We previously published an article titled “TinyML: The Next Wave of Artificial Intelligence Revolution“, and held several events related to machine learning. We believe everyone has a certain understanding of TinyML, so why did we choose TinyML?
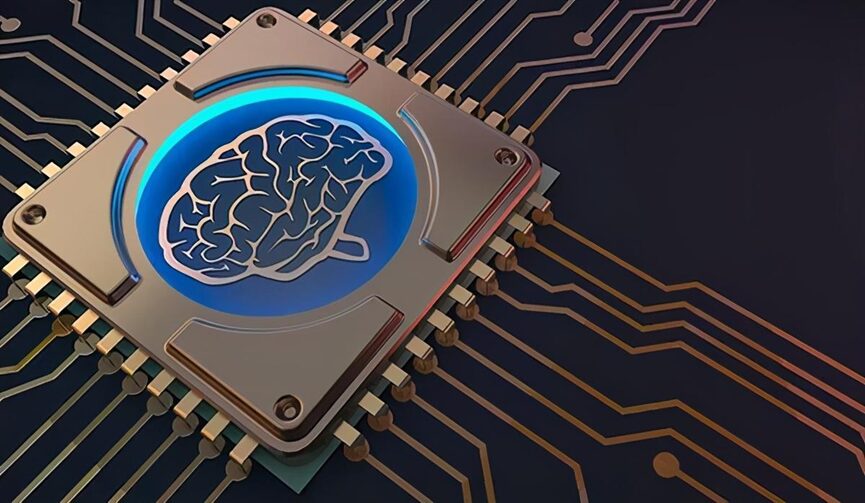
Artificial Intelligence (AI) is rapidly transitioning from the cloud to the edge, entering increasingly smaller IoT devices. These IoT devices are often very small and face many challenges such as power consumption, latency, and accuracy. Traditional machine learning models cannot meet these requirements; so how does micro machine learning fit in?
TinyML takes edge AI a step further, making it possible to run deep learning models on microcontrollers (MCUs), which have far more limited resources compared to the small computers we carry in our pockets and on our wrists.
The working mechanism of TinyML algorithms is almost identical to traditional machine learning models, typically involving model training completed on the user’s computer or in the cloud. Post-training processing is where TinyML truly shines, often referred to as “deep compression”.
Flowchart of deep learning model compression (Source: https://arxiv.org/pdf/1510.00149.pdf)
The main advantage of TinyML is its portability. Running on inexpensive microcontrollers with small batteries and low power consumption means that with TinyML, it is easy to integrate ML into almost anything at a low cost.
Additionally, due to its local computing nature, TinyML enhances security, as there is no need to send data to the cloud. This can be crucial when handling personal data in applications like IoT.
Previously, complex circuits were necessary for a device to perform a variety of actions. Now, machine learning is increasingly making it possible to abstract this hardware intelligence into software, simplifying embedded devices and making them lighter and more flexible.TinyML is set to become part of our daily lives.
Applications of TinyML include:
-
-
Object Recognition and Classification
-
-
-
An example of a TinyML application in daily life is the audio wake word detection model used internally by Google and Android devices. An example of the wake word detection component is shown below.
To “wake up” when hearing the phrase “OK Google”, Android devices use a 14 kB voice detection ML model that runs on a DSP. Many other virtual assistants operate similarly.
Components of the wake word application
Other examples of TinyML applications from Harvard students include road deer detection in cars (an example of object detection), audio-based mosquito detection (an example of audio recognition), and more.
The ability to run machine learning models on resource-constrained devices opens up many new possibilities. The development of AI may make standard machine learning more energy-efficient, helping to alleviate concerns about the environmental impact of data science. Additionally, TinyML allows embedded devices to be endowed with new intelligence based on data-driven algorithms that can be applied to a wide range of areas, from predictive maintenance to bird call detection in forests.
While some ML practitioners will undoubtedly continue to scale up models, a new trend is emerging to develop machine learning algorithms that are more memory, computational, and energy-efficient. Currently, TinyML is still in its infancy, with much room for future development.
Hard禾 is about to launch a three-month design competition, offering not only full refunds but also cash prizes, enriching your resume content. Join the group early to learn more about the competition details.
The Hard禾 team is dedicated to providing standardized core skill courses for electronic engineers and related students, helping everyone effectively enhance their professional abilities at various stages of learning and working.
Hard禾 Academy
Let’s explore and advance together in the field of electronics.
Follow the Hard禾 public account for direct access to classes.
Click to read the original text for more details.