Introduction:
For interdisciplinary fields, a rough overview is just the first step through the door.

The camera is a core sensor for ADAS, and compared to millimeter-wave radar and laser radar, its greatest advantage lies in recognition (whether an object is a car or a person, what color a sign is). The automotive industry is price-sensitive, and the hardware cost of cameras is relatively low. With the rapid development of computer vision in recent years, the number of startups entering ADAS perception from the camera perspective is quite considerable.
These startups can be collectively referred to as vision solution providers. They possess core visual sensor algorithms and provide a complete set of solutions, including onboard camera modules, chips, and software algorithms, to downstream customers. In the OEM mode, vision solution providers act as secondary suppliers, collaborating with Tier 1 suppliers to define products for OEMs. In the aftermarket, besides providing a complete set of equipment, there is also a model for selling algorithms. This article will analyze the functions of visual ADAS, hardware requirements, evaluation standards, etc., while the content of “【Cheyun Report】 Introduction to ADAS Vision Solutions (Part 2)” will refer to Mobileye’s detailed interpretation of products from 11 domestic suppliers.
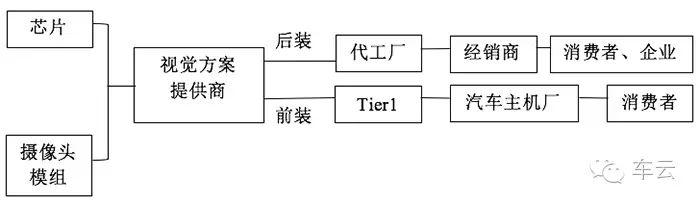
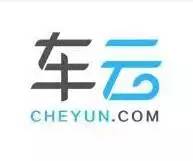
1. Functions Achievable by Visual ADAS
Due to safety records and parking needs, cameras are widely used in vehicles for functions like dash cams and reverse imaging. Generally, wide-angle cameras installed at various positions on the vehicle collect images, which are calibrated and processed by algorithms to generate images or stitch them together to supplement the driver’s visual blind spots, without involving vehicle control, thus focusing more on video processing. The technology has matured and is gradually becoming widespread.
Currently, in driving assistance functions, cameras can independently achieve many functions and gradually evolve according to the development of autonomous driving.
These functions emphasize the processing of input images, extracting valid target motion information from the captured video stream for further analysis, providing warning information, or directly activating control mechanisms. Compared to video output functions, which emphasize real-time performance at high speeds, this part of the technology is in a period of rapid development.
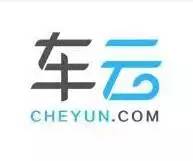
2. Software and Hardware Requirements for Visual ADAS
Visual ADAS products consist of software and hardware, mainly including camera modules, core algorithm chips, and software algorithms. In terms of hardware, driving environments (vibration, temperature variations, etc.) must be considered, with the fundamental requirement being compliance with automotive-grade standards.
(1) Automotive ADAS Camera Modules
Automotive ADAS camera modules need to be customized for development. To meet the needs of vehicles operating in all weather conditions, they generally must perform well in situations with high contrast (entering and exiting tunnels), balancing overly bright or dark areas in images (wide dynamic range); they should be sensitive to light (high sensitivity) while avoiding excessive pressure on the chip (not merely pursuing high pixels).
The camera module is fundamental. Just like a good base photo allows for beautification, ensuring that the captured image is sufficient is essential for the algorithm to perform effectively.
Moreover, in terms of parameters, the requirements for ADAS cameras differ from those for dash cams. Cameras used for dash cams need to capture as much environmental information around the front of the vehicle as possible (the position of the rearview mirror looking at the two front wheels, with a horizontal field of view of about 110 degrees). ADAS cameras emphasize reserving more judgment time while driving, needing to see further. Similar to camera lenses, wide-angle and telephoto cannot be achieved simultaneously; thus, a balance must be struck in hardware selection for ADAS.
(2) Core Algorithm Chips
Image-related algorithms have high requirements for computing resources, so chip performance is crucial. If deep learning is layered onto the algorithms to help improve recognition rates, the hardware performance requirements only increase, with key performance indicators being computing speed, power consumption, and cost.
Currently, most chips used in ADAS cameras are monopolized by foreign companies, with major suppliers including Renesas Electronics, STMicroelectronics, Freescale, ADI, Texas Instruments, NXP, Fujitsu, Xilinx, and NVIDIA, providing chip solutions including ARM, DSP, ASIC, MCU, SOC, FPGA, and GPU.
ARM, DSP, ASIC, MCU, and SOC are embedded solutions for software programming, while FPGA offers faster processing speeds due to direct hardware programming compared to embedded solutions.
GPU and FPGA have strong parallel processing capabilities. For image-related texts, especially when using deep learning algorithms that require simultaneous calculations of multiple pixel points, FPGA and GPU hold advantages. The design philosophy of these two types of chips is similar, aimed at processing a large number of simple, repetitive calculations. GPUs have stronger performance but also higher energy consumption, while FPGAs, with programming and optimization done directly at the hardware level, consume much less power.
Thus, when balancing algorithms and processing speed, especially for pre-installed systems with stable algorithms, FPGAs are seen as a hot solution. FPGAs are a good choice, but they also require high technical expertise. This is due to the fact that computer vision algorithms are written in C language, while FPGA hardware language is Verilog; the two languages differ, and those who migrate algorithms to FPGAs must have both software and hardware backgrounds. In today’s market, where talent is expensive, this presents a significant cost.
At present, there are various options for automotive-grade chips suitable for traditional computer vision algorithms, but low-power, high-performance chips that are applicable for traditional algorithms layered with deep learning algorithms have yet to emerge.
(3) Algorithms
The source of ADAS visual algorithms is computer vision.
Traditional computer vision object recognition can be roughly divided into several steps: image input, preprocessing, feature extraction, feature classification, matching, and recognition completion.
Two areas particularly rely on professional experience: the first is feature extraction. There are many features that can be used when recognizing obstacles, making feature design particularly critical. To determine whether an obstacle ahead is a car, reference features might include the car’s taillights or the shadow cast by the vehicle’s chassis on the ground. The second area is preprocessing and post-processing; preprocessing includes smoothing noise in the input image, enhancing contrast, and edge detection. Post-processing refers to reprocessing the candidate results of classification recognition.
Algorithms developed in research may not perform well in real-world environments. This is because research-derived algorithms may introduce conditions such as weather and complex road situations, and in the real world, besides focusing on the performance of algorithms in complex environments, the robustness of algorithms under various conditions (stability) must also be considered.
A significant change in algorithms is the penetration of deep learning.
Deep learning allows computers to simulate human thought processes through neural networks, enabling self-learning and judgment. By directly inputting calibrated raw data into the computer, such as selecting a series of images of irregular vehicles, the computer can learn what constitutes a car. This simplifies the perception process to two steps: inputting images and outputting results, eliminating the need for feature extraction and preprocessing.
There is a consensus in the industry that deep learning will surpass traditional visual algorithms in perception. Currently, deep learning algorithm models are open-sourced, and since there are not many types of algorithms, there is potential for excellent results to emerge as the threshold for entry is lowered. However, due to the lack of suitable vehicle-side platforms, there is still some distance to go before commercialization.
Industry views on the application of deep learning in ADAS are generally objective and calm. Many believe that deep learning algorithms are akin to black box algorithms, similar to the process of human intuitive decision-making, which can quickly output results but are difficult to trace back to the reasons after an accident. Therefore, when using deep learning, rational decision-making should be incorporated, and designs should be partitioned.
Others believe that traditional computer vision algorithms are more