This article designs a new portable hyperspectral imaging device, LeafSpec-Dicot, for hyperspectral imaging of dicot leaves (such as soybean). The device can acquire a complete hyperspectral image of a dicot leaf in 20 seconds, with high spatial and spectral resolution. The data quality of the device is validated by detecting the nitrogen content of soybean plants, using two methods to establish nitrogen content prediction models based on hyperspectral images: one is a linear regression model based on the average normalized difference vegetation index (NDVI), and the other is a deep learning neural network model based on the NDVI heatmap distribution of the entire leaf. The results show that the latter can better utilize the spatial distribution information of NDVI on the leaf, improving the accuracy and correlation of the predictions. This novel hyperspectral imaging device can provide high-quality data for phenotypic analysis of dicot plants.
The main structure of LeafSpec-Dicot is as follows:
The scanning mechanism (composed of a motor-driven gear frame, two glass plates, an encoder, and two limit switches) clamps the leaf between the two glass plates, protecting the leaf from sliding damage while moving the hyperspectral camera along the gear frame for line-by-line scanning of the leaf. The encoder is used to measure the movement distance of the hyperspectral camera, and the limit switches are used to restrict the scanning range. The hyperspectral camera is a line-scanning camera with a spectral range of 400-1000 nm, a spectral resolution of 2.8 nm, and a spatial resolution of 0.1 mm. The light source consists of two 12 W halogen lamps that emit a smooth, broad-spectrum light covering both visible and infrared light. The halogen lamps are installed in a light box that illuminates only a narrow long area of the leaf, corresponding to the hyperspectral camera, with the light box outlet covered by a plastic sheet to evenly diffuse the halogen lamp light. Additionally, it includes peripheral circuits and a microprocessor (Raspberry Pi 4), allowing the device to communicate with smartphones via Bluetooth to transmit image data.
Finally, 64 soybean plants (divided into two genotypes Pioneer P34T21SE and Thorne), each genotype divided into four treatment groups (high nitrogen low water, high nitrogen high water, low nitrogen low water, low nitrogen high water), with 8 plants per group. Hyperspectral imaging was performed on the three mature compound leaves at the top of each soybean plant, followed by whiteboard correction of the original hyperspectral images, leaf segmentation, and calculation of the NDVI heatmap for each image. Two methods were used to establish nitrogen content prediction models based on hyperspectral images, and the results showed that the deep learning neural network model based on the NDVI heatmap distribution of the entire leaf could better utilize the spatial distribution information of NDVI on the leaf, improving the accuracy and correlation of the predictions. The R2 value on the test set was 0.871, and the RMSE value was 0.276, outperforming the linear regression model based on average NDVI.
Figure 1 LeafSpec handheld HSC for dicot plants: (a) LeafSpec external structure; (b) LeafSpec internal structure
Figure 2 NDVI heatmap of the entire leaf captured by Leafspec-Dicot
Figure 3 Linear relationship between nitrogen content and average NDVI of leaves
Figure 4 Correlation between N prediction values modeled using average NDVI and measured data
Figure 5 Correlation between N prediction values using deep learning network and measured data
Li X, Chen Z, Wang J, et al. LeafSpec-Dicot: An Accurate and Portable Hyperspectral Imaging Device for Dicot Leaves[J]. Sensors, 2023, 23(7): 3687.
Editor
Extended Reading
-
Plant Phenotyping Information Directory Summary 2018 -
Plant Phenotyping Information Directory Summary 2019 -
Plant Phenotyping Information Directory Summary 2020 -
Plant Phenotyping Information Directory Summary 2021 -
Plant Phenotyping Information Directory Summary 2022 -
Plant Phenotyping Information Directory Summary 2023 -
Plant Phenotyping Information Classification Special Collection -
What Does the Best-Selling Field Phenotyping Platform Look Like?
The following video is sourced from the Plant Phenotyping Circle
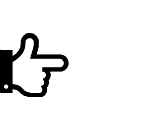
Click here to read the original text