Every week a book
Let reading enrich life
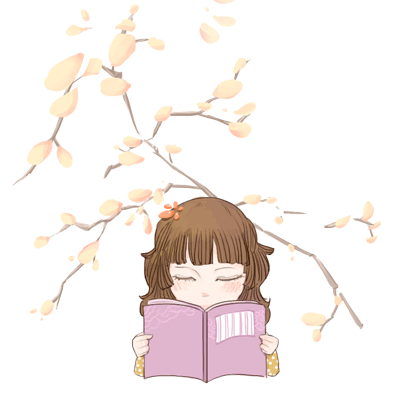

21 Jun: For over a century, scientists have adhered to the statistical adage that “correlation does not imply causation,” leading to a reluctance to discuss causal relationships.
Today, this taboo has finally been broken.
Artificial intelligence research expert Judea Pearl and his colleagues have led a revolution in causal relationships that establishes the central role of causal research in scientific exploration.
Source丨This content is a synthesis from the book “Why: The Science of Causality”
Editor丨Axiang; Intern Sichen
Image source丨Network, Tuchong Creative
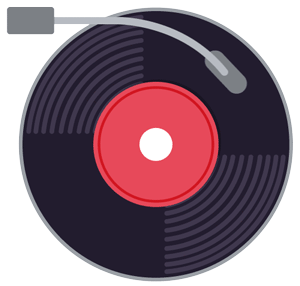
Deep learning is precisely the manifestation of artificial “non” intelligence, as its research subject is correlation rather than causation, sitting at the very bottom of the causal relationship ladder.
Prologue
1
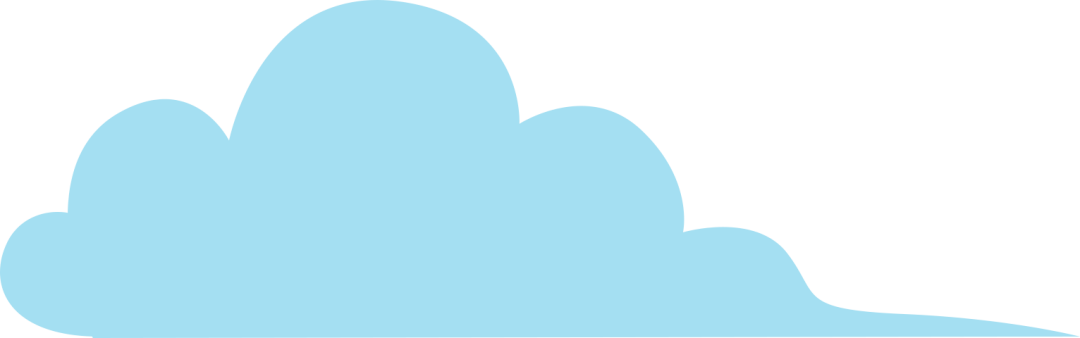
Whenever someone mentions how powerful “driverless” car technology is and what kind of expectations the public has placed on it, I think of a scene from the HBO series Silicon Valley:
The assistant of Silicon Valley mogul venture capitalist Gregory arranged for a driverless car to take the startup’s junior employee Jared home, thinking this plot was merely to illustrate a type of Silicon Valley arrogance.
At first, everything went smoothly, but halfway through the journey, the car suddenly began executing previously set commands, ignoring Jared’s cries of “Stop” and “Help,” and headed towards another destination: an uninhabited island four thousand miles away.
