This article is transcribed and summarized by ReadLecture (readlecture.cn). ReadLecture focuses on audio, video, and text transcription, summarization, and translation. For video PPT and speech extraction, lecture learning, course review, foreign language course translation, meeting summarization, podcast summarization, personal knowledge base construction, and text material organization, use ReadLecture!
For more lecture and interview insights, please follow the WeChat public account “ReadLecture Content Selection” to get more!
Video Source
bilibili: https://www.bilibili.com/video/BV1QxB9YuERU?p=1
Outline
The mind map is summarized by ReadLecture (readlecture.cn)
Summary
One-Sentence Summary
This article introduces the latest research progress of the Vision-Language-Action model (VLA) in robotic manipulation tasks, exploring its applications, challenges, and future development directions.
Conclusion
-
Robotic manipulation tasks are very common in daily life, but currently, robots have not yet reached human-level performance in these tasks. -
The Vision-Language-Action model (VLA) aims to address issues such as data scarcity and the sim-to-real gap in robotic manipulation tasks by integrating visual, linguistic, and action information. -
Through pre-training and fine-tuning on large-scale datasets, VLA can generalize across various tasks and embodied forms. -
Current research shows that VLA performs well in handling complex and long-range tasks, but still faces challenges in generalization ability and data diversity. -
Future research directions include improving data collection methods, exploring new pre-training strategies, and integrating reinforcement learning techniques.
Deep Q&A
-
What are the main challenges of robotic manipulation tasks?
-
Main challenges include data scarcity, the sim-to-real gap, diversity of embodied forms, and action multi-modality.
How does the Vision-Language-Action model (VLA) address data scarcity?
-
VLA reduces reliance on specific task data by generalizing across multiple tasks through pre-training on large-scale datasets.
How does VLA handle the diversity of embodied forms?
-
VLA designs a universal output space that can be trained across data from various embodied forms, absorbing skills from different forms.
How does the current VLA model perform in long-range tasks?
-
The current VLA model performs excellently in long-range tasks but still requires further improvements to tackle more complex tasks.
What are the key future research directions for VLA?
-
Future research focuses on improving data collection methods, exploring new pre-training strategies, integrating reinforcement learning, and constructing larger-scale datasets.
Keyword Tags
-
Robotic Manipulation -
Vision-Language-Action Model -
Pre-training -
Embodied Forms -
Generalization Ability
Target Audience
-
Robotics Researchers
-
Researchers interested in robotic manipulation tasks and vision-language action models.
-
AI practitioners who want to learn how to apply vision-language models to robotic manipulation tasks.
-
Engineering technicians interested in the integration of robotic hardware and software.
Terminology Explanation
-
Vision-Language-Action Model (VLA): A model that integrates visual, linguistic, and action information for robotic manipulation tasks. -
Embodiment: Refers to the physical form of robots, such as robotic arms, end-effectors, etc. -
Sim-to-Real Gap: The difference between simulated environments and real environments, affecting the model’s performance in real-world scenarios. -
Action Multi-modality: The ability to complete the same task through various action sequences. -
Pre-training: Training on large-scale datasets to learn general skills, facilitating subsequent fine-tuning.
Content Review
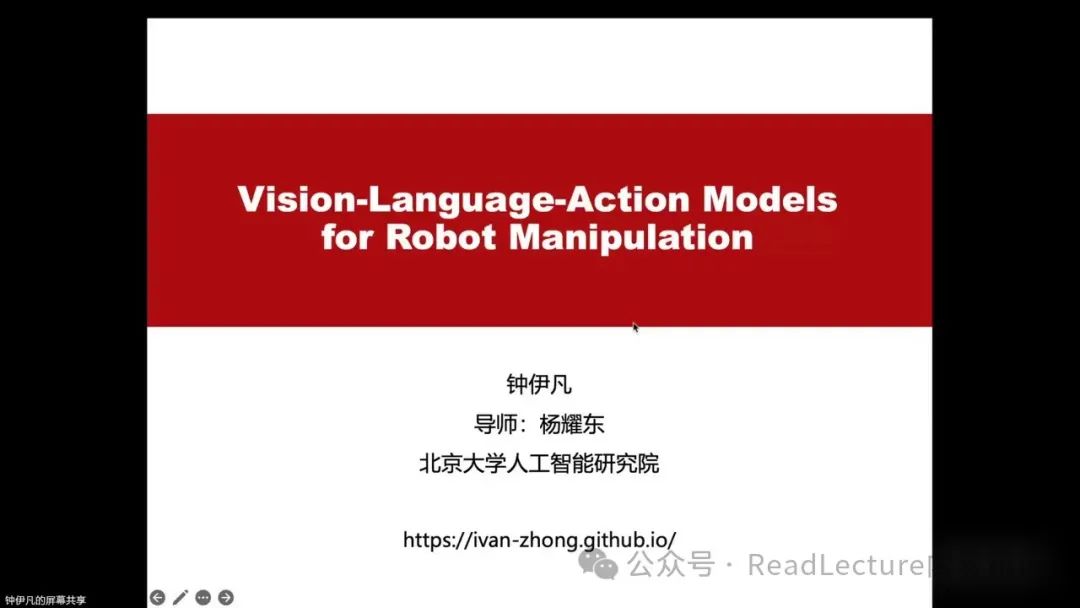
Hello everyone, I am Zhong Yifan, currently a second-year PhD student at the Institute of Artificial Intelligence, Peking University. My supervisor is Professor Yang Yaodong. Today, I will give a report mainly sharing the latest research progress of Vision-Language-Action Models in robotic manipulation tasks, summarizing the insights these studies bring to us, their current limitations, and future research directions.
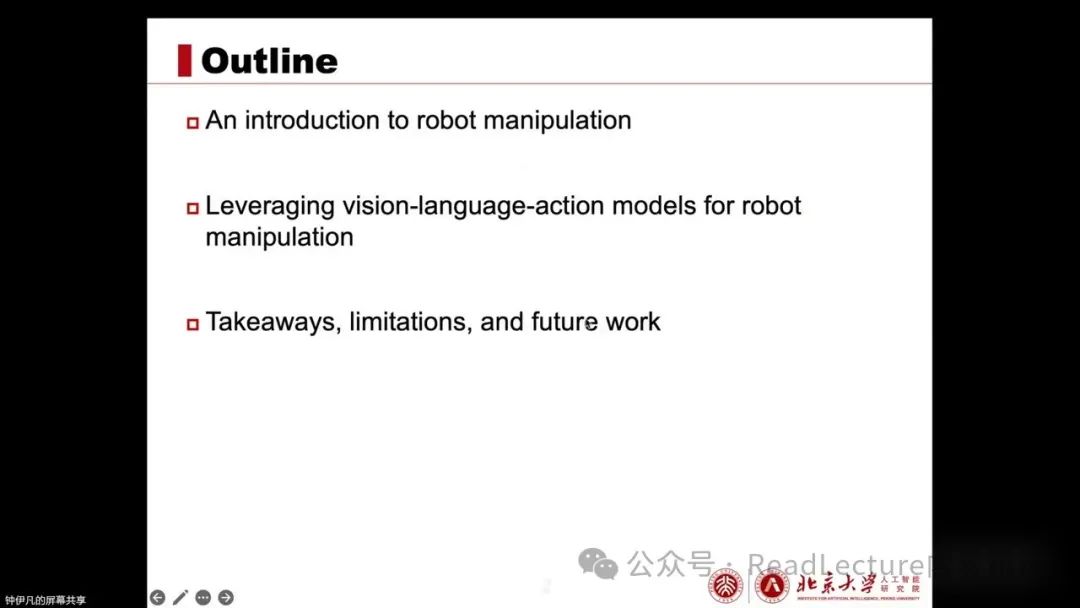
Therefore, my report is divided into three parts. The first part introduces robotic manipulation tasks and the challenges they face. The second part discusses how to use vision-language action models to design these models and the entire training process to solve robotic manipulation tasks. The third part summarizes the insights, limitations, and future work directions brought by these models. The first part will introduce robotic manipulation tasks.
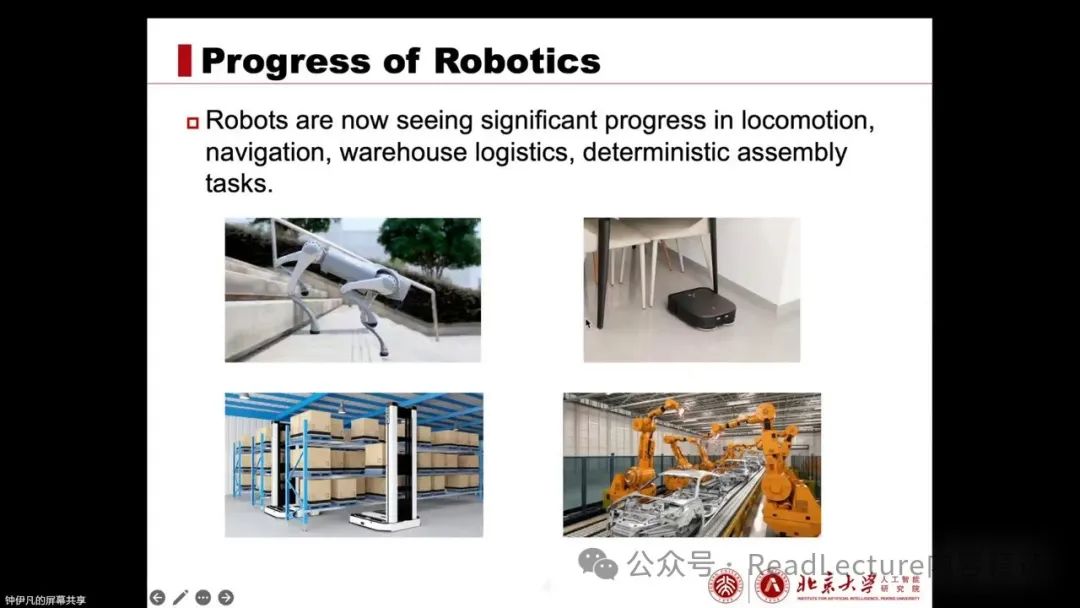
Let us first review the development of robotics. So far, significant progress has been made in walking navigation, warehousing logistics, and deterministic assembly tasks.
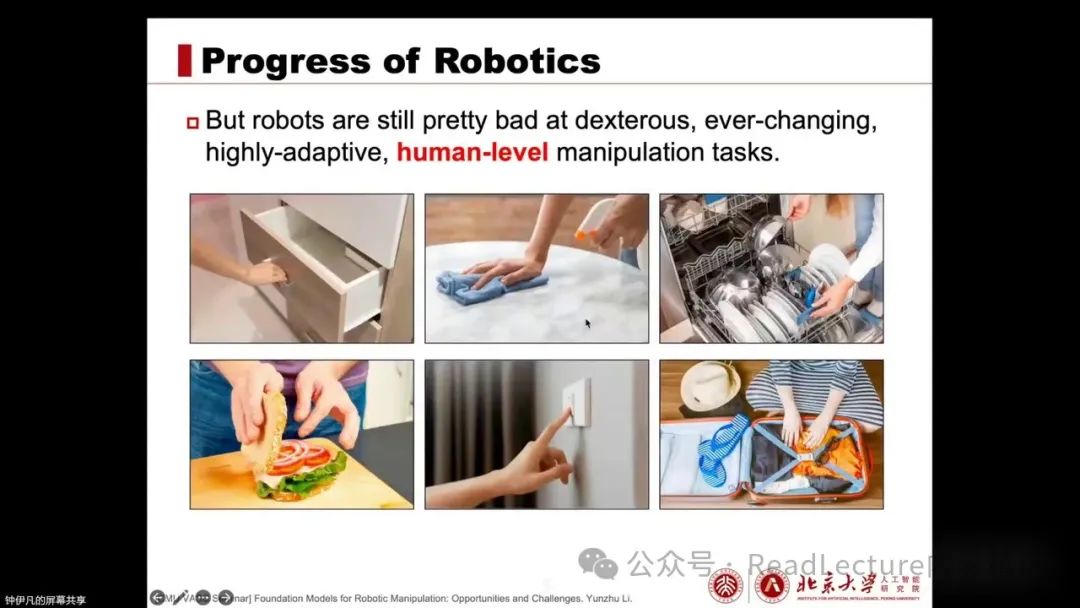
However, there are still many manipulation tasks in our daily lives that we hope robots can accomplish. For example, opening cabinets, placing items, wiping tables, washing dishes, cooking, organizing clothes, etc. These tasks are very common in life, dexterous and variable, with strong adaptability and potentially random.
However, currently, robots do not perform well in these manipulation tasks and have not yet reached human-level operational performance. Therefore, how to enable robots to accomplish these manipulation tasks is an urgent problem to solve.
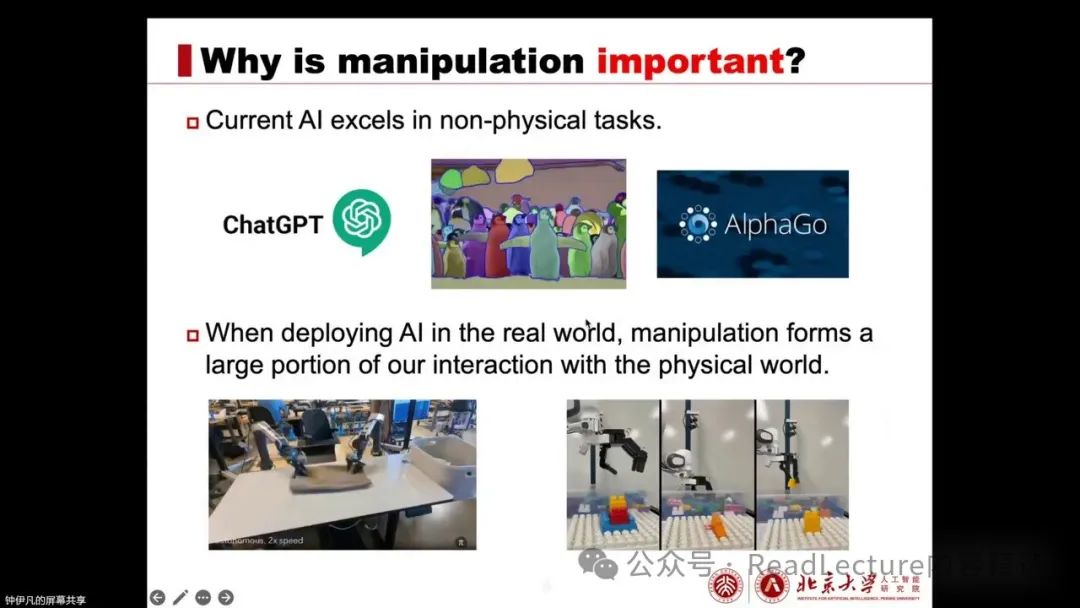
We are currently discussing robotic manipulation tasks. Why is this important? Because current artificial intelligence has made significant progress in non-physical world tasks. For example, ChatGPT excels in dialogue tasks, Sam performs well in segmentation tasks, and AlphaGo has surpassed humans in the specific task of Go.
However, we ultimately hope robots can enter our lives and help us complete various tasks in the real world. If robots are deployed in the real world, manipulation becomes an important medium for us to interact with the physical world. Therefore, we hope robots can master this ability as well.
For example, the left image shows the recently released RT-2 model, which is folding clothes; the right image shows a robotic hand performing an assembly task.
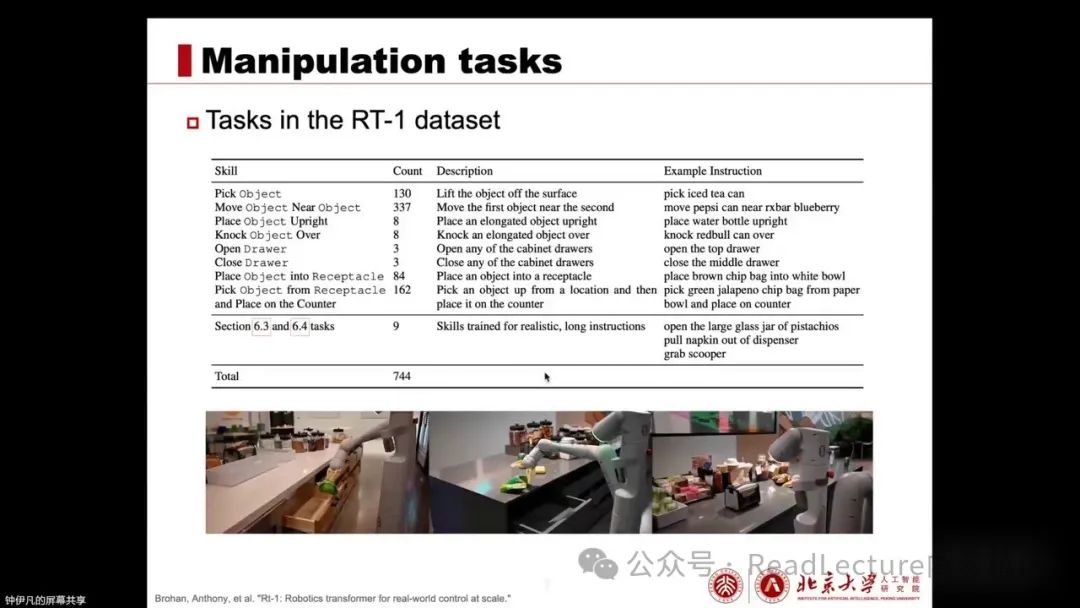
We all hope robots can do well. Now, let’s briefly introduce what robotic manipulation tasks (Robot Manipulation) may include.
