▲Click on the above Leiphone to follow
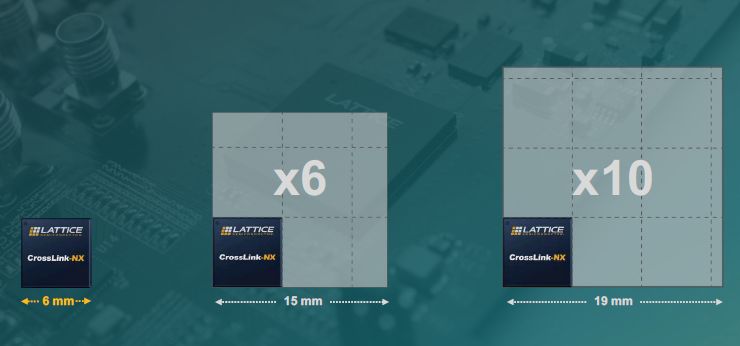
For FPGA to be applied in edge AI computing, high cost and development difficulty are two issues.
Written by | Bao Yonggang
A new wave of AI has placed higher demands on chips, but there is still no strict and recognized standard for AI chips. Therefore, CPUs, GPUs, FPGAs, and ASICs that can run deep learning algorithms can all be called AI chips. Although they are all called AI chips, in the context of AI’s landing in 2019, the efficiency of AI chips is more worth paying attention to.
So, at the edge, can FPGAs drive the popularization of AI together with ASICs designed specifically for edge AI?
After mergers and integrations, the top three FPGA suppliers globally are Xilinx, Intel, and Lattice. Interestingly, the top two suppliers launched the “largest” FPGA one after another this year. In August, Xilinx announced the launch of the world’s largest capacity FPGA, the Virtex UltraScale+ VU19P, which is based on TSMC’s 16nm process, integrating 35 billion transistors, 9 million system logic units, with a DDR4 memory bandwidth of up to 1.5 Terabits per second, a transceiver bandwidth of up to 4.5 Terabits per second, and more than 2000 user I/Os.
Three months later, in November, Intel announced the launch of the world’s largest capacity FPGA, the Stratix 10 GX 10M, manufactured using a 14nm process, integrating 44.3 billion transistors, with a core area of about 1400 square millimeters, having 10.2 million logic units in a package area of 70×74 millimeters, along with 25,920 data interface buses (EMIB), more than twice the previous record, each interface throughput is 2Gbps, internal total bandwidth is 6.5TB/s, with 308Mb storage, 6912 DSPs (18×19 arrangement), 2304 user I/O pins, and 48 transceivers (0.84Tb/s bandwidth).
Clearly, the introduction of larger capacity FPGAs by Xilinx and Intel is aimed at meeting the demands of big data and cloud AI, expanding the application of FPGAs from industrial and automotive sectors to data centers. In the semiconductor industry, the head effect is particularly evident; even if ranked third in market share, if Lattice also launches large-capacity FPGAs, the situation could be very difficult.
The adjustments made by Xilinx and Intel in the FPGA market have also given Lattice opportunities for market expansion. In recent years, Lattice has focused on the consumer market, but the consumer market evolves very rapidly and requires corresponding products to be launched based on different market demands.
The Director of Product Marketing for Lattice Asia Pacific, Chen Yingren, said: “When market demand is unclear and changes rapidly, it is difficult to reuse technology. We are now adopting a new platform-based model, which maximizes design reuse, lowers development costs, and accelerates product iteration.”
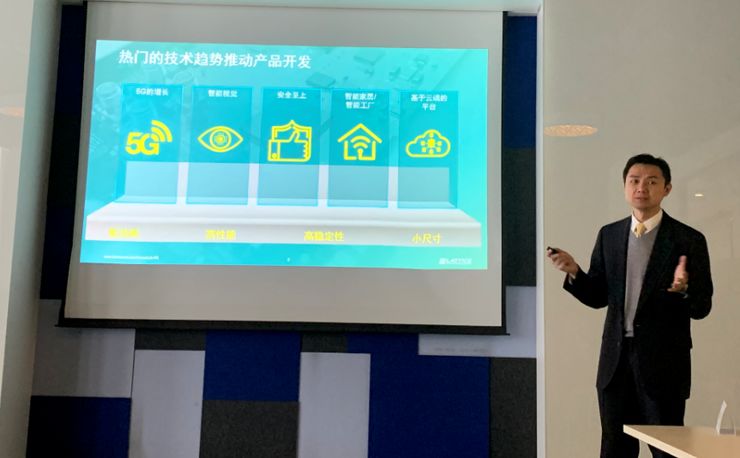
From this perspective, Lattice has found a suitable positioning and product route amid market changes. However, for FPGA to be applied in edge AI computing, high costs and development difficulties are two issues. In this regard, Chen Yingren stated that many customers are using our FPGAs for mass production, so our FPGA prices are very friendly. In addition, over the past four years, the number of FPGAs shipped by Lattice has reached the level of 1 billion pieces, which also proves that our products are very reliable.
As for the design threshold, Chen Yingren pointed out that since the FPGA ecosystem is not as convenient as C language, many people feel that the design threshold for FPGAs is relatively high. This can again be divided into two parts; one part is tools, Lattice’s newly launched platform CrossLink-NX will use Radiant 2.0, the debugging tools have made significant improvements, support industry-standard SDC, added ECO compiler, and signal integrity analysis, and will continue to be updated to make it easier for engineers who understand FPGAs to use.
The other part is reference designs, for those engineers who are not very familiar with FPGAs, they do not know the differences between these tools and need some reference designs to get started more easily, which is also Lattice’s strength. Additionally, the sensAI we launched includes a modular hardware platform, demonstration examples, reference designs, neural network IP cores, software development tools, and custom design services necessary for evaluating, developing, and deploying FPGA-based machine learning and AI solutions. All these can lower the design threshold for FPGAs.