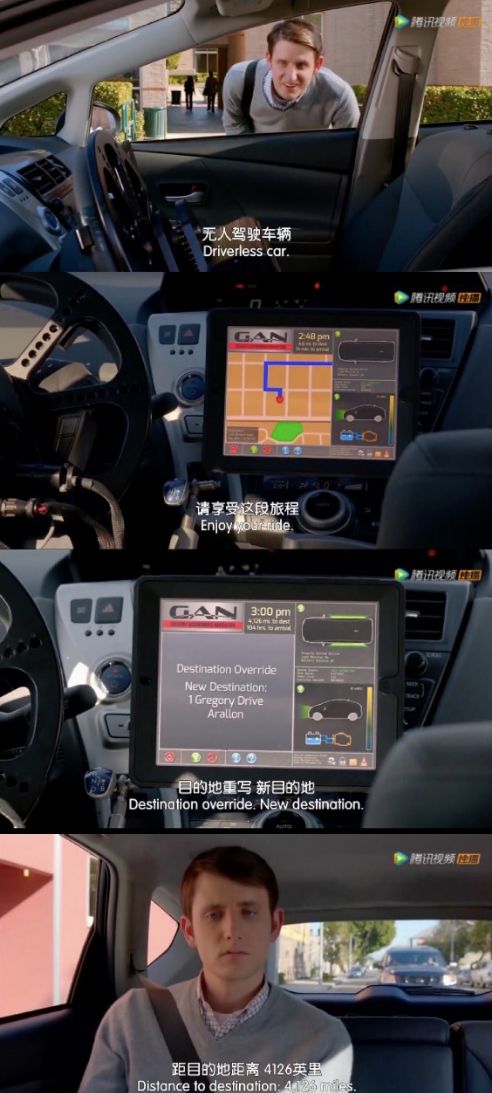
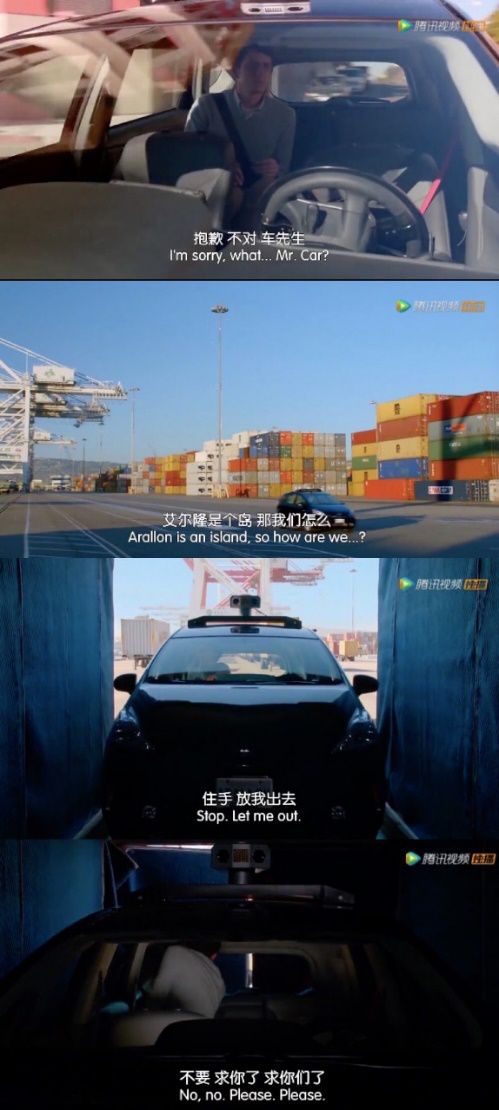
Screenshot from the TV series Silicon Valley
Jared ultimately got saved, just when I thought the plot was about to turn into “Castaway.”
For most viewers, this is merely a dark comedic point in the show, but art is derived from reality, and in reality, if a driverless car suddenly loses control, the consequences are truly unimaginable.
On May 7, 2016, in Florida, a Tesla Model S owner died after using the Auto Pilot mode, leading to a fatal accident.
This was the first fatal accident involving the auto-pilot mode, forcing all enthusiasts of driverless technology to confront the safety concerns it brings.
Tesla once released a statement:
“Achieving a 99% accuracy rate for driverless cars is relatively easy, but reaching 99.9999% is much more difficult, and that is our ultimate goal, because if a car traveling at 70 miles per hour fails, the consequences are dire.”
Tesla did not say 100%.
In the future, even if these tech companies claim that driverless technology has developed to an incredibly mature state, there will still be people who find it hard to trust getting into a driverless car; from a psychological perspective, such cars will never feel “safe enough” compared to “self-driving.”