In the RT-1 paper, robotic manipulation tasks may include the following:
-
Picking up an object -
Placing an object next to another object -
Opening or closing a drawer
Below are some images showing the process of Google robots completing these tasks.
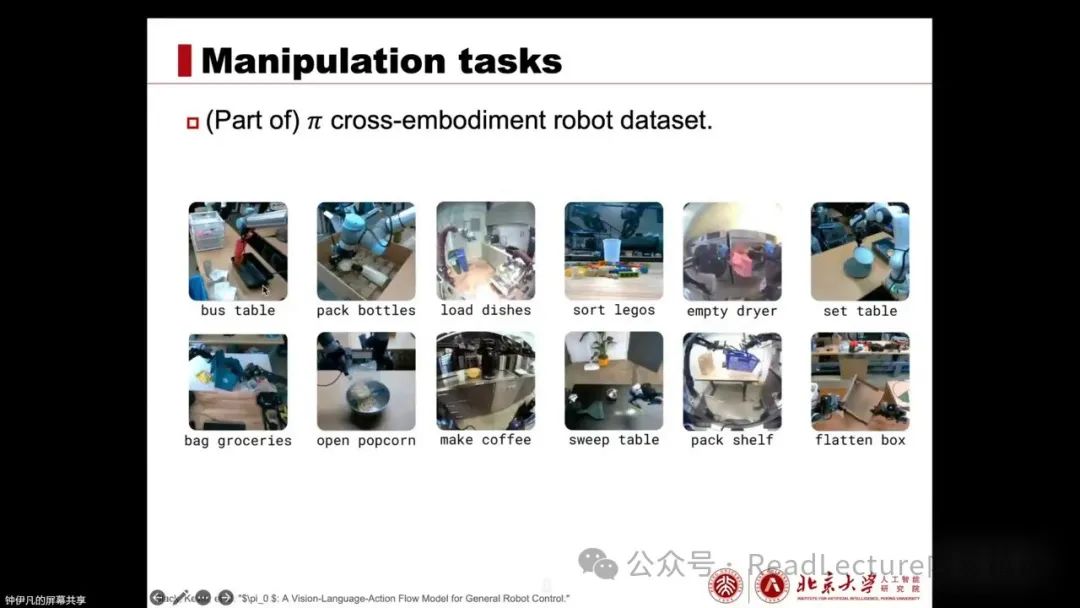
There are also some tasks demonstrated in the Payling paper. It collected some robotic datasets that include various robotic manipulation tasks. For example, there might be sorting LEGO blocks or taking clothes out of a dryer.
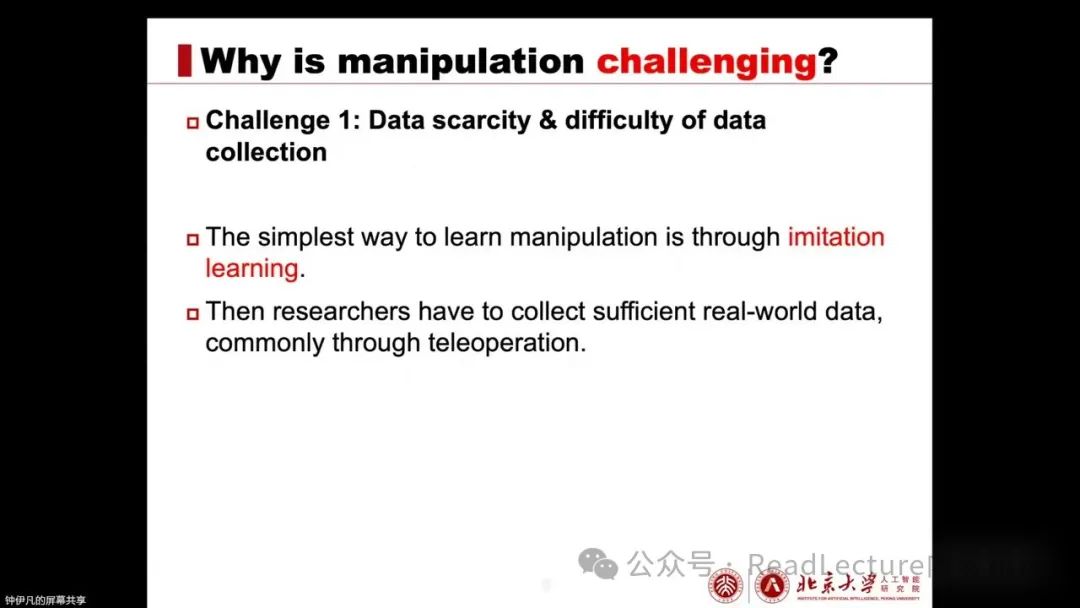
These tasks are relatively more complex, more general, and more common. So why did we just mention that manipulation is important? Next, let’s discuss why manipulation tasks are challenging and why we cannot do them directly. Why is there a need for extensive research, and why do we have a long way to go?
First, the first issue is data scarcity and the difficulty of data collection. Because, similar to what we did in natural language processing (NLP) or computer vision (CV), one of the simplest ways to learn manipulation is through imitation learning. However, imitation learning requires researchers to collect a large amount of real-world data to learn the task very well.
When collecting this data, teleoperation is typically used, which is a method of remote operation. However, collecting data through teleoperating robots requires a lot of labor and time, and may also involve safety issues. Moreover, for each target task, we may need to collect a large amount of data. Therefore, the workload of data collection is actually unbearable.
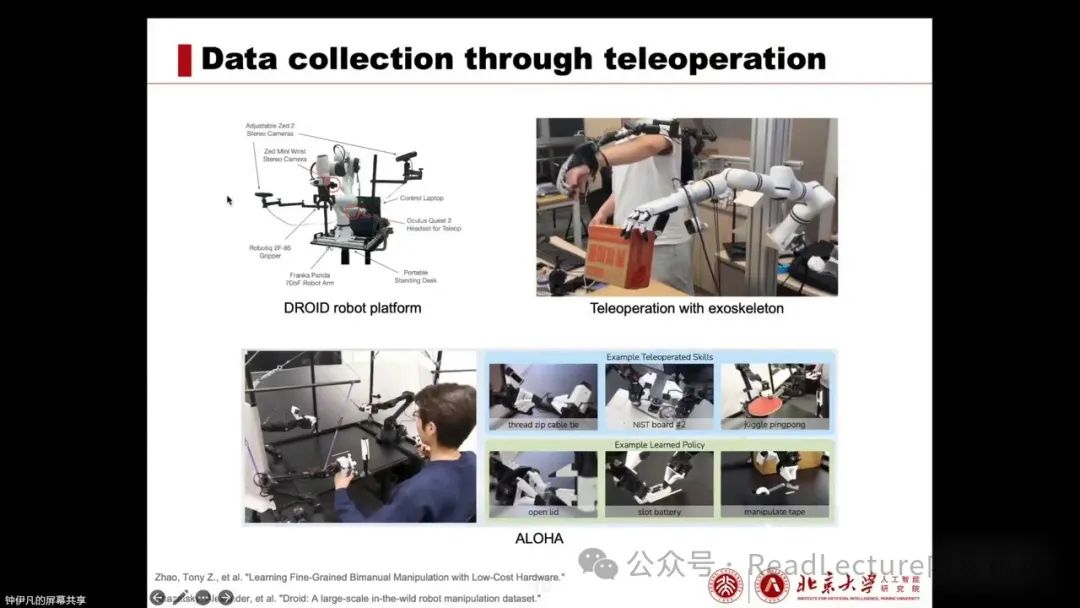
For example, here are some data collection platforms. The top left is the Droid data collection platform. The top right is a diagram showing remote operation through an exoskeleton, using a robotic arm to collect data that can be used for subsequent robotic arm training. The bottom is a robotic platform proposed in the Aloha paper, which completes tasks through remote operation and collects data.
The first challenge mentioned is the challenge of data collection. The second challenge is the Think2Real gap.
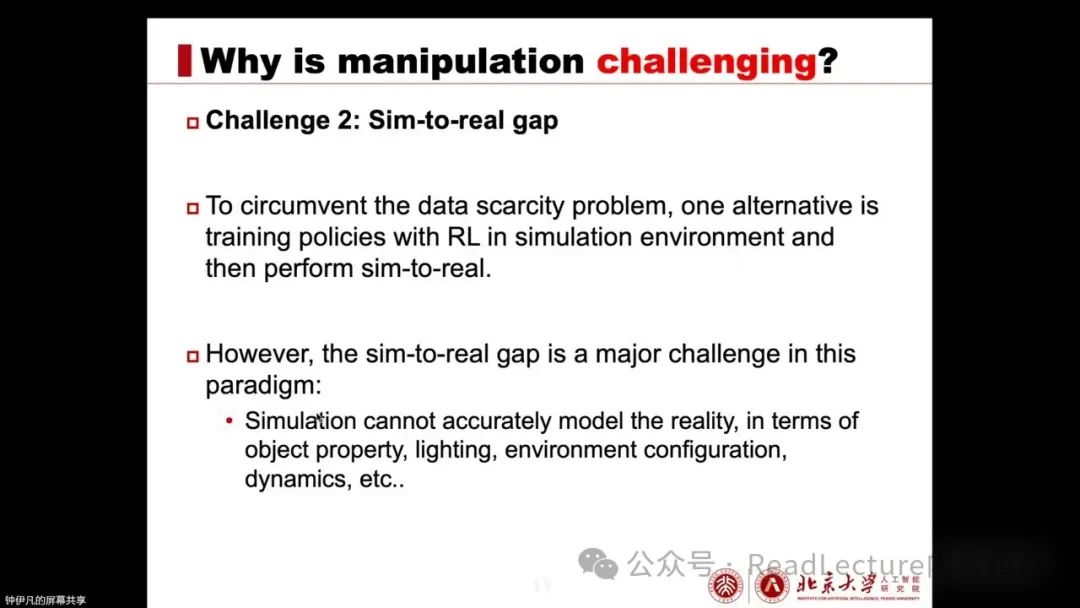
Let me explain what this means. As mentioned earlier, collecting data in the real world faces many difficulties and challenges, making it hard to gather a large amount of data. Therefore, another possible solution is to use simulated data. Simulated data can be used to train strategies through reinforcement learning methods, as simulated data can be collected infinitely, and multiple environments can be collected in parallel, with relatively low workload and cost. Therefore, we can train in simulated environments using reinforcement learning methods and then transfer the strategies trained in simulations to real environments using the Sim-to-Real method, hoping they can work directly.
This is the Sim-to-Real approach. In general, it involves training in a simulated environment and deploying in a real environment to solve the problem of difficult data collection in real environments. However, in this paradigm, the Sim-to-Real gap is the biggest challenge. The main reason is that we hope the simulated environment corresponds to the real environment, so that the strategies trained in simulations can be directly used in real environments, but in reality, this is not the case. Even the best simulation platforms currently available still have certain differences from the real environment, such as object properties, lighting, environmental settings, and dynamics. This causes strategies trained in simulations to often not work directly in real environments and may require further adjustments. Therefore, the Sim-to-Real gap makes this solution not very effective for completing tasks directly.
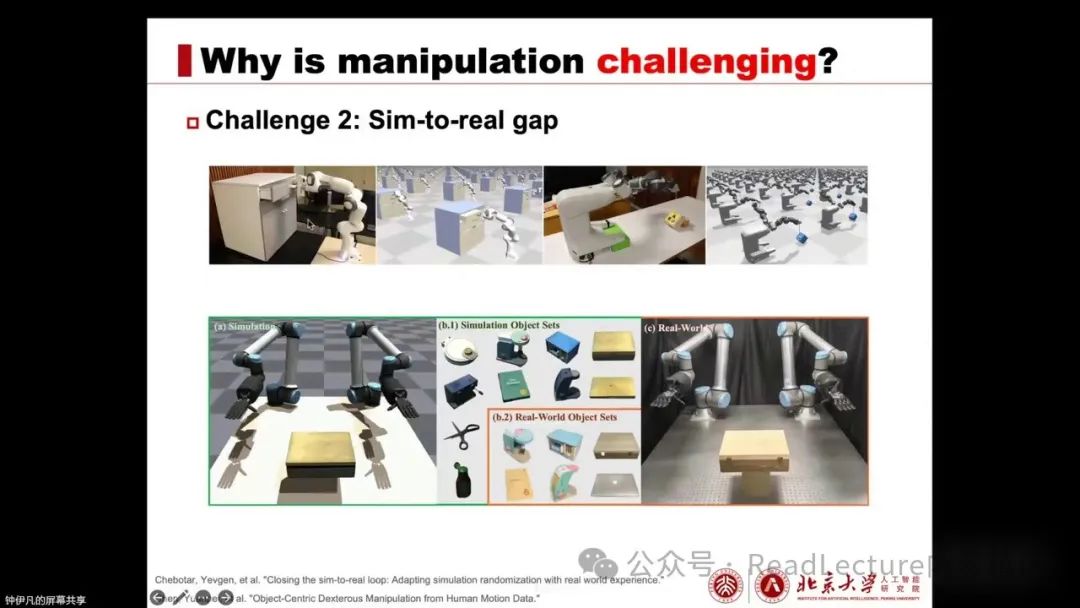
Here I give a few examples, such as the left and right images corresponding to scenarios trained in simulation and deployed in reality. You can see that we try to simulate the real environment in simulation to complete tasks and model such environments. After large-scale parallel training in simulations, we deploy on real machines.
The main challenge here is to accurately model the real environment, ensuring that all dynamic characteristics are fully consistent, which is itself difficult. Due to these difficulties,
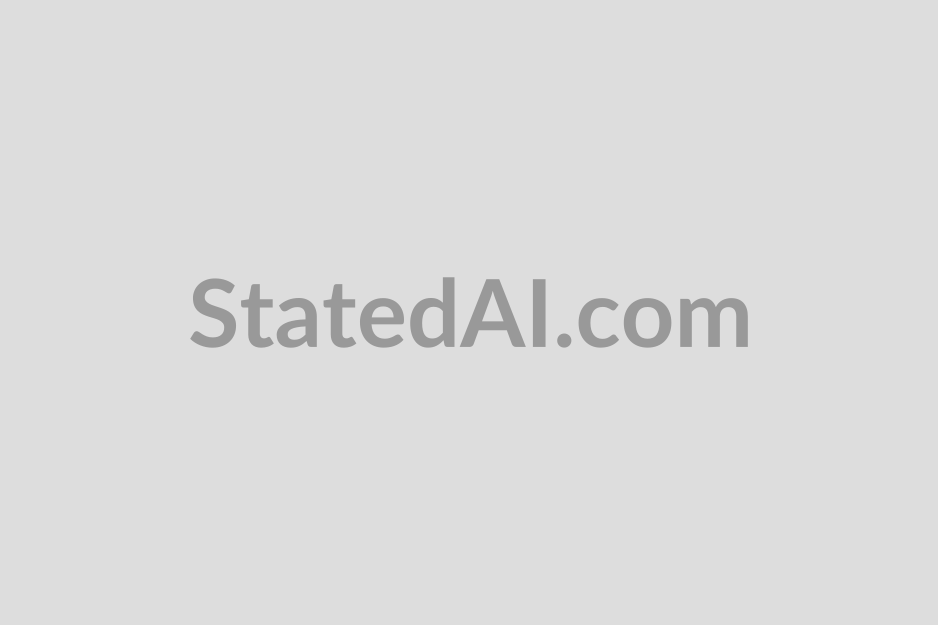
this leads to strategies trained not being directly applicable in real environments. The third challenge is that robots have different embodiment forms. For example, current robotic manipulation tasks are mostly completed by a robotic arm and an end-effector. However, there are many types of robotic arms and end-effectors. Different embodiment forms lead to strategies that cannot be directly generalized. For example, here are three different robotic arms that may have different degrees of freedom, dynamics, and kinematics, as well as different modalities. Some may have two arms, some may have one arm, and some may be equipped with multiple cameras.
