“
Simulation is one of the most important tools in the process of robot learning and research, which refers to the technology of simulating actual physical systems through computers. Robot simulation is based on interactive computer graphics technology and robotics theory, generating geometric shapes of robots and displaying them in 3D, used to describe the dynamic changes of robots and their working environment.In this technical article, we invited Xiaomi engineer—— Xie Yan, focusing on the robot simulation platform as the main discussion topic, introducing the necessity of simulation to evaluation metrics, combined with specific physics engines and simulation software, providing a multi-layered introduction to robot simulation platforms.
”
1. Necessity of Simulation
Why is simulation necessary? This may be the first question for most beginners. The traditional product development process usually requires going through product definition -> concept design -> program review -> detailed design -> prototype trial production -> testing -> program optimization, etc. However, as the complexity of products increases, especially for robots, which are multi-degree-of-freedom complex systems, the disadvantages of directly adopting the above processes become increasingly significant.
First, when problems are found during testing, it is necessary to iterate and optimize the program; especially for hardware design, processing, and assembly, the process is time-consuming and labor-intensive. Once problems arise during this process, the iteration cycle can easily be prolonged.
1. Necessity of Simulation
Figure 1 Traditional Development Process

2. Introduction to Simulation
2. Introduction to Simulation
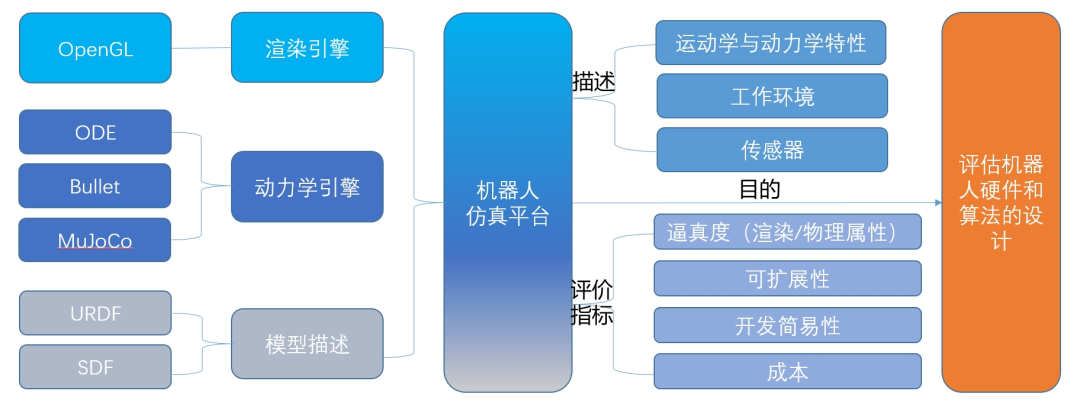
▍Model Description
Currently, the industry mainly uses URDF (Unified Robot Description Format) and SDF (Simulation Description Format) files to describe robot models, both of which are XML syntax frameworks used to describe robots. Although URDF is the standard format in ROS, it lacks many functions to describe more complex scenarios. For example, URDF can only specify the kinematic and dynamic characteristics of a single robot; it cannot specify friction characteristics and handle closed-chain structures. Additionally, it cannot specify environmental information such as lighting, height maps, etc. The introduction of SDF aims to solve the shortcomings of URDF, allowing for a complete description of all aspects of the robot, with greater scalability suitable for complex simulations.
▍Dynamics Engine
It can calculate motion, rotation, and collision based on the physical properties of objects. The basis of the dynamics engine is system dynamics, which is a mathematical description of the laws of motion of objects in the real world, primarily based on ordinary differential equations, and the core task of the physics engine is to solve these ordinary differential equations.
