise for advancing diagnosis, prognosis, and clinical decision-making. However, networks are inherently complex and high-dimensional, challenging their interpretation and clinical translation. Therefore, it is necessary to summarize methods for regional and global parameterization of networks, with hub studies facilitating quantifiable assessments of spatial graphs and node features. Additionally, the identification and measurement of hubs can promote reproducibility and comparison among various neuroscience methods (e.g., MRI fiber tracking, structural covariance, fMRI, EEG, and MEG). Although network neuroscience provides a rich array of different hub forms, including various network centrality and core-periphery organization concepts, it remains to be determined which approaches offer practical advantages and effectiveness in epilepsy research and treatment outcome measurements. Evaluating the specificity and sensitivity of different hub mapping tools across different cohorts in imaging modalities, including their optimal combinations, is needed to clarify the added value of establishing these methods in clinical settings. Although there is currently a lack of such datasets in the epilepsy field to select the best modalities or hub mapping techniques, the current literature recommends positioning network hubs as markers of disease and interpreting factors influencing clinical trajectories in epilepsy, including seizure control and cognition. As emphasized, hub mapping has revealed network changes in several epilepsy syndromes, aiding in understanding the mechanisms of seizure propagation and providing markers for postoperative seizure recurrence in refractory patients. Explorations at the connectome level and targeted investigations of brain network hubs will benefit from increasingly available datasets that provide comprehensive neuroimaging, connectomics, electrophysiological, and clinical phenotype data from the same participants. Notably, routinely acquired imaging and electrophysiological data in clinical settings are expected to facilitate multi-site data aggregation, supporting future assessments of the predictive value of network and hub mapping tools in epilepsy research. These are exciting developments in the field of epilepsy, requiring key steps in the future to verify, integrate, and translate these advances into clinical decision-making.
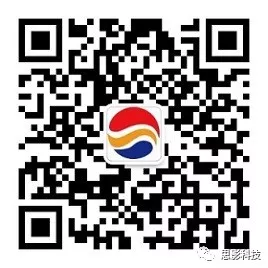
Welcome to browse Si Ying’s data processing business and course introduction. (Please click directly on the text below to browse all courses of Si Ying Technology. You are welcome to add WeChat ID:19962074063or18983979082 for consultation. After registration, we will contact you promptly and reserve spots for registered students):
Nuclear Magnetic:
Nanjing:
The 30th Advanced Brain Network Data Processing Class (Nanjing, 1.18-23)
The 135th MRI Brain Imaging Basic Class (Guangzhou, 1.20-24)
EEG and Infrared, Eye Movement:
Chongqing:
The 9th EEG Connectivity and Network Class (Guangzhou, 2.10-14)
The 58th EEG Data Processing Introductory Class (Nanjing, 2.9-13)
The 59th EEG Data Processing Introductory Class (Beijing, 2.16-20)
The 14th EEG Machine Learning Class (Matlab Version, Shanghai, 2.16-20)
Data Processing Business Introduction:
Si Ying Technology Brain Structural MRI (T1) Imaging Data Processing Business
Si Ying Technology Rodent (Mouse and Rat) Neuroimaging Data Processing Business
Si Ying PET Data Analysis Business
Si Ying Magnetic Resonance Spectroscopy (MRS) Data Analysis Business
Si Ying Expression Profiling Analysis Business
Si Ying Technology Deep Learning Radiomics Data Processing
Si Ying Technology Primate Brain Structural Analysis Business (T1 and DWI)
Si Ying Sleep EEG Data Processing Business
Eyepiece Functional MRI Stimulation System Introduction