To enhance the effectiveness of targeted mining of sensor network sample data, a method based on IoT technology for targeted mining of sensor network sample data is proposed. A data processing platform based on IoT technology is designed, including IoT nodes, edge data aggregation devices, and the DAE-RNI model. The DAE-RNI model optimizes the incomplete data filling algorithm based on Mahalanobis distance to achieve filling of incomplete data in sensor network samples.
The DAE-RNI model is a data compression and reconstruction architecture consisting of a data preprocessing module, an encoder, and a decoder. The data preprocessing module is responsible for data filling and mining, while the decoder and encoder are responsible for the encoding output of the data, each consisting of two fully connected layers. The two fully connected layers of the encoder are represented as fully connected layer 1 and fully connected layer 2, responsible for encoding the data to obtain effective low-dimensional representations; the two fully connected layers of the decoder are represented as fully connected layer 3 and fully connected layer 4, responsible for decoding the data to map the hidden layer representation in low-dimensional space to high-dimensional space, thus obtaining reconstruction results closest to the original data.
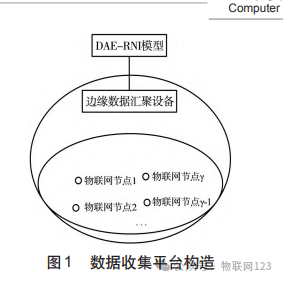
Experiment 1:Results of Targeted Mining Root Mean Square Error TestThe root mean square error of targeted mining is tested for the IoT technology mining method, long short-term memory network mining method, and TF-IDF targeted mining method, where the test results of the designed method are the root mean square error of targeted mining.
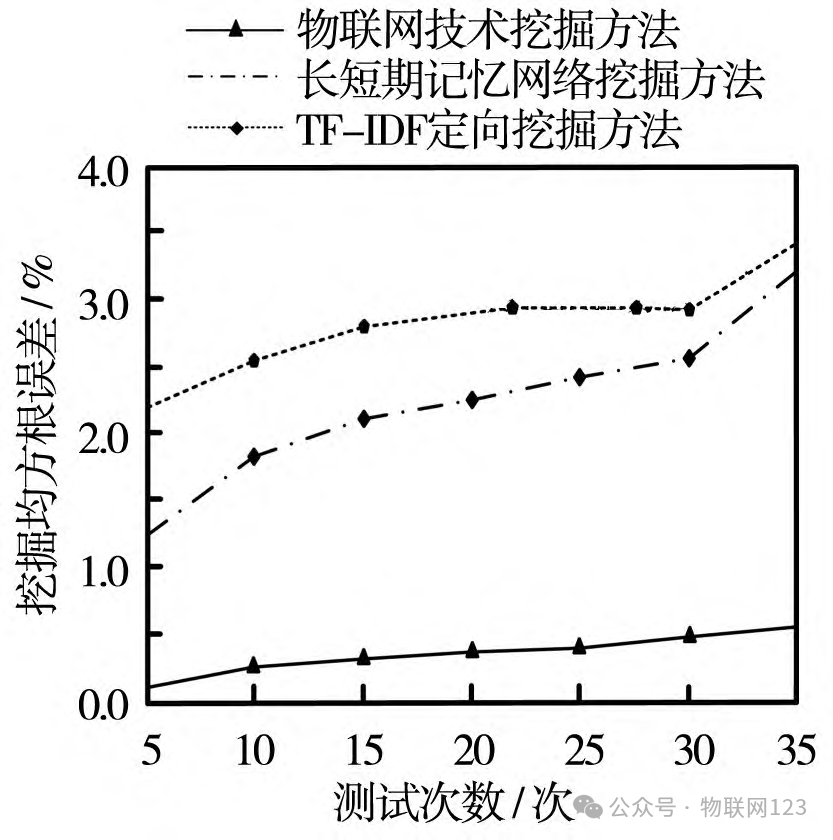
Experiment 2:Results of Targeted Mining Mean Absolute Error TestThe mean absolute error of the three methods is tested, where the test results of the designed method are the mean absolute error of targeted mining.
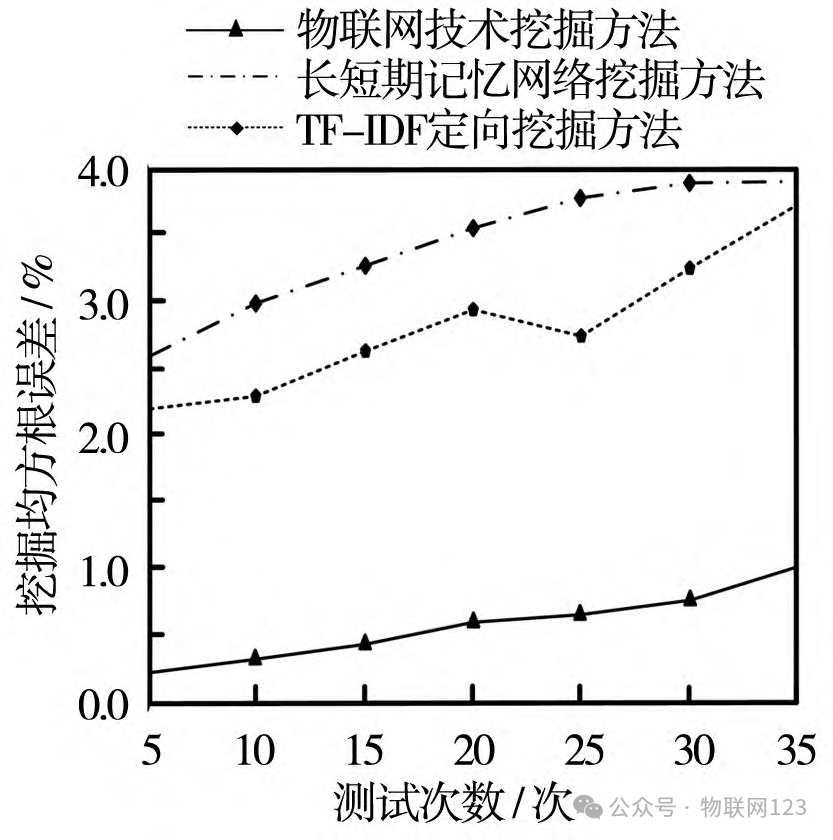
Results of Experiment 1:The root mean square error of targeted mining using the IoT technology mining method is overall below 0.5%, while the root mean square errors of the long short-term memory network mining method and TF-IDF targeted mining method are above 2.0% and 1.0%, respectively, indicating that the designed method has a higher mining accuracy and better mining performance.
Results of Experiment 2:The mean absolute error of targeted mining using the IoT technology mining method is overall below1.0%, while the mean absolute errors of the long short-term memory network mining method and TF-IDF targeted mining method are above 2.0% and 2.5%, respectively, which also indicates that the designed method has a higher mining accuracy and better mining performance.
5. Results
The new big data parallel classification system for work order labels utilizes multiple hardware devices such as big data collectors and parallel processors, defining a standard information entropy sequence, and optimizes parallel parameters based on the variational modal decomposition results of the data information. From a practical perspective, under the influence of this new classification system, the mutual interference of big data information during the scheduling process of business work orders has been effectively suppressed, enabling accurate on-demand classification of information parameters.
References:
Disclaimer: The images and text used in this article are reprinted. If there are any copyright issues, please inform us immediately, and we will confirm and delete the content based on the proof materials you provide. The content of this article reflects the author’s personal views and does not represent the views or positions of IoT 123. |