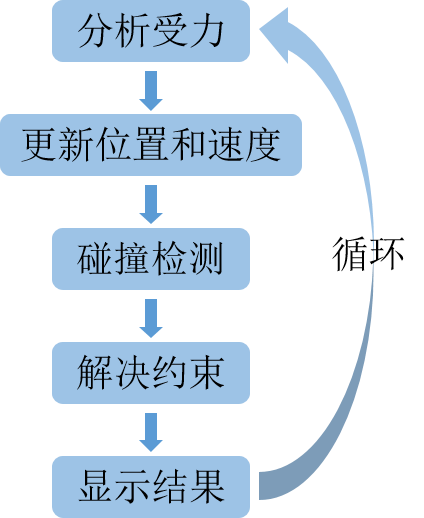
Figure 4 Physics Engine Workflow
▍Rendering Engine
3. Simulation Evaluation Metrics
3. Simulation Evaluation Metrics
Table 1 Simulation Evaluation Metrics
|
|
|
|
|
|
|
|
|
|
|
|
|
|
|
|
|
|
4. Introduction to Physics Engines
>>>> Bullet Physics (Bullet)
Introduction: Bullet[2] physics engine is an open-source, professional dynamics library that integrates rigid body, soft body, and collision detection. Developed by Erwin Coumans, it was originally designed for game development but is now widely used in robotics, virtual reality, computer graphics, and other fields. Bullet3 supports various physical simulations, including rigid bodies, soft bodies, vehicles, water, gas, etc.
Features: Open-source powerhouse, with discrete and continuous collision detection for rigid and soft body simulations; collision shapes include: spheres, boxes, cylinders, cones, convex hulls using GJK, non-convex meshes, and triangle meshes; supports soft body simulations: cloth, ropes, and deformable objects; provides rich rigid body and soft body constraints; features velocity-level MLCP and simplified coordinate Featherstone ABA algorithms; rich plugins: supports plugins for Maya, Softimage, can be integrated into Houdini, Cinema 4D, LightWave 3D, Blender, Godot, and Poser; Collada 1.4 physics content import; directed optimization for PlayStation 3 Cell SPU, CUDA, and OpenCL.
>>>> Open Dynamics Engine (ODE)
Introduction: ODE[3] (Open Dynamics Engine, an open-source dynamics engine) is a well-known free library for simulating joint-connected rigid body dynamics, developed by Russell Smith with the help of some contributors. Verified application scenarios include simulating ground vehicles, legged robots, and mobile objects in virtual display environments. It is characterized by being fast, flexible, robust, and has built-in collision detection capabilities.
Features: Supports rigid bodies with arbitrary mass distribution; joint types: spherical, revolute, prismatic, fixed, rotary motors, linear motors, universal joints; collision primitives: spheres, boxes, cylinders, capsules, planes, and triangle meshes, convex bodies; collision spaces: quadtrees, hash spaces, and simple spaces; simulation methods: derived motion equations based on the Lagrange multiplier velocity model of Trinkle/Stuwart and Anitescu/Potra; uses first-order integrators, fast but not accurate enough, with higher-order integrators to be released later; time step method selection: can use standard “big matrix” methods or newer iterative QuickStep methods; contact and friction models: based on Baraff’s Dantzig LCP solver, although ODE implements a faster approximation for the Coulomb friction model; open native C interface (though ODE is mostly written in C++), and C++ interface.
>>>> Multi-Joint Dynamics with Contact (MuJoCo)
Introduction: MuJoCo[4] is a physics engine developed by Emo Todorov for Roboti LLC, aimed at promoting research and development in robotics, biomechanics, graphics, animation, and other fields that require fast and accurate simulation. It provides a unique combination of speed, accuracy, and modeling capabilities, but it is not just a better simulator. Instead, it is the first full-featured simulator designed from scratch, aimed at model-based optimization, especially optimization conducted through contact. MuJoCo makes it possible to scale computationally intensive techniques (such as optimal control, physically consistent state estimation, system identification, and automated mechanism design) and apply them to complex dynamic systems with rich contact behavior. It also has more traditional applications, such as testing and validating control schemes before deployment on physical robots, interactive scientific visualization, virtual environments, animation, and games.
Features: Simulations in generalized coordinates to avoid joint conflicts; can define inverse dynamics well even with contact; unified continuous-time representation of constraints through convex optimization; constraints include soft contacts, limits, dry friction, equality constraints; can simulate particle systems, cloth, ropes, and soft bodies; includes actuators for motors, cylinders, muscles, tendons, and slider-crank; optional Newton, conjugate gradient, or projected Gauss-Seidel solvers; optional pyramid or elliptical friction cones, dense or sparse Jacobian equations; optional Euler or Runge-Kutta numerical integrators; multi-threaded sampling and finite difference approximation; intuitive XML model format (called MJCF) and built-in model compiler; cross-platform GUI with interactive 3D visualization in OpenGL; runtime modules written in ANSI C and manually optimized for performance.