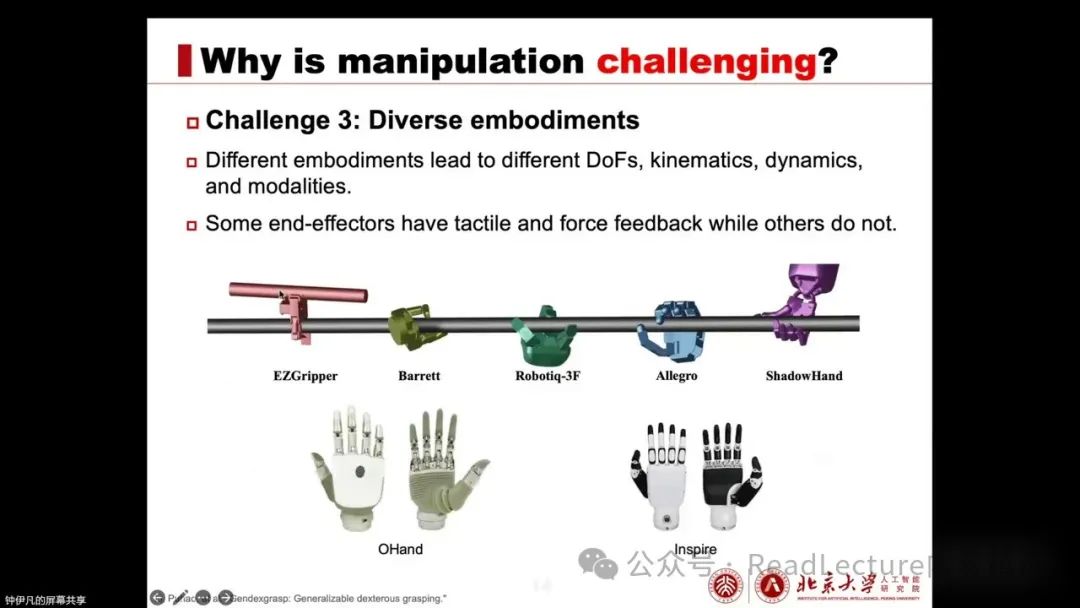
Some may only be equipped with a single camera, and the same goes for end-effectors. Some use dexterous hands with five fingers, while others may use two-fingered grippers, which are all different. These differences cause the training strategies to have to consider these situations. Moreover, some end-effectors may have tactile sensory feedback, while others may have force tactile feedback.
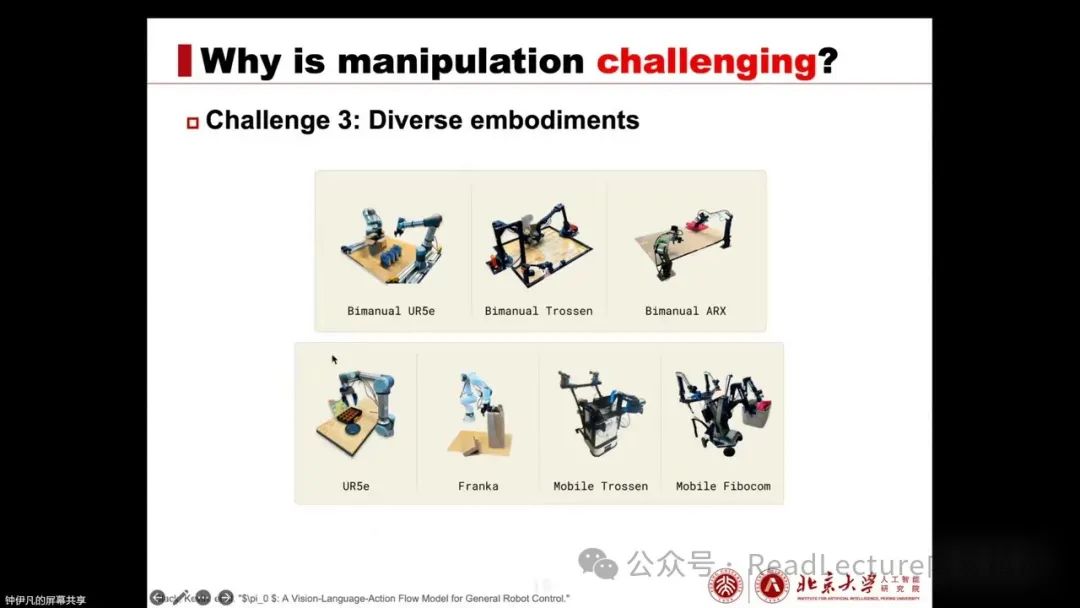
However, some may not have any. For example, here are seven robots used in the Payling paper. Such a diverse range of embodiment forms makes it difficult to share data and training between different embodiments. The same algorithm or design may work effectively on one embodiment but may not necessarily perform well on another. This is because it relates to the form, operation, and control methods. Therefore, multiple embodiment forms bring additional challenges to training and tasks.
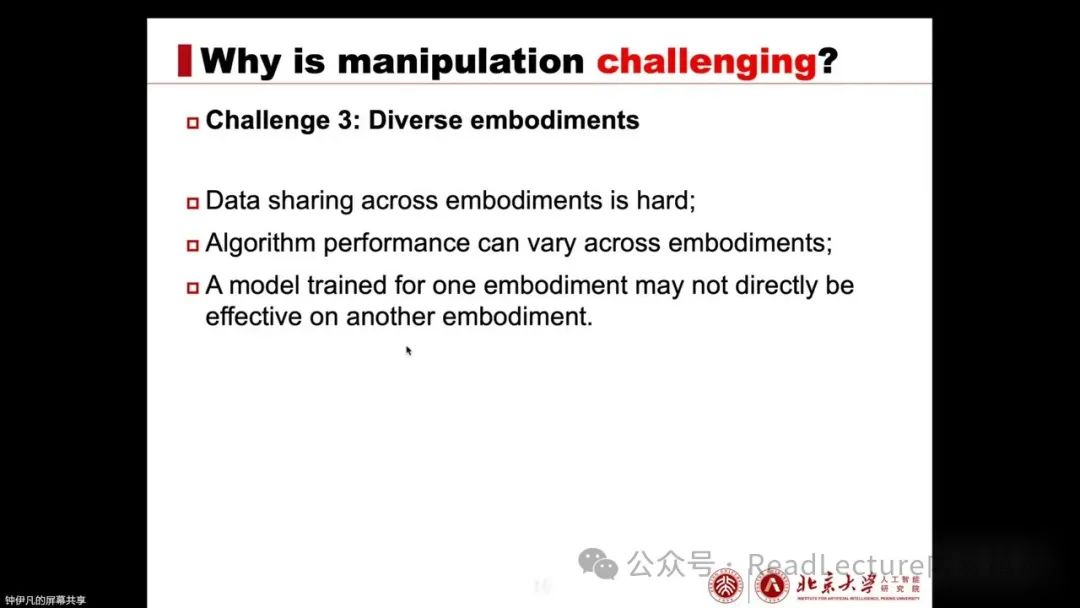
This issue also has a challenge, which is the problem of action multi-modality. Action multi-modality means that for the same task, there may be many different ways to solve it, i.e., many different actions can be used to complete it. For example, we see the pushT task on the right, where the task is to push the gray T to the position of the green T and ensure it fully covers the green T. Starting from the current blue point, it can either go around from the top or from the bottom to push.
Therefore, even if we have collected a large amount of data, this data may contain various different solutions. How to learn a strategy from this data that can model various different solutions is also a challenge. If we directly use regression methods for supervised learning on these trajectories, the final learned strategy may not be able to complete the task well.
Thus, our final strategy needs to be able to model this diverse action distribution. This is also a challenge of the task.
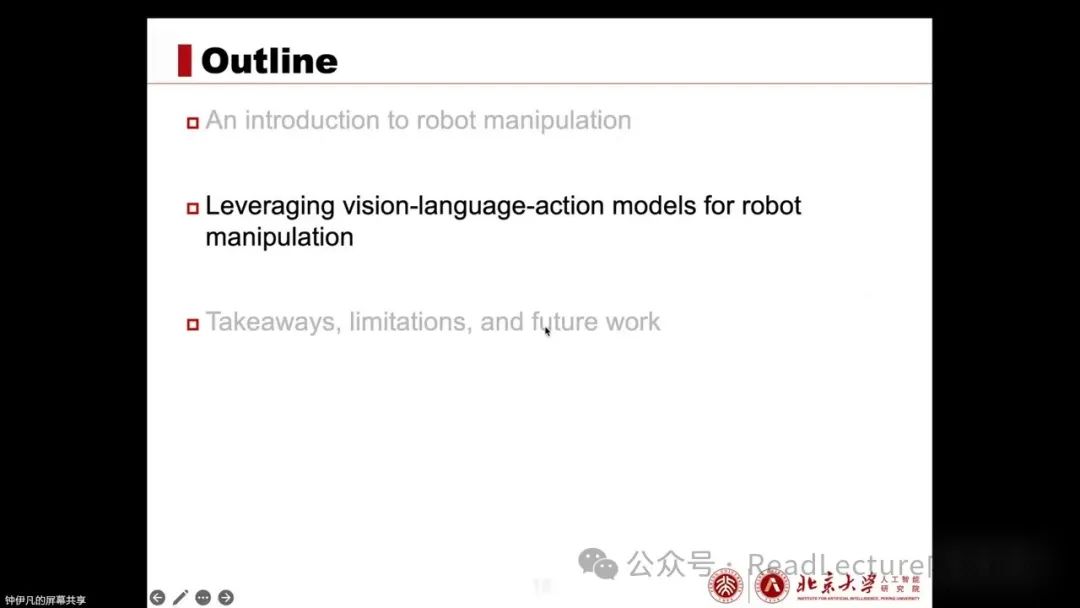
We see that robotic manipulation tasks are actually a widespread, very important, and challenging problem. Today we will discuss some research work using vision-language action models to solve robotic manipulation problems.
First, let’s introduce what the Vision-Language-Action model is.
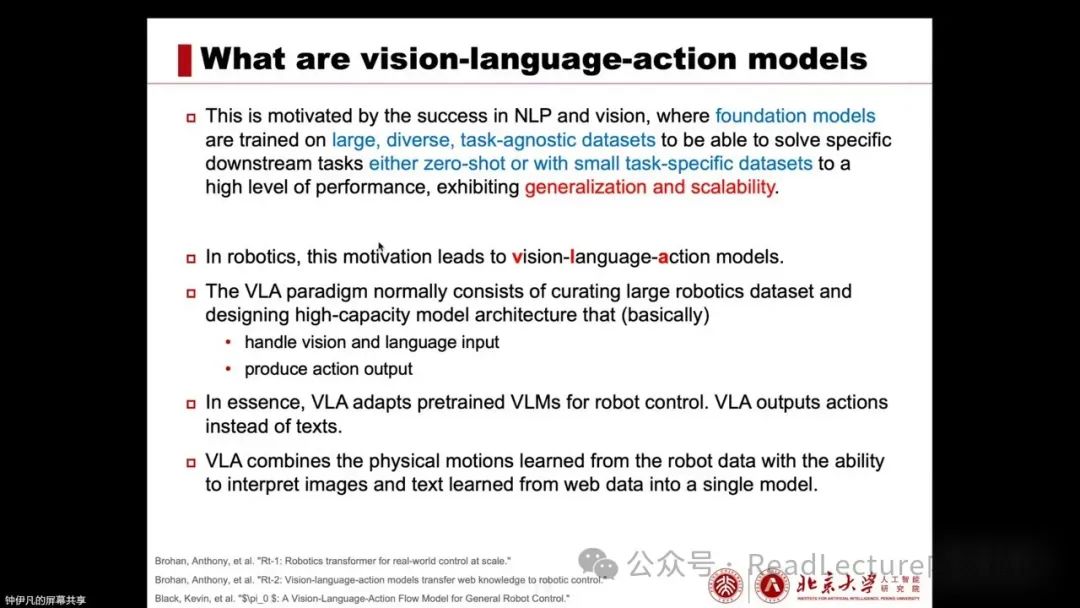
Abbreviated as VLA, it is mainly inspired by the successes in NLP and computer vision. If we can train foundational models on a large, diverse, and task-agnostic dataset, then these foundational models can achieve zero-shot capabilities on downstream tasks. Additionally, if there is a dataset for fine-tuning on the target task, it can quickly achieve high performance and demonstrate strong generalization and scalability as the amount of data and model parameters increase.
Based on these inspirations, we hope to develop a similar foundation model in the robotics field, namely the Vision-Language-Action models (VLA). This name explains its input and output: in robotic manipulation tasks, there may be language instructions provided by humans, as well as visual information from cameras in the scene, with the final output being the robot’s actions. Therefore, it is called Vision-Language-Action models.
The paradigm typically involves collecting a large number of robotic datasets for pre-training while also collecting high-quality datasets for subsequent fine-tuning, and designing high-capacity model architectures to effectively handle these inputs and outputs. At its core, VLA directly uses or fine-tunes existing VLMs (pre-trained vision-language models) for robotic control tasks, outputting actions instead of text. Thus, VLA aims to combine the actions learned on robotic data with the visual and linguistic information learned during the VLM PreTrain or VLA phase in one model, demonstrating powerful robotic control capabilities.
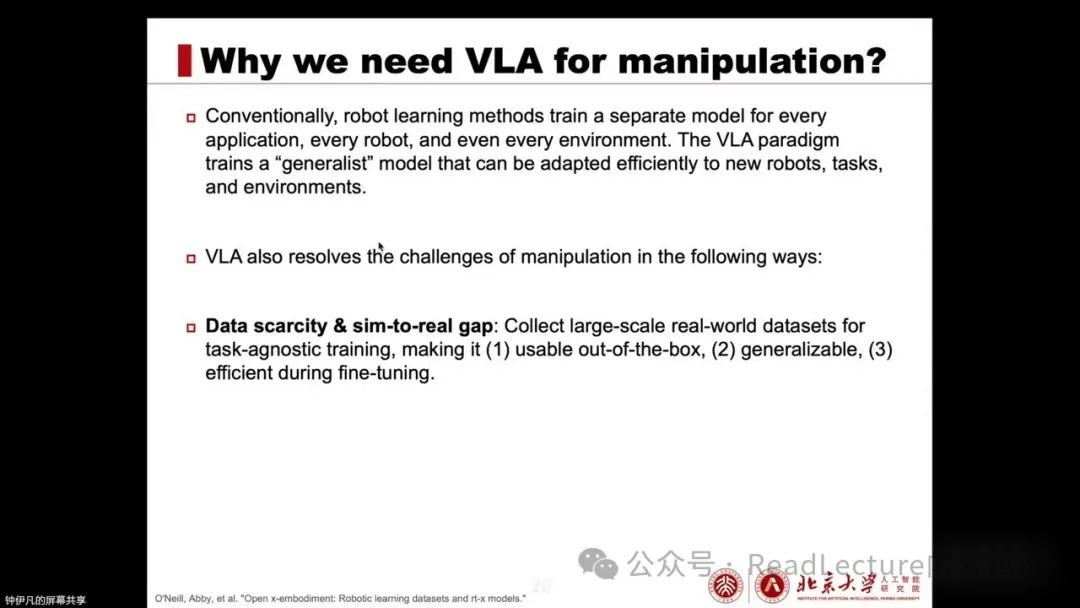
Next, we will discuss why we might need Vision-Language-Action models (VLA) to handle robotic manipulation tasks. If there is no VLA, traditional methods involve training a strategy for each target task, each robot, and even each environment. However, VLA adopts the foundational model approach, aiming to train a universal strategy that can generalize across various scenarios, robots, and tasks. At the same time, if it encounters new robots, tasks, or environments, it can quickly adapt through fine-tuning and other methods.
VLA also addresses the operational challenges we mentioned earlier from several aspects. For example, for the issues of data scarcity and the sim-to-real gap, VLA’s training collects a large number of real-world datasets for task-agnostic training. Once training is complete, it has a rich knowledge base that can be used directly on many tasks with a degree of freedom. Meanwhile, due to its backbone from visual-language models, it possesses strong generalization capabilities. Even if it performs poorly on some target tasks, it can quickly improve through efficient fine-tuning due to the influence of pre-training and visual-language models.
In addition, regarding the issue of diverse embodied forms, VLA designs a universal output space that encompasses multiple embodied forms. This way, it can train across cross-modal and cross-embodiment data, absorbing skills from various forms, ultimately being able to work on each form. For the action multi-modality issue, VLA can adopt generative modeling methods to model multi-modal distributions, thus effectively addressing the aforementioned problems.
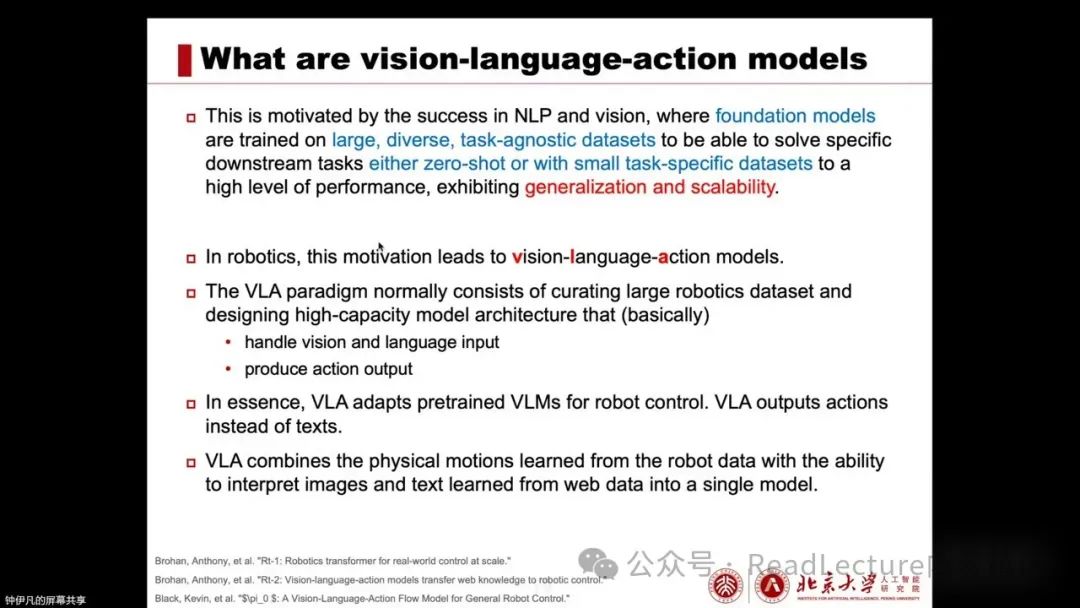
Next, we will introduce current work. First, as we just mentioned, VLA mainly requires us to collect a large number of high-quality datasets and design a high-capacity model architecture. So, let’s first take a look at the current data collection efforts.