Furthermore, FPGA itself is parallel processing, and many deep learning algorithms require parallel processing. By converting AI models through sensAI, executing AI inference on FPGA is very suitable.
With friendly prices and lowering design thresholds, for FPGA to be applied at the edge, it still needs to have a significant advantage over ASICs to be attractive. In this regard, Chen Yingren told Leiphone: “We focus on maximizing the advantages of FPGAs, such as low latency. Another advantage is that FPGAs can be quickly brought to market. Moreover, FPGAs are flexible due to their inherent programmability, with I/O and configurations being very flexible. The flexibility of the I/O interfaces is very suitable for the edge, allowing the connection of various sensors.”
He further explained the importance of flexibility at the edge; if measured from the perspective of performance and power consumption ratio, FPGAs may not seem advantageous compared to ASICs. However, FPGAs can provide different interfaces, which allows them to connect to different sensors and meet the needs of product intelligent upgrades. Especially since AI technology is continuously evolving, when upgrading existing products, the topology of ASICs is already fixed, and when an electronic billboard wants to add a sensor to better meet the needs, ASICs can be very difficult, while FPGAs can easily connect using either SPI or USB interfaces.
Since FPGAs are changing to meet the needs of edge AI in terms of cost, performance, and development threshold, what kind of FPGA can better meet the needs of edge AI? Lattice’s answer is the previously mentioned CrossLink-NX. CrossLink-NX is based on Lattice’s NEXUS platform, featuring a 75% reduction in power consumption, a 100-fold increase in reliability, minimum size, and high-performance network edge computing capabilities.
Specifically, CrossLink-NX has 192 programmable I/Os, D-PHY speeds of 2.5Gbps, and 40K logic units, mainly used for video bridge processing.
The realization of low power consumption is due to Lattice using Samsung’s 28nm FD-SOI process in CrossLink-NX. Chen Yingren explained that the process we selected has a very thin Buried Oxide, which can reduce failure rates by 100 times, thus increasing reliability by 100 times, and the static power consumption can be reduced by up to 75% compared to competitors.
In terms of performance, CrossLink-NX offers 17K-40K logic units, providing low power consumption and high-performance modes, and has added a lot of embedded storage RAM, along with DSP modules, allowing CrossLink-NX to achieve an embedded storage/logic ratio of 170 bits, which is several times higher than competitors’ products, making it more effective in processing graphics and AI inference.
While achieving a high storage/logic ratio, CrossLink-NX also realizes a small size, using an optimized 4-input lookup table to allow its product to achieve a small size, with 40K logic units on a 6x6mm area. Chen Yingren believes that a 4-input lookup table is sufficient for current and future products because we are pursuing not the highest performance, but low power consumption and the resulting cost and convenience.
Of course, what makes CrossLink-NX more suitable for edge AI is also the ultra-fast I/O startup and fast I/O transmission. In terms of transmission speed, Lattice uses hard-core I/O to improve the rate, with MIPI D-PHY speed increased from the previous 1.5Gbps to 2.5Gbps. Chen Yingren stated that most SoCs now have a speed of 2.5Gbps, so our FPGAs can more easily cooperate with SoCs to meet the needs of embedded vision and edge AI.
In terms of startup speed, the I/O configuration speed of CrossLink-NX is less than 3ms, and device configuration is less than 8ms. This speed is not only much faster than MCUs or SoCs, but also stabilizes the system by configuring the I/O first and fixing the voltage.
Leiphone (public account: Leiphone) learned that CrossLink-NX will initially support industrial-grade temperatures and will undergo automotive certification. Currently, more than 30 customers have begun trial use of CrossLink-NX, and the supply time is even ahead of expectations.
Chen Yingren also stated that software and IP are also ready, and in the future, sensAI will also support CrossLink-NX. For AI applications, we may also cooperate with third parties to provide a one-stop solution, allowing customers who do not understand FPGAs and AI algorithms to apply them more easily.
Edge AI and the AI market have enormous potential. When AI places higher demands on chip computing power, all chip providers do not want to miss the opportunities brought by AI. FPGA companies are no exception, but FPGAs are inherently better at signal processing and network acceleration. However, to exert greater value in the era of big data AI, the top two FPGA companies have launched larger-scale FPGAs to better meet the cloud AI market. This gives Lattice a great opportunity to maximize technology reuse through a platform-based approach while stepping into the industrial and automotive markets.
To better seize this opportunity, Lattice’s new products optimize performance, stability, and I/O in various aspects, leveraging the low latency and high flexibility characteristics of FPGAs in edge AI to meet edge AI computing needs as much as possible. For the market, increasing a competitive option is certainly a good thing; as for how the market will accept it, we will continue to pay attention.
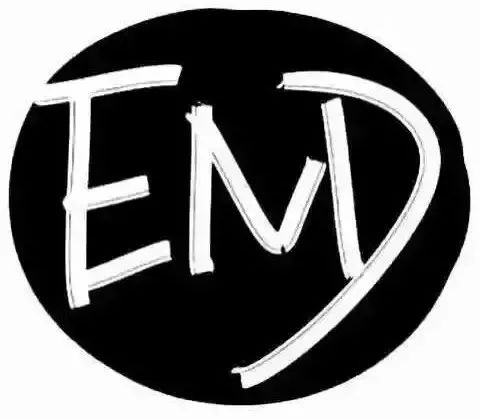
Previous Recommendations
100,000 AI talents vote for you, companies scan the code to sign up

Leave a Comment
Your email address will not be published. Required fields are marked *