>>>> Dynamic Animation and Robotics Toolkit (DART)
Introduction: DART[5] (Dynamic Animation and Robotics Toolkit) is a collaborative, cross-platform open-source library created by the graphics lab and humanoid robot lab at Georgia Tech. This library provides data structures and algorithms for kinematics and dynamics applications in robotics and computer animation. Because DART uses generalized coordinates to represent articulated rigid body systems and Featherstone’s articulated body algorithm to calculate motion dynamics, its accuracy and stability have been significantly improved. DART has a wide range of applications in robotics and computer animation, as it includes a multi-body dynamics simulator and various kinematic tools for control and motion planning.
Features: Supports multiple platforms: Ubuntu, Archlinux, FreeBSD, macOS, and Windows; provides an extensible API to solve various optimization problems, such as nonlinear programming and multi-objective optimization.
Supports various collision detectors: FCL, Bullet, and ODE; supports various collision shapes, including primitive shapes, concave meshes, and probabilistic voxel grids; through customizable inertia and material properties, supports numerous primitive and arbitrary body shapes; provides comprehensive access to motion states of arbitrary entities and coordinate systems (e.g., transformation, position, velocity, or acceleration).
Plug-and-play hierarchical whole-body inverse kinematics solvers; uses Lie group representations and Featherstone hybrid algorithms for articulated dynamic systems to achieve high performance; provides comprehensive APIs for dynamic quantities and their derivatives, such as mass matrix, Coriolis forces, gravity, other external and internal forces; uses implicit LCP to handle contact and collision, ensuring no penetration, directional friction, and approximate Coulomb friction cone conditions; provides multiple constraint solvers: Lemke method, Dantzig method, and PSG method; supports “island” techniques to subdivide constraint handling for improved performance.
RaiSim |
Bullet |
ODE |
MuJoCo |
DART |
|
Initial release |
Unreleased |
2006 |
2001 |
2015 |
2012 |
Author |
J. Hwangbo D. Kang |
E. Coumans |
R. Smith |
E. Todorov |
J. Lee et al |
License |
Proprietary |
Zlib (open-source) |
GPL / BSD (open-source) |
Apache (open-source) |
BSD |
Main purpose |
Robotics |
Game, Graphics |
Game, Graphics |
Robotics |
Robotics |
Language |
C++ |
C / C++ |
C++ |
C |
C++ |
API |
C++ |
C++ / Python |
C |
C |
C++ |
Contacts |
Hard |
Hard/Soft |
Hard/Soft |
Soft |
Hard |
Solver |
Bisection |
MLCP |
LCP |
Newton / PGS / CG |
LCP |
Integrator |
Semi-implicit Euler |
Semi-implicit Euler |
Semi-implicit Euler |
Semi-implicit Euler / RK4 |
Semi-implicit Euler |
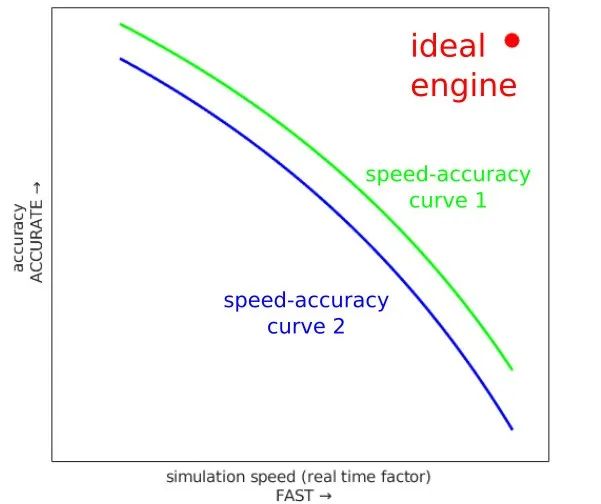
The table below describes the performance results of each physics engine based on the speed-accuracy curve evaluation[7].
