Posted by: ArduinoTEAM — May 26th, 2020
Running Machine Learning (ML) on microcontrollers is one of the most exciting developments in recent years, allowing small battery-powered devices to detect complex movements, recognize sounds, or find anomalies in sensor data. To make it accessible for every embedded developer to build and deploy these models, we have launched top-notch support for Edge Nano on the Arduino Nano 33 BLE Sense and other 32-bit Arduino boards.
The trend of running ML on microcontrollers is known as Embedded ML or TinyML. This means that devices can make intelligent decisions without sending data to the cloud—this is great from both efficiency and privacy perspectives. Now, even powerful deep learning models (based on artificial neural networks) can be applied to microcontrollers. Over the past year, significant progress has been made to make deep learning models smaller and faster to run on embedded hardware through projects like TensorFlow Lite Micro, uTensor, and Arm’s CMSIS-NN; however, creating high-quality datasets, extracting the right features, training, and deploying these models is still complex.
With Edge Impulse, you can now quickly collect real-world sensor data, train ML models on the cloud, and then deploy the models back to Arduino devices. From there, you can integrate the model into your Arduino sketch with a single function call. This way, your sensors become smarter and can understand complex events in the real world. Built-in examples allow you to collect data from accelerometers and microphones, but it’s easy to integrate other sensors with just a few lines of code.
Excited? Here’s how to build your first deep learning model using Arduino Nano 33 BLE Sense (there’s also a video tutorial: Setting Up Arduino Nano 33 BLE Sense with Edge Impulse: https://docs.edgeimpulse.com/docs/arduino-nano-33-ble-sense).