The first well-known work is RT-1. In its paper, they collected a large-scale dataset covering approximately 130,000 episodes involving over 700 tasks. The collection process used 13 robots over a period of 17 months. This dataset covers tasks such as picking up an object, opening and closing drawers, and other similar tasks.
Here, an episode refers to successfully completing a task from start to finish, while a task refers to a command the system can execute.
Below are some photos of Google robots completing tasks.
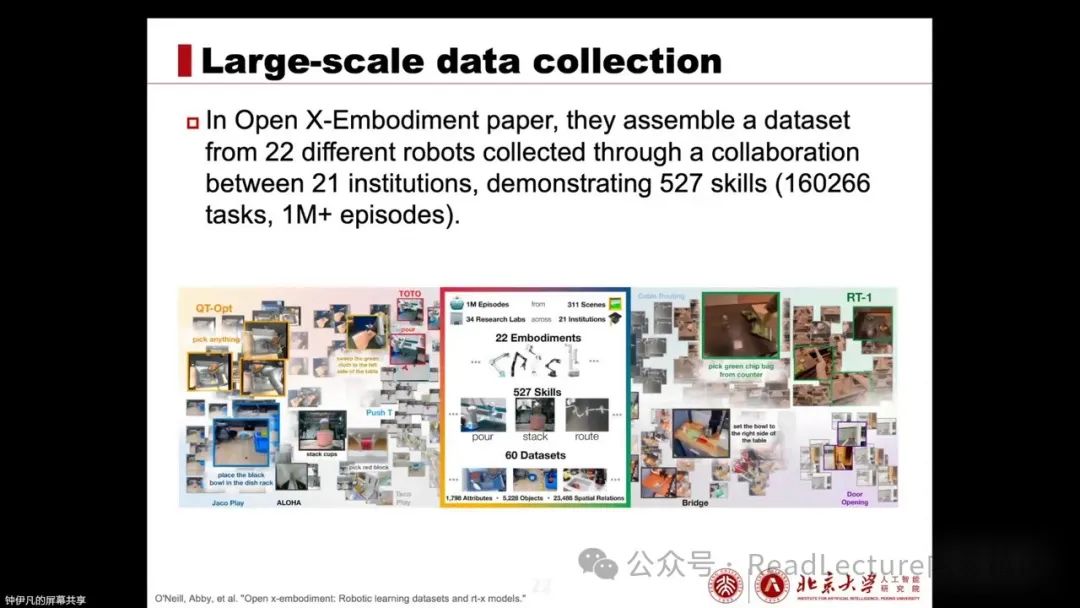
After the content we just covered, there is a more famous work called OpenX-Embodiment, which mainly integrates some of the previously available datasets, such as RT-1 being included in OpenX-Embodiment. Other well-known datasets, such as Bridge and ALOHA, are also included in this dataset.
This dataset covers a total of 22 different robots, collected by approximately 21 institutions, presenting over 1 million episodes, with data being very diverse and large-scale. In many subsequent papers, researchers will consider training their vision-language action models (VLA) on the OpenX-Embodiment dataset.
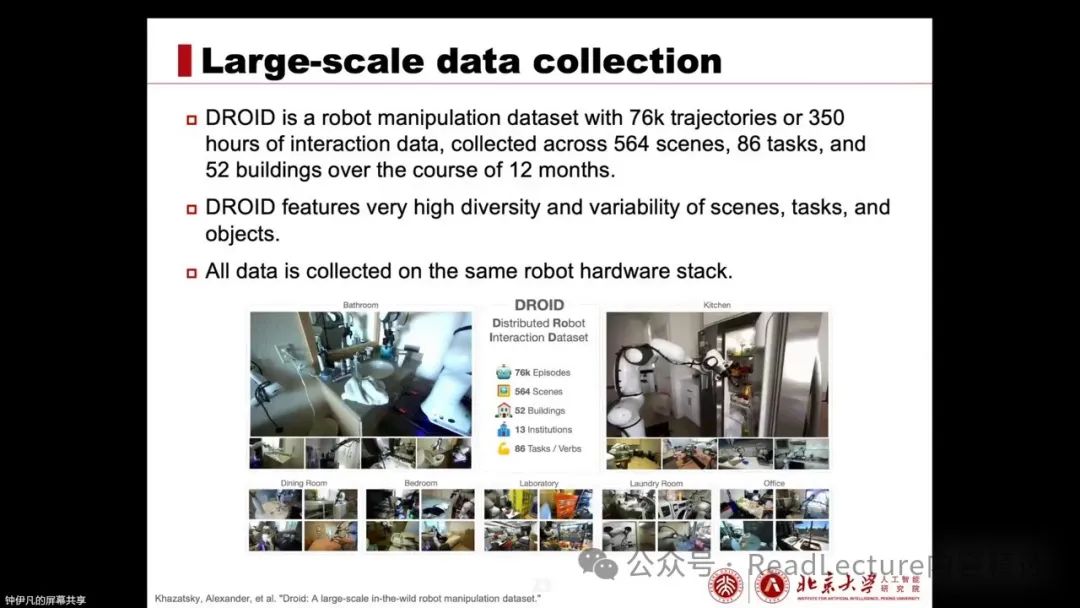
Another work is DROID, which uses robotic operation datasets. Unlike the previous OpenX Embodiment, DROID designed a unified robotic platform and collected data across various locations and institutions worldwide, integrating it into a large dataset.
This dataset contains 76K trajectories or 350 hours of interaction data, covering 564 scenes and 86 tasks, collected across 52 buildings over a duration of 12 months. The most significant feature of DROID is its extremely high data diversity, with rich variations in scenes, tasks, and objects. All data were collected on the same robotic hardware platform, unlike OXE, which aggregated data from different robots.
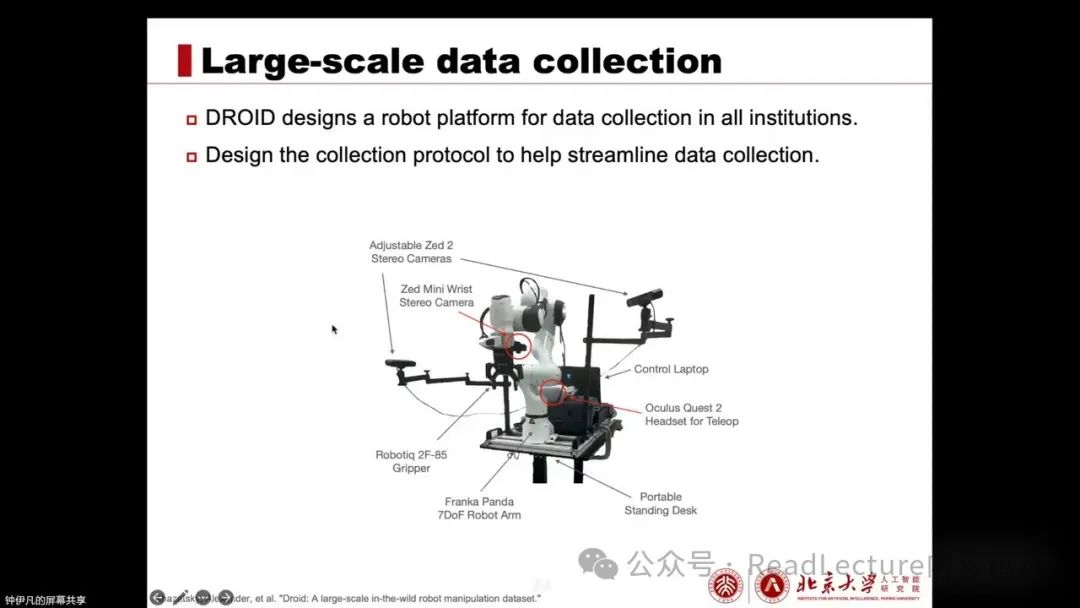
Here is the robot platform designed by DROID for data collection. After designing such a unified platform, researchers worldwide can use the same robotic platform to collect data. After data collection, everyone can share and use it, thereby avoiding the previously mentioned cross-embodiment issues. Meanwhile, they also designed an efficient data collection process to ensure high data quality.
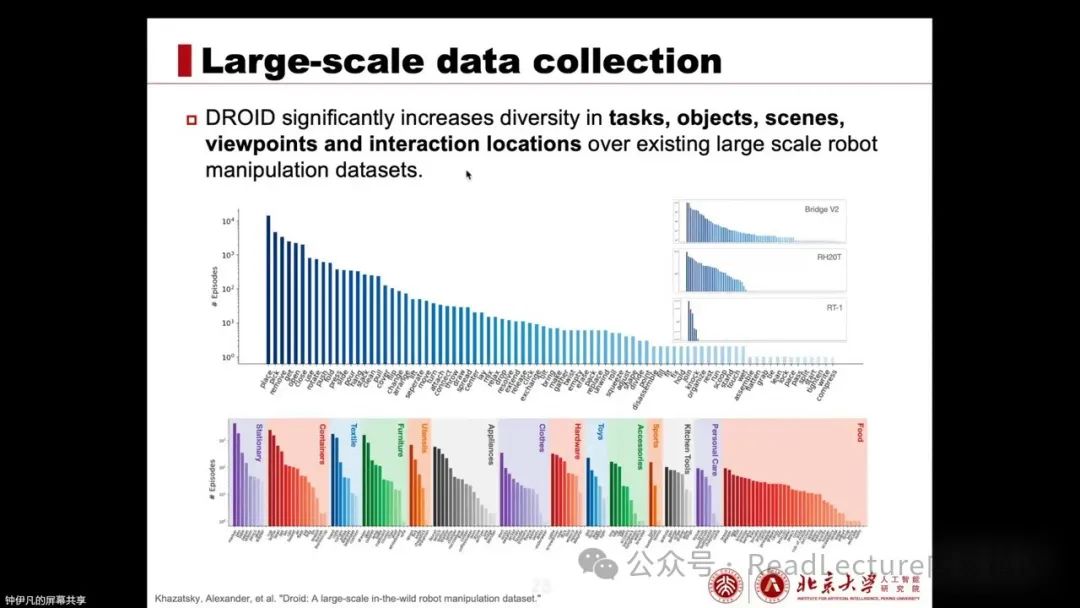
Additionally, the paper also includes some analysis of DROID data, focusing on exploring the applications of this data in tasks.
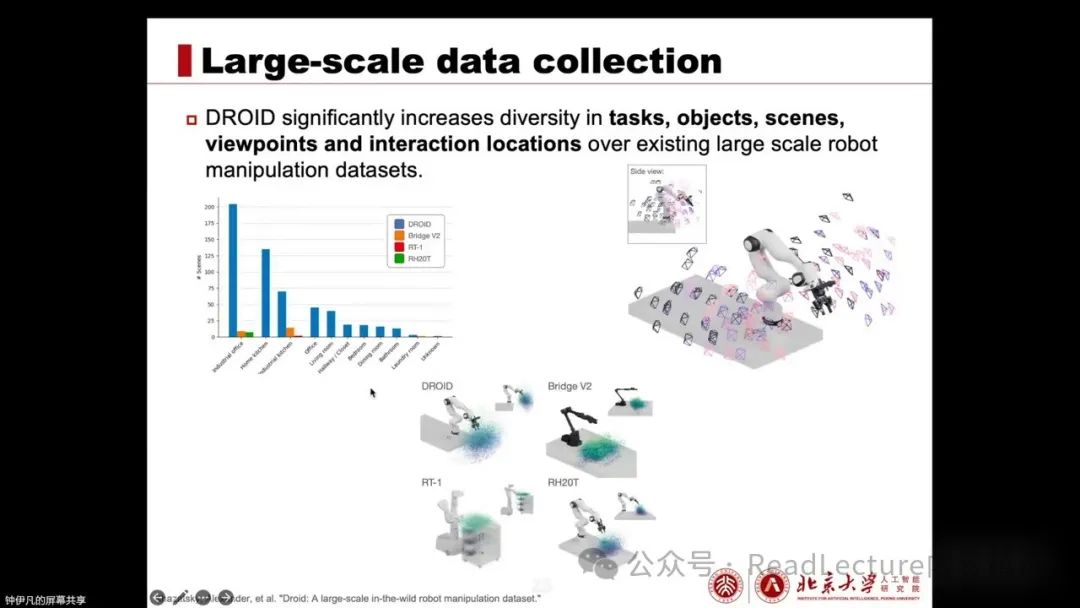
In terms of scenes, objects, camera angles, and robot interaction locations and places, there is extreme diversity, far exceeding existing large-scale robotic operation datasets. This diversity is very important, and subsequent experimental analysis also verifies the key role of diversity in robotic performance. Therefore, Joy’s main work has made significant contributions to diversity.
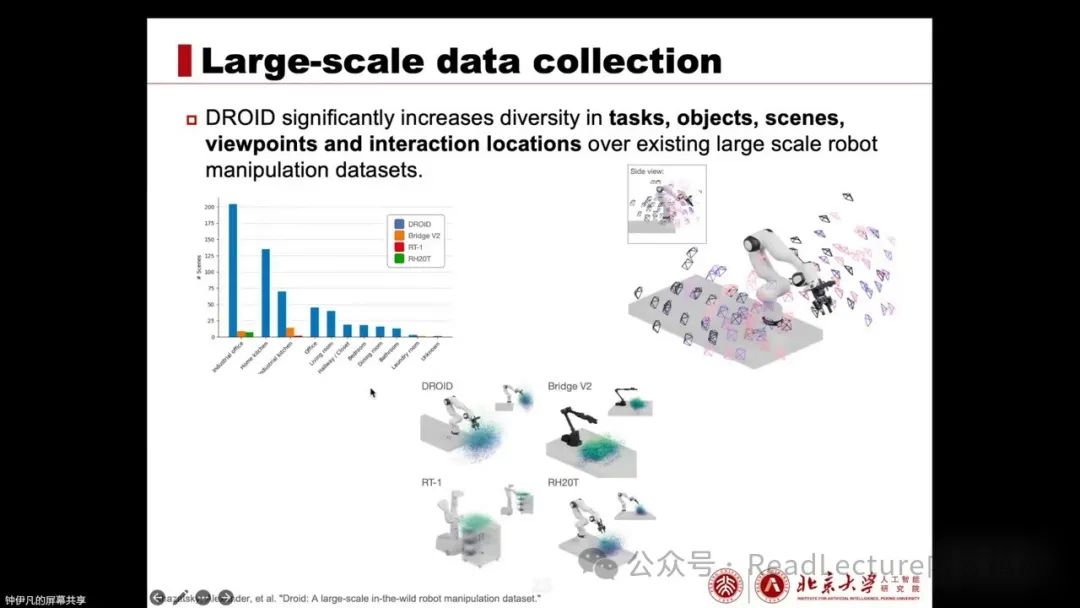
And also made a very significant contribution. After discussing these well-known large-scale data collection efforts, let’s take a look at the VLA architecture, which is aimed at training an effective VLA model. On this page, I mainly summarize and succinctly compare some typical papers, allowing for a vertical comparison to observe the evolution and progress of VLA.
RT1 is relatively an early VLA model, trained on a large dataset, namely the RT1 dataset, and performs well on tasks in in-domain data while also demonstrating some zero-shot generalization capabilities. RT2 uses pre-trained VLM, training simultaneously on vision-language tasks and robotic data, ultimately showcasing the real-world generalization brought by vision-language models and some emergent capabilities. These are benefits derived from the training data of VLM.
RTX further combines the models obtained from training RT1 and RT2 on cross-modal datasets like OpenXEmbodiment, achieving better in-domain performance and learning from different embodiment forms, demonstrating good cross-embodiment learning capabilities. RTX introduces action hierarchy on top of RT2, where an intermediate language-motion layer effectively enhances data sharing on multi-task datasets and allows humans to better incorporate error correction during robot execution, improving its generalization performance.
