With the rapid development of smart automotive technology, in-vehicle entertainment and information systems have become an indispensable part of modern vehicles. These systems not only cater to the entertainment needs of the car owner but also involve multiple functions such as navigation, driving assistance, and vehicle networking.
As technology continues to advance, the demand for computing power in in-vehicle systems is constantly increasing. To meet this demand, the combination of edge computing and Graphics Processing Unit (GPU) computing power is becoming one of the key technologies to enhance the performance of in-vehicle entertainment and information systems.
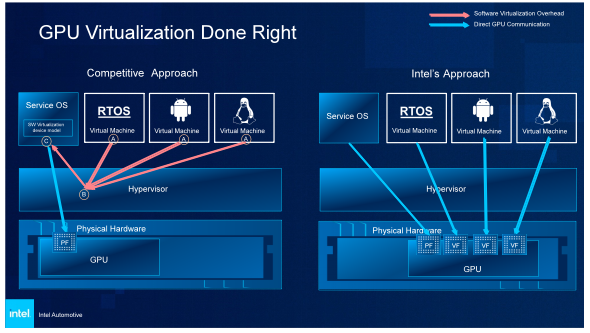
This article will explore in detail the principles, algorithm logic, application scenarios, current status, and future development trends of edge GPU computing.
1. Principles of Edge GPU Computing
1.1 Overview of Edge Computing
Edge computing refers to a computing framework that pushes data processing capabilities from the cloud to the network edge. Traditional cloud computing relies on transmitting data to remote data centers for processing, while edge computing deploys processing capabilities closer to the data source to reduce latency, improve bandwidth utilization, and ensure data real-time processing and security.
Through edge computing, sensors, cameras, and other devices within the vehicle can interact directly with local computing units, reducing latency and improving computational efficiency.
1.2 Basic Concepts and Advantages of GPUs
GPUs were originally used for graphics processing, but with the development of fields such as deep learning and computer vision, their applications have gradually expanded to computing. Compared to traditional Central Processing Units (CPUs), GPUs possess more powerful parallel computing capabilities, allowing them to process large amounts of data simultaneously, thus having significant advantages in handling large-scale data.
GPUs are particularly suitable for applications that require massive parallel data processing, such as video processing, image recognition, and machine learning.
1.3 Edge GPU Computing Power
Edge GPU computing power refers to the ability to efficiently process data using GPUs in an edge computing environment. It combines the low latency of edge computing with the high parallel processing capabilities of GPUs, making it especially suitable for in-vehicle entertainment and information systems that require real-time, low-latency, and high computational demands.
Edge GPU computing can efficiently process large amounts of data generated by in-vehicle cameras, sensors, and other devices, performing real-time image processing, video analysis, voice recognition, and other tasks, thereby enhancing the overall performance of in-vehicle systems.
2. Algorithms for Edge GPU Computing
2.1 Image Processing and Computer Vision Algorithms
In-vehicle entertainment and information systems need to process large amounts of video and image data in real-time, such as front, rear, and surround images captured by in-vehicle cameras.
Utilizing the parallel computing capabilities of GPUs can accelerate the image processing process. For example, tasks such as image classification, object detection, and autonomous driving assistance systems based on deep learning can all be accelerated using GPUs. Common algorithms include:
2.2 Video Stream Processing and Compression Algorithms
In-vehicle entertainment systems need to process video streams from multiple cameras in real-time, performing encoding, decoding, processing, and transmission. The powerful computing capabilities of GPUs can accelerate the video stream processing, ensuring smooth playback of high-definition video in in-vehicle systems.
Video compression and decompression algorithms, such as H.264 and HEVC, can significantly reduce processing time and improve the system’s real-time response capabilities through GPU acceleration.
2.3 Voice Recognition and Natural Language Processing
Voice recognition is a key technology in modern in-vehicle entertainment and information systems, allowing users to control the in-vehicle system via voice, reducing operational burdens during driving.
Edge GPU computing can accelerate voice recognition algorithms, such as Deep Neural Networks (DNN) and Long Short-Term Memory (LSTM), making in-vehicle voice assistants smarter and more responsive. Key steps in voice recognition include:
2.4 Autonomous Driving and Driving Assistance Algorithms
Autonomous driving and driving assistance systems are core applications of in-vehicle information systems, relying on a large amount of sensor data (such as radar, LiDAR, cameras, etc.) to analyze the surrounding environment in real-time.
Edge GPU computing can process these sensor data in real-time, performing complex calculations such as object recognition, path planning, and behavior prediction. Common algorithms include:
Deep Reinforcement Learning: Used for path planning and decision-making in autonomous vehicles.
3. Application Scenarios of Edge GPU Computing
3.1 In-Vehicle Entertainment Systems
In-vehicle entertainment systems need to provide rich audio and video content, supporting real-time streaming, high-definition movie decoding, gaming, and other functions.
Edge GPU computing enables these functions to be efficiently realized, especially during long-distance driving, providing a smoother and higher quality entertainment experience. For instance, when playing high-definition videos on the in-vehicle central control screen, GPUs can accelerate video decoding to ensure smooth playback of high-resolution videos.
3.2 Autonomous Driving and Driving Assistance
Autonomous driving is a revolutionary application within in-vehicle systems. The role of edge GPU computing in autonomous driving mainly lies in real-time perception, decision-making, and control.
Through GPU-accelerated computer vision and deep learning algorithms, real-time analysis of road conditions, obstacles, and other traffic participants can be performed to support decision-making in autonomous driving systems.
3.3 Vehicle-to-Everything (V2X)
V2X is the foundation for achieving intelligent transportation, allowing vehicles to exchange data through in-vehicle communication systems, enhancing driving safety. The combination of edge computing and GPU computing can enable real-time interaction between in-vehicle devices and other traffic facilities (such as traffic lights, traffic signs, etc.).
Data processing accelerated by GPUs can improve the response speed and accuracy of V2X systems, reducing traffic accidents and congestion.
3.4 Intelligent Navigation and Traffic Condition Analysis
Applications of edge GPU computing in intelligent navigation systems mainly involve analyzing and processing real-time traffic information. The in-vehicle system obtains real-time traffic condition data through sensors and networks, utilizing GPU acceleration to process and provide optimal route choices for drivers.
This can not only reduce traffic congestion but also enhance the driving experience.
4. Current Status and Development Trends
4.1 Current Status
Currently, many high-end smart automotive brands have begun to adopt edge computing and GPU acceleration technologies. For example, Tesla, BMW, and Mercedes-Benz have all implemented GPU-accelerated processing technologies in their autonomous driving systems and in-vehicle entertainment systems, enhancing the intelligence level of their vehicles.
However, despite the achievements in applying edge GPU computing in in-vehicle systems, the overall adoption rate remains low, and costs are high.
4.2 Development Trends
5. Conclusion
Edge GPU computing provides strong computational support for in-vehicle entertainment and information systems, especially in areas such as image processing, voice recognition, autonomous driving, and V2X. With ongoing technological advancements, edge GPU computing will play an increasingly important role in driving in-vehicle systems towards greater intelligence and efficiency.
In the future, with further optimization of hardware and algorithms, edge GPU computing will become one of the indispensable core technologies for smart vehicles.