2
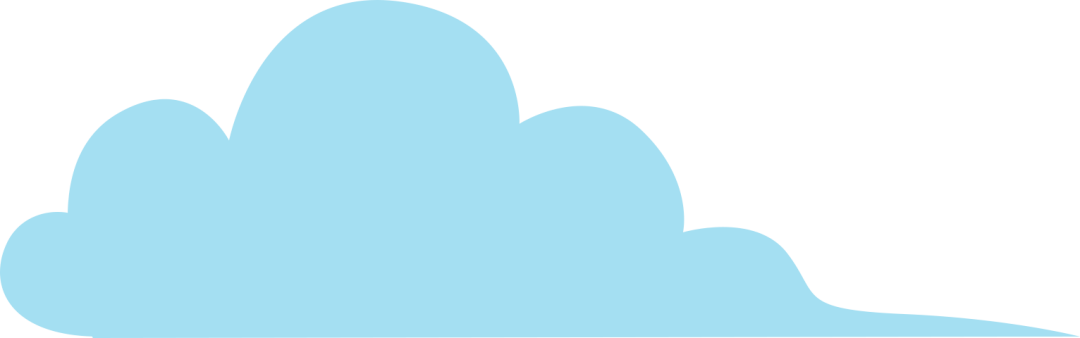
Significant advancements in driverless technology are inseparable from deep learning algorithms.
In the eyes of Judea Pearl, the father of Bayesian networks, deep learning is precisely the manifestation of artificial “non” intelligence, as its research subject is correlation rather than causation, sitting at the very bottom of the causal relationship ladder.
Pearl once mentioned in an interview with Quanta Magazine:
All the tremendous achievements of deep learning are, to some extent, merely curve fitting of data.
From a mathematical perspective, no matter how cleverly you manipulate the data, and what you read while manipulating the data, it is still a curve fitting training process, even if it appears complex.
The development of artificial intelligence has benefited from Pearl’s early research in many ways, yet he has overturned himself in his latest work “Why: The New Science of Causality.”
Pearl believes that current artificial intelligence and machine learning are actually at the lowest level of the causal relationship ladder, passively accepting observational results, considering questions like “what would happen if I see…”.
While strong AI requires achieving the third level of “counterfactual” reasoning.
For example, if a driverless car’s programmer wants the car to respond differently in new situations, they must explicitly add descriptions of these new responses in the program code.
The machine will not understand that a pedestrian holding a bottle of whiskey might react differently to a honk, and any operating system at the lowest level of the causal relationship ladder inevitably lacks this flexibility and adaptability.
AI that cannot perform causal inference is merely “artificial stupidity,” and will never be able to perceive the causal essence of the world through data.
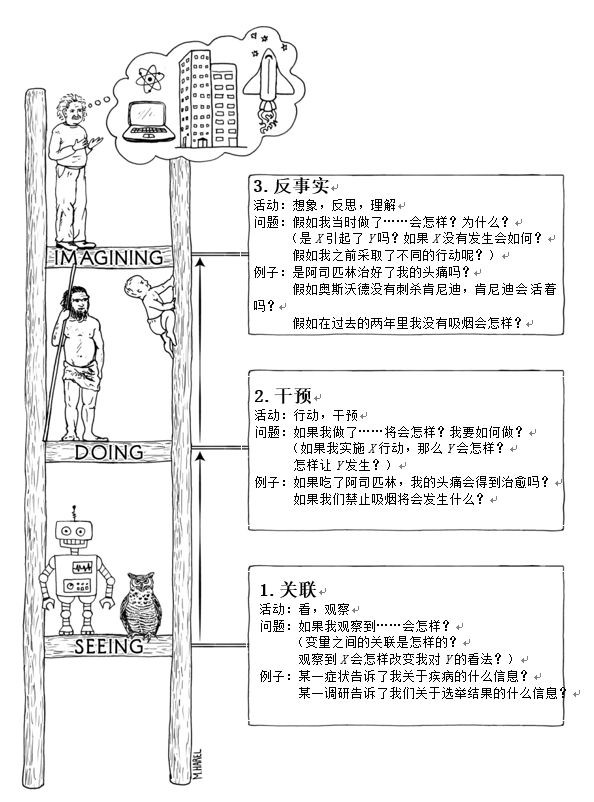
Each level of the causal relationship ladder has a representative organism
(Source: “Why: The Science of Causality”
Illustration by Maya Harel)
3
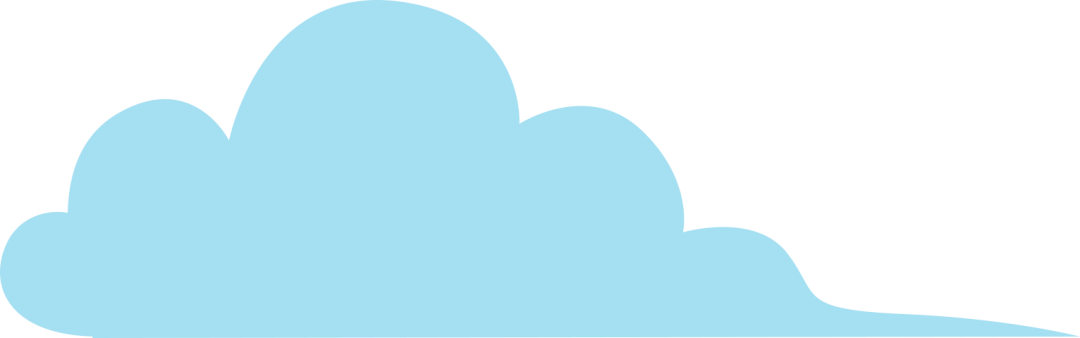
In March 2016, AlphaGo defeated the long-considered strongest human Go master Lee Sedol 4-1, shocking the world, igniting countless imaginations about the development of artificial intelligence, and bringing a sense of crisis to many.
Unfortunately, this AI feat can only prove that:for certain tasks, deep learning is useful.