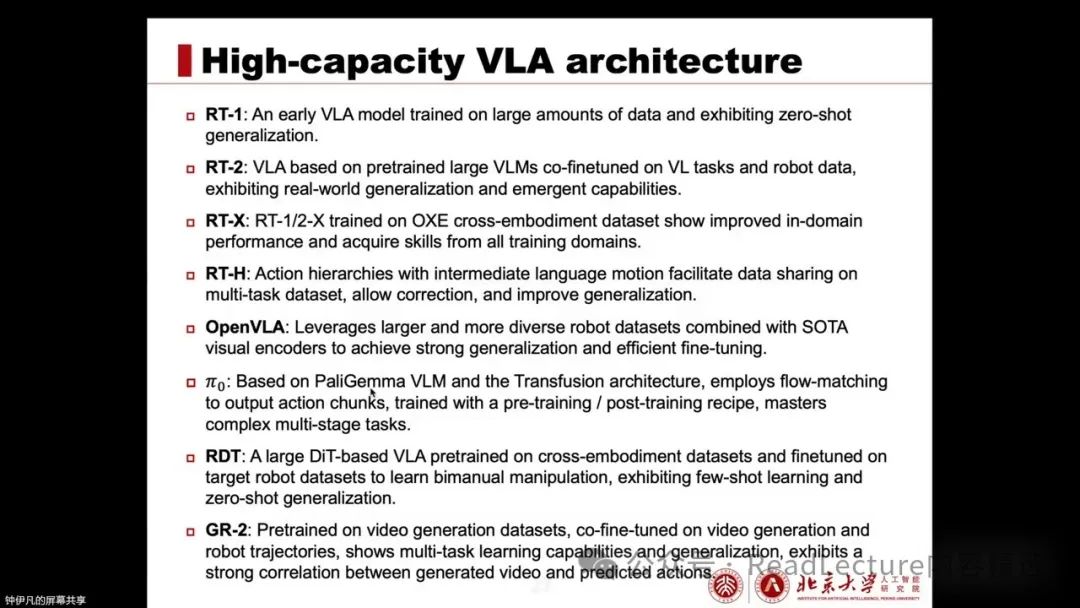
OpenVLA is a more advanced model structure, utilizing a larger and more diverse robotic dataset than ever before, thus gaining strong generalization performance and efficient functional performance. This brings it closer to the current foundation models.
Tailin is a model recently released by Physical Intelligence, based on the PaliGemma VLM and Transfusion architecture, using flow-matching to generate the final action chunks. It proposes a pre-training and post-training scheme, ultimately mastering very long and complex tasks, such as taking clothes from the washing machine and folding them, a task that can last several minutes.
RDT is mainly based on the diffusion transformer VLA, trained on cross-embodiment datasets, and fine-tuned on the target robot’s embodiment form, learning dual gripper operation capabilities and demonstrating certain few-shot learning and few-shot generalization effects.
GR2 differs from previous models in that its pre-training phase mainly uses video datasets, adopting a video generation pre-training scheme, and during fine-tuning, it jointly fine-tunes on video generation and robotic trajectories. This joint fine-tuning ultimately achieves multi-task learning and similar generalization capabilities. Because it predicts both video generation and robotic trajectories, the predicted video has a strong correlation with the executed actions, which also provides many insights for model improvement.
Next, we will introduce these works one by one.
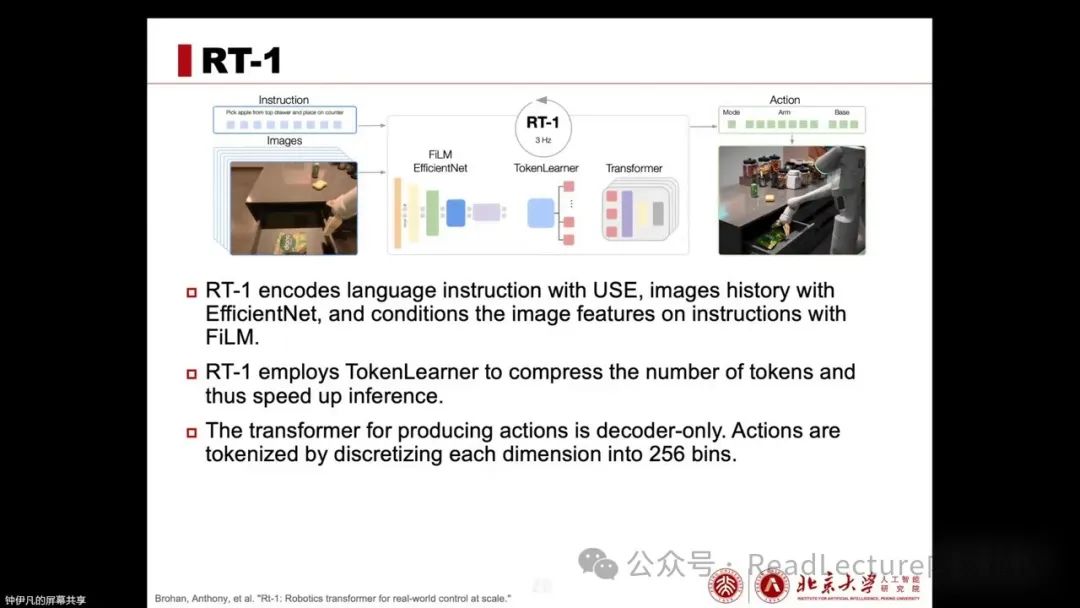
First, RT-1 is a relatively early model with a smaller scale compared to current models. Its components are also relatively early; for example, it processes conditions through language instructions and image history. Language instructions are encoded using the Universal Sentence Encoder, while images are encoded using EfficientNet, which is then processed conditionally via FiLM. The features extracted by EfficientNet consider image features after instructions. These features are then compacted into tokens using TokenLearner, making the tokens more task-adapted and fewer in number, thus reducing the model’s computational burden.
These tokens are then processed through a decoder-only transformer, directly outputting actions. Since the transformer outputs actions, the actions need to be discretized into 256 intervals. RT-1 performs imitation learning on the previously mentioned diverse RT-1 dataset, ultimately allowing for inference at a frequency of 3 Hz.
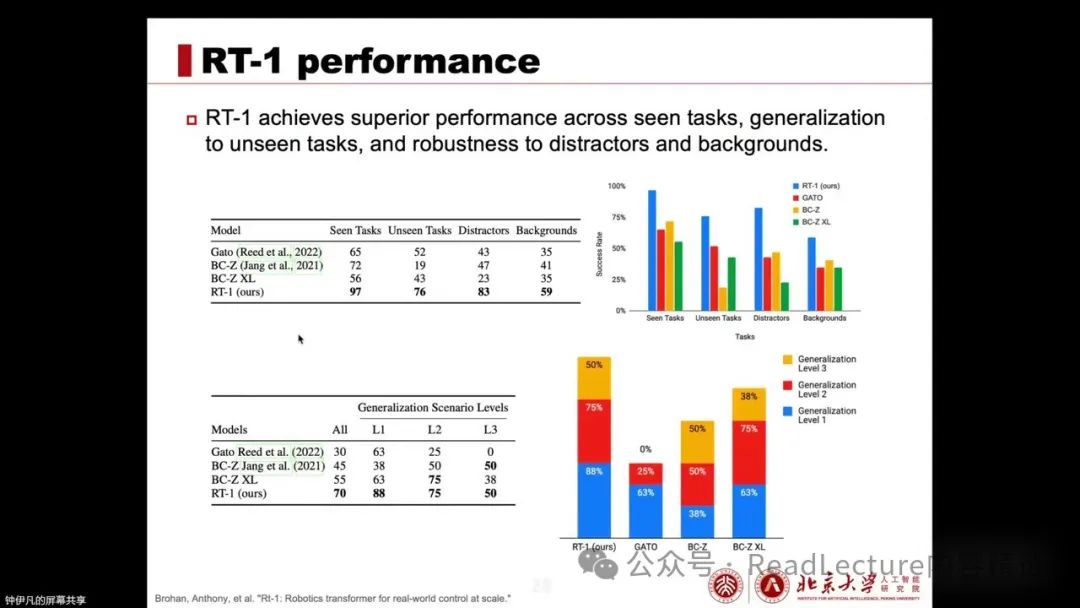
Next, let’s look at the performance of RT-1. First, RT-1 outperforms previous works in both seen and unseen tasks, as well as in scenarios with distracting objects or different backgrounds, showing stronger generalization capabilities. In terms of generalization across varying difficulties, RT-1 also performs excellently.
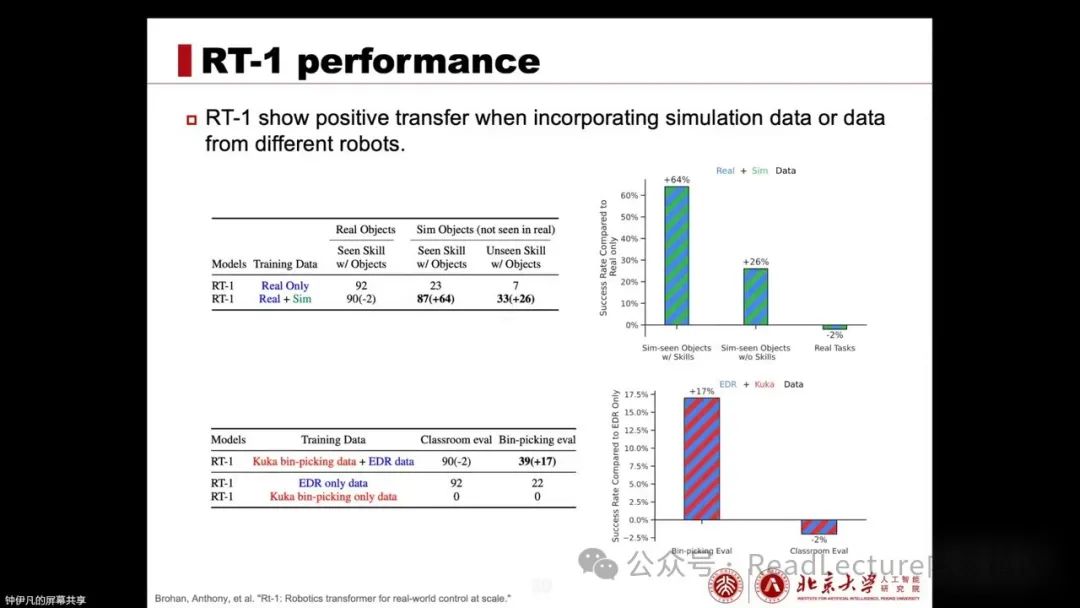
All outperform the original. At the same time, RT-1 conducted an experiment to verify the impact of retraining the existing RT-1 model on RT-1 and combining some simulated data or other embodiment intelligence data for training. The experimental results show that if simulated data or data from other robots is included in training, RT-1 demonstrates strong positive transfer capabilities, with the original performance remaining largely unchanged. However, the performance exhibited in other simulated data and data from other robots can also be achieved on its own robot. This indicates that RT-1 can learn and apply the contents from this data.
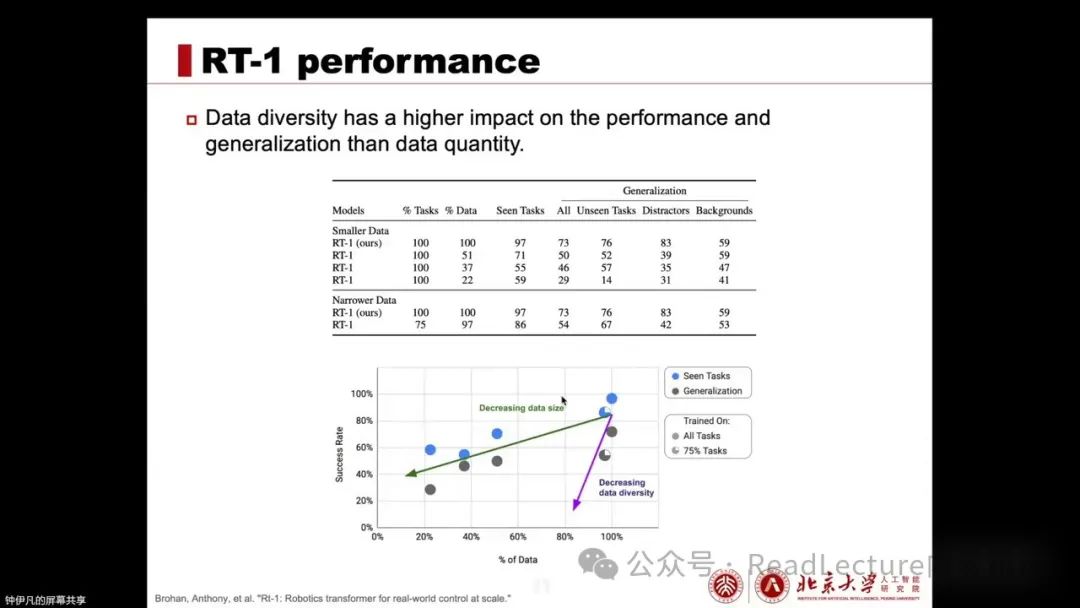
Additionally, it conducted an experiment to verify how performance would decline if the data volume is reduced. The experiment found that if data diversity remains unchanged but the data volume decreases, or if the data volume decreases slightly but data diversity significantly decreases, the comparison between these two groups of experiments indicates that data diversity has a greater impact on performance and generalization ability than data volume. Therefore, data diversity is very important.
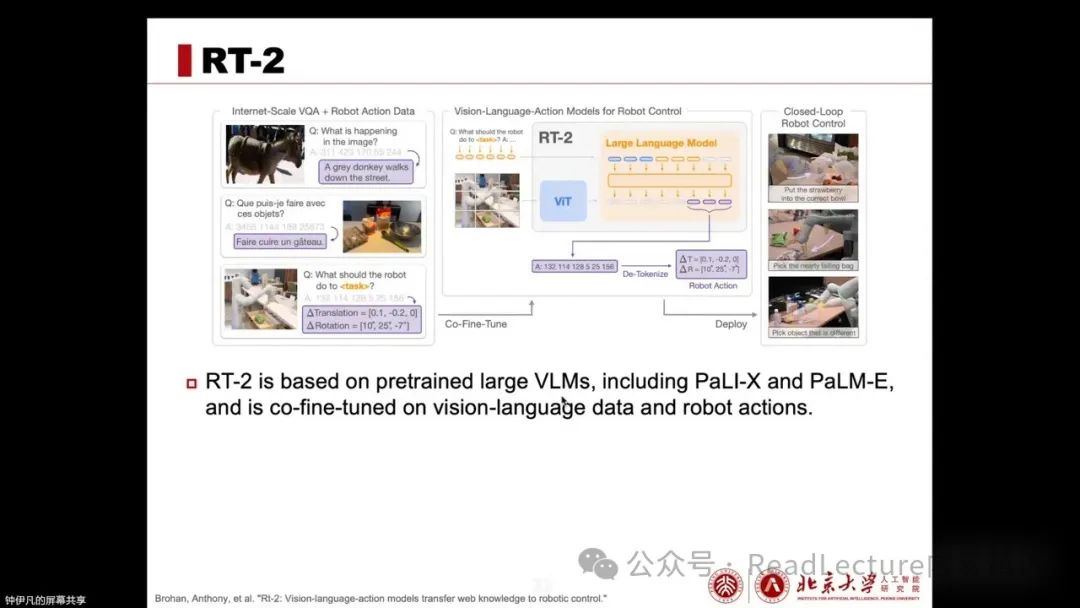
After introducing RT-1, let’s look at RT-2. RT-2 is the next model in the RT series, which differs from RT-1 in that it uses pre-trained VLMs (Vision-Language Models) such as PaLI-X and PaLM-E. Both of these models have been trained on vision-language tasks. Building on these models, RT-2 further co-fine-tunes on visual-language data and robotic actions.
The co-fine-tuning method involves inputting from VIT and Language Model, with outputs sometimes being text and sometimes robotic actions. To align actions with text in the same space, as with RT-1, actions need to be discretized and correspond to the VLM’s token vocabulary. Since the vocabulary of VLM is extensive while actions are limited to 256 tokens, during action reasoning, only action tokens will be sampled, while other language tokens will be masked. This ensures that only action tokens are sampled during action reasoning.
Due to the large size of the model, e.g., PaLI-X has a maximum of 55B parameters, making it difficult to perform inference on small computing units like robots, it is deployed on multi-TPU cloud services for real-time inference.
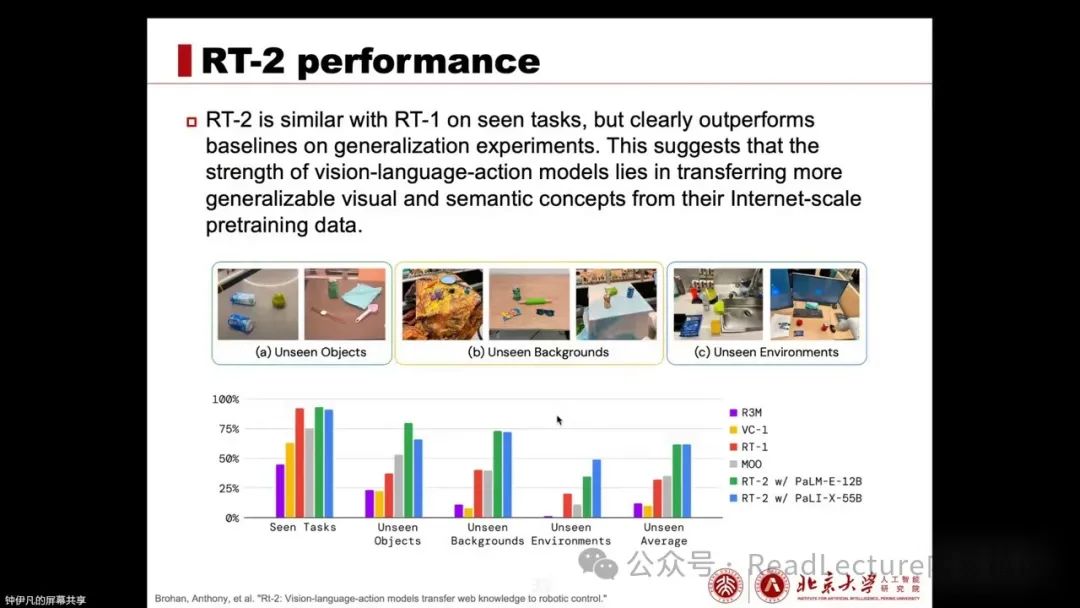
Next, let’s look at the performance of RT-2. Compared to RT-1, RT-2 performs comparably on seen tasks because RT-1 also exhibits very good performance on seen tasks. However, for more general and unseen tasks, RT-2 retains rich world knowledge and masters many such insights through co-training with visual-language data, thanks to the pre-trained vision-language model (VLM). Therefore, in unseen objects, backgrounds, and environments, RT-2’s generalization ability is relatively better.
