Apache IoTDB is a time-series database designed for the industrial Internet of Things (IoT), developed independently in China and open-sourced to Apache as a top-level project. With the continuous introduction of features such as triggers and UDFs, the application scenarios of IoTDB are becoming increasingly rich. This article focuses on sharing the new features of IoTDB and the considerations for technology selection in industrial IoT application solutions, hoping to assist relevant professionals in making technology choices and overall architecture design.
This Edition’s Guest
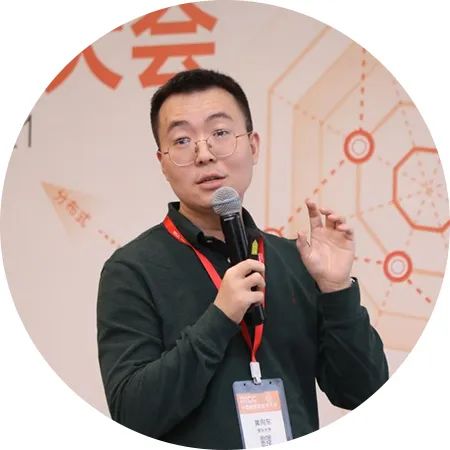
Huang Xiangdong
Assistant Researcher, School of Software, Tsinghua University
[Guest Introduction] Huang Xiangdong is an assistant researcher at the School of Software, Tsinghua University, a young talent supported by the China Association for Science and Technology, a communication committee member of the CCF Database Special Committee, a member of the China Communications Society Open Source Technology Special Committee, a member of the Apache Software Foundation, and Vice President of the Apache IoTDB project. His main research area is big data management technology, focusing on industrial big data management. He has been granted over 30 national invention patents. He has participated in the development of China’s next-generation meteorological big data platform and has been responsible for the development of the industrial IoT time-series database management system software IoTDB, which became the first Apache top-level project initiated by Chinese universities. He has led one project funded by the National Natural Science Foundation of China and one project funded by the China Postdoctoral Science Foundation. He won the first prize for technological invention from the Ministry of Education in 2018 and the first prize for scientific and technological progress from the Chinese Meteorological Society in 2018.
Below is the transcript of Huang Xiangdong‘s speech at the DTCC2021 conference:
IoTDB Project Overview
Industrial IoT time-series data is the digital record of the physical quantities of industrial equipment, consisting of timestamped data that contains rich industrial semantics. IoTDB, initiated by Tsinghua University’s School of Software, is a high-performance, lightweight time-series data management system focused on the industrial IoT, providing functions for data collection, storage, and analysis.
In 2012, General Electric (GE), a leader in international industrial internet, pointed out that “fully utilizing massive time-series data to drive industrial innovation, competition, and growth is a historic opportunity brought by big data technology for the new industrial revolution.”
One can observe the Seattle report in the database field, which points out that a development prospect for databases will form in the next five years. Some reports specifically mention that IoT will bring new challenges to database write queries, including: massive sequence storage, complex metadata management, rich query requirements, and edge-cloud collaboration. Consequently, time-series data has become a focal point in both industry and academia.
If the vertical axis of the industrial IoT time-series data in the figure is removed and replaced with “value,” it will transform from industrial IoT time-series data into time-series data. This shows that real-time time-series data is very common; the behaviors of people, software, and machines continuously generate time-series data.
Regarding TSDB, the explanation on Wikipedia states that a time-series database is software used to store time-series data and index it by time (point or interval). Its main application scenarios are divided into three: APM monitoring, IoT applications, and data analysis. Currently, there are various options for time-series data management systems, including ClickHouse, Druid, HBase, OpenTSDB, Parquet on HDFS/S3, PG/MySQL, TimeScaleDB, MatrixDB, InfluxDB, M3DB, TDengine, Apache IoTDB, Skywalking EMQx, etc. Therefore, different selection solutions will exist when facing different systems.
New Features of Apache IoTDB
IoTDB operates under an open-source model in the Apache community, integrating with a series of open-source software, with the final goal of building an open-source solution for the full lifecycle management of time-series data, covering all aspects from data collection, storage, processing, to analysis and application.
In the collection phase, there are many open-source software options, such as EDGENT and PLC4X. In the analysis phase, Spark and Hive can be used for big data analysis. In the application phase, open-source programs such as Grafana, Calcite, and Karaf can be selected.
From a historical perspective, IoTDB originated from Tsinghua University’s School of Software. In 2017, we open-sourced it. In 2018, many experienced partners in the Apache community assisted in improving IoTDB, gradually transforming it from a demo form to a product form. In 2019, the first version of Apache IoTDB was released.
In fact, it can be seen that the release speed of IoTDB is not very fast, but basically one or two versions are released every year, with improvements in writing, querying, and stability in each version. In 2019, IoTDB optimized the function of reorganizing out-of-order data. In 2020, IoTDB significantly improved query performance and provided new memory control features. In 2021, the community officially launched the IoTDB 0.12 series version and maintained five minor versions from 0.12.0 to 0.12.4.
▶︎ Feature 1: Data Model Defined from Edge Devices
In recent years, the frequency of equipment component upgrades in traditional industrial enterprises has increased. During the system upgrade process, the data collection methods are also changing, adding or reducing measurement points. Ultimately, this will form a table structure. However, when actually reading the data, it is found that the columns of a table often need to change.