RaiSim |
Bullet |
ODE |
MuJoCo |
DART |
|
friction model test |
++ |
+++ |
– |
+ |
– |
single-body elastic collision test |
++++ |
++ |
+++ |
– |
+ |
single-body hard contact test |
+++ |
+ |
++ |
+ |
+ |
single-body energy test |
++++ |
++ |
+++ |
– |
+ |
articulated-robot-system speed test for quadrupedal robot |
+++++ |
+++ |
+ |
++++ |
++ |
articulated-robot-system momentum test |
++++ |
+++ |
++ |
+++++ (RK4) |
+ |
+++ (Euler) |
-
+: Good simulation result, more + indicates better performance
-
-: Unable to simulate due to inaccurate or anomalous models
From Table 3, it can be seen that ODE generally handles objects of single primitive shapes well, while MuJoCo and DART have advantages in multi-body simulations. The multi-body system simulation of ODE is very time-consuming, but it is worth noting that it performs excellently in the articulated-robot-system momentum test: despite its obvious inefficiency, it has good momentum retention capability using maximum coordinate representation. Bullet can accurately and efficiently simulate the sliding of objects with frictional contact. RaiSim, with its unique contact solving methods and efficient multi-body dynamics algorithms, typically performs well in both single-body and multi-body tasks.
However, these engines also have their limitations:
-
ODE and DART’s LCP contact models cannot simulate the sliding of objects under certain conditions; -
DART is not suitable for multi-object simulation scenarios; -
Due to the lack of a solver error correction mechanism, Bullet suffers from significant position-level drift; -
MuJoCo’s soft contact model cannot control contact elasticity.
Robot system design cannot be separated from the support of simulation tools. Robot simulation allows us to quickly validate algorithms or improve safety without physical hardware, avoiding experiments that could damage our equipment (for example, in reinforcement learning, a lot of random exploration is required). Generally speaking, robot simulation tools are built on top of physics engines, such as those based on ODE, Bullet, etc. In some cases, we only need to use the physics engine to meet our needs, but generally, we also want to observe the correctness of the robot’s operation through a visualization platform. Below, we will introduce and analyze some well-known simulation platforms in the field of robot simulation.