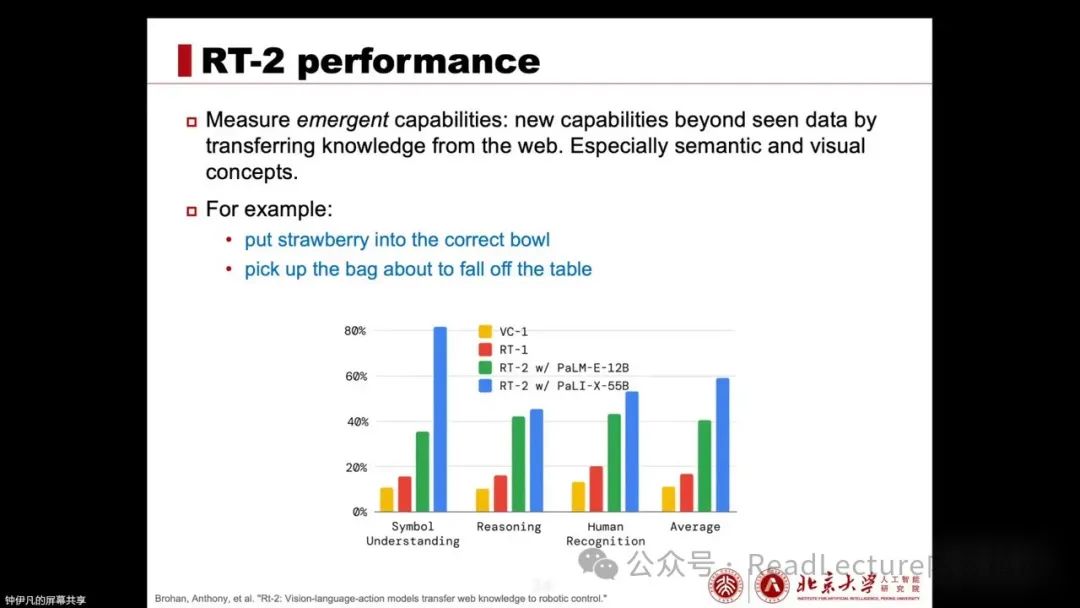
At the same time, this pre-training data from visual-language models endows it with many emergent capabilities. These capabilities mean it can transfer knowledge from online data and apply it in robotic experiments.
For example, there are tasks such as putting strawberries in the correct bowl. This requires it to recognize each bowl according to the classification of different fruits or contents, thus needing to correctly identify strawberries as fruit and place them in the fruit bowl, not in other bowls. Additionally, there are tasks like “pick up the bag about to fall off the table,” which require it to recognize which bag is about to fall and take appropriate action.
These tasks require semantic reasoning capabilities, which are gained from VLM data rather than directly from robotic data. Therefore, this series of experiments demonstrates that RT-2 can showcase visual-language capabilities in robotic scenarios through its VLM backbone network and training on VLM data.
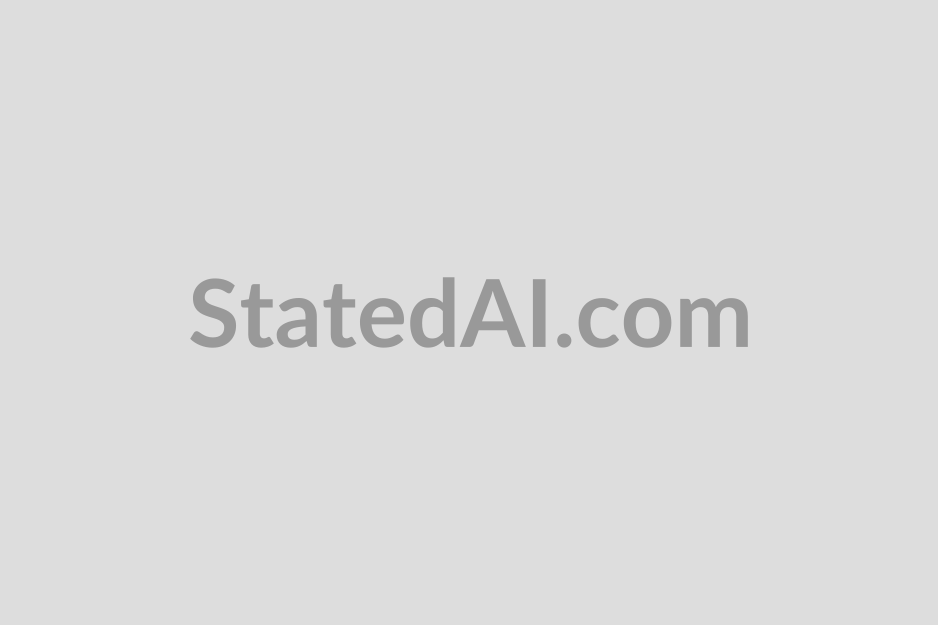
Thus, this method is very effective, and ablation experiments regarding the impact of parameter quantities were also conducted. The experimental conclusions indicate that, first, through co-fine-tuning, better generalization performance can be achieved, and secondly, if the model size increases,
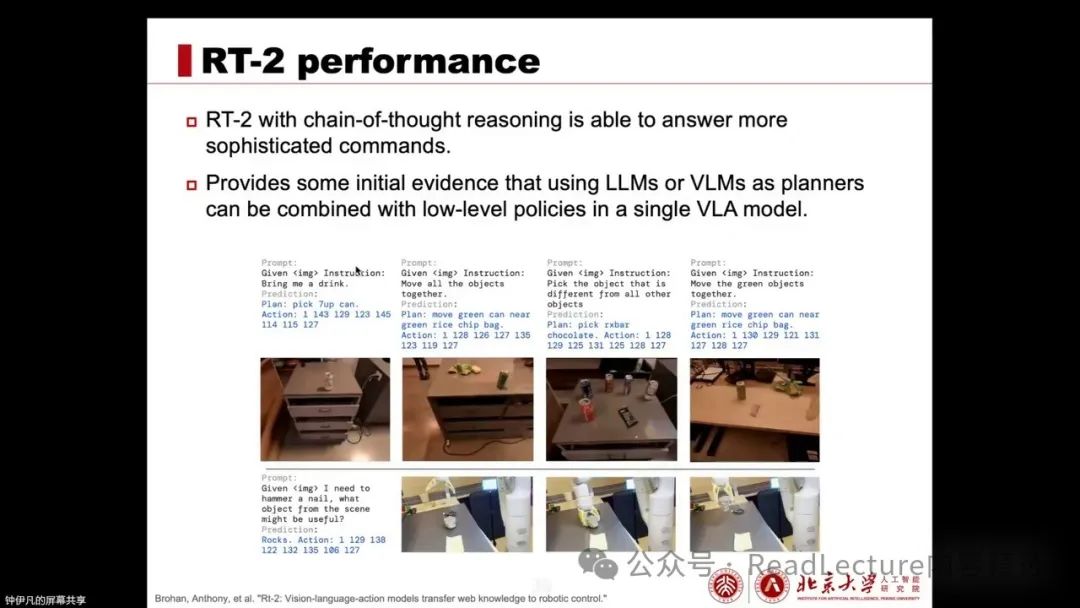
its performance will also improve. At the same time, RT-2, based on VLM, can utilize an effective scheme from VLM, namely chain-of-thought reasoning. If chain-of-thought reasoning is incorporated into the model’s training, it can solve some complex instructions. This confirms that combining the LM and VLM as planners with direct low-level control can be integrated into a VLA model.
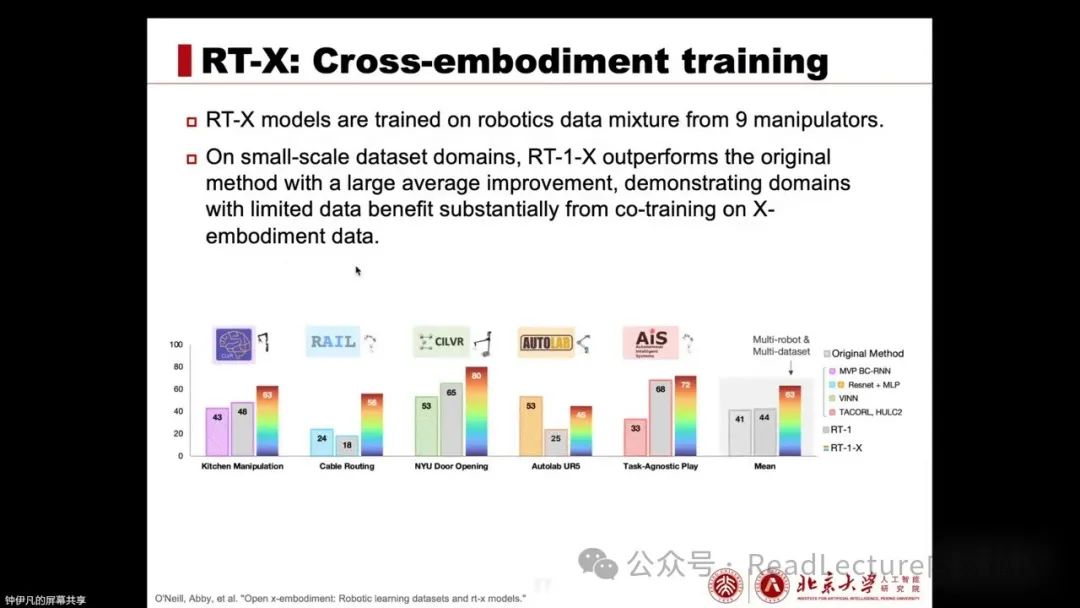
Next, we will discuss a work called RT-X, which trains RT-1 and RT-2 on the OpenXEmbodiment dataset. Due to the OpenXEmbodiment dataset being much larger than the RT-1 dataset, it exhibits more powerful performance after training on a more diverse dataset. This is the contribution of the RT-X series.
RT-X is a model trained on robotic data from nine robotic arms. Experimental results show that, although the model itself did not change, only the data volume increased, RT-1-X can already outperform the previously proposed methods on that dataset. This indicates that it can benefit greatly from the collaborative training of large-scale data on smaller datasets.
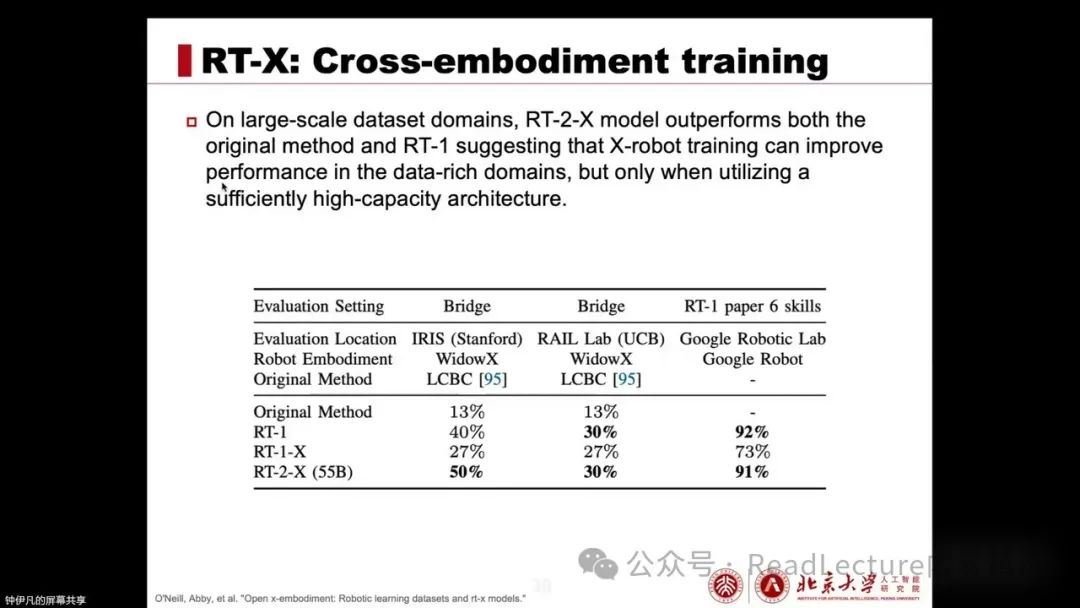
For large-scale datasets, RT-1X performs poorly, while RT-2X performs exceptionally well, not only surpassing RT-1 but also exceeding the original methods proposed on that dataset. This indicates that in scenarios with a large amount of data, to further enhance capabilities, a high-capacity model structure may be required.
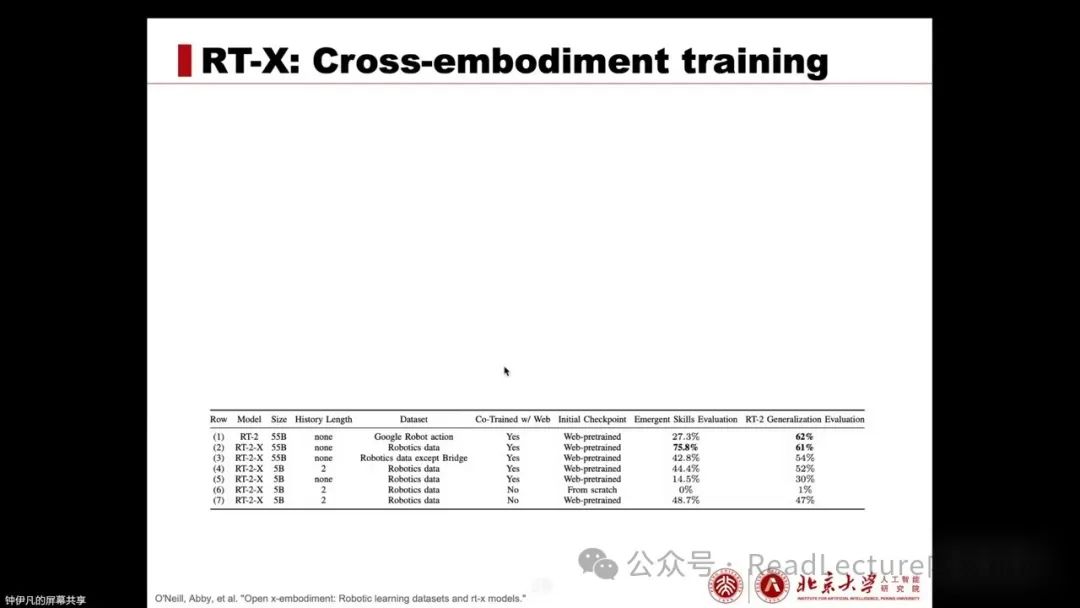
Additionally, it has several other conclusions, which we list as follows:
Using cross-embodiment training allows the RT-X model to learn skills from other training domains. If the input includes image history, it can enhance generalization performance. The model itself utilizes web-scale pre-training, which is very important. Meanwhile, the larger the model, the greater its capacity, and the stronger its ability to transfer skills between different embodiments.
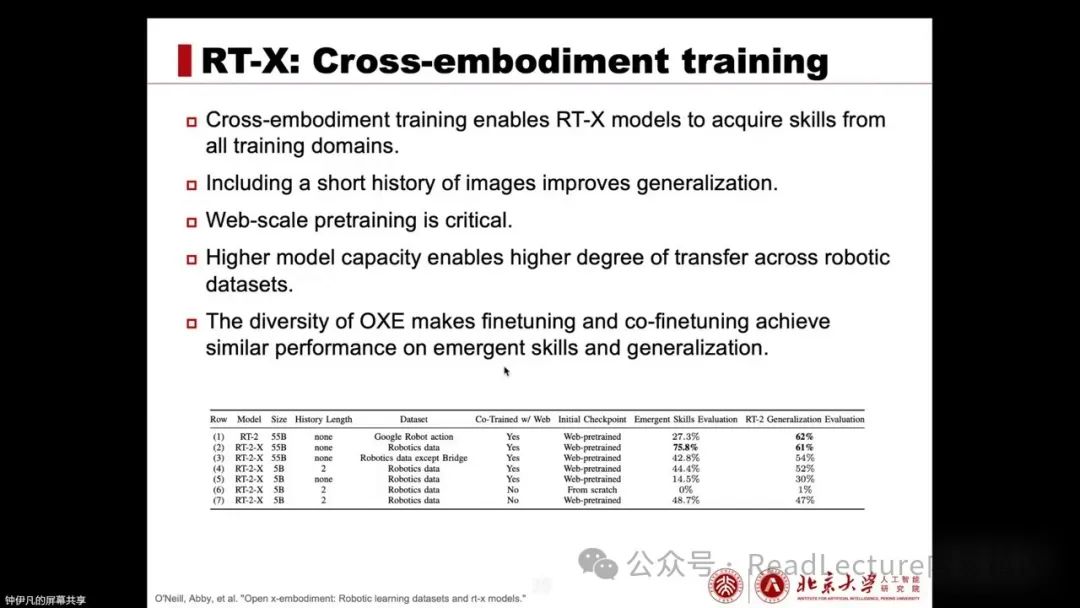
At the same time, the diversity of the OxEm dataset means that during the fine-tuning phase, training solely on the OxEm dataset and co-fine-tuning on both the OxEm and internet data yields similar performance in terms of emergent skills and generalization. This mainly indicates that the OxEm dataset has high diversity.