People realized that in simulatable environments and states, AlphaGo’s algorithm is suitable for intelligent searches in large probabilistic spaces, while for decision-making problems in difficult-to-simulate environments (including the aforementioned driverless cars), such algorithms are still helpless.
Deep learning methods are similar to convolutional neural networks and do not handle uncertainty in a rigorous or clear manner, and the network architecture can evolve independently.
After completing new training, the programmer does not know what calculations it is performing or why they are effective.
The AlphaGo team did not predict at the beginning that this program would defeat the best human players within a year or five years, nor could they explain why the program’s execution produced such good results.
If robots are like AlphaGo, lacking clarity, then humans cannot communicate meaningfully with them to make them “intelligent.”
Assume you have a robot in your home, and while you sleep, the robot turns on the vacuum cleaner and starts working, at which point you tell it, “You shouldn’t wake me up.”
Your intention is to let it understand that turning on the vacuum cleaner at this time is wrong, but you certainly do not want it to interpret your complaint as meaning it cannot use the vacuum cleaner upstairs anymore.
At this point, the robot must understand the causal relationships behind it: the vacuum cleaner makes noise, noise wakes people up, and this makes you unhappy.
This simple command for us humans actually contains a wealth of information.
The robot needs to understand: it can vacuum when you are not sleeping, it can vacuum when no one is home, or it can vacuum when the vacuum cleaner is silent.
In this light, does it seem that the amount of information contained in our daily communication is indeed overwhelming?
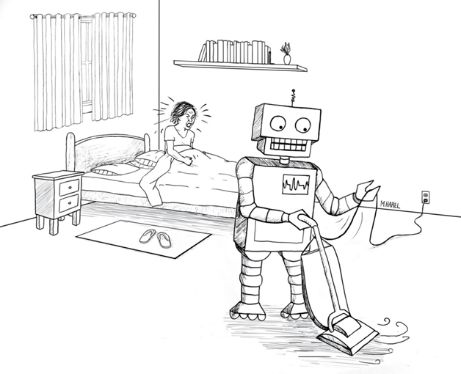
A smart robot considers the causal impacts of its actions.
(Source: “Why: The Science of Causality”
Illustration by Maya Harel)
Therefore, the key to making robots truly “intelligent” lies in understanding the phrase “I should take a different action”, whether this phrase is told to it by a human or derived from its own analysis.
If a robot knows its current motivation is to do X=x0, and it can evaluate whether a different choice, doing X=x1, would yield better results, then it is strong artificial intelligence.
4
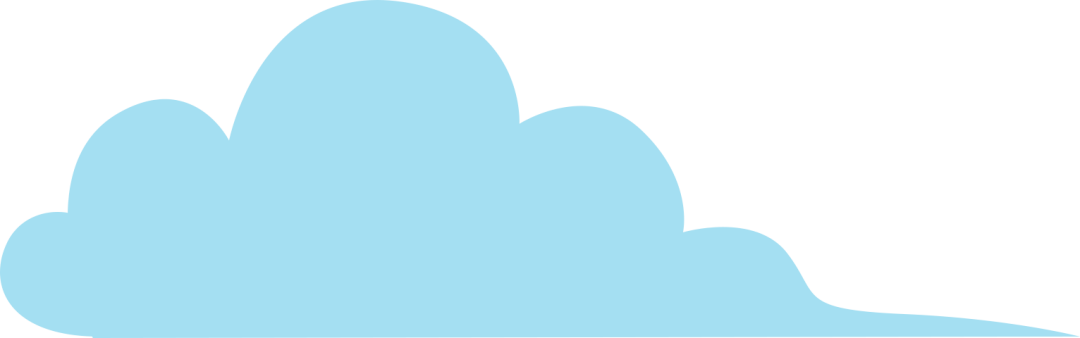
Yuval Noah Harari, the author of “Sapiens,” believes that the human ability to depict fictional things is a cognitive revolution in human evolution, and counterfactual reasoning is a uniquely human ability and true intelligence.
Every advancement and development of humanity is inseparable from counterfactual reasoning; imagination helps humanity survive, adapt, and ultimately dominate the world.
Causal theory provides a set of tools for counterfactual reasoning,which, if applied in the field of artificial intelligence, holds the promise of achieving true strong AI.
Regarding the question of whether robots with free will can be developed, Pearl’s answer is an absolute yes.
He believes: people must understand how to program robots and what can be derived from it.
For some reason, in terms of evolution, this free will will also be necessary at the computational level.
The first sign of robots having free will will be counterfactual communication, such as “You should do better.”
If a group of football-playing robots starts communicating in this language, then we will know they possess free will.
“You should pass the ball to me; I was waiting, but you didn’t pass it to me!”
“You should…” means what you should have done but didn’t.
Thus, the first sign is communication; the second is playing better football.
Previously, discussions on strong artificial intelligence were mostly limited to philosophical levels, and academia has always maintained a cautious attitude towards “strong AI.” There hasn’t been too much hope.
However, scientific progress never stops due to failure; whether in driverless cars or the development of other AI technologies, it ultimately relies on “humans.” Will humanity research robots capable of understanding causal dialogue? Will we be able to create artificial intelligence as imaginative as a three-year-old child?
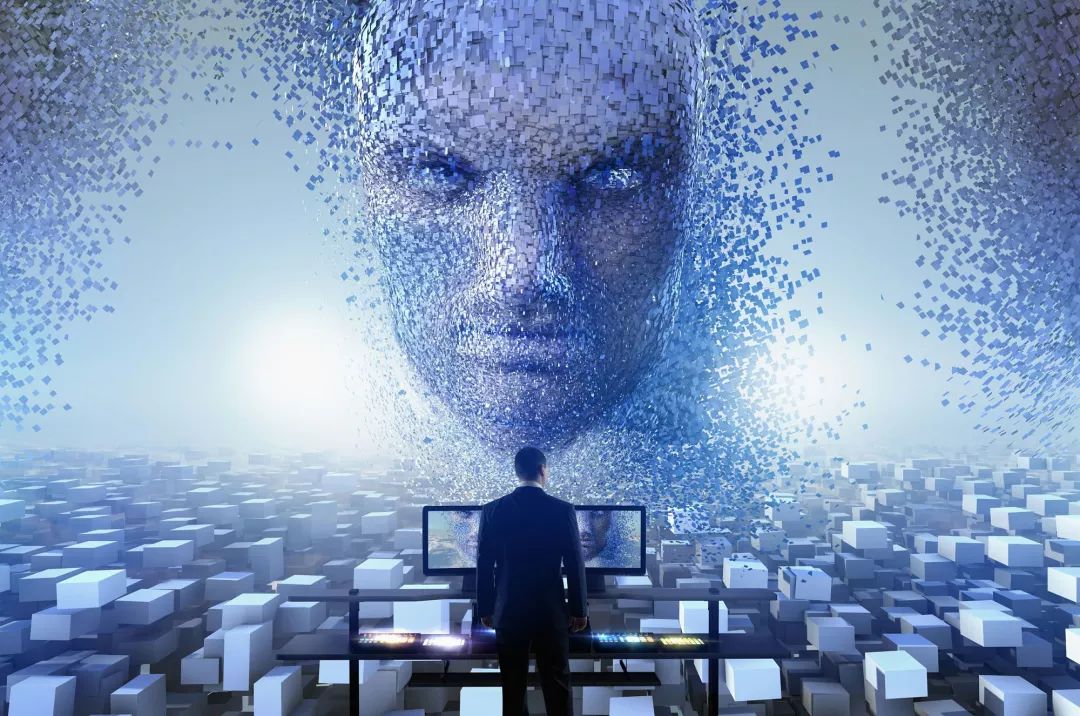
Image source / Tuchong
The key to answering these questions is, if humanity itself cannot understand the ladder of causation, how can we make the “artificial” become “intelligent”?
Machines need not replicate humans but can outperform them, which is a terrifying fact.
If we can replace associative reasoning with causal relationships and enter the world of counterfactual reasoning along the ladder of causation, then the rise of machines will be unstoppable.
Pearl provides a clear and accessible explanation of how to achieve this goal in his book.
Looking back, actually my daily life has not produced much close association with the term “artificial intelligence,” but I once felt an inexplicable and strong sense of awe when I learned that AlphaGo defeated Lee Sedol.
The pace of technological advancement seems to always exceed our imagination; searching for the keyword “major breakthroughs” on your phone will instantly drown you in a sea of technological fast food. What will machines become? How will they treat humanity?
Only by trying to understand causal relationships can we face these questions with less confusion and more faith.

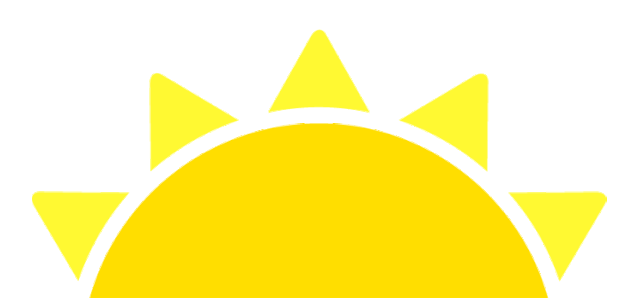
Book Giveaway
Artificial intelligence research expert Judea Pearl believes that the profound significance of causal research may be reflected in the field of artificial intelligence.
Today’s machine learning relies on statistical and probabilistic correlations, while causal reasoning can transform computers into true scientists, granting them human-level intelligence and even moral consciousness.
Perhaps this is the most important thing we can do for the machines that are about to take over our lives.
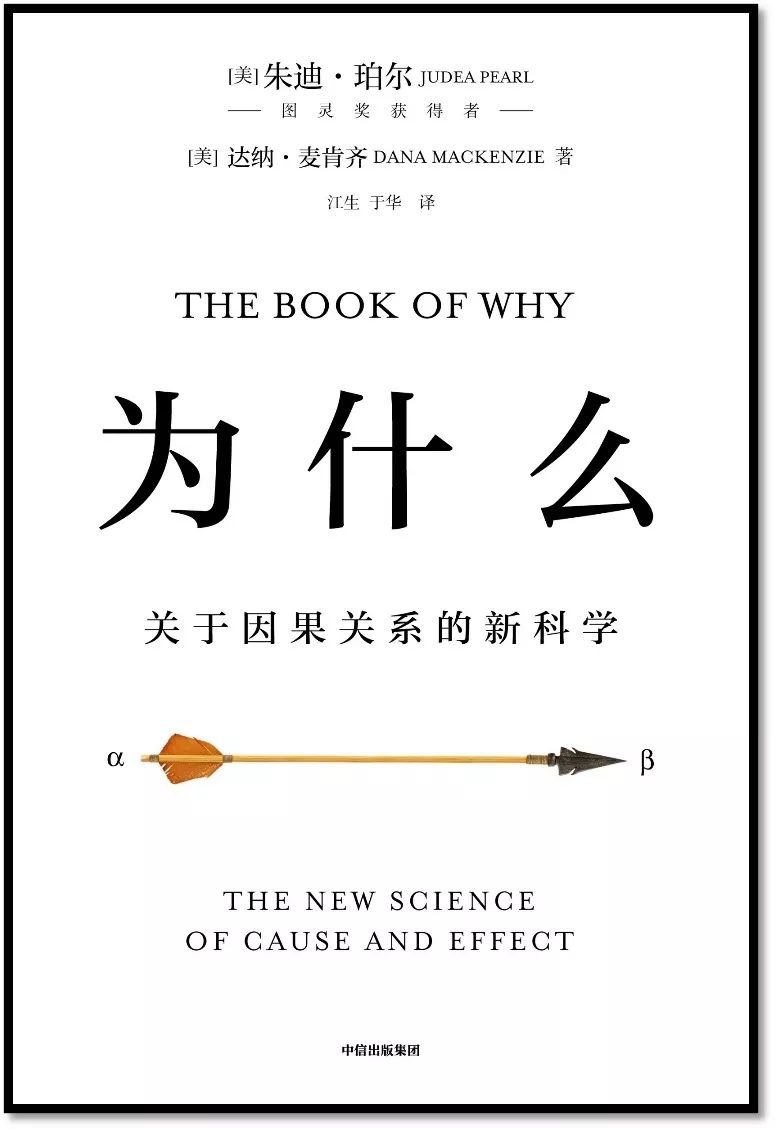
“Why: The Science of Causality”
Authors:[USA] Judea Pearl
[USA] Dana Mackenzie
Translator: Jiang Sheng, Yu Hua
Publisher: CITIC Publishing Group
Now, 21 Jun is giving away free books!
How to get it?
Leave a comment below this weekend’s reading, and the reader with the highest likes (at least 50 likes) will receive a free book. Additionally, 21 Jun will also select some readers with heartfelt comments to receive a free copy of “Why: The Science of Causality.”
To provide more opportunities for readers, during the four consecutive giveaways, the same reader can only win once (the same WeChat ID, phone number, and address are considered the same reader).
We will announce the winners tomorrow night during the night reading. Those who receive the free books should remember to leave your address on time; overdue will not be accepted (so the deadline for the first like is between 20:00 and 21:00 on August 4; note: the accurate time is based on 21 Jun’s screenshot; if unexpected news occurs, it may also be captured earlier).
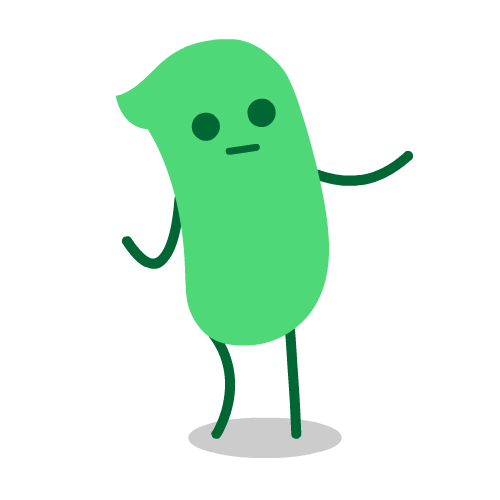
21 Jun

Friends, what artificial intelligence technologies do you think have already impacted our lives? What do you think about the author’s exploration of the causal relationships behind strong AI?
Let’s chat about your views!
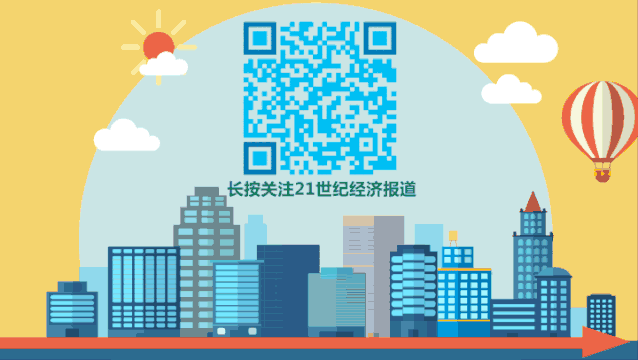