However, Webots also has many drawbacks. First, the modeling process in Webots is relatively cumbersome, and it lacks robust support for closed-chain structure robots, making it cumbersome to simulate parallel robots. Secondly, the data from the Webots simulation process cannot be automatically saved, and the visualization is not very well done, making it inconvenient to use. Finally, the Webots console does not support Chinese, the software interface’s Chinese translation is incomplete, and the help documentation does not have a Chinese version.
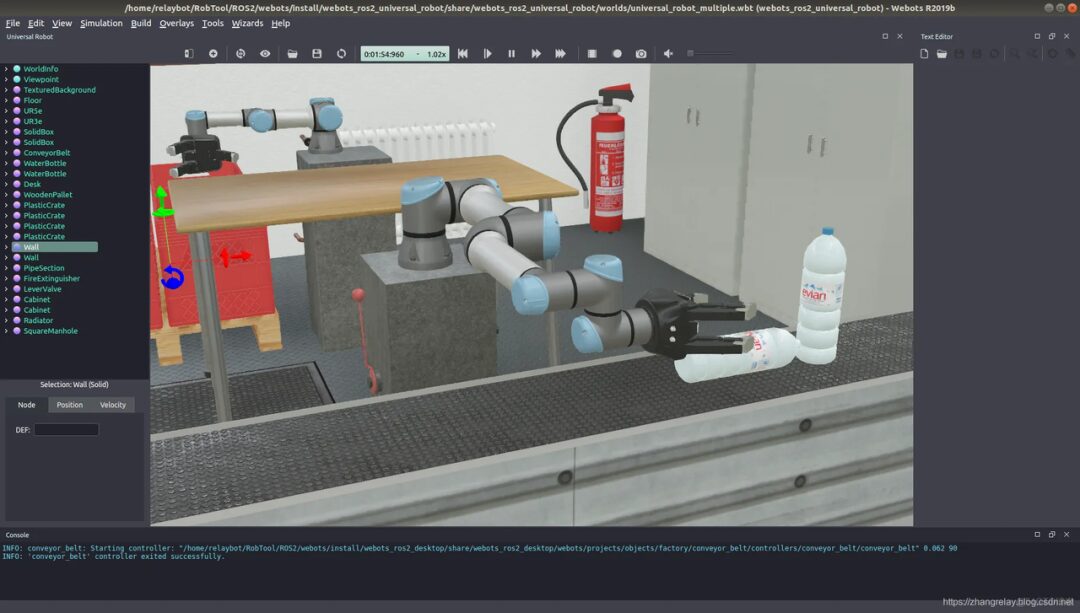
>>>> V-rep
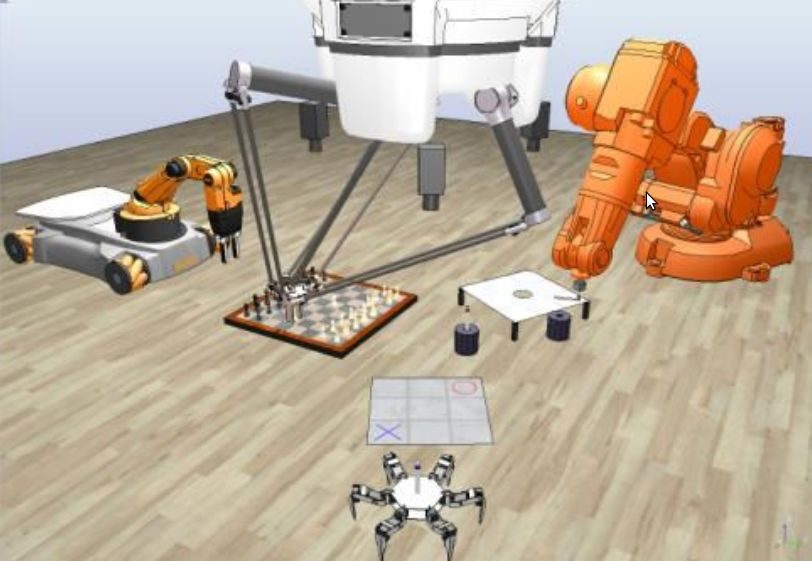
Gazebo[10] simulator is a free open-source platform for designing, developing, testing, and visualizing almost any type of robot. Gazebo runs on Linux, Windows, and Mac, and is the default simulator for ROS, allowing the invocation of various open-source algorithms and direct deployment to real machines. Gazebo supports various high-performance physics engines, such as ODE, Bullet, Simbody, and DART. The Gazebo simulator also comes with some robot models, such as PR2, DX, Irobot Create, and TurtleBot, so you can quickly get started even without your own robot models. It also supports a wide range of sensors and can simulate noise and sensor failures to accurately simulate real-world problems. Gazebo can even run in the cloud and interact with the simulator using a simple web browser.
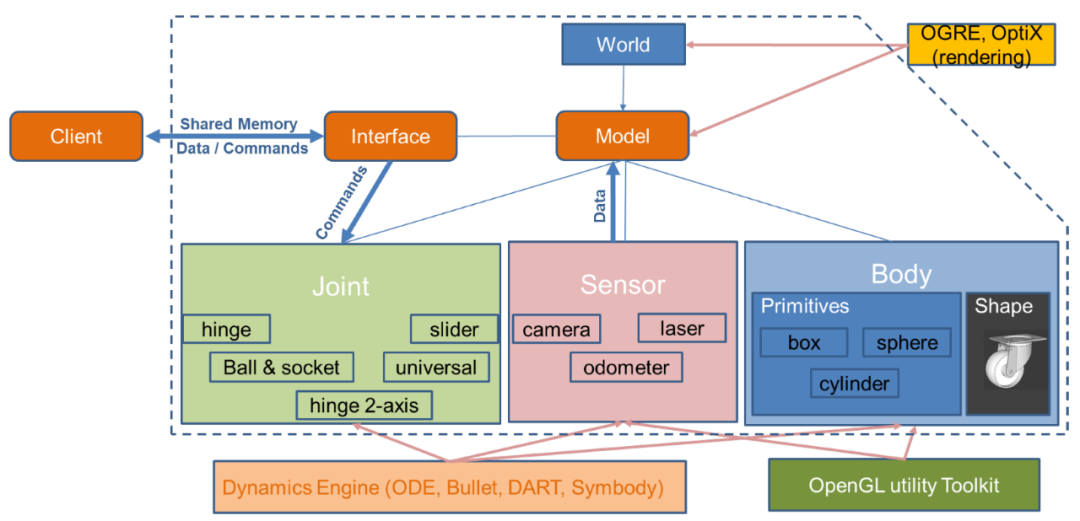
Figure 9 Gazebo System Architecture
Image Source:https://www.mdpi.com/2075-1702/7/2/42
Figure 10 Gazebo Simulator Interface
Image Source:https://alternativeto.net/software/gazebo-robot-simulator/about/
>>>> ISAAC
