Click the blue“Radar Journal”to follow

Keywords: High-Resolution Range Profile / Target Recognition / Feature Extraction / Radar Sensor Network / Contrastive Learning
Background Introduction
The High-Resolution Range Profile (HRRP) of radar reflects the distribution of the target’s scattering center along the radar line of sight, containing rich geometric structure and size information of the target. In the past, radar target recognition technology based on HRRP mainly used a single radar as the data acquisition source. However, recent studies have shown that a radar sensor network (Radar Sensor Network, RSN) composed of multiple radar sensors can capture the spatial diversity characteristics of the target’s radar cross-section through widely separated antennas, significantly improving recognition accuracy by utilizing the scattering variations of the target from different angles.
Current RSN target recognition methods still face some problems and challenges. Firstly, existing literature lacks in-depth research on the correlation and difference information mining of echo data obtained from different radar sensors; secondly, the fusion strategies of existing methods are relatively simple, mostly employing data stitching, feature stitching, and weighted decision methods, making it difficult to fully utilize multi-source information; finally, most existing studies require a large number of labeled samples to obtain prior knowledge of the target..
Team Work
Figure 1 Professor Liang Jing’s Research Group at the University of Electronic Science and Technology of China
This work has been published in the online-first paper of the “Radar Journal” titled “Radar Sensor Network HRRP Unsupervised Target Feature Extraction Method Based on Multiple Contrastive Loss” (Wan Hao, Liang Jing)..
Paper Introduction
This paper proposes a radar sensor network HRRP target recognition method based on multiple contrastive loss, first utilizing ResNet as a feature extraction network to obtain target feature information from preprocessed multi-sensor HRRP data. Secondly, two simple neural network projection heads are stacked on the feature network to map the features to the contrastive loss space and semantic loss space, respectively. In the contrastive loss space, instance-level loss and Fisher loss are introduced to enhance the network’s ability to mine discriminative features, reducing the relative and absolute distances between different echoes of the same target obtained by different sensors while increasing the distance between different target echo samples. In the semantic loss space, semantic labels are constrained by discriminative features to achieve consistency between semantic information and discriminative features. Finally, the extracted features are used to complete downstream target recognition tasks.
Then, target recognition experiments were conducted on the measured civil aircraft HRRP dataset, comparing four classic unsupervised feature extraction methods and three supervised learning methods for HRRP target recognition. The experimental results demonstrate the superiority of the proposed method.
Next, t-SNE was used to perform two-dimensional feature visualization analysis on the HRRP dataset before and after processing by the proposed method, further validating the feature extraction capability of MCL.
Finally, the paper concludes with a summary and an outlook on future research directions.
Author Introduction
Liang Jing, PhD, Professor at the University of Electronic Science and Technology, PhD supervisor. Research directions include radar sensor networks, distributed collaborative signal processing, fuzzy logic, and machine learning.
Related Readings
[Results] A Class Activation Mapping Method for SAR Target Recognition Deep Networks (Video)
[Results] A Deep Network for SAR Target Recognition Based on Attribute Scattering Center Convolution Kernel Modulation (Video)
[Results] A Data Separability Metric Method in Radar Target Recognition Evaluation (Video)
[Results] A Feature Optimization Method for Radar Road Target Recognition Based on Histogram Analysis and Adaptive Genetics (Video)
[Review] Research Progress and Outlook on Intelligent Detection and Recognition Technology for Aircraft Targets in SAR Images (Video)
Academic Report | Zero-shot SAR Image Target Recognition (Video)
Future Stars | Open Set Recognition and Continual Learning and Their Applications in Radar Target Recognition (Video)
PhD Forum | Research on Small Sample Incremental Recognition Technology for SAR Image Targets (Video)
PhD Forum | Initial Exploration of Joint Physical Models and Data-driven SAR Target Detection and Recognition (Video)
Fourth Radar Journal PhD Forum Excellent Academic Report Release
Good News! “Radar Journal” Selected for the Excellent Action Plan of Chinese Science and Technology Journals
Editor: Wu Guanlin
The information published by this account aims to promote communication, and the content is the responsibility of the author, not representing the views of this account. If there are any issues involving text, images, copyrights, etc., please contact this account within 20 days, and we will handle it promptly. “Radar Journal” has the final interpretation right.
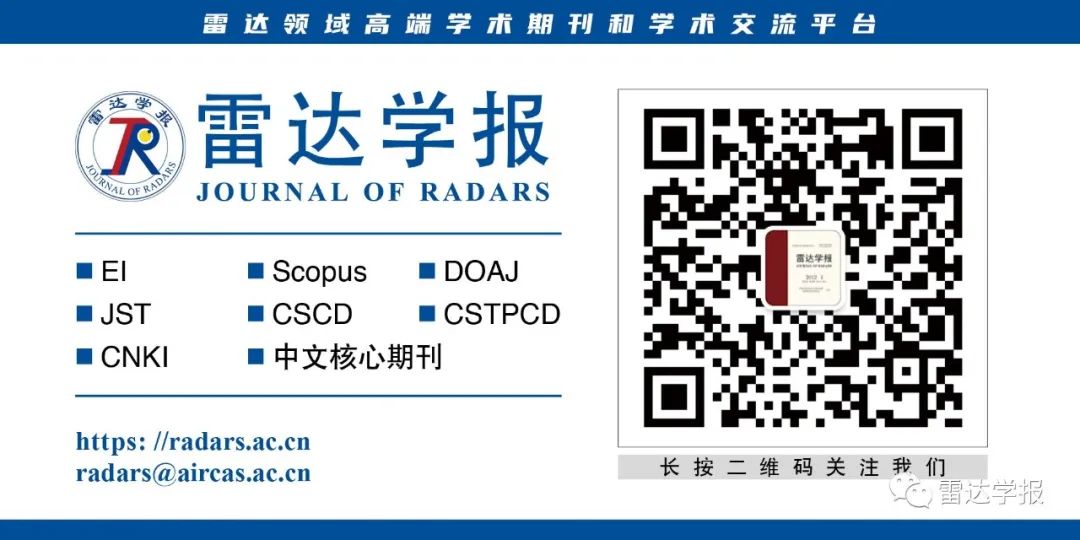