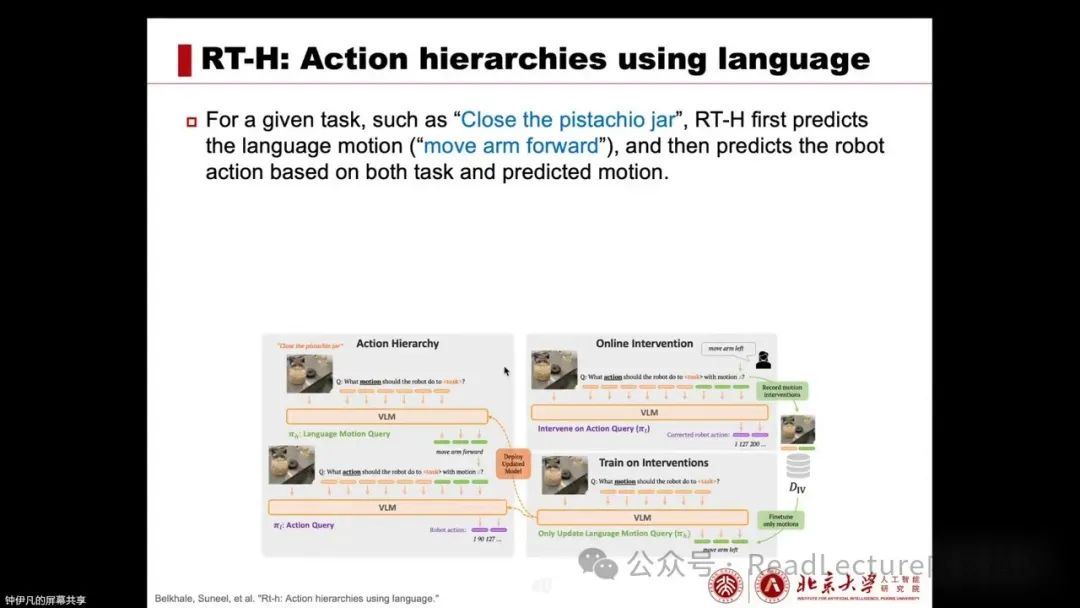
Next, we will introduce a work called RT-H, which improves upon RT-2. The main idea of its improvement is that even though we have a lot of robotic manipulation data, some tasks may look dissimilar at the instruction level, but the action sequences are very similar. For instance, picking up a cup and picking up an apple may be similar, while picking up a cup and putting something down may look dissimilar at the instruction level but have similar action sequences, both requiring first picking up an object, with only the last step differing.
RT-H proposes adding an intermediate layer, namely the language-motion layer, to predict actions for given tasks. For example, for the tasks of “opening a jar” or “closing a jar,” the system first predicts the language action, describing the action to be executed from a linguistic perspective, such as “move the arm forward.” Then, the language action is input into the vision-language action model (VLM) along with the top-level task to predict the specific robotic action.
By doing this, RT-H adds a layer of language action prediction before action prediction, thereby better achieving multi-task data sharing. Even if tasks appear dissimilar at the instruction level, once decomposed into language actions, many parts are similar, and the actions are also relatively similar, promoting better data sharing. RT-H is based on the structure of RT-2 and jointly trained on internet data, language action data, and action data.
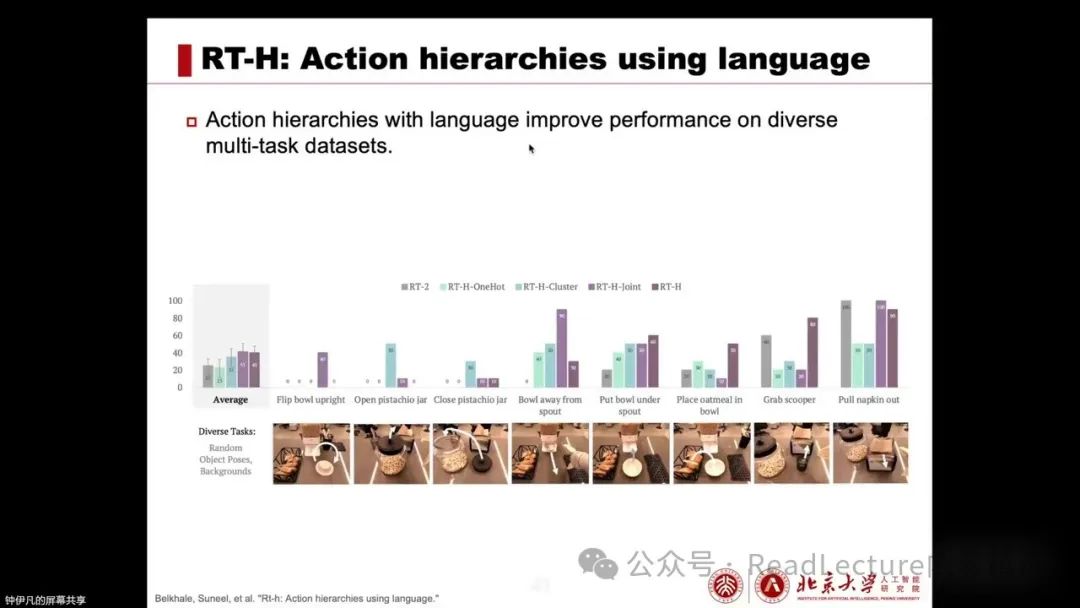
In practice, this action hierarchy approach can enhance performance on diverse multi-task datasets.
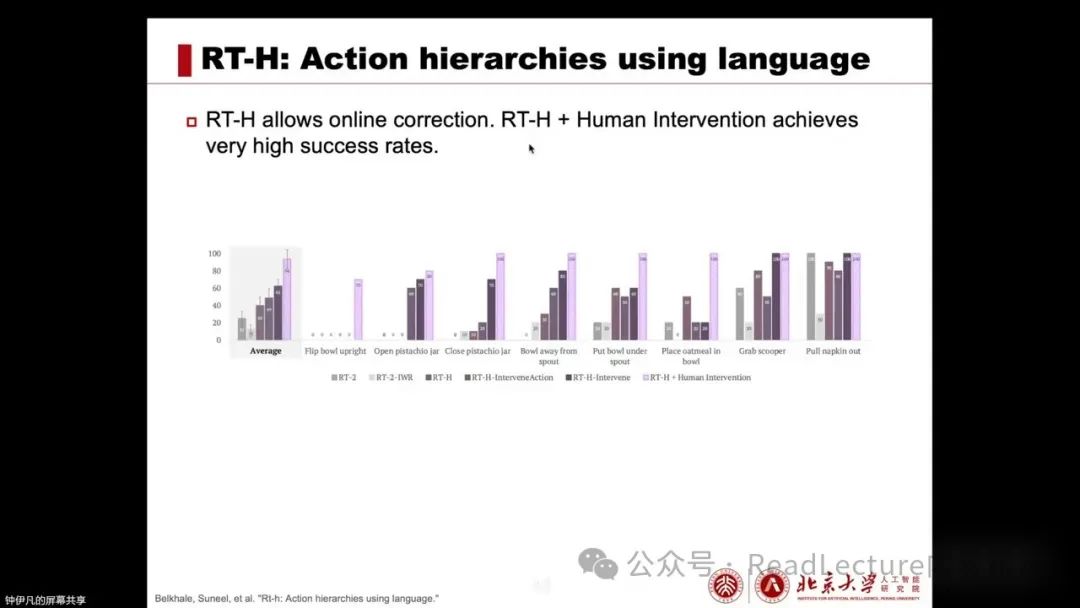
At the same time, RT-H also possesses language action capabilities, allowing humans to easily intervene and correct the model. For example, the current task may be to close a jar lid. The robot will predict the next action, but if it deviates from the target, a human can intervene, modifying its predicted language action to better align with the correct action sequence. Based on this, RT-H will continue to predict.
Since language actions are output in linguistic form, humans can easily check, modify, and correct them, guiding the model to complete the correct task. In this way, with human guidance, the model can complete many tasks with a high success rate.
Furthermore, corrections to language actions can also be used for the model’s learning and training. Experiments have shown that this correction effect is better than manual operation corrections. They also found that RT-H can generalize to new scenes, new objects, and even new tasks with just a few corrections, achieving good generalization effects.
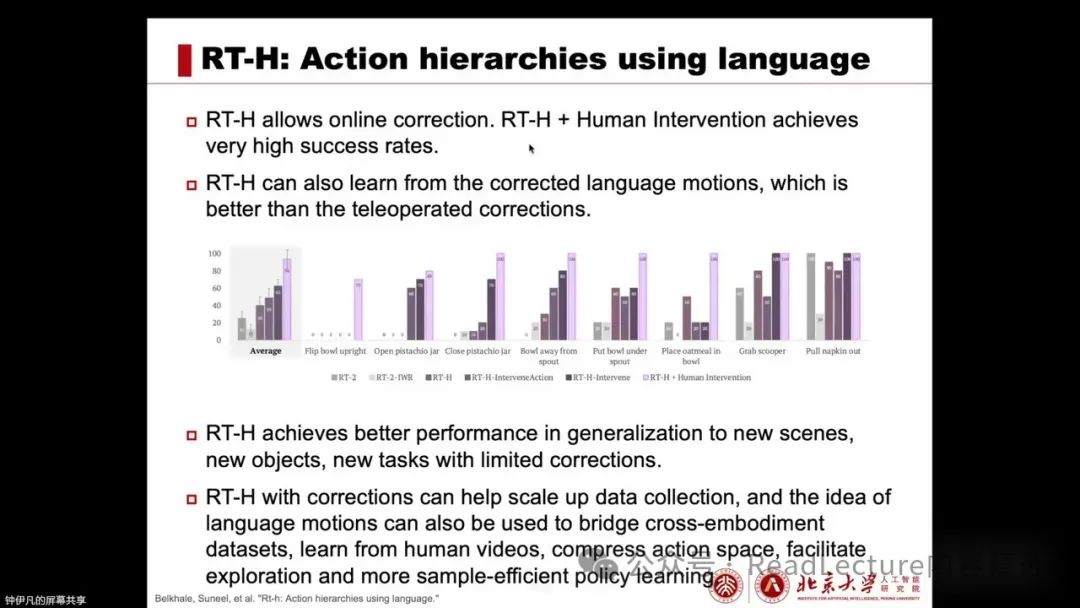
Finally, the entire thought process of this article teaches us that RT-H introduces a structure such as language motion. Through correction schemes, it can effectively enhance the efficiency of data collection. Meanwhile, the idea of language motion as an intermediate layer can connect datasets from different embodied forms. Furthermore, it allows us to learn from human videos and compress the action space.
For some simple tasks, language motion may only have a few options, but for more complex embodied tasks, such as zero-hand tasks, language motion may not be as simple. However, in this task, the conclusion holds, namely that language motion can compress the action space. Additionally, due to the lower dimensionality of language motion, it can also promote exploration by the model, making strategy learning more sample-efficient.
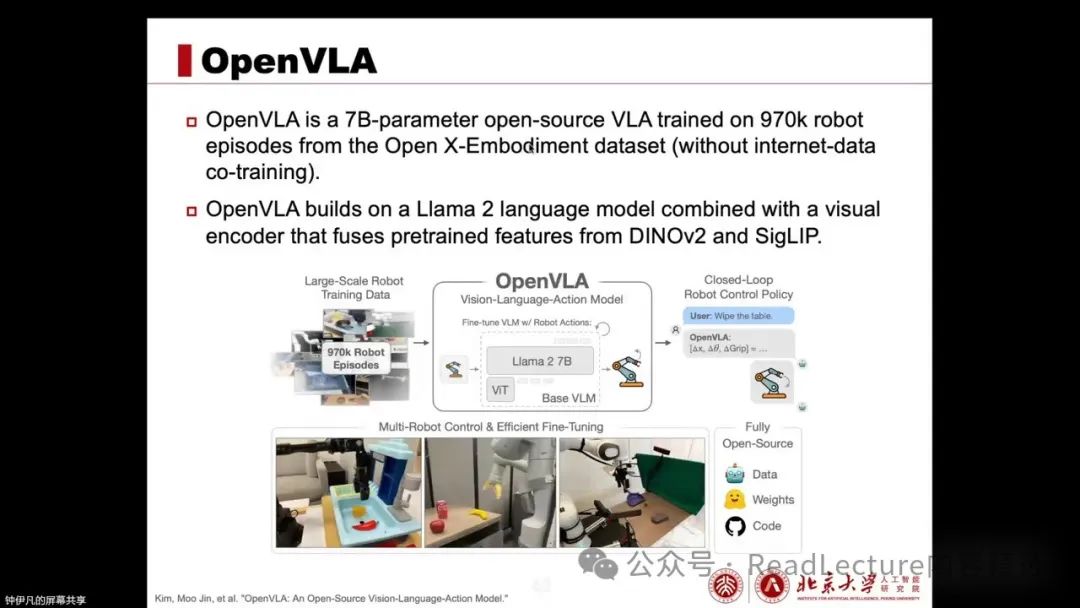
Next, we introduce a work called OpenVLA, which improves upon previous methods mainly in two aspects: first, its training dataset is larger and more diverse; second, its model structure has also been improved.
OpenVLA is trained on the OpenX-Embodiment’s 970K robotic operation dataset, and it does not use internet data for joint training, only training on robotic data. The previous RT-2 used PolyX and POME, while OpenVLA is based on PrismaticVLM, which combines the Llama27B language model, DynoVR, and SigLiP visual encoders.
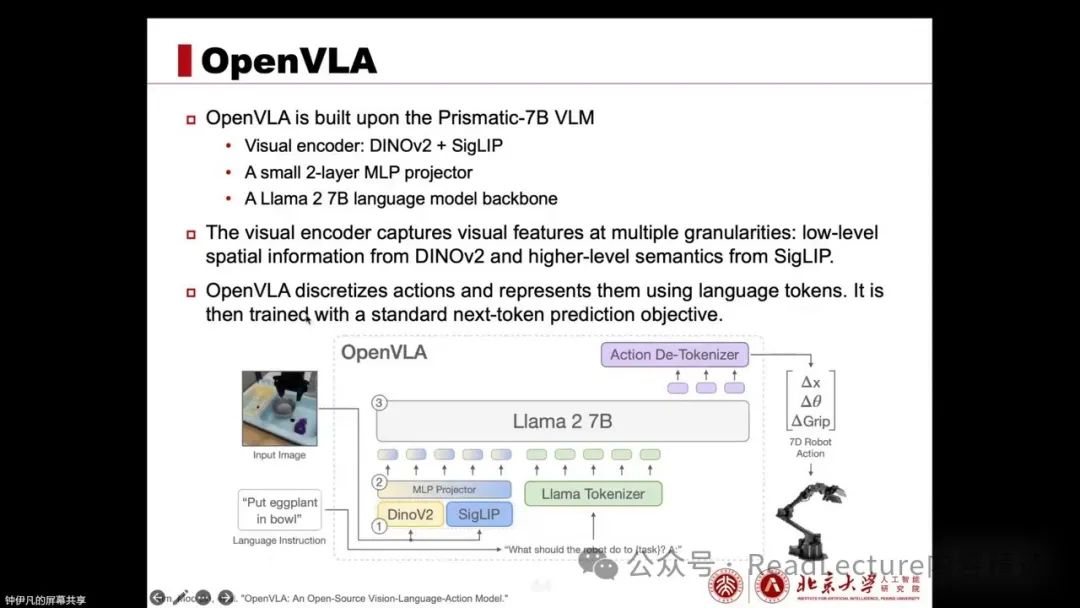
In other words, its composition includes input images, extracting low-level spatial information and high-level semantic features through DINOv2 and SigLIP, respectively. Then, through a 2-layer MLP projector, these features are projected into the token space of the language model. Finally, through the Llama2 7B language model, it directly outputs an action.