Sign up for a free Edge Impulse account!
-
Install Node.js and Arduino CLI.
-
Insert the Arduino Nano 33 BLE Sense development board.
-
Download the Arduino Nano 33 BLE Sense firmware—this is a special firmware package (source code) that contains all the code for quickly collecting data from its sensors. Launch the Flash script suitable for your platform to refresh the firmware.
-
Start the Edge Impulse daemon and connect your board to Edge Impulse. Open a terminal or command prompt and run:
$ npm install edge-impulse-cli -g
$ edge-impulse-daemon
-
Collect some data and build a model. We have organized two end-to-end tutorials: Detecting gestures using accelerometers or detecting audio events using microphones.
-
Now, your device will appear in the “Devices” tab of the Edge Impulse studio, allowing you to collect some data and build a model.
-
Once completed, you can deploy the model back to Arduino Nano 33 BLE Sense. It can be a binary file containing the complete ML model or an Arduino library integrated into any sketch.
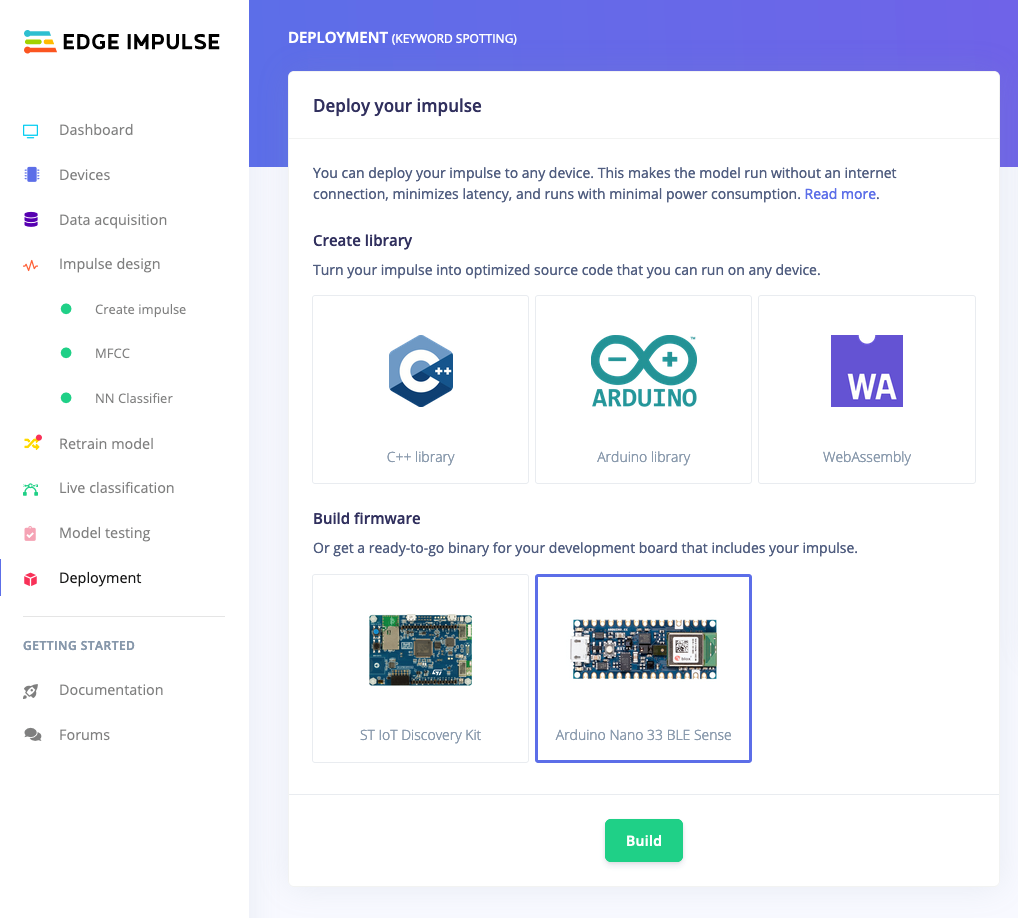
-
Your machine learning model is now running on the Arduino board. Open the serial monitor and run “AT + RUNIMPULSE” to start classifying real-world data!
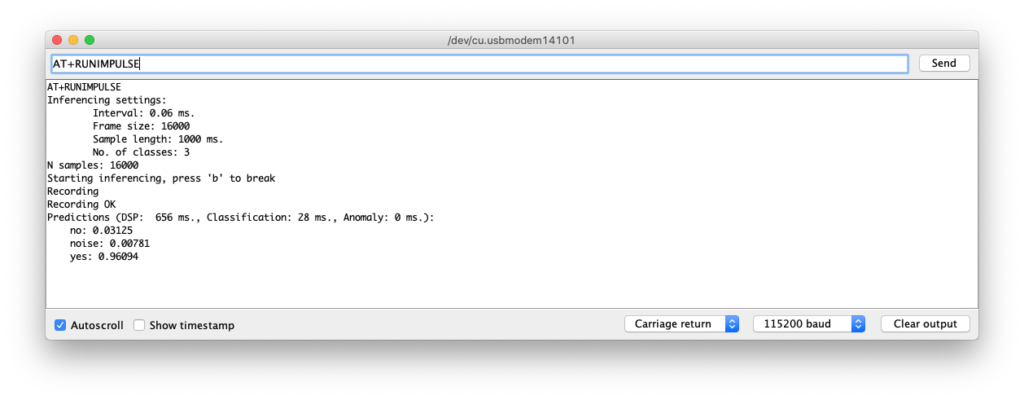
Integrate with Your Favorite Arduino Platform
END
Yinghe Academy
The Yinghe team is dedicated to providing electronic engineers and students in related fields with standardized core skills courses, helping everyone effectively enhance their professional capabilities at various stages of learning and work.
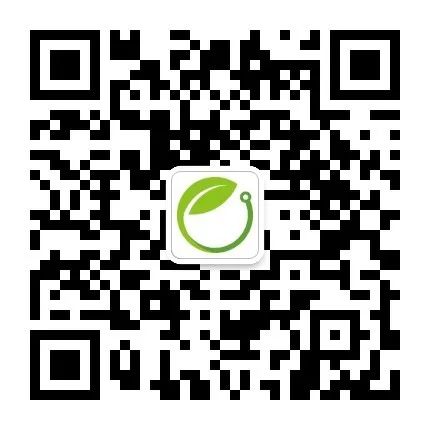
Yinghe Academy
Exploring the electronic field together
Follow the Yinghe public account for instant access to classes
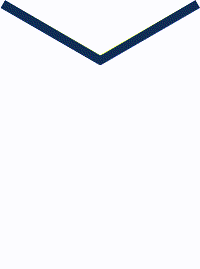
Click to read the original text for more