▶︎ Feature 2: From Simple to Complex Loads
Due to the excessive number of measurement points on devices, in a relational database model, such a table will be forced to be vertically split. If a database does not set the number of columns in a table, it cannot allocate memory and will also impact the system.
Moreover, complex industrial devices consist of many independent sub-devices or components. From the database perspective, each measurement point is collected independently, with inconsistent collection frequencies and time. Although the table structure is most suitable for querying, providing the strongest expression capability for users, during writing and management, users do not need to see such a table. Based on this, a hierarchical structure to manage data is a natural idea for industrial enterprises. In this hierarchical structure, the collection frequency of measurement points on devices can vary.
In the application process, IoTDB has consistently used the tree structure shown above, which is more in line with asset management and scheduling methods.
IoTDB defines several concepts; in the context of industrial IoT, devices or apparatus that have direct physical quantities will generate data, and all physical quantities belong to what is called an entity. For example, a wind turbine, a vehicle, a bridge, etc., all belong to entities. The collection of multiple entities, with their data physically isolated on disk, is called a storage group.
Physical quantities (conditions, fields, measurement points, variables) can measure the measurement information recorded by devices. They can be univariate or multivariate. Univariate physical quantities can independently collect power, voltage, current, support displacement, wind speed, vehicle speed, longitude, latitude, etc. Multivariate physical quantities can collect GPS (longitude, latitude), etc. simultaneously.
Thus, we define time series (entity + physical quantity), including univariate series (entity + univariate physical quantity) and multivariate series (entity + multivariate physical quantity). For IoTDB, our storage becomes entity + physical quantity, such as the rotational speed of a wind turbine or the GPS of a vehicle.
What impact do different modeling methods have on the system? It is evident that each univariate series will have its own timeline. If multiple univariate series have the same timeline, it will lead to redundancy and waste of spatial resources; therefore, they should be stored as multivariate series.
In the future, IoTDB will attempt to identify whether a scenario is suitable for univariate or multivariate series. At this stage, users need to define it themselves.
In real applications, there will also be massive entities of the same type and model, each with the same set of physical quantities, such as a batch of identical vehicles or a batch of identical wind turbines. We call this a physical quantity template. Using physical quantity templates can significantly reduce the cost of metadata management.
Different types of sequences have different encoding and compression method choices to provide better compression rates. Among them, PLAIN is suitable for data with large variations, which is irregular and hard to predict. RLE is suitable for data with mostly the same values. TS_2DIFF is suitable for data with stable changes. GORILLA is suitable for floating-point numbers with small changes. DICTIONARY is suitable for TEXT types with small cardinality.
▶︎ Feature 3: From Time-Series Data Queries to Time-Series Data Processing
Storing data incurs costs for users, while analyzing the value within the data can create benefits for users. Therefore, it is essential for IoTDB to possess analytical capabilities, especially for analysis in the industrial domain. Can we assign analysis tasks and the processing of those tasks to IoTDB?
For databases, there are several opportunities for data analysis. The first opportunity is when data just arrives, which may undergo initial processing. When data arrives, IoTDB provides a computation model based on sliding windows and single points to achieve stateful and stateless computations. To limit computational complexity, IoTDB only allows analytical tasks to compute a single series or multiple series under one device.
The second opportunity occurs when data has been stored in the database but has not yet been queried. During this time, we can perform pre-analysis on the data, which can be represented by an index, aimed at allowing users to access data more quickly.
The third opportunity is during query computation, such as calculating the average value or absolute value of raw data. The concept of query-time computation in databases inevitably brings up user-defined functions (UDFs). For UDFs, there are two types of time-series data: one is UDTF (User Defined Time Series Function), which takes n series as input and outputs one series; the other is UDAF (User Defined Aggregate Function), which takes n series as input and outputs one point.
In recent years, we have been conducting research on the data quality of time-series data. By integrating UDFs with these algorithms into IoTDB, we have formed a set of IoTDB-Quality data quality algorithm library for time-series data, which is not strongly bound to IoTDB.
IoTDB supports user-defined down-sampling. If the down-sampling capabilities or functions provided by the system do not meet business needs, for example, using the average when data is dense and selecting maximum or minimum when data is sparse, then the DB’s down-sampling capability may not support such rich customizations, and custom functions can be used to implement this.
Additionally, one important industrial application of time-series data is real-time alerts. Once an alert occurs, a rapid response is required. However, in real applications, when observed values fall below specified thresholds, it can lead to a large number of alerts. To be usable in production, it is often necessary to filter out false alarms, which are anomalies caused by data fluctuations.
We use UDFs to address the issue of false alarms. For such alerts, we follow several steps: jump change clearance, threshold filtering, matching true alerts, anomaly degree calculation, anomaly alerting, and manual confirmation.
Conclusion
From the two versions of IoTDB 0.12 and 0.13, we have been contemplating: as the degree of data utilization increases, what new features should IoTDB present? Therefore, we have been developing UDF and trigger functionalities. Furthermore, in the development of the distributed version, IoTDB will continue to strengthen its capabilities regarding time partitioning and virtual storage groups. In the future, IoTDB will provide increasingly rich capabilities and better performance for everyone.
Recent Article Highlights
Five Ways Big Data Projects Can Go Wrong
When Pravega Meets TiDB, How to Build a Real-Time Data Warehouse?
How is the Global “Heartthrob” MySQL Faring in China?