NVIDIA ISAAC[11] platform is a simulation platform developed by NVIDIA, a leading graphics card manufacturer, providing developers with a powerful end-to-end platform for developing, simulating, and deploying AI-supported robots. It can run on GPU-enabled desktops or in the cloud on AWS, Google Cloud, or Azure. It also supports NVIDIA Jetson AGX Xavier, Jetson TX2, and Jetson Nano. Since the ISAAC robotics platform is manufactured by NVIDIA, it offers the best graphics among all robot simulation platforms.
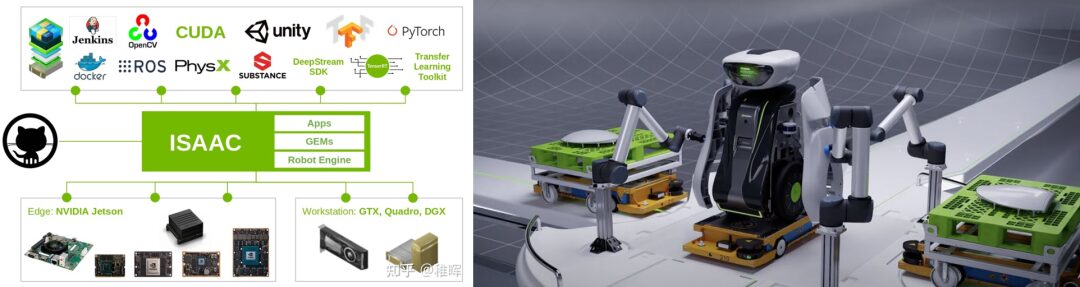
>>>> Drake
Drake[12] is a C++ toolbox developed by the Massachusetts Institute of Technology’s Computer Science and Artificial Intelligence Laboratory (CSAIL) Robot Locomotion Group. With the development of the core development team led by the Toyota Research Institute, the team has significantly expanded. It is a collection of tools for analyzing robot dynamics and building robot control systems, emphasizing optimization-based design/analysis.[13]
Drake is designed to simulate very complex robot dynamics (e.g., friction, contact, aerodynamics, etc.), while always emphasizing revealing structural characteristics (sparsity, analytical gradients, polynomial structure, uncertainty quantification, etc.) in control equations and using this information for advanced planning, control, and analysis algorithms.
Drake has three main components: 1) multi-body dynamics engine; 2) a “system framework” for organizing and combining system models from the library into block diagrams; 3) an optimization framework for mathematical programming. Each of these components is important for robotics research.
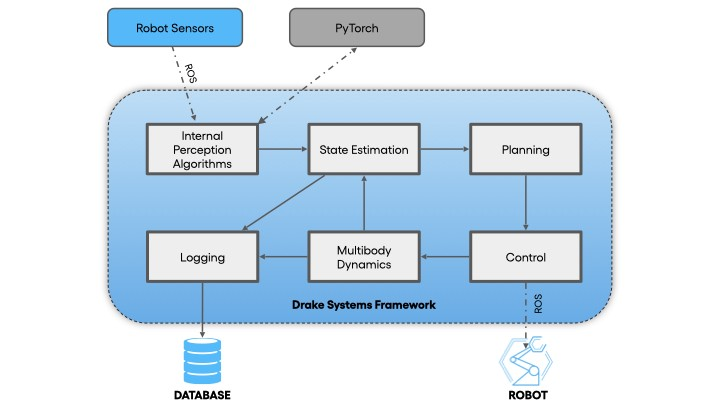
Image Source:https://medium.com/toyotaresearch/drake-model-based-design-in-the-age-of-robotics-and-machine-learning-59938c985515
Each of these components is crucial for robotics research, and Drake’s vision is to promote the powerful combination of these three components in a single application. Drake supports symbolic computation, which is very useful for optimization problems. Drake also provides a series of sensor models, actuator models, low-level controllers, and low-level perception algorithms. Drake provides a Python interface for rapid validation of new algorithms while also working to provide reliable open-source implementations for many state-of-the-art algorithms.
Image Source:https://www.youtube.com/watch?v=_8af5NgbKVc
>>>> MATLAB
MATLAB is a commercial mathematical software produced by MathWorks, USA. It is a versatile scientific computing platform that integrates many powerful functions such as algorithm development, data analysis, and matrix computation into an easy-to-operate window environment.
The Simulink[14] under MATLAB is considered capable of “simulating any system.” Due to the powerful capabilities of MATLAB/Simulink, it has been widely used in the field of robotics. The built-in Simscape toolbox can be used to design and analyze three-dimensional rigid mechanical structures (such as automotive platforms, robotic arms, etc.). In addition, it can model multi-domain systems using electrical, hydraulic, or pneumatic components and other components. The Robotics System Toolbox allows you to connect directly to sensors via ROS and perform calibration, denoising, and other operations on the returned information.
Figure 14 MATLAB/Simulink Simscape Simulator
>>>> PyBullet
PyBullet[15] is a simulation environment developed based on the Bullet physics engine and is a strong competitor to Gazebo. Developed by Erwin Coumans and other contributors, it allows users to control the Bullet3 physics engine using the Python programming language and perform physical simulations.
PyBullet is closely integrated with Python and is widely used in reinforcement learning (RL). This environment can combine with TensorFlow to implement RL training, such as DQN, PPO, TRPO, DDPG, etc.[16] Currently, it is commonly seen simulating multi-joint robots.
PyBullet is a fast and easy-to-use Python module for robot simulation and machine learning, focusing on Sim-to-Real conversion; using PyBullet, you can load articulated bodies from URDF, SDF, MJCF, and other file formats; PyBullet provides forward dynamics simulation, inverse dynamics computation, forward and inverse kinematics, collision detection, and ray intersection queries; the Bullet Physics SDK includes PyBullet robot examples, such as the simulated quadruped robot Minitaur, simulated humans running using TensorFlow for decision-making, and KUKA grasping objects; simplified coordinate multi-body, rigid body, and deformable bodies are handled by a unified LCP constraint solver.
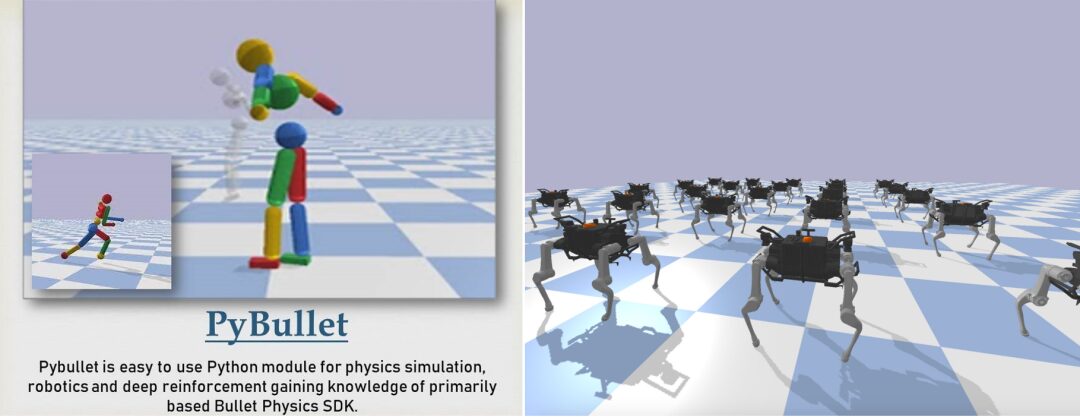
>>>> Mujoco
MuJoCo, full name Multi-Joint dynamics with Contact, is a physics engine for simulating multi-joint movements in contact with the surrounding environment, applicable in fields such as robotics actions, biomechanics, graphics and animation, and machine learning. Acquired and open-sourced by DeepMind in 2021, it can run on Linux, Windows, and Mac.
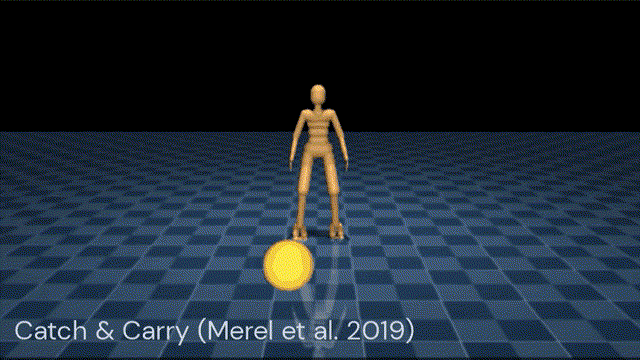
Figure 16 MuJoCo Simulator
Figure 17 MuJoCo Simulator 2
Image Source: https://www.youtube.com/watch?v=3dlM1dvBtko
6. Conclusion
References:
[1] https://raisim.com/
[2] https://pybullet.org/wordpress/
[3] http://www.ode.org/
[4] https://mujoco.org/
[5] https://dartsim.github.io/
[6] Erez, Tom, Yuval Tassa, and Emanuel Todorov. “Simulation tools for model-based robotics: Comparison of bullet, havok, mujoco, ode and physx.” 2015 IEEE international conference on robotics and automation (ICRA). IEEE, 2015.
[7] https://leggedrobotics.github.io/SimBenchmark/
[8] https://cyberbotics.com/
[9] https://www.coppeliarobotics.com/
[10] https://gazebosim.org/
[11] https://www.nvidia.com/en-us/deep-learning-ai/industries/robotics/
[12] https://drake.mit.edu/
[13] Posa, Michael, Scott Kuindersma, and Russ Tedrake. “Optimization and stabilization of trajectories for constrained dynamical systems.” 2016 IEEE International Conference on Robotics and Automation (ICRA). IEEE, 2016.
[14] https://www.mathworks.com/products/simulink.html
[15] https://pybullet.org/wordpress/
[16] Mower, Christopher, et al. “ROS-PyBullet Interface: A framework for reliable contact simulation and human-robot interaction.” Conference on Robot Learning. PMLR, 2023.
[17] Todorov, Emanuel. “Convex and analytically-invertible dynamics with contacts and constraints: Theory and implementation in mujoco.” 2014 IEEE International Conference on Robotics and Automation (ICRA). IEEE, 2014.
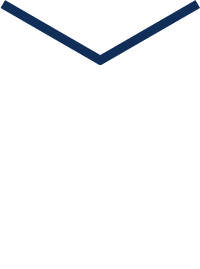
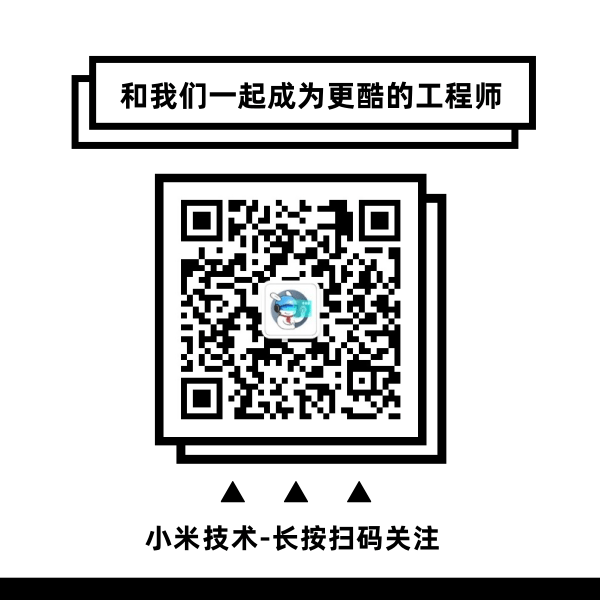