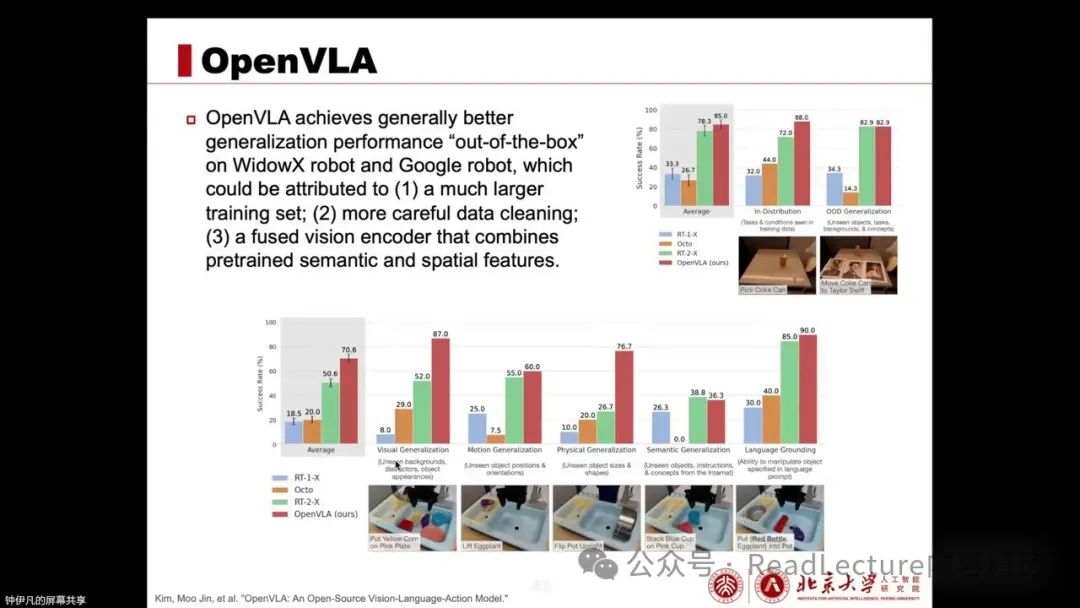
Its performance has been validated through experiments. For example, after being trained on the large-scale OXE dataset, the model demonstrates strong out-of-the-box execution capabilities, outperforming previous models such as RT-1, RT-2, and Octo on multiple datasets. This is primarily attributed to its larger and more diverse training set and meticulous data cleaning. Simultaneously, it uses a visual encoder that integrates DINOv2 and CGleaf.
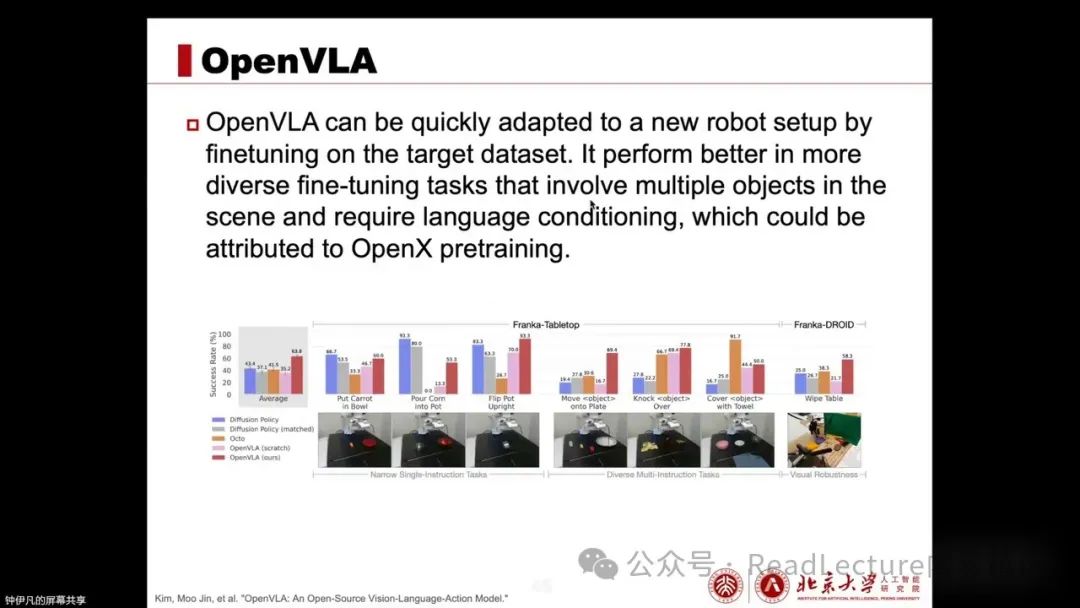
At the same time, another contribution of OpenVLA is exploring the fine-tuning performance of the VLA model. After completing pre-training, it tests whether OpenVLA can quickly fine-tune on specified tasks. It conducts some rapid fine-tuning experiments on target tasks and evaluates the effects.
The results show that OpenVLA performs better than previous methods on complex, diverse tasks involving multiple instructions and objects. This is mainly due to the strong performance brought by OpenX’s pre-training. Compared to training from scratch, OpenVLA’s performance significantly improves, primarily due to the powerful performance provided by OpenX’s embodied intelligence pre-training.
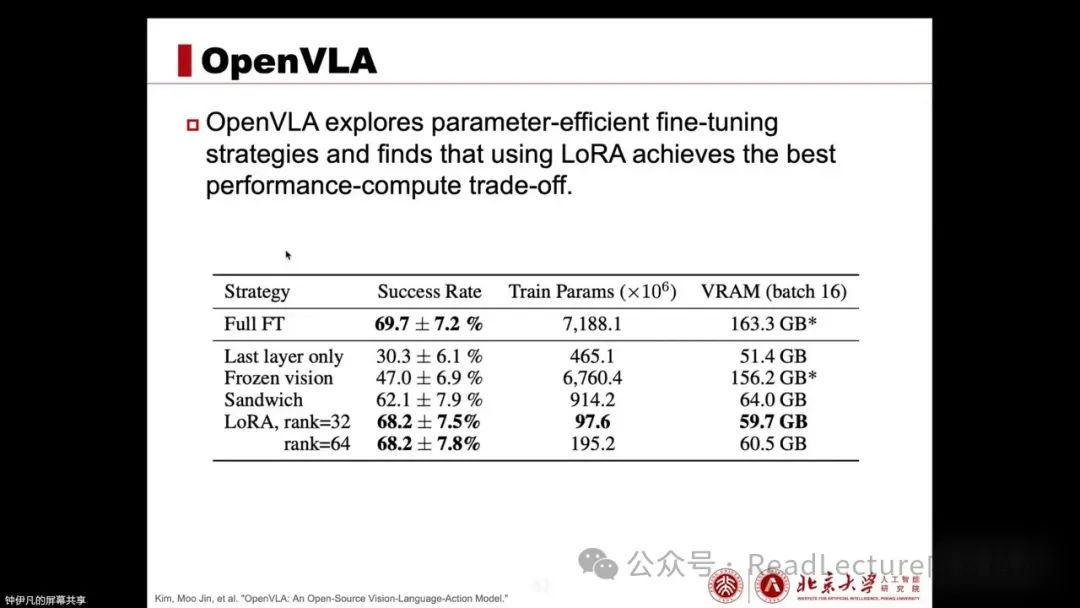
At the same time, it also explores parameter-efficient fine-tuning. The research finds that LoRA performs excellently in maintaining performance while consuming fewer computational resources, making it a very effective parameter-efficient fine-tuning method. This allows us to fine-tune large VLA models under low-resource conditions.
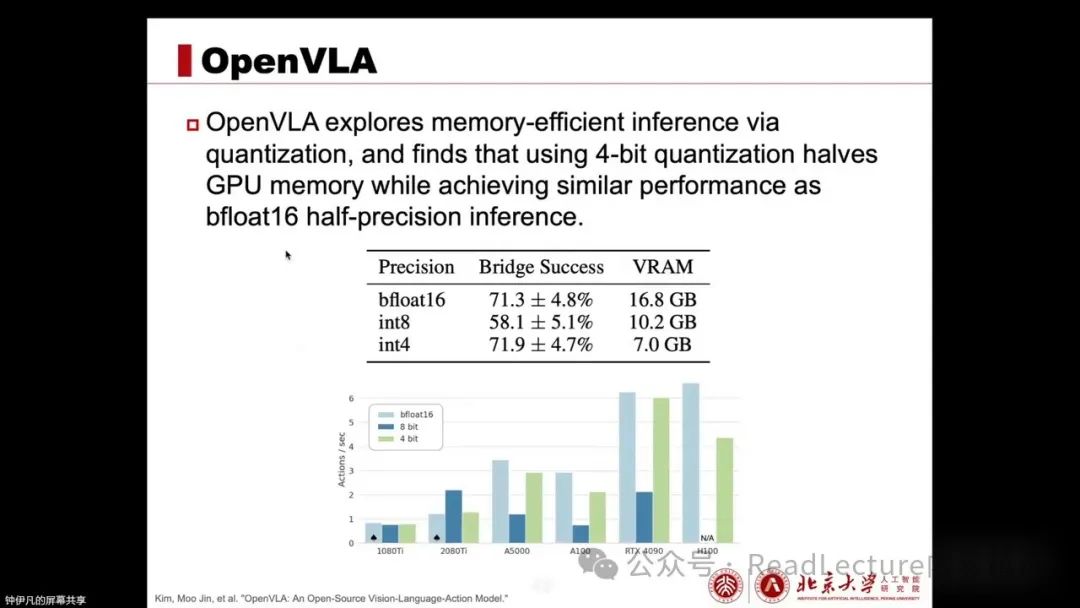
It also validates memory-efficient inference. Through this quantization approach, experimental results indicate that using 4-bit quantization can halve GPU memory while maintaining performance close to the original. These are significant contributions found in the experiments, providing references and considerations for our future VLA research.
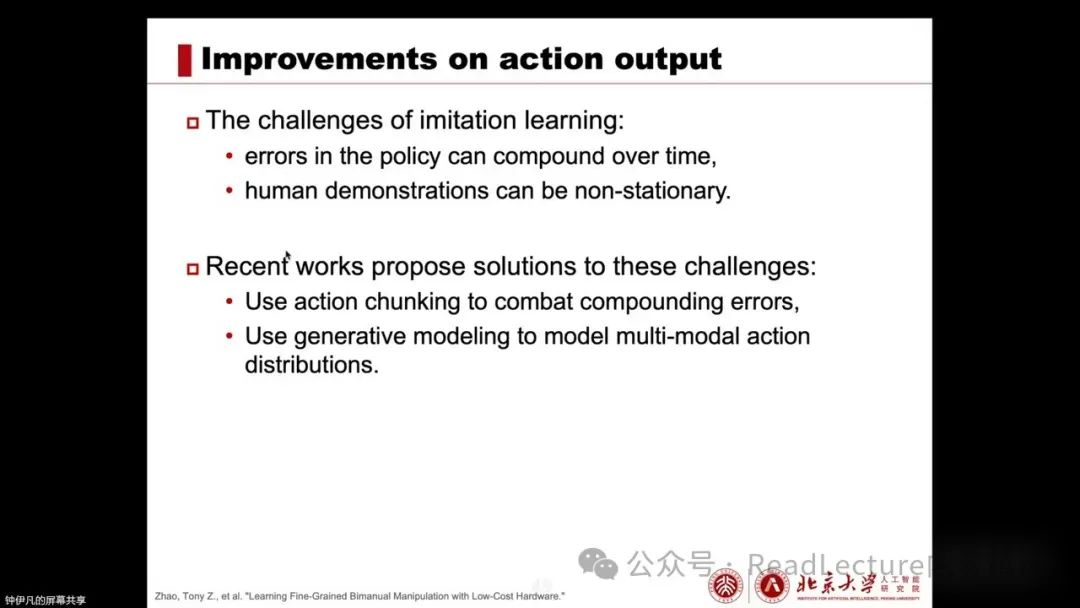
Having introduced so many vision-language action model works, we can summarize what we have gained, limitations, and future work. First, the training methods of these models usually involve pre-training on large, diverse datasets to learn some general and widely applicable robotic manipulation skills. Then, fine-tuning on high-quality datasets for target tasks to improve performance.
Experimental observations indicate that pre-training on large-scale diverse datasets primarily brings zero-shot generalization capabilities across various tasks and enhances the efficiency of subsequent fine-tuning. At the same time, experiments also verify that even in cases of insufficient data, training across embodiment forms can lead to positive transfer across embodiments.
Utilizing high-performance visual and text encoders trained on web-scale data can bring strong instruction-following capabilities, world knowledge, generalization, and emergent skills to robotic manipulation strategies. Transferring knowledge from online data to real-world scenarios brings semantic reasoning capabilities.
Most experiments indicate that in the action output part, using action chunking combined with generative models usually shows strong performance, potentially outperforming single-step autoregressive generation.
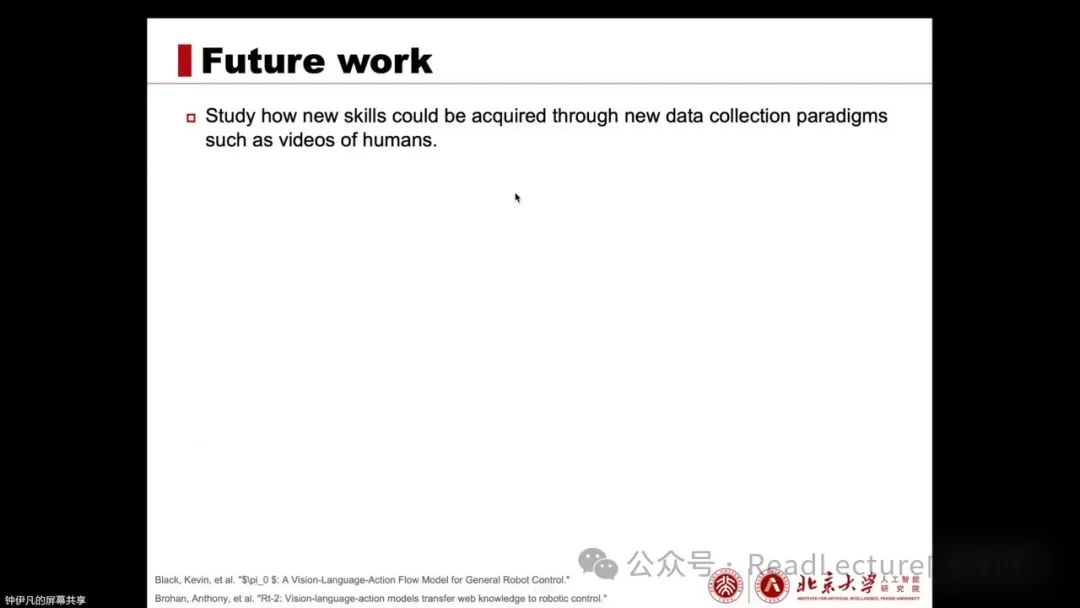
Now, let’s look at the current limitations. First, a major limitation is that all methods discussed today are imitation learning methods, thus facing the challenges of imitation learning. For example, they cannot surpass the performance of the demonstrators. At the same time, although there is some generalization capability, it is still far from universal generalization. Further improvements can be made for unseen objects, environments, tasks, especially for unseen actions and embodiment forms. Additionally, further improvements can be made in aligning or training data across embodiment forms. Recently, the paper by Kai Ming’s group, HPT, proposed some solutions in this regard, and interested students can take a look.
For long-range tasks, the current methods still face challenges. So what future work is worth pursuing, or what has not been done well and needs research? First, as mentioned earlier, it is currently challenging to generalize to new actions and new skills. Is it possible to learn new skills that are not present in RobotData through new data collection methods or by learning from human videos?
We also want to know how the pre-training dataset should be structured, and in slightly more complex tasks, while current performance has some capabilities, it cannot be said to completely solve the tasks, and there is still a distance to achieve high-quality task completion. This requires us to explore what kind of data is needed and how much data is required to achieve near-perfect performance.
Moreover, we want to know how much positive transfer might occur with very diverse data. We hope it happens, but we also do not want excessive diversity to negatively impact performance. At the same time, we hope to continue to build large and high-quality datasets or discover efficient training methods or have efficient data sources. For example, NLP uses self-supervised methods or methods of language modeling on internet data for model pre-training. At this time, the data sources are very efficient because they can be directly collected from the internet, with many sources and large quantities. Training methods also do not require human annotation, making them very efficient. We also hope to have such data sources and methods in the robotics field.
Thus, we can continue to build VLA foundation models to complete robotic manipulation tasks. Additionally, we can consider using simulated data. Current models do not utilize simulated data much. Furthermore, since all methods are imitation learning, we can also consider using reinforcement learning methods to further improve performance.
The references I shared during the presentation are listed above. That concludes the content, thank you.
👉 “The Artifact is Coming” ReadLecture summarizes lecture videos in one click, with text and images, 2 hours of video in 5 minutes of reading!
Previous Highlights
-
Deep and hardcore interpretation by the Peking University alignment team: OpenAI o1 opens the “post-training” era of reinforcement learning new paradigms
-
The Chinese Generative AI Conference is about to land in Shanghai, with comprehensive explanations of large models, AI Infra, edge AI, video generation, and embodied intelligence, with 30+ heavyweight guests to preview!
-
Embodied intelligence is the most effective way to achieve AGI (Yushu Technology Founder & CEO Wang Xingxing)
-
Key technology research on embodied intelligence: manipulation, decision-making, navigation (Peking University Dong Hao)
-
Foundation model construction for embodied intelligence object manipulation skills (National University of Singapore Shao Lin)
-
Embodied multimodal large model systems for general-purpose robots (Peking University Wang He)
-
Building embodied consciousness (Tsinghua University Aerospace Institute Associate Professor Sui Yanan)
-
[PPT + Manuscript + Notes] Sun Yat-sen University Lin Jing: APR Multimodal Perception and Interaction for Embodied Intelligence
-
[2/12] Reinforcement fine-tuning + dozens of data = PhD experts? Domestic tech giants are about to revise next year’s technical planning… OpenAI 12